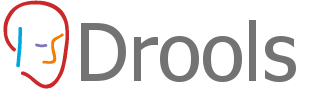
Welcome
1. Introduction
1.1. Introduction
KIE (Knowledge Is Everything) is an umbrella project introduced to bring our related technologies together under one roof. It also acts as the core shared between our projects.
KIE contains the following different but related projects offering a complete portfolio of solutions for business automation and management:
-
Drools is a business-rule management system with a forward-chaining and backward-chaining inference-based rules engine, allowing fast and reliable evaluation of business rules and complex event processing. A rules engine is also a fundamental building block to create an expert system which, in artificial intelligence, is a computer system that emulates the decision-making ability of a human expert.
-
jBPM is a flexible Business Process Management suite allowing you to model your business goals by describing the steps that need to be executed to achieve those goals.
-
OptaPlanner is a constraint solver that optimizes use cases such as employee rostering, vehicle routing, task assignment and cloud optimization.
-
Business Central is is a full featured web application for the visual composition of custom business rules and processes.
-
UberFire is a web-based workbench framework inspired by Eclipse Rich Client Platform.
The 7.x series will follow a more agile approach with more regular and iterative releases. We plan to do some bigger changes than normal for a series of minor releases, and users need to be aware those are coming before adopting.
-
UI sections and links will become object oriented, rather than task oriented. https://en.wikipedia.org/wiki/Object-oriented_user_interface
-
Authoring/Library will become project oriented, rather than repository oriented. You’ll create, browse and open projects rather than repositories. The repository concept will be pushed lower, for instance it’ll be created automaticaly when you create the projcet.
-
The old form modeller will be removed and only the new one made available. Although old forms will continue to render.
-
The new designer will continue to mature with more nodes and improved UXD. Eventually it’ll become the default editor, but we will not remove the old one until there is feature parity in BPMN2 support.
-
Continued UXD improvements in lots of places.
-
We will introduce the AppFormer project, this will be a re-org and consolidation of existing projects and result in some artifact renames. UberFire will become AppFormer-Core, forms, data modeller and dashbuilder will come under AppFormer. Dashbuilder will most likely becalled Appformer-Insight.
The 8.x series will come towards the end of this year. We have ongoing parallel work to introduce concepts of workspaces with improved git support, that will have a built in workflow for forking and pull requests. This will be combined with horizontal scaling and improved high availability. These changes are important for usability and cloud scalability, but too much of a change for a minor release, hence the bump to 8.x
1.2. Getting Involved
We are often asked "How do I get involved". Luckily the answer is simple, just write some code and submit it :) There are no hoops you have to jump through or secret handshakes. We have a very minimal "overhead" that we do request to allow for scalable project development. Below we provide a general overview of the tools and "workflow" we request, along with some general advice.
If you contribute some good work, don’t forget to blog about it :)
1.2.1. Sign up to jboss.org
Signing to jboss.org will give you access to the JBoss wiki, forums and JIRA. Go to https://www.jboss.org/ and click "Register".
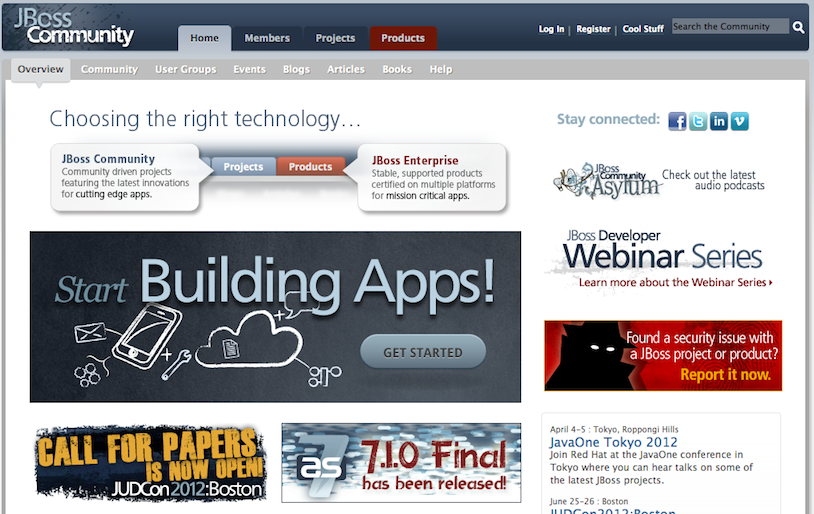
1.2.2. Sign the Contributor Agreement
The only form you need to sign is the contributor agreement, which is fully automated via the web. As the image below says "This establishes the terms and conditions for your contributions and ensures that source code can be licensed appropriately"
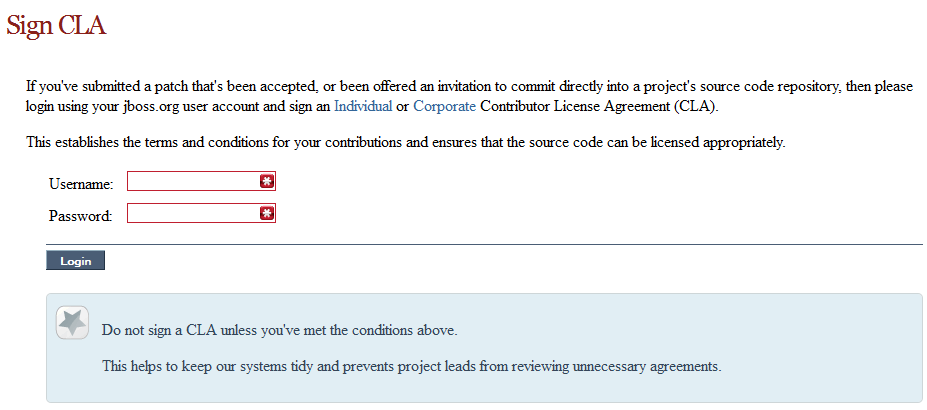
1.2.3. Submitting issues via JIRA
To be able to interact with the core development team you will need to use JIRA, the issue tracker. This ensures that all requests are logged and allocated to a release schedule and all discussions captured in one place. Bug reports, bug fixes, feature requests and feature submissions should all go here. General questions should be undertaken at the mailing lists.
Minor code submissions, like format or documentation fixes do not need an associated JIRA issue created.
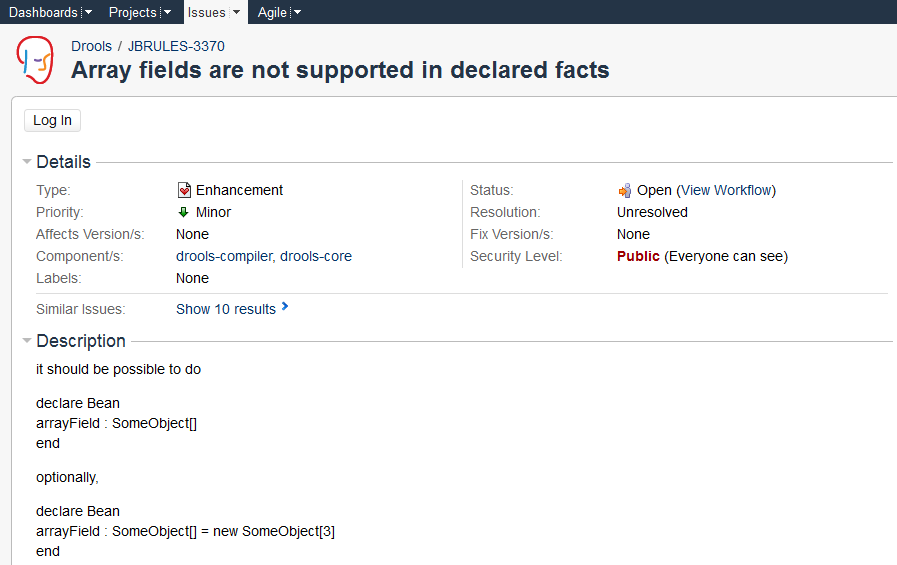
1.2.4. Fork GitHub
With the contributor agreement signed and your requests submitted to JIRA you should now be ready to code :) Create a GitHub account and fork any of the Drools, jBPM or Guvnor repositories. The fork will create a copy in your own GitHub space which you can work on at your own pace. If you make a mistake, don’t worry blow it away and fork again. Note each GitHub repository provides you the clone (checkout) URL, GitHub will provide you URLs specific to your fork.
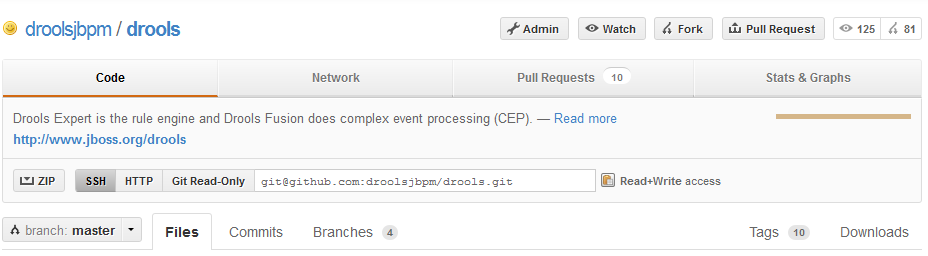
1.2.5. Writing Tests
When writing tests, try and keep them minimal and self contained. We prefer to keep the DRL fragments within the test, as it makes for quicker reviewing. If their are a large number of rules then using a String is not practical so then by all means place them in separate DRL files instead to be loaded from the classpath. If your tests need to use a model, please try to use those that already exist for other unit tests; such as Person, Cheese or Order. If no classes exist that have the fields you need, try and update fields of existing classes before adding a new class.
There are a vast number of tests to look over to get an idea, MiscTest is a good place to start.
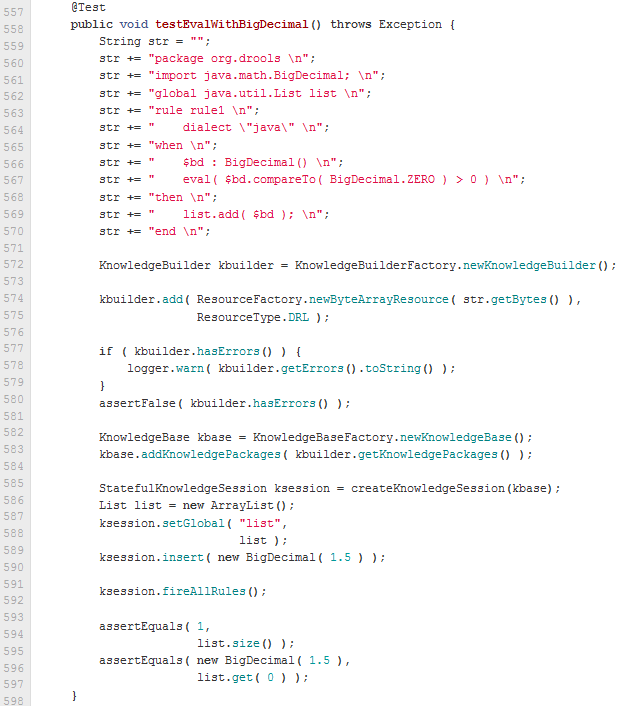
1.2.6. Commit with Correct Conventions
When you commit, make sure you use the correct conventions. The commit must start with the JIRA issue id, such as DROOLS-1946. This ensures the commits are cross referenced via JIRA, so we can see all commits for a given issue in the same place. After the id the title of the issue should come next. Then use a newline, indented with a dash, to provide additional information related to this commit. Use an additional new line and dash for each separate point you wish to make. You may add additional JIRA cross references to the same commit, if it’s appropriate. In general try to avoid combining unrelated issues in the same commit.
Don’t forget to rebase your local fork from the original master and then push your commits back to your fork.
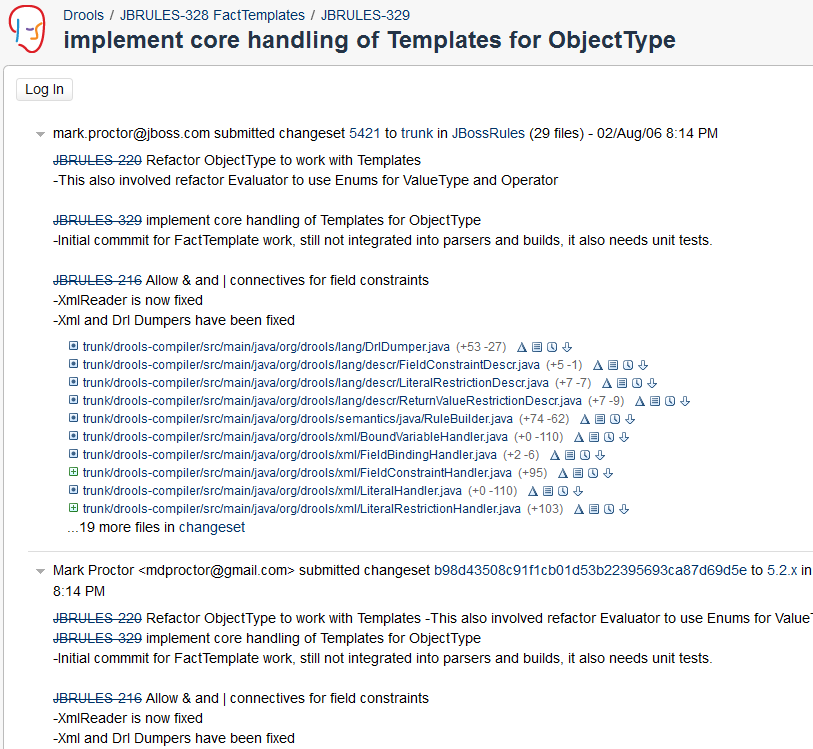
1.2.7. Submit Pull Requests
With your code rebased from original master and pushed to your personal GitHub area, you can now submit your work as a pull request. If you look at the top of the page in GitHub for your work area their will be a "Pull Request" button. Selecting this will then provide a gui to automate the submission of your pull request.
The pull request then goes into a queue for everyone to see and comment on. Below you can see a typical pull request. The pull requests allow for discussions and it shows all associated commits and the diffs for each commit. The discussions typically involve code reviews which provide helpful suggestions for improvements, and allows for us to leave inline comments on specific parts of the code. Don’t be disheartened if we don’t merge straight away, it can often take several revisions before we accept a pull request. Luckily GitHub makes it very trivial to go back to your code, do some more commits and then update your pull request to your latest and greatest.
It can take time for us to get round to responding to pull requests, so please be patient. Submitted tests that come with a fix will generally be applied quite quickly, where as just tests will often way until we get time to also submit that with a fix. Don’t forget to rebase and resubmit your request from time to time, otherwise over time it will have merge conflicts and core developers will general ignore those.
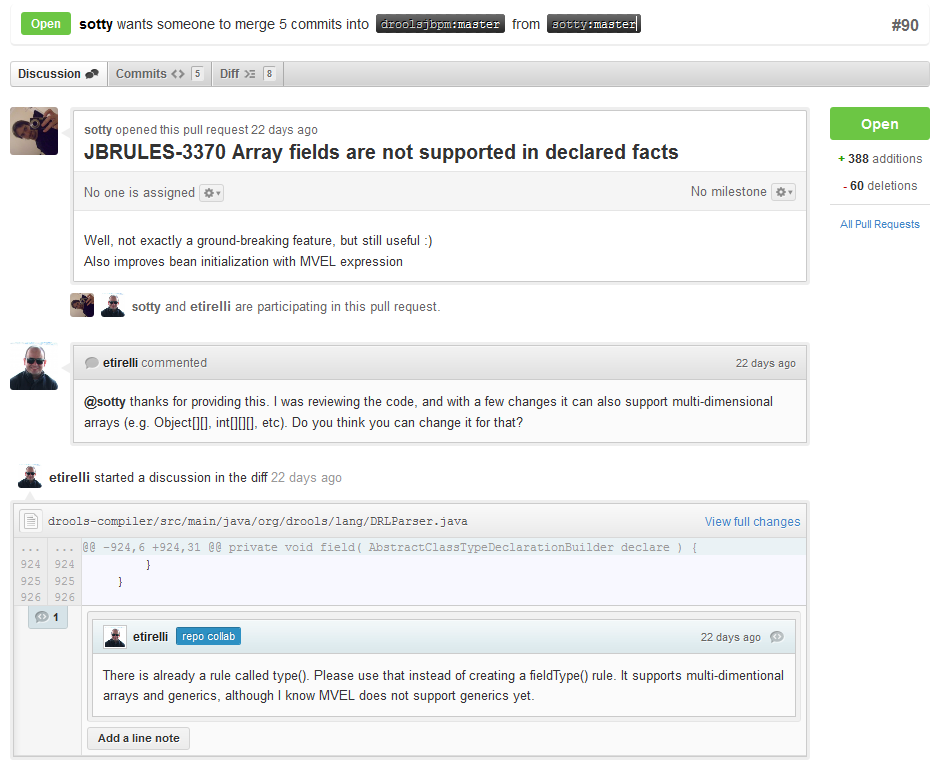
1.3. Installation and Setup (Core and IDE)
1.3.1. Installing and using
Drools provides an Eclipse-based IDE (which is optional), but at its core only Java 1.5 (Java SE) is required.
A simple way to get started is to download and install the Eclipse plug-in - this will also require the Eclipse GEF framework to be installed (see below, if you don’t have it installed already). This will provide you with all the dependencies you need to get going: you can simply create a new rule project and everything will be done for you. Refer to the chapter on Business Central and IDE for detailed instructions on this. Installing the Eclipse plug-in is generally as simple as unzipping a file into your Eclipse plug-in directory.
Use of the Eclipse plug-in is not required. Rule files are just textual input (or spreadsheets as the case may be) and the IDE (also known as Business Central) is just a convenience. People have integrated the Drools engine in many ways, there is no "one size fits all".
Alternatively, you can download the binary distribution, and include the relevant JARs in your projects classpath.
1.3.1.1. Dependencies and JARs
Drools is broken down into a few modules, some are required during rule development/compiling, and some are required at runtime. In many cases, people will simply want to include all the dependencies at runtime, and this is fine. It allows you to have the most flexibility. However, some may prefer to have their "runtime" stripped down to the bare minimum, as they will be deploying rules in binary form - this is also possible. The core Drools engine can be quite compact, and only requires a few 100 kilobytes across 3 JAR files.
The following is a description of the important libraries that make up JBoss Drools
-
knowledge-api.jar - this provides the interfaces and factories. It also helps clearly show what is intended as a user API and what is just an engine API.
-
knowledge-internal-api.jar - this provides internal interfaces and factories.
-
drools-core.jar - this is the core Drools engine, runtime component. Contains both the RETE engine and the LEAPS engine. This is the only runtime dependency if you are pre-compiling rules (and deploying via Package or RuleBase objects).
-
drools-compiler.jar - this contains the compiler/builder components to take rule source, and build executable rule bases. This is often a runtime dependency of your application, but it need not be if you are pre-compiling your rules. This depends on drools-core.
-
drools-jsr94.jar - this is the JSR-94 compliant implementation, this is essentially a layer over the drools-compiler component. Note that due to the nature of the JSR-94 specification, not all features are easily exposed via this interface. In some cases, it will be easier to go direct to the Drools API, but in some environments the JSR-94 is mandated.
-
drools-decisiontables.jar - this is the decision tables 'compiler' component, which uses the drools-compiler component. This supports both excel and CSV input formats.
There are quite a few other dependencies which the above components require, most of which are for the drools-compiler, drools-jsr94 or drools-decisiontables module. Some key ones to note are "POI" which provides the spreadsheet parsing ability, and "antlr" which provides the parsing for the rule language itself.
if you are using Drools in J2EE or servlet containers and you come across classpath issues with "JDT", then you can switch to the janino compiler. Set the system property "drools.compiler": For example: -Ddrools.compiler=JANINO. |
For up to date info on dependencies in a release, consult the released POMs, which can be found on the Maven repository.
1.3.1.2. Use with Maven, Gradle, Ivy, Buildr or Ant
The JARs are also available in the central Maven repository (and also in https://repository.jboss.org/nexus/index.html#nexus-search;gavorg.drools~[the JBoss Maven repository]).
If you use Maven, add KIE and Drools dependencies in your project’s pom.xml like this:
<dependencyManagement>
<dependencies>
<dependency>
<groupId>org.drools</groupId>
<artifactId>drools-bom</artifactId>
<type>pom</type>
<version>...</version>
<scope>import</scope>
</dependency>
...
</dependencies>
</dependencyManagement>
<dependencies>
<dependency>
<groupId>org.kie</groupId>
<artifactId>kie-api</artifactId>
</dependency>
<dependency>
<groupId>org.drools</groupId>
<artifactId>drools-compiler</artifactId>
<scope>runtime</scope>
</dependency>
...
<dependencies>
This is similar for Gradle, Ivy and Buildr. To identify the latest version, check the Maven repository.
If you’re still using Ant (without Ivy), copy all the JARs from the download zip’s binaries directory and manually verify that your classpath doesn’t contain duplicate JARs.
1.3.1.3. Runtime
The "runtime" requirements mentioned here are if you are deploying rules as their binary form (either as KnowledgePackage objects, or KnowledgeBase objects etc). This is an optional feature that allows you to keep your runtime very light. You may use drools-compiler to produce rule packages "out of process", and then deploy them to a runtime system. This runtime system only requires drools-core.jar and knowledge-api for execution. This is an optional deployment pattern, and many people do not need to "trim" their application this much, but it is an ideal option for certain environments.
1.3.1.4. Installing IDE (Rule Workbench)
The rule workbench (for Eclipse) requires that you have Eclipse 3.4 or greater, as well as Eclipse GEF 3.4 or greater. You can install it either by downloading the plug-in or using the update site.
Another option is to use the JBoss IDE, which comes with all the plug-in requirements pre packaged, as well as a choice of other tools separate to rules. You can choose just to install rules from the "bundle" that JBoss IDE ships with.
Installing GEF (a required dependency)
GEF is the Eclipse Graphical Editing Framework, which is used for graph viewing components in the plug-in.
If you don’t have GEF installed, you can install it using the built in update mechanism (or downloading GEF from the Eclipse.org website not recommended). JBoss IDE has GEF already, as do many other "distributions" of Eclipse, so this step may be redundant for some people.
Open the Help→Software updates…→Available Software→Add Site… from the help menu. Location is:
http://download.eclipse.org/tools/gef/updates/releases/
Next you choose the GEF plug-in:
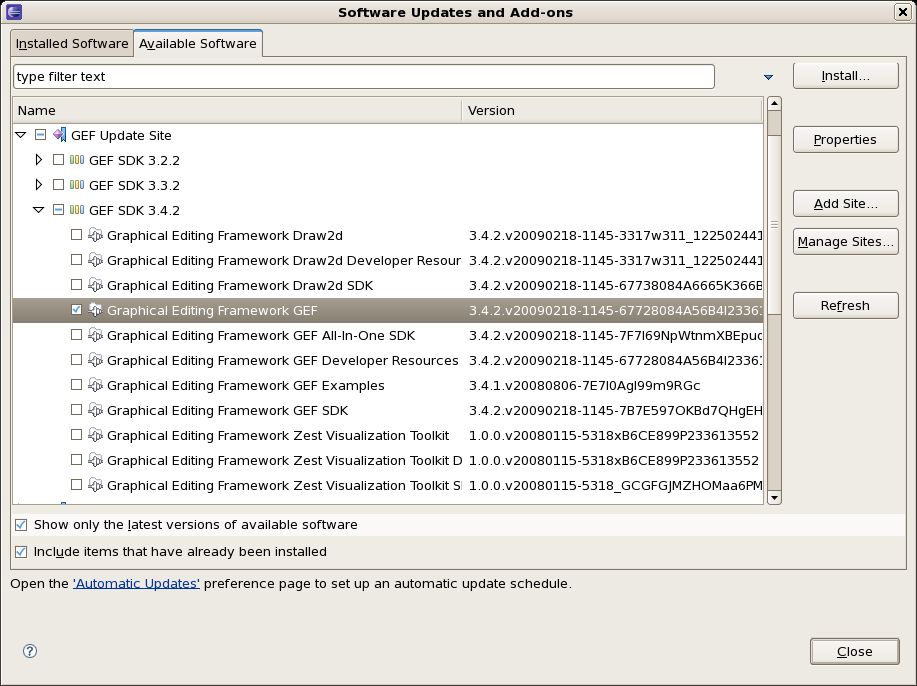
Press next, and agree to install the plug-in (an Eclipse restart may be required). Once this is completed, then you can continue on installing the rules plug-in.
Installing GEF from zip file
To install from the zip file, download and unzip the file. Inside the zip you will see a plug-in directory, and the plug-in JAR itself. You place the plug-in JAR into your Eclipse applications plug-in directory, and restart Eclipse.
Installing Drools plug-in from zip file
Download the Drools Eclipse IDE plugin from the link below. Unzip the downloaded file in your main eclipse folder (do not just copy the file there, extract it so that the feature and plugin JARs end up in the features and plugin directory of eclipse) and (re)start Eclipse.
To check that the installation was successful, try opening the Drools perspective: Click the 'Open Perspective' button in the top right corner of your Eclipse window, select 'Other…' and pick the Drools perspective. If you cannot find the Drools perspective as one of the possible perspectives, the installation probably was unsuccessful. Check whether you executed each of the required steps correctly: Do you have the right version of Eclipse (3.4.x)? Do you have Eclipse GEF installed (check whether the org.eclipse.gef_3.4..jar exists in the plugins directory in your eclipse root folder)? Did you extract the Drools Eclipse plugin correctly (check whether the org.drools.eclipse_.jar exists in the plugins directory in your eclipse root folder)? If you cannot find the problem, try contacting us (e.g. on irc or on the user mailing list), more info can be found no our homepage here:
Drools Runtimes
A Drools runtime is a collection of JARs on your file system that represent one specific release of the Drools project JARs. To create a runtime, you must point the IDE to the release of your choice. If you want to create a new runtime based on the latest Drools project JARs included in the plugin itself, you can also easily do that. You are required to specify a default Drools runtime for your Eclipse workspace, but each individual project can override the default and select the appropriate runtime for that project specifically.
You are required to define one or more Drools runtimes using the Eclipse preferences view. To open up your preferences, in the menu Window select the Preferences menu item. A new preferences dialog should show all your preferences. On the left side of this dialog, under the Drools category, select "Installed Drools runtimes". The panel on the right should then show the currently defined Drools runtimes. If you have not yet defined any runtimes, it should like something like the figure below.
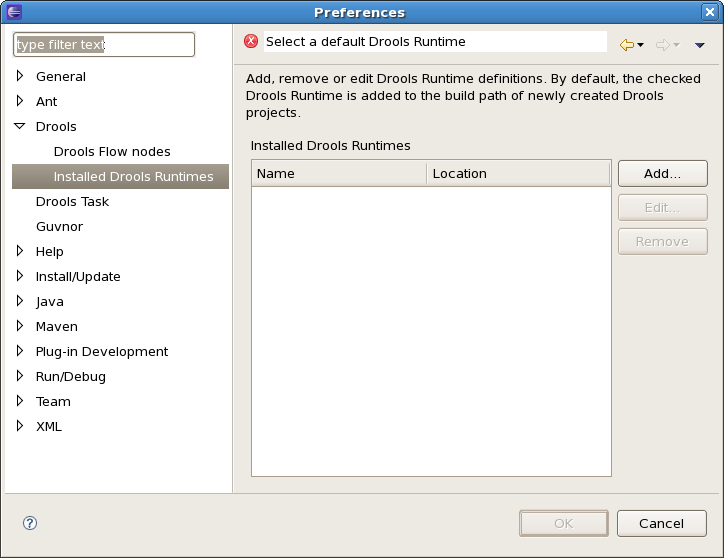
To define a new Drools runtime, click on the add button. A dialog as shown below should pop up, requiring the name for your runtime and the location on your file system where it can be found.
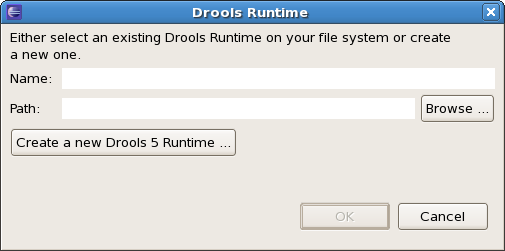
In general, you have two options:
-
If you simply want to use the default JARs as included in the Drools Eclipse plugin, you can create a new Drools runtime automatically by clicking the "Create a new Drools 5 runtime …" button. A file browser will show up, asking you to select the folder on your file system where you want this runtime to be created. The plugin will then automatically copy all required dependencies to the specified folder. After selecting this folder, the dialog should look like the figure shown below.
-
If you want to use one specific release of the Drools project, you should create a folder on your file system that contains all the necessary Drools libraries and dependencies. Instead of creating a new Drools runtime as explained above, give your runtime a name and select the location of this folder containing all the required JARs.
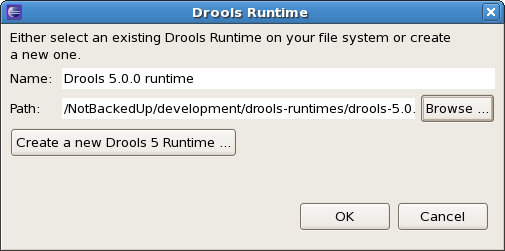
After clicking the OK button, the runtime should show up in your table of installed Drools runtimes, as shown below. Click on checkbox in front of the newly created runtime to make it the default Drools runtime. The default Drools runtime will be used as the runtime of all your Drools project that have not selected a project-specific runtime.
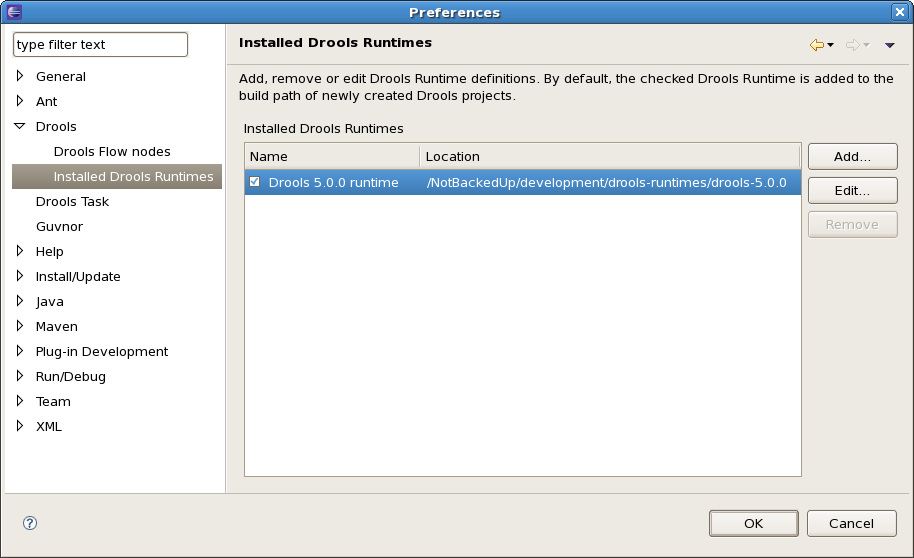
You can add as many Drools runtimes as you need. For example, the screenshot below shows a configuration where three runtimes have been defined: a Drools 4.0.7 runtime, a Drools 5.0.0 runtime and a Drools 5.0.0.SNAPSHOT runtime. The Drools 5.0.0 runtime is selected as the default one.
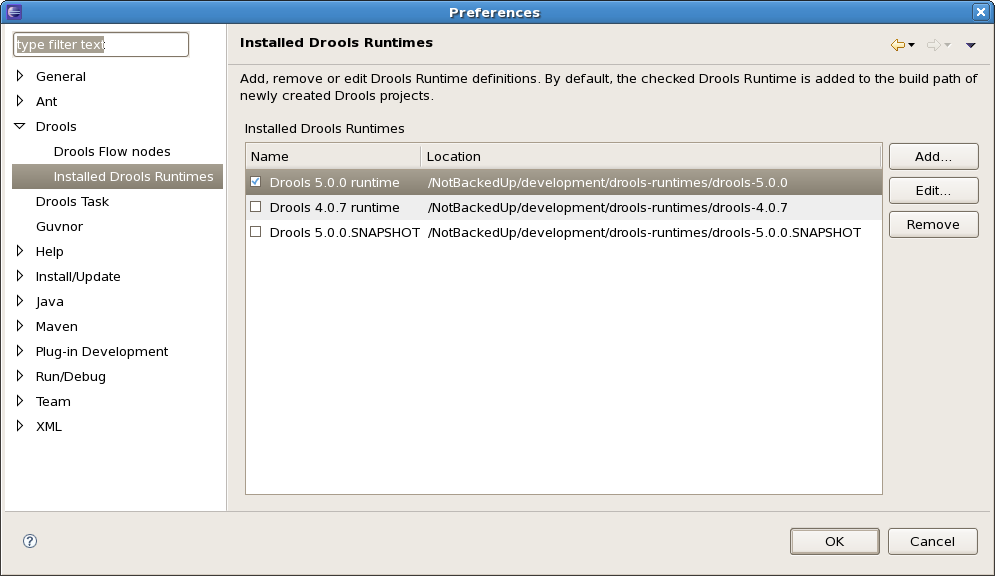
Note that you will need to restart Eclipse if you changed the default runtime and you want to make sure that all the projects that are using the default runtime update their classpath accordingly.
Whenever you create a Drools project (using the New Drools Project wizard or by converting an existing Java project to a Drools project using the "Convert to Drools Project" action that is shown when you are in the Drools perspective and you right-click an existing Java project), the plugin will automatically add all the required JARs to the classpath of your project.
When creating a new Drools project, the plugin will automatically use the default Drools runtime for that project, unless you specify a project-specific one. You can do this in the final step of the New Drools Project wizard, as shown below, by deselecting the "Use default Drools runtime" checkbox and selecting the appropriate runtime in the drop-down box. If you click the "Configure workspace settings …" link, the workspace preferences showing the currently installed Drools runtimes will be opened, so you can add new runtimes there.
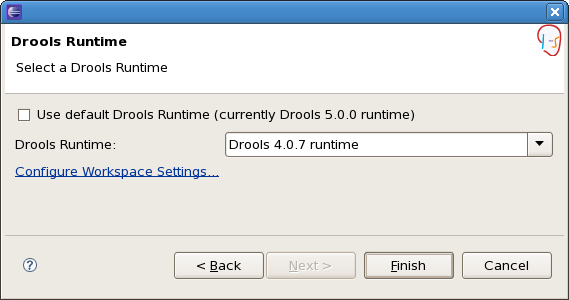
You can change the runtime of a Drools project at any time by opening the project properties (right-click the project and select Properties) and selecting the Drools category, as shown below. Check the "Enable project specific settings" checkbox and select the appropriate runtime from the drop-down box. If you click the "Configure workspace settings …" link, the workspace preferences showing the currently installed Drools runtimes will be opened, so you can add new runtimes there. If you deselect the "Enable project specific settings" checkbox, it will use the default runtime as defined in your global preferences.
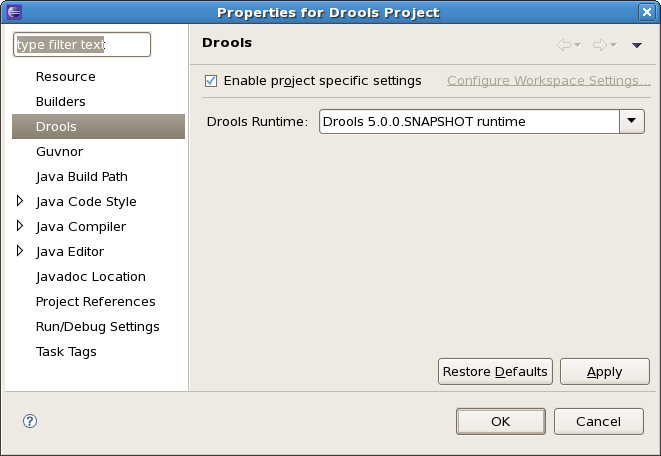
1.3.2. Building from source
1.3.2.1. Getting the sources
The source code of each Maven artifact is available in the JBoss Maven repository as a source JAR. The same source JARs are also included in the download zips. However, if you want to build from source, it’s highly recommended to get our sources from our source control.
Git allows you to fork our code, independently make personal changes on it, yet still merge in our latest changes regularly and optionally share your changes with us. To learn more about git, read the free book Git Pro.
1.3.2.2. Building the sources
In essense, building from source is very easy, for example if you want to build the guvnor project:
$ git clone git@github.com:kiegroup/guvnor.git
...
$ cd guvnor
$ mvn clean install -DskipTests -Dfull
...
However, there are a lot potential pitfalls, so if you’re serious about building from source and possibly contributing to the project, follow the instructions in the README file in droolsjbpm-build-bootstrap.
1.3.3. Eclipse
1.3.3.1. Importing Eclipse Projects
With the Eclipse project files generated they can now be imported into Eclipse. When starting Eclipse open the workspace in the root of your subversion checkout.
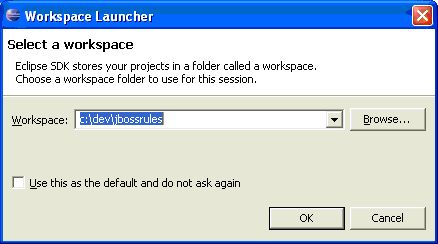
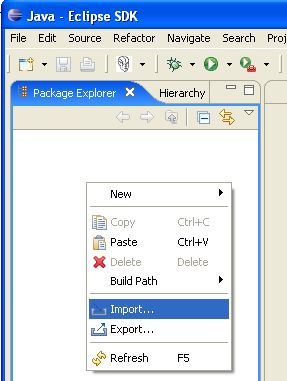
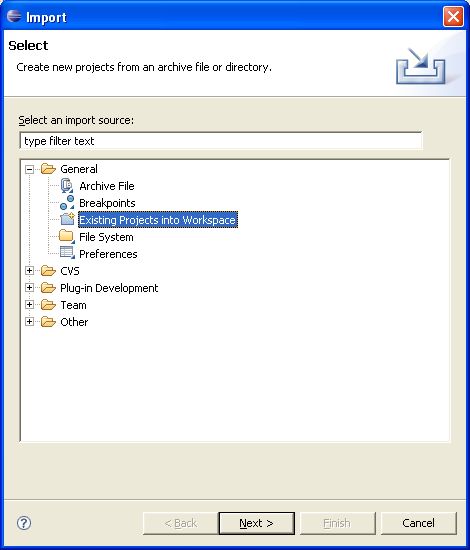
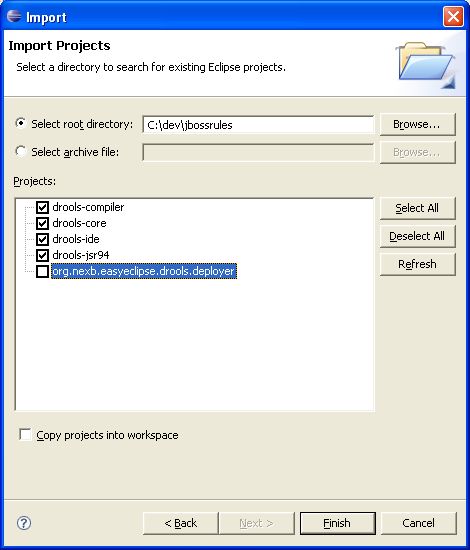
When calling mvn install
all the project dependencies were downloaded and added to the local Maven repository.
Eclipse cannot find those dependencies unless you tell it where that repository is.
To do this setup an M2_REPO
classpath variable.
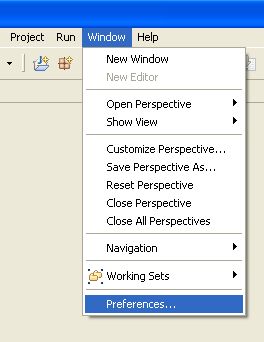
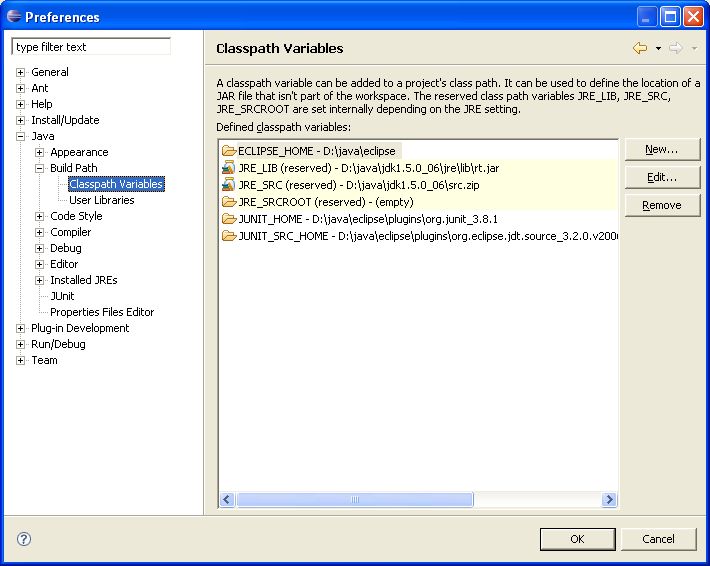
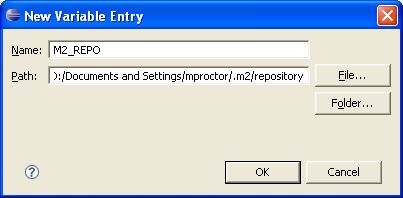
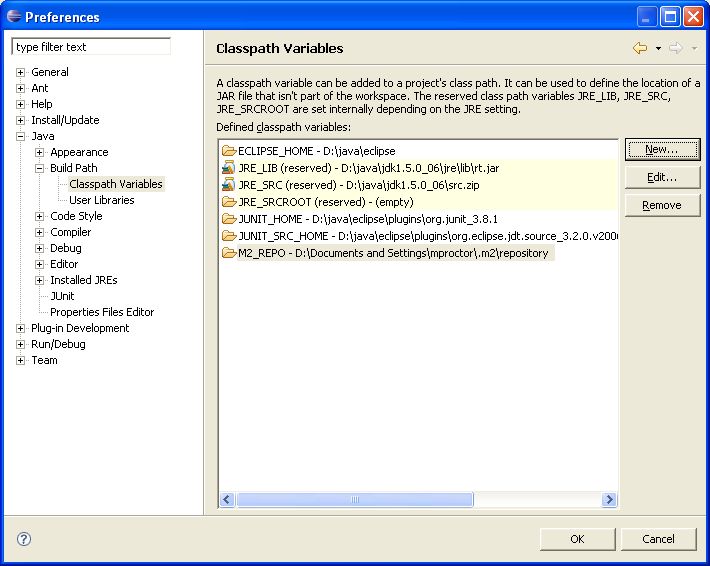
KIE
KIE is the shared core for Drools and jBPM. It provides a unified methodology and programming model for building, deploying and utilizing resources.
2. KIE
2.1. Overview
2.1.1. Anatomy of Projects
The process of researching an integration knowledge solution for Drools and jBPM has simply used the "kiegroup" group name. This name permeates GitHub accounts and Maven POMs. As scopes broadened and new projects were spun KIE, an acronym for Knowledge Is Everything, was chosen as the new group name. The KIE name is also used for the shared aspects of the system; such as the unified build, deploy and utilization.
KIE currently consists of the following subprojects:
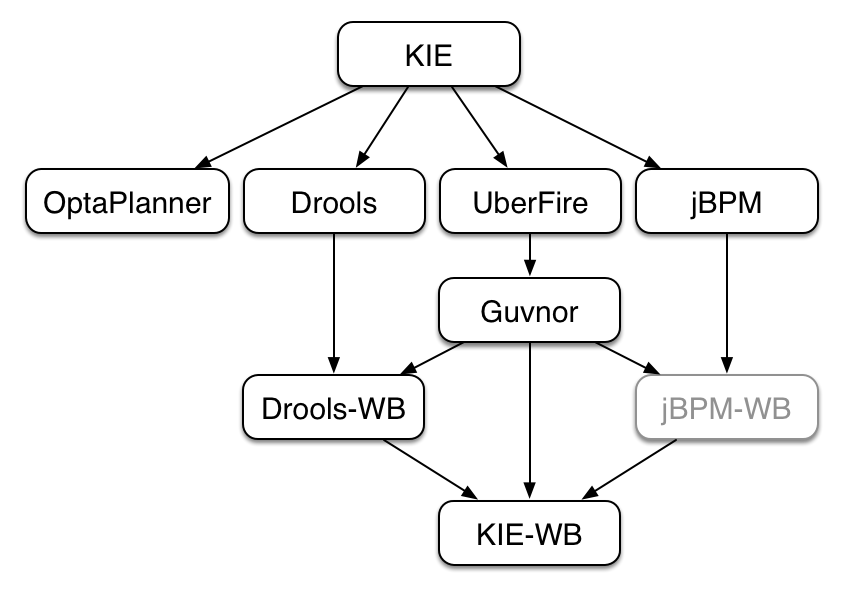
OptaPlanner, a local search and optimization tool, has been spun off from Drools Planner and is now a top level project with Drools and jBPM. This was a natural evolution as Optaplanner, while having strong Drools integration, has long been independent of Drools.
From the Polymita acquisition, along with other things, comes the powerful Dashboard Builder which provides powerful reporting capabilities. Dashboard Builder is currently a temporary name and after the 6.0 release a new name will be chosen. Dashboard Builder is completely independent of Drools and jBPM and will be used by many projects at JBoss, and hopefully outside of JBoss :)
UberFire is the new base Business Central project, spun off from the ground up rewrite. UberFire provides Eclipse-like workbench capabilities, with panels and pages from plugins. The project is independent of Drools and jBPM and anyone can use it as a basis of building flexible and powerful workbenches like Business Central. UberFire will be used for console and workbench development throughout JBoss.
It was determined that the Guvnor brand leaked too much from its intended role; such as the authoring metaphors, like Decision Tables, being considered Guvnor components instead of Drools components. This wasn’t helped by the monolithic projects structure used in 5.x for Guvnor. In 6.0 Guvnor’s focus has been narrowed to encapsulate the set of UberFire plugins that provide the basis for building a web based IDE. Such as Maven integration for building and deploying, management of Maven repositories and activity notifications via inboxes. Drools and jBPM build Business Central distributions using Uberfire as the base and including a set of plugins, such as Guvnor, along with their own plugins for things like decision tables, guided editors, BPMN2 designer, human tasks. Business Central is called business-central.
KIE-WB is the uber workbench that combined all the Guvnor, Drools and jBPM plugins. The jBPM-WB is ghosted out, as it doesn’t actually exist, being made redundant by KIE-WB.
2.1.2. Lifecycles
The different aspects, or life cycles, of working with KIE system, whether it’s Drools or jBPM, can typically be broken down into the following:
-
Author
-
Authoring of knowledge using a UI metaphor, such as: DRL, BPMN2, decision table, class models.
-
-
Build
-
Builds the authored knowledge into deployable units.
-
For KIE this unit is a JAR.
-
-
Test
-
Test KIE knowledge before it’s deployed to the application.
-
-
Deploy
-
Deploys the unit to a location where applications may utilize (consume) them.
-
KIE uses Maven style repository.
-
-
Utilize
-
The loading of a JAR to provide a KIE session (KieSession), for which the application can interact with.
-
KIE exposes the JAR at runtime via a KIE container (KieContainer).
-
KieSessions, for the runtime’s to interact with, are created from the KieContainer.
-
-
Run
-
System interaction with the KieSession, via API.
-
-
Work
-
User interaction with the KieSession, via command line or UI.
-
-
Manage
-
Manage any KieSession or KieContainer.
-
2.1.3. Installation environment options for Drools
With Drools, you can set up a development environment to develop business applications, a runtime environment to run those applications to support decisions, or both.
-
Development environment: Typically consists of one Business Central installation and at least one KIE Server installation. You can use Business Central to design decisions and other artifacts, and you can use KIE Server to execute and test the artifacts that you created.
-
Runtime environment: Consists of one or more KIE Server instances with or without Business Central. Business Central has an embedded Drools controller. If you install Business Central, use the Menu → Deploy → Execution servers page to create and maintain containers. If you want to automate KIE Server management without Business Central, you can use the headless Drools controller.
You can also cluster both development and runtime environments. A clustered development or runtime environment consists of a unified group or "cluster" of two or more servers. The primary benefit of clustering Drools development environments is high availability and enhanced collaboration, while the primary benefit of clustering Drools runtime environments is high availability and load balancing. High availability decreases the chance of a loss of data when a single server fails. When a server fails, another server fills the gap by providing a copy of the data that was on the failed server. When the failed server comes online again, it resumes its place in the cluster. Load balancing shares the computing load across the nodes of the cluster to improve the overall performance.
Clustering of the runtime environment is currently supported on Red Hat JBoss EAP 7.2 only. Clustering of Business Central is currently a Technology Preview feature that is not yet intended for production use. |
2.1.4. Decision-authoring assets in Drools
Drools supports several assets that you can use to define business decisions for your decision service. Each decision-authoring asset has different advantages, and you might prefer to use one or a combination of multiple assets depending on your goals and needs.
The following table highlights the main decision-authoring assets supported in Drools projects to help you decide or confirm the best method for defining decisions in your decision service.
Asset | Highlights | Authoring tools | Documentation |
---|---|---|---|
Decision Model and Notation (DMN) models |
|
Business Central or other DMN-compliant editor |
|
Guided decision tables |
|
Business Central |
|
Spreadsheet decision tables |
|
Spreadsheet editor |
|
Guided rules |
|
Business Central |
|
Guided rule templates |
|
Business Central |
|
DRL rules |
|
Business Central or integrated development environment (IDE) |
|
Predictive Model Markup Language (PMML) models |
|
PMML or XML editor |
2.1.5. Project storage and build options with Drools
As you develop a Drools project, you need to be able to track the versions of your project with a version-controlled repository, manage your project assets in a stable environment, and build your project for testing and deployment. You can use Business Central for all of these tasks, or use a combination of Business Central and external tools and repositories. Drools supports Git repositories for project version control, Apache Maven for project management, and a variety of Maven-based, Java-based, or custom-tool-based build options.
The following options are the main methods for Drools project versioning, storage, and building:
Versioning option | Description | Documentation |
---|---|---|
Business Central Git VFS |
Business Central contains a built-in Git Virtual File System (VFS) that stores all processes, rules, and other artifacts that you create in the authoring environment. Git is a distributed version control system that implements revisions as commit objects. When you commit your changes into a repository, a new commit object in the Git repository is created. When you create a project in Business Central, the project is added to the Git repository connected to Business Central. |
NA |
External Git repository |
If you have Drools projects in Git repositories outside of Business Central, you can import them into Drools spaces and use Git hooks to synchronize the internal and external Git repositories. |
NA |
Management option | Description | Documentation | ||
---|---|---|---|---|
Business Central Maven repository |
Business Central contains a built-in Maven repository that organizes and builds project assets that you create in the authoring environment. Maven is a distributed build-automation tool that uses repositories to store Java libraries, plug-ins, and other build artifacts. When building projects and archetypes, Maven dynamically retrieves Java libraries and Maven plug-ins from local or remote repositories to promote shared dependencies across projects.
|
|||
External Maven repository |
If you have Drools projects in an external Maven repository, such as Nexus or Artifactory, you can create a |
Build option | Description | Documentation |
---|---|---|
Business Central (KJAR) |
Business Central builds Drools projects stored in either the built-in Maven repository or a configured external Maven repository. Projects in Business Central are packaged automatically as knowledge JAR (KJAR) files with all components needed for deployment when you build the projects. |
|
Standalone Maven project (KJAR) |
If you have a standalone Drools Maven project outside of Business Central, you can edit the project |
|
Embedded Java application (KJAR) |
If you have an embedded Java application from which you want to build your Drools project, you can use a |
|
CI/CD tool (KJAR) |
If you use a tool for continuous integration and continuous delivery (CI/CD), you can configure the tool set to integrate with your Drools Git repositories to build a specified project. Ensure that your projects are packaged and built as KJAR files to ensure optimal deployment. |
NA |
2.1.6. Project deployment options with Drools
After you develop, test, and build your Drools project, you can deploy the project to begin using the business assets you have created. You can deploy a Drools project to a configured KIE Server, to an embedded Java application, or into a Red Hat OpenShift Container Platform environment for an enhanced containerized implementation.
The following options are the main methods for Drools project deployment:
Deployment option | Description | Documentation |
---|---|---|
Deployment to KIE Server |
KIE Server is the server provided with Drools that runs the decision services, process applications, and other deployable assets from a packaged and deployed Drools project (KJAR file). These services are consumed at run time through an instantiated KIE container, or deployment unit. You can deploy and maintain deployment units in KIE Server using Business Central or using a headless Drools controller with its associated REST API (considered a managed KIE Server instance). You can also deploy and maintain deployment units using the KIE Server REST API or Java client API from a standalone Maven project, an embedded Java application, or other custom environment (considered an unmanaged KIE Server instance). |
|
Deployment to an embedded Java application |
If you want to deploy Drools projects to your own Java virtual machine (JVM) environment, microservice, or application server, you can bundle the application resources in the project WAR files to create a deployment unit similar to a KIE container. You can also use the core KIE APIs (not KIE Server APIs) to configure a KIE scanner to periodically update KIE containers. |
2.1.7. Asset execution options with Drools
After you build and deploy your Drools project to KIE Server or other environment, you can execute the deployed assets for testing or for runtime consumption. You can also execute assets locally in addition to or instead of executing them after deployment.
The following options are the main methods for Drools asset execution:
Execution option | Description | Documentation |
---|---|---|
Execution in KIE Server |
If you deployed Drools project assets to KIE Server, you can use the KIE Server REST API or Java client API to execute and interact with the deployed assets. You can also use Business Central or the headless Drools controller outside of Business Central to manage the configurations and KIE containers in the KIE Server instances associated with your deployed assets. |
|
Execution in an embedded Java application |
If you deployed Drools project assets in your own Java virtual machine (JVM) environment, microservice, or application server, you can use custom APIs or application interactions with core KIE APIs (not KIE Server APIs) to execute assets in the embedded engine. |
|
Execution in a local environment for extended testing |
As part of your development cycle, you can execute assets locally to ensure that the assets you have created in Drools function as intended. You can use local execution in addition to or instead of executing assets after deployment. |
Smart Router (KIE Server router)
Depending on your deployment and execution environment, you can use a Smart Router to aggregate multiple independent KIE Server instances as though they are a single server. Smart Router is a single endpoint that can receive calls from client applications to any of your services and route each call automatically to the KIE Server that runs the service. For more information about Smart Router, see KIE Server router. |
2.1.8. Example decision management architectures with Drools
The following scenarios illustrate common variations of Drools installation, asset authoring, project storage, project deployment, and asset execution in a decision management architecture. Each section summarizes the methods and tools used and the advantages for the given architecture. The examples are basic and are only a few of the many combinations you might consider, depending on your specific goals and needs with Drools.
- Drools on Wildfly with Business Central and KIE Server
-
-
Installation environment: Drools on Wildfly
-
Project storage and build environment: External Git repository for project versioning synchronized with the Business Central Git repository using Git hooks, and external Maven repository for project management and building configured with KIE Server
-
Asset-authoring tool: Business Central
-
Main asset types: Decision Model and Notation (DMN) models for decisions
-
Project deployment and execution environment: KIE Server
-
Scenario advantages:
-
Stable implementation of Drools in an on-premise development environment
-
Access to the repositories, assets, asset designers, and project build options in Business Central
-
Standardized asset-authoring approach using DMN for optimal integration and stability
-
Access to KIE Server functionality and KIE APIs for asset deployment and execution
-
Figure 2. Drools on Wildfly with Business Central and KIE Server -
- Drools on Wildfly with an IDE and KIE Server
-
-
Installation environment: Drools on Wildfly
-
Project storage and build environment: External Git repository for project versioning (not synchronized with Business Central) and external Maven repository for project management and building configured with KIE Server
-
Asset-authoring tools: Integrated development environment (IDE), such as Eclipse, and a spreadsheet editor or a Decision Model and Notation (DMN) modeling tool for other decision formats
-
Main asset types: Drools Rule Language (DRL) rules, spreadsheet decision tables, and Decision Model and Notation (DMN) models for decisions
-
Project deployment and execution environment: KIE Server
-
Scenario advantages:
-
Flexible implementation of Drools in an on-premise development environment
-
Ability to define business assets using an external IDE and other asset-authoring tools of your choice
-
Access to KIE Server functionality and KIE APIs for asset deployment and execution
-
Figure 3. Drools on Wildfly with an IDE and KIE Server -
- Drools with an IDE and an embedded Java application
-
-
Installation environment: Drools libraries embedded within a custom application
-
Project storage and build environment: External Git repository for project versioning (not synchronized with Business Central) and external Maven repository for project management and building configured with your embedded Java application (not configured with KIE Server)
-
Asset-authoring tools: Integrated development environment (IDE), such as Eclipse, and a spreadsheet editor or a Decision Model and Notation (DMN) modeling tool for other decision formats
-
Main asset types: Drools Rule Language (DRL) rules, spreadsheet decision tables, and Decision Model and Notation (DMN) models for decisions
-
Project deployment and execution environment: Embedded Java application, such as in a Java virtual machine (JVM) environment, microservice, or custom application server
-
Scenario advantages:
-
Custom implementation of Drools in an on-premise development environment with an embedded Java application
-
Ability to define business assets using an external IDE and other asset-authoring tools of your choice
-
Use of custom APIs to interact with core KIE APIs (not KIE Server APIs) and to execute assets in the embedded engine
-
Figure 4. Drools with an IDE and an embedded Java application -
2.2. Build, Deploy, Utilize and Run
2.2.1. Introduction
6.0 introduces a new configuration and convention approach to building KIE bases, instead of using the programmatic builder approach in 5.x. The builder is still available to fall back on, as it’s used for the tooling integration.
Building now uses Maven, and aligns with Maven practices. A KIE project or module is simply a Maven Java project or module; with an additional metadata file META-INF/kmodule.xml. The kmodule.xml file is the descriptor that selects resources to KIE bases and configures those KIE bases and sessions. There is also alternative XML support via Spring and OSGi BluePrints.
While standard Maven can build and package KIE resources, it will not provide validation at build time. There is a Maven plugin which is recommended to use to get build time validation. The plugin also generates many classes, making the runtime loading faster too.
The example project layout and Maven POM descriptor is illustrated in the screenshot
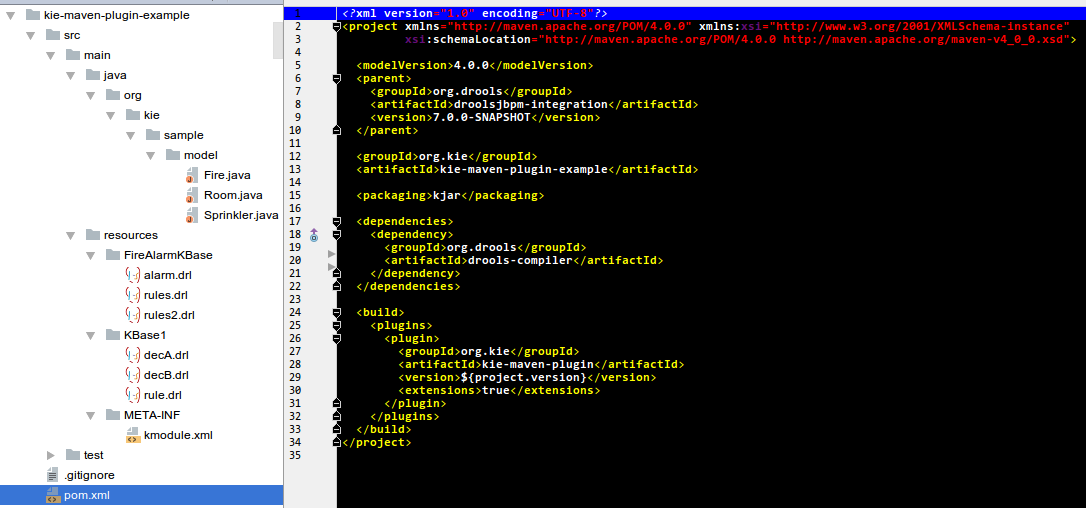
KIE uses defaults to minimise the amount of configuration. With an empty kmodule.xml being the simplest configuration. There must always be a kmodule.xml file, even if empty, as it’s used for discovery of the JAR and its contents.
Maven can either 'mvn install' to deploy a KieModule to the local machine, where all other applications on the local machine use it. Or it can 'mvn deploy' to push the KieModule to a remote Maven repository. Building the Application will pull in the KieModule and populate the local Maven repository in the process.
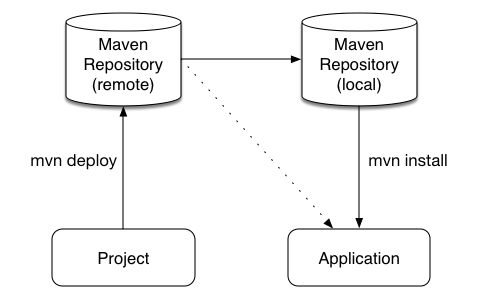
JARs can be deployed in one of two ways. Either added to the classpath, like any other JAR in a Maven dependency listing, or they can be dynamically loaded at runtime. KIE will scan the classpath to find all the JARs with a kmodule.xml in it. Each found JAR is represented by the KieModule interface. The terms classpath KieModule and dynamic KieModule are used to refer to the two loading approaches. While dynamic modules supports side by side versioning, classpath modules do not. Further once a module is on the classpath, no other version may be loaded dynamically.
Detailed references for the API are included in the next sections, the impatient can jump straight to the examples section, which is fairly self-explanatory on the different use cases.
2.2.2. Building
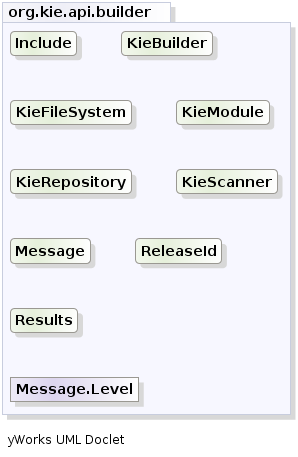
2.2.2.1. Creating and building a Kie Project
A Kie Project has the structure of a normal Maven project with the only peculiarity of including a kmodule.xml file defining in a declaratively way the KieBase
s and KieSession
s that can be created from it.
This file has to be placed in the resources/META-INF folder of the Maven project while all the other Kie artifacts, such as DRL or a Excel files, must be stored in the resources folder or in any other subfolder under it.
Since meaningful defaults have been provided for all configuration aspects, the simplest kmodule.xml file can contain just an empty kmodule tag like the following:
<?xml version="1.0" encoding="UTF-8"?>
<kmodule xmlns="http://www.drools.org/xsd/kmodule"/>
In this way the kmodule will contain one single default KieBase
.
All Kie assets stored under the resources folder, or any of its subfolders, will be compiled and added to it.
To trigger the building of these artifacts it is enough to create a KieContainer
for them.
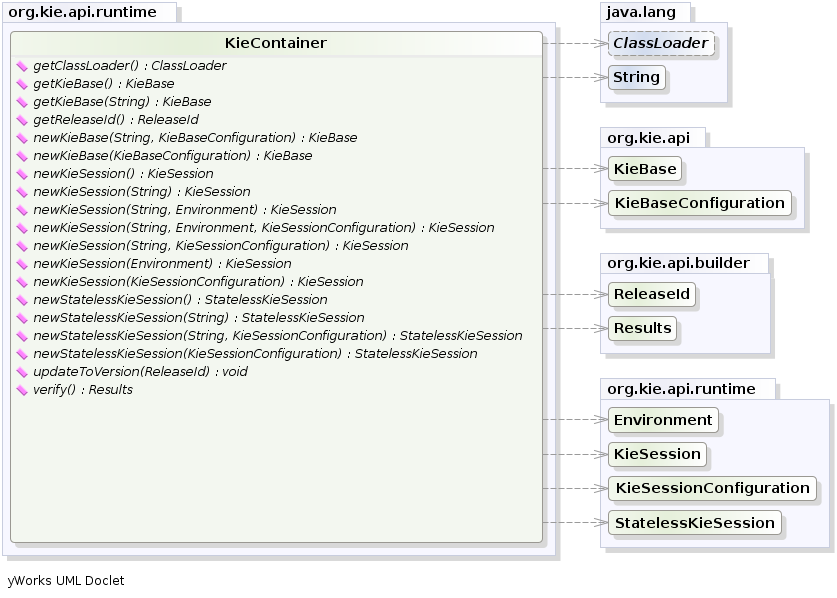
For this simple case it is enough to create a KieContainer
that reads the files to be built from the classpath:
KieServices kieServices = KieServices.Factory.get();
KieContainer kContainer = kieServices.getKieClasspathContainer();
` KieServices` is the interface from where it possible to access all the Kie building and runtime facilities:
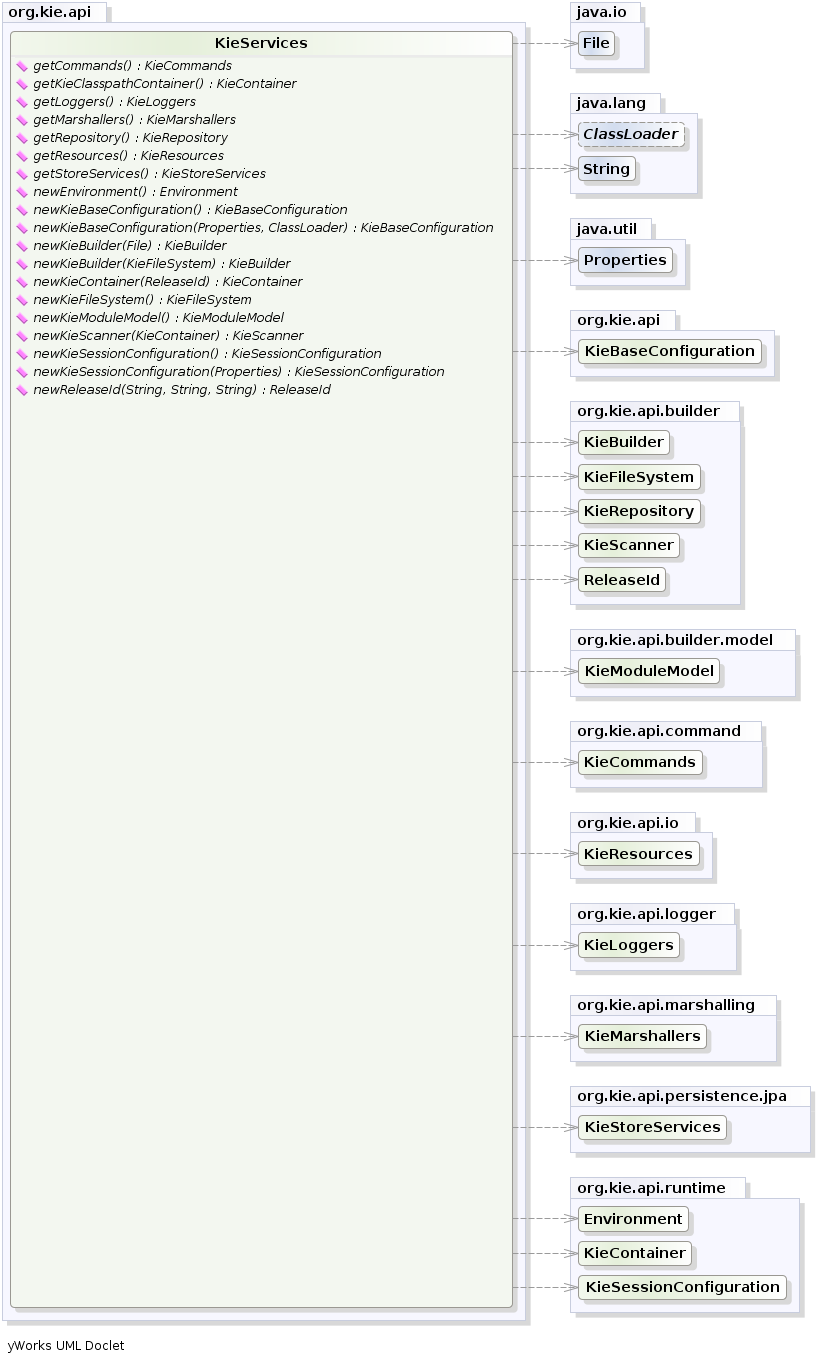
In this way all the Java sources and the Kie resources are compiled and deployed into the KieContainer which makes its contents available for use at runtime.
2.2.2.2. The kmodule.xml file
As explained in the former section, the kmodule.xml file is the place where it is possible to declaratively configure the KieBase
(s) and KieSession
(s) that can be created from a KIE project.
In particular a KieBase
is a repository of all the application’s knowledge definitions.
It will contain rules, processes, functions, and type models.
The KieBase
itself does not contain data; instead, sessions are created from the KieBase
into which data can be inserted and from which process instances may be started.
Creating the KieBase
can be heavy, whereas session creation is very light, so it is recommended that KieBase
be cached where possible to allow for repeated session creation.
However end-users usually shouldn’t worry about it, because this caching mechanism is already automatically provided by the KieContainer
.
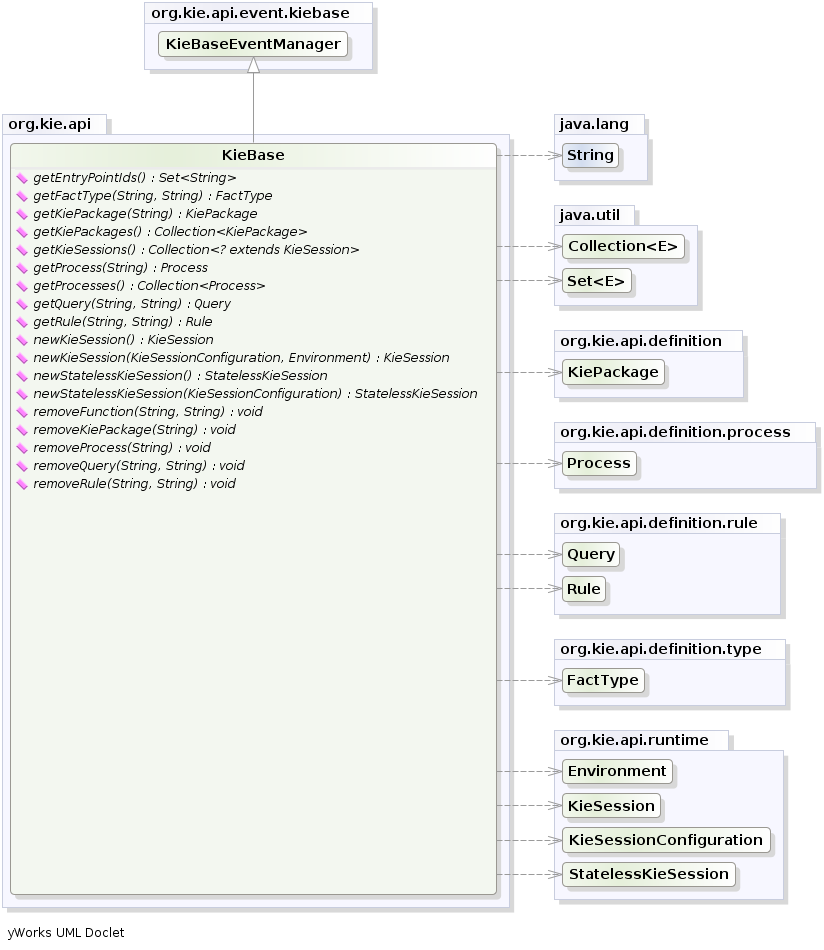
Conversely the KieSession
stores and executes on the runtime data.
It is created from the KieBase
or more easily can be created directly from the KieContainer
if it has been defined in the kmodule.xml file
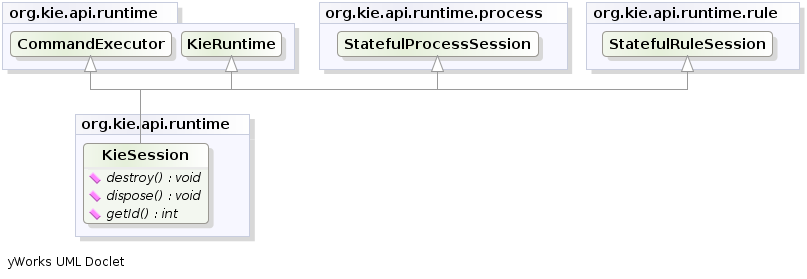
The kmodule.xml allows to define and configure one or more KieBase
s and for each KieBase
all the different KieSession
s that can be created from it, as showed by the follwing example:
<kmodule xmlns:xsi="http://www.w3.org/2001/XMLSchema-instance"
xmlns="http://www.drools.org/xsd/kmodule">
<configuration>
<property key="drools.evaluator.supersetOf" value="org.mycompany.SupersetOfEvaluatorDefinition"/>
</configuration>
<kbase name="KBase1" default="true" eventProcessingMode="cloud" equalsBehavior="equality" declarativeAgenda="enabled" packages="org.domain.pkg1">
<ksession name="KSession2_1" type="stateful" default="true"/>
<ksession name="KSession2_2" type="stateless" default="false" beliefSystem="jtms"/>
</kbase>
<kbase name="KBase2" default="false" eventProcessingMode="stream" equalsBehavior="equality" declarativeAgenda="enabled" packages="org.domain.pkg2, org.domain.pkg3" includes="KBase1">
<ksession name="KSession3_1" type="stateful" default="false" clockType="realtime">
<fileLogger file="drools.log" threaded="true" interval="10"/>
<workItemHandlers>
<workItemHandler name="name" type="org.domain.WorkItemHandler"/>
</workItemHandlers>
<calendars>
<calendar name="monday" type="org.domain.Monday"/>
</calendars>
<listeners>
<ruleRuntimeEventListener type="org.domain.RuleRuntimeListener"/>
<agendaEventListener type="org.domain.FirstAgendaListener"/>
<agendaEventListener type="org.domain.SecondAgendaListener"/>
<processEventListener type="org.domain.ProcessListener"/>
</listeners>
</ksession>
</kbase>
</kmodule>
Here the
tag contains a list of key-value pairs that are the optional properties used to configure the KieBase
s building process.
For instance this sample kmodule.xml file defines an additional custom operator named supersetOf
and implemented by the org.mycompany.SupersetOfEvaluatorDefinition
class.
After this 2 KieBase
s have been defined and it is possible to instance 2 different types of KieSession
s from the first one, while only one from the second.
A list of the attributes that can be defined on the kbase tag, together with their meaning and default values follows:
Attribute name | Default value | Admitted values | Meaning |
---|---|---|---|
name |
none |
any |
The name with which retrieve this KieBase from the KieContainer. This is the only mandatory attribute. |
includes |
none |
any comma separated list |
A comma separated list of other KieBases contained in this kmodule. The artifacts of all these KieBases will be also included in this one. |
packages |
all |
any comma separated list |
By default all the Drools artifacts under the resources folder, at any level, are included into the KieBase. This attribute allows to limit the artifacts that will be compiled in this KieBase to only the ones belonging to the list of packages. |
default |
false |
true, false |
Defines if this KieBase is the default one for this module, so it can be created from the KieContainer without passing any name to it. There can be at most one default KieBase in each module. |
equalsBehavior |
identity |
identity, equality |
Defines the behavior of Drools when a new fact is inserted into the Working Memory. With identity it always create a new FactHandle unless the same object isn’t already present in the Working Memory, while with equality only if the newly inserted object is not equal (according to its equal method) to an already existing fact. |
eventProcessingMode |
cloud |
cloud, stream |
When compiled in cloud mode the KieBase treats events as normal facts, while in stream mode allow temporal reasoning on them. |
declarativeAgenda |
disabled |
disabled, enabled |
Defines if the Declarative Agenda is enabled or not. |
Similarly all attributes of the ksession tag (except of course the name) have meaningful default. They are listed and described in the following table:
Attribute name | Default value | Admitted values | Meaning |
---|---|---|---|
name |
none |
any |
Unique name of this KieSession. Used to fetch the KieSession from the KieContainer. This is the only mandatory attribute. |
type |
stateful |
stateful, stateless |
A stateful session allows to iteratively work with the Working Memory, while a stateless one is a one-off execution of a Working Memory with a provided data set. |
default |
false |
true, false |
Defines if this KieSession is the default one for this module, so it can be created from the KieContainer without passing any name to it. In each module there can be at most one default KieSession for each type. |
clockType |
realtime |
realtime, pseudo |
Defines if events timestamps are determined by the system clock or by a psuedo clock controlled by the application. This clock is specially useful for unit testing temporal rules. |
beliefSystem |
simple |
simple, jtms, defeasible |
Defines the type of belief system used by the KieSession. |
As outlined in the former kmodule.xml sample, it is also possible to declaratively create on each KieSession
a file (or a console) logger, one or more WorkItemHandler
s and Calendar
s plus some listeners that can be of 3 different types: ruleRuntimeEventListener, agendaEventListener and processEventListener
Having defined a kmodule.xml like the one in the former sample, it is now possible to simply retrieve the KieBases and KieSessions from the KieContainer using their names.
KieServices kieServices = KieServices.Factory.get();
KieContainer kContainer = kieServices.getKieClasspathContainer();
KieBase kBase1 = kContainer.getKieBase("KBase1");
KieSession kieSession1 = kContainer.newKieSession("KSession2_1");
StatelessKieSession kieSession2 = kContainer.newStatelessKieSession("KSession2_2");
It has to be noted that since KSession2_1 and KSession2_2 are of 2 different types (the first is stateful, while the second is stateless) it is necessary to invoke 2 different methods on the KieContainer
according to their declared type.
If the type of the KieSession
requested to the KieContainer
doesn’t correspond with the one declared in the kmodule.xml file the KieContainer
will throw a RuntimeException
.
Also since a KieBase
and a KieSession
have been flagged as default is it possible to get them from the KieContainer
without passing any name.
KieContainer kContainer = ...
KieBase kBase1 = kContainer.getKieBase(); // returns KBase1
KieSession kieSession1 = kContainer.newKieSession(); // returns KSession2_1
Since a Kie project is also a Maven project the groupId, artifactId and version declared in the pom.xml file are used to generate a ReleaseId
that uniquely identifies this project inside your application.
This allows creation of a new KieContainer from the project by simply passing its ReleaseId
to the KieServices
.
KieServices kieServices = KieServices.Factory.get();
ReleaseId releaseId = kieServices.newReleaseId( "org.acme", "myartifact", "1.0" );
KieContainer kieContainer = kieServices.newKieContainer( releaseId );
2.2.2.3. Building with Maven
The KIE plugin for Maven ensures that artifact resources are validated and pre-compiled, it is recommended that this is used at all times.
To use the plugin simply add it to the build section of the Maven pom.xml and activate it by using packaging kjar
.
<packaging>kjar</packaging>
...
<build>
<plugins>
<plugin>
<groupId>org.kie</groupId>
<artifactId>kie-maven-plugin</artifactId>
<version>7.23.0.Final</version>
<extensions>true</extensions>
</plugin>
</plugins>
</build>
The plugin comes with support for all the Drools/jBPM knowledge resources.
However, in case you are using specific KIE annotations in your Java classes, like for example @kie.api.Position
, you will need to add compile time dependency on kie-api
into your project.
We recommend to use the provided scope for all the additional KIE dependencies.
That way the kjar stays as lightweight as possible, and not dependant on any particular KIE version.
Building a KIE module without the Maven plugin will copy all the resources, as is, into the resulting JAR. When that JAR is loaded by the runtime, it will attempt to build all the resources then. If there are compilation issues it will return a null KieContainer. It also pushes the compilation overhead to the runtime. In general this is not recommended, and the Maven plugin should always be used.
2.2.2.4. Defining a KieModule programmatically
It is also possible to define the KieBase
s and KieSession
s belonging to a KieModule programmatically instead of the declarative definition in the kmodule.xml file.
The same programmatic API also allows in explicitly adding the file containing the Kie artifacts instead of automatically read them from the resources folder of your project.
To do that it is necessary to create a KieFileSystem
, a sort of virtual file system, and add all the resources contained in your project to it.
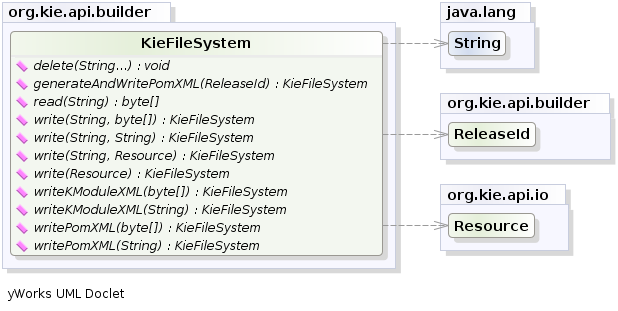
Like all other Kie core components you can obtain an instance of the KieFileSystem
from the KieServices
.
The kmodule.xml configuration file must be added to the filesystem.
This is a mandatory step.
Kie also provides a convenient fluent API, implemented by the KieModuleModel
, to programmatically create this file.
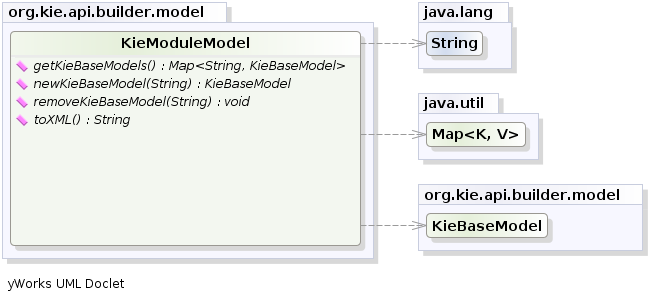
To do this in practice it is necessary to create a KieModuleModel
from the KieServices
, configure it with the desired KieBase
s and KieSession
s, convert it in XML and add the XML to the KieFileSystem
.
This process is shown by the following example:
KieServices kieServices = KieServices.Factory.get();
KieModuleModel kieModuleModel = kieServices.newKieModuleModel();
KieBaseModel kieBaseModel1 = kieModuleModel.newKieBaseModel( "KBase1 ")
.setDefault( true )
.setEqualsBehavior( EqualityBehaviorOption.EQUALITY )
.setEventProcessingMode( EventProcessingOption.STREAM );
KieSessionModel ksessionModel1 = kieBaseModel1.newKieSessionModel( "KSession1" )
.setDefault( true )
.setType( KieSessionModel.KieSessionType.STATEFUL )
.setClockType( ClockTypeOption.get("realtime") );
KieFileSystem kfs = kieServices.newKieFileSystem();
kfs.writeKModuleXML(kieModuleModel.toXML());
At this point it is also necessary to add to the KieFileSystem
, through its fluent API, all others Kie artifacts composing your project.
These artifacts have to be added in the same position of a corresponding usual Maven project.
KieFileSystem kfs = ...
kfs.write( "src/main/resources/KBase1/ruleSet1.drl", stringContainingAValidDRL )
.write( "src/main/resources/dtable.xls",
kieServices.getResources().newInputStreamResource( dtableFileStream ) );
This example shows that it is possible to add the Kie artifacts both as plain Strings and as Resource
s.
In the latter case the Resource
s can be created by the KieResources
factory, also provided by the KieServices
.
The KieResources
provides many convenient factory methods to convert an InputStream
, a URL
, a File
, or a String
representing a path of your file system to a Resource
that can be managed by the KieFileSystem
.
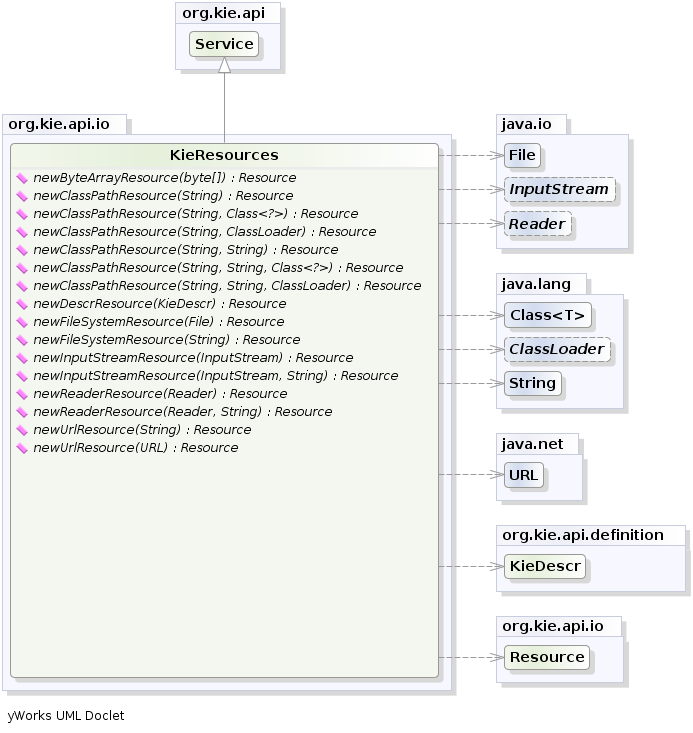
Normally the type of a Resource
can be inferred from the extension of the name used to add it to the KieFileSystem
.
However it also possible to not follow the Kie conventions about file extensions and explicitly assign a specific ResourceType
to a Resource
as shown below:
KieFileSystem kfs = ...
kfs.write( "src/main/resources/myDrl.txt",
kieServices.getResources().newInputStreamResource( drlStream )
.setResourceType(ResourceType.DRL) );
Add all the resources to the KieFileSystem
and build it by passing the KieFileSystem
to a KieBuilder
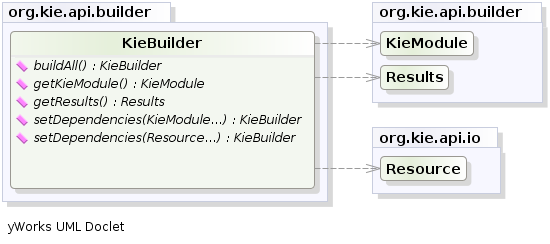
When the contents of a KieFileSystem
are successfully built, the resulting KieModule
is automatically added to the KieRepository
.
The KieRepository
is a singleton acting as a repository for all the available KieModule
s.
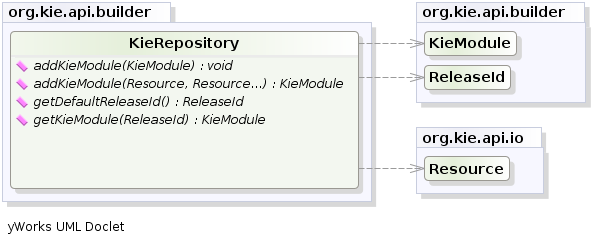
After this it is possible to create through the KieServices
a new KieContainer
for that KieModule
using its ReleaseId
.
However, since in this case the KieFileSystem
doesn’t contain any pom.xml file (it is possible to add one using the KieFileSystem.writePomXML
method), Kie cannot determine the ReleaseId
of the KieModule
and assign to it a default one.
This default ReleaseId
can be obtained from the KieRepository
and used to identify the KieModule
inside the KieRepository
itself.
The following example shows this whole process.
KieServices kieServices = KieServices.Factory.get();
KieFileSystem kfs = ...
kieServices.newKieBuilder( kfs ).buildAll();
KieContainer kieContainer = kieServices.newKieContainer(kieServices.getRepository().getDefaultReleaseId());
At this point it is possible to get KieBase
s and create new KieSession
s from this KieContainer
exactly in the same way as in the case of a KieContainer
created directly from the classpath.
It is a best practice to check the compilation results.
The KieBuilder
reports compilation results of 3 different severities: ERROR, WARNING and INFO.
An ERROR indicates that the compilation of the project failed and in the case no KieModule
is produced and nothing is added to the KieRepository
.
WARNING and INFO results can be ignored, but are available for inspection.
KieBuilder kieBuilder = kieServices.newKieBuilder( kfs ).buildAll();
assertEquals( 0, kieBuilder.getResults().getMessages( Message.Level.ERROR ).size() );
2.2.2.5. Changing the Default Build Result Severity
In some cases, it is possible to change the default severity of a type of build result. For instance, when a new rule with the same name of an existing rule is added to a package, the default behavior is to replace the old rule by the new rule and report it as an INFO. This is probably ideal for most use cases, but in some deployments the user might want to prevent the rule update and report it as an error.
Changing the default severity for a result type, configured like any other option in Drools, can be done by API calls, system properties or configuration files. As of this version, Drools supports configurable result severity for rule updates and function updates. To configure it using system properties or configuration files, the user has to use the following properties:
// sets the severity of rule updates
drools.kbuilder.severity.duplicateRule = <INFO|WARNING|ERROR>
// sets the severity of function updates
drools.kbuilder.severity.duplicateFunction = <INFO|WARNING|ERROR>
2.2.2.6. Building and running Drools in a fat jar
Many modules of Drools (e.g. drools-core, drools-compiler) have a file named kie.conf
containing the names of the classes implementing the services
provided by the corresponding module. When running Drools in a fat JAR, for example created by the Maven Shade Plugin, those various kie.conf
files
need to be merged, otherwise , the fat JAR will contain only 1 kie.conf from a single dependency, resulting into errors. You can merge resources in
the Maven Shade Plugin using transformers, like this:
<transformer implementation="org.apache.maven.plugins.shade.resource.AppendingTransformer">
<resource>META-INF/kie.conf</resource>
</transformer>
For instance this is required when running Drools in a Vert.x application. In this case the Maven Shade Plugin can be configured as it follows:
<plugin>
<groupId>org.apache.maven.plugins</groupId>
<artifactId>maven-shade-plugin</artifactId>
<version>3.1.0</version>
<executions>
<execution>
<phase>package</phase>
<goals>
<goal>shade</goal>
</goals>
<configuration>
<transformers>
<transformer implementation="org.apache.maven.plugins.shade.resource.ManifestResourceTransformer">
<manifestEntries>
<Main-Class>io.vertx.core.Launcher</Main-Class>
<Main-Verticle>${main.verticle}</Main-Verticle>
</manifestEntries>
</transformer>
<transformer implementation="org.apache.maven.plugins.shade.resource.AppendingTransformer">
<resource>META-INF/services/io.vertx.core.spi.VerticleFactory</resource>
</transformer>
<transformer implementation="org.apache.maven.plugins.shade.resource.AppendingTransformer">
<resource>META-INF/kie.conf</resource>
</transformer>
</transformers>
<artifactSet>
</artifactSet>
<outputFile>${project.build.directory}/${project.artifactId}-${project.version}-fat.jar</outputFile>
</configuration>
</execution>
</executions>
</plugin>
2.2.3. Deploying
2.2.3.1. KieBase
The KieBase
is a repository of all the application’s knowledge definitions.
It will contain rules, processes, functions, and type models.
The KieBase
itself does not contain data; instead, sessions are created from the KieBase
into which data can be inserted and from which process instances may be started.
The KieBase
can be obtained from the KieContainer
containing the KieModule
where the KieBase
has been defined.
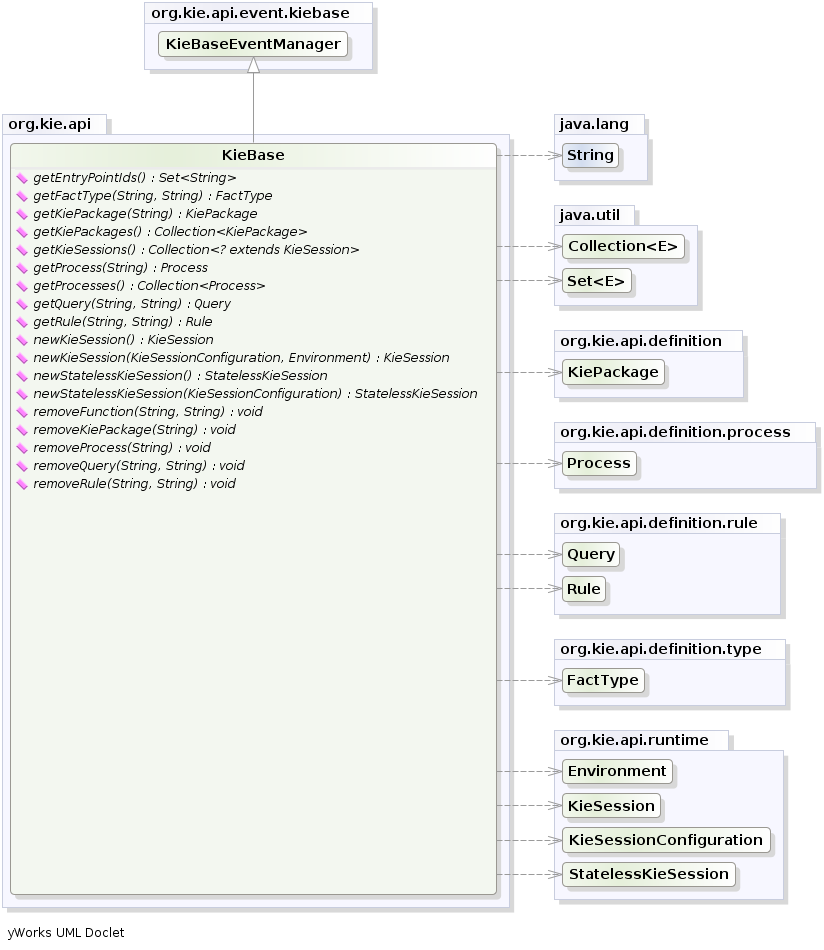
Sometimes, for instance in a OSGi environment, the KieBase
needs to resolve types that are not in the default class loader.
In this case it will be necessary to create a KieBaseConfiguration
with an additional class loader and pass it to KieContainer
when creating a new KieBase
from it.
KieServices kieServices = KieServices.Factory.get();
KieBaseConfiguration kbaseConf = kieServices.newKieBaseConfiguration( null, MyType.class.getClassLoader() );
KieBase kbase = kieContainer.newKieBase( kbaseConf );
2.2.3.2. KieSessions and KieBase Modifications
KieSessions will be discussed in more detail in section "Running". The KieBase
creates and returns KieSession
objects, and it may optionally keep references to those.
When KieBase
modifications occur those modifications are applied against the data in the sessions.
This reference is a weak reference and it is also optional, which is controlled by a boolean flag.
2.2.3.3. KieScanner
The KieScanner
allows continuous monitoring of your Maven repository to check whether a new release of a Kie project has been installed.
A new release is deployed in the KieContainer
wrapping that project.
The use of the KieScanner
requires kie-ci.jar to be on the classpath.
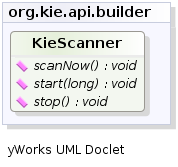
A KieScanner
can be registered on a KieContainer
as in the following example.
KieServices kieServices = KieServices.Factory.get();
ReleaseId releaseId = kieServices.newReleaseId( "org.acme", "myartifact", "1.0-SNAPSHOT" );
KieContainer kContainer = kieServices.newKieContainer( releaseId );
KieScanner kScanner = kieServices.newKieScanner( kContainer );
// Start the KieScanner polling the Maven repository every 10 seconds
kScanner.start( 10000L );
In this example the KieScanner
is configured to run with a fixed time interval, but it is also possible to run it on demand by invoking the scanNow()
method on it.
If the KieScanner
finds, in the Maven repository, an updated version of the Kie project used by that KieContainer
it automatically downloads the new version and triggers an incremental build of the new project.
At this point, existing KieBase
s and KieSession
s under the control of KieContainer
will get automatically upgraded with it - specifically, those KieBase
s obtained with getKieBase()
along with their related KieSession
s, and any KieSession
obtained directly with KieContainer.newKieSession()
thus referencing the default KieBase
.
Additionally, from this moment on, all the new KieBase
s and KieSession
s created from that KieContainer
will use the new project version.
Please notice however any existing KieBase
which was obtained via newKieBase()
before the KieScanner upgrade, and any of its related KieSession
s, will not get automatically upgraded; this is because KieBase
s obtained via newKieBase()
are not under the direct control of the KieContainer
.
The KieScanner
will only pickup changes to deployed jars if it is using a SNAPSHOT, version range, the LATEST, or the RELEASE setting.
Fixed versions will not automatically update at runtime.
In case you don’t want to install a maven repository, it is also possible to have a KieScanner
that works
by simply fetching update from a folder of a plain file system. You can create such a KieScanner
as simply as
KieServices kieServices = KieServices.Factory.get();
KieScanner kScanner = kieServices.newKieScanner( kContainer, "/myrepo/kjars" );
where "/myrepo/kjars" will be the folder where the KieScanner
will look for kjar updates. The jar files placed in this folder
have to follow the maven convention and then have to be a name in the form {artifactId}-{versionId}.jar
2.2.3.4. Maven Versions and Dependencies
Maven supports a number of mechanisms to manage versioning and dependencies within applications. Modules can be published with specific version numbers, or they can use the SNAPSHOT suffix. Dependencies can specify version ranges to consume, or take avantage of SNAPSHOT mechanism.
StackOverflow provides a very good description for this, which is reproduced below.
Since Maven 3.x metaversions RELEASE and LATEST are no longer supported for the sake of reproducible builds. |
See the POM Syntax section of the Maven book for more details.
Here’s an example illustrating the various options. In the Maven repository, com.foo:my-foo has the following metadata:
<metadata>
<groupId>com.foo</groupId>
<artifactId>my-foo</artifactId>
<version>2.0.0</version>
<versioning>
<release>1.1.1</release>
<versions>
<version>1.0</version>
<version>1.0.1</version>
<version>1.1</version>
<version>1.1.1</version>
<version>2.0.0</version>
</versions>
<lastUpdated>20090722140000</lastUpdated>
</versioning>
</metadata>
If a dependency on that artifact is required, you have the following options (other version ranges can be specified of course, just showing the relevant ones here): Declare an exact version (will always resolve to 1.0.1):
<version>[1.0.1]</version>
Declare an explicit version (will always resolve to 1.0.1 unless a collision occurs, when Maven will select a matching version):
<version>1.0.1</version>
Declare a version range for all 1.x (will currently resolve to 1.1.1):
<version>[1.0.0,2.0.0)</version>
Declare an open-ended version range (will resolve to 2.0.0):
<version>[1.0.0,)</version>
Declare the version as LATEST (will resolve to 2.0.0):
<version>LATEST</version>
Declare the version as RELEASE (will resolve to 1.1.1):
<version>RELEASE</version>
Note that by default your own deployments will update the "latest" entry in the Maven metadata, but to update the "release" entry, you need to activate the "release-profile" from the Maven super POM. You can do this with either "-Prelease-profile" or "-DperformRelease=true"
2.2.3.5. Settings.xml and Remote Repository Setup
The maven settings.xml is used to configure Maven execution. Detailed instructions can be found at the Maven website:
The settings.xml file can be located in 3 locations, the actual settings used is a merge of those 3 locations.
-
The Maven install:
$M2_HOME/conf/settings.xml
-
A user’s install:
${user.home}/.m2/settings.xml
-
Folder location specified by the system property
kie.maven.settings.custom
The settings.xml is used to specify the location of remote repositories. It is important that you activate the profile that specifies the remote repository, typically this can be done using "activeByDefault":
<profiles>
<profile>
<id>profile-1</id>
<activation>
<activeByDefault>true</activeByDefault>
</activation>
...
</profile>
</profiles>
Maven provides detailed documentation on using multiple remote repositories:
2.2.4. Running
2.2.4.1. KieBase
The KieBase
is a repository of all the application’s knowledge definitions.
It will contain rules, processes, functions, and type models.
The KieBase
itself does not contain data; instead, sessions are created from the KieBase
into which data can be inserted and from which process instances may be started.
The KieBase
can be obtained from the KieContainer
containing the KieModule
where the KieBase
has been defined.
KieBase kBase = kContainer.getKieBase();
2.2.4.2. KieSession
The KieSession
stores and executes on the runtime data.
It is created from the KieBase
.
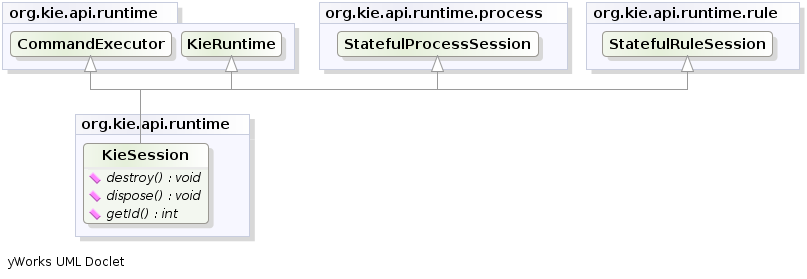
KieSession ksession = kbase.newKieSession();
2.2.4.3. KieRuntime
KieRuntime
The KieRuntime
provides methods that are applicable to both rules and processes, such as setting globals and registering channels.
("Exit point" is an obsolete synonym for "channel".)
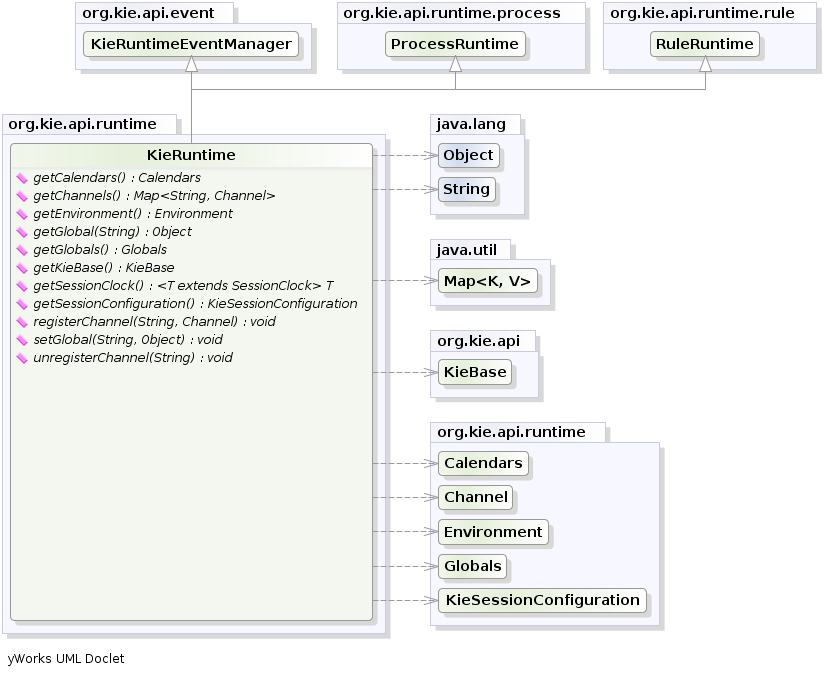
Globals are named objects that are made visible to the Drools engine, but in a way that is fundamentally different from the one for facts: changes in the object backing a global do not trigger reevaluation of rules. Still, globals are useful for providing static information, as an object offering services that are used in the RHS of a rule, or as a means to return objects from the Drools engine. When you use a global on the LHS of a rule, make sure it is immutable, or, at least, don’t expect changes to have any effect on the behavior of your rules.
A global must be declared in a rules file, and then it needs to be backed up with a Java object.
global java.util.List list
With the KIE base now aware of the global identifier and its type, it is now possible to call ksession.setGlobal()
with the global’s name and an object, for any session, to associate the object with the global.
Failure to declare the global type and identifier in DRL code will result in an exception being thrown from this call.
List list = new ArrayList();
ksession.setGlobal("list", list);
Make sure to set any global before it is used in the evaluation of a rule.
Failure to do so results in a NullPointerException
.
2.2.4.4. Event Model
The event package provides means to be notified of Drools engine events, including rules firing, objects being asserted, etc. This allows separation of logging and auditing activities from the main part of your application (and the rules).
The KieRuntimeEventManager
interface is implemented by the KieRuntime
which provides two interfaces, RuleRuntimeEventManager
and ProcessEventManager
.
We will only cover the RuleRuntimeEventManager
here.
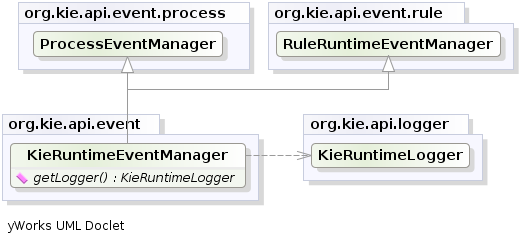
The RuleRuntimeEventManager
allows for listeners to be added and removed, so that events for the working memory and the agenda can be listened to.
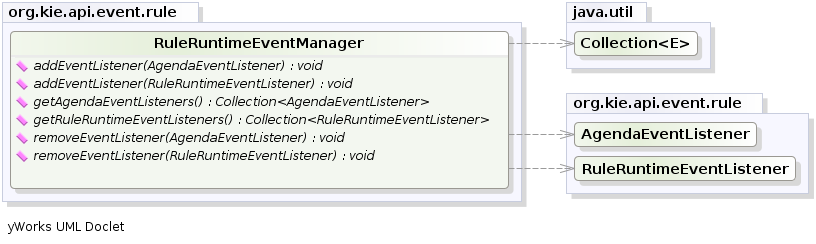
The following code snippet shows how a simple agenda listener is declared and attached to a session. It will print matches after they have fired.
ksession.addEventListener( new DefaultAgendaEventListener() {
public void afterMatchFired(AfterMatchFiredEvent event) {
super.afterMatchFired( event );
System.out.println( event );
}
});
Drools also provides DebugRuleRuntimeEventListener
and DebugAgendaEventListener
which implement each method with a debug print statement.
To print all Working Memory events, you add a listener like this:
ksession.addEventListener( new DebugRuleRuntimeEventListener() );
All emitted events implement the KieRuntimeEvent
interface which can be used to retrieve the actual KnowlegeRuntime
the event originated from.
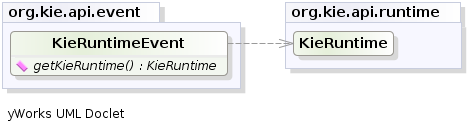
The events currently supported are:
-
MatchCreatedEvent
-
MatchCancelledEvent
-
BeforeMatchFiredEvent
-
AfterMatchFiredEvent
-
AgendaGroupPushedEvent
-
AgendaGroupPoppedEvent
-
ObjectInsertEvent
-
ObjectDeletedEvent
-
ObjectUpdatedEvent
-
ProcessCompletedEvent
-
ProcessNodeLeftEvent
-
ProcessNodeTriggeredEvent
-
ProcessStartEvent
2.2.4.5. KieRuntimeLogger
The KieRuntimeLogger uses the comprehensive event system in Drools to create an audit log that can be used to log the execution of an application for later inspection, using tools such as the Eclipse audit viewer.
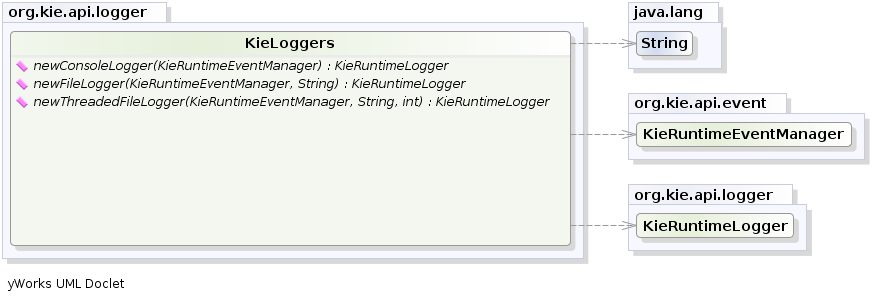
KieRuntimeLogger logger =
KieServices.Factory.get().getLoggers().newFileLogger(ksession, "logdir/mylogfile");
...
logger.close();
2.2.4.6. Commands and the CommandExecutor
KIE has the concept of stateful or stateless sessions. Stateful sessions have already been covered, which use the standard KieRuntime, and can be worked with iteratively over time. Stateless is a one-off execution of a KieRuntime with a provided data set. It may return some results, with the session being disposed at the end, prohibiting further iterative interactions. You can think of stateless as treating an engine like a function call with optional return results.
The foundation for this is the CommandExecutor
interface, which both the stateful and stateless interfaces extend.
This returns an ExecutionResults
:
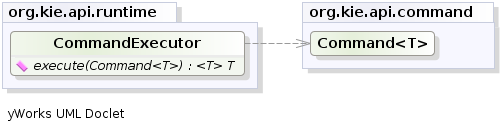
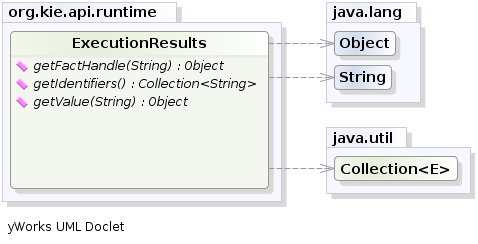
The CommandExecutor
allows for commands to be executed on those sessions, the only difference being that the StatelessKieSession executes fireAllRules()
at the end before disposing the session.
The commands can be created using the CommandExecutor
.The Javadocs provide the full list of the allowed comands using the CommandExecutor
.
setGlobal and getGlobal are two commands relevant to both Drools and jBPM.
Set Global calls setGlobal underneath.
The optional boolean indicates whether the command should return the global’s value as part of the ExecutionResults
.
If true it uses the same name as the global name.
A String can be used instead of the boolean, if an alternative name is desired.
StatelessKieSession ksession = kbase.newStatelessKieSession();
ExecutionResults bresults =
ksession.execute( CommandFactory.newSetGlobal( "stilton", new Cheese( "stilton" ), true);
Cheese stilton = bresults.getValue( "stilton" );
Allows an existing global to be returned. The second optional String argument allows for an alternative return name.
StatelessKieSession ksession = kbase.newStatelessKieSession();
ExecutionResults bresults =
ksession.execute( CommandFactory.getGlobal( "stilton" );
Cheese stilton = bresults.getValue( "stilton" );
All the above examples execute single commands.
The BatchExecution
represents a composite command, created from a list of commands.
It will iterate over the list and execute each command in turn.
This means you can insert some objects, start a process, call fireAllRules and execute a query, all in a single execute(…)
call, which is quite powerful.
The StatelessKieSession will execute fireAllRules()
automatically at the end.
However the keen-eyed reader probably has already noticed the FireAllRules
command and wondered how that works with a StatelessKieSession.
The FireAllRules
command is allowed, and using it will disable the automatic execution at the end; think of using it as a sort of manual override function.
Any command, in the batch, that has an out identifier set will add its results to the returned ExecutionResults
instance.
Let’s look at a simple example to see how this works.
The example presented includes command from the Drools and jBPM, for the sake of illustration.
They are covered in more detail in the Drool and jBPM specific sections.
StatelessKieSession ksession = kbase.newStatelessKieSession();
List cmds = new ArrayList();
cmds.add( CommandFactory.newInsertObject( new Cheese( "stilton", 1), "stilton") );
cmds.add( CommandFactory.newStartProcess( "process cheeses" ) );
cmds.add( CommandFactory.newQuery( "cheeses" ) );
ExecutionResults bresults = ksession.execute( CommandFactory.newBatchExecution( cmds ) );
Cheese stilton = ( Cheese ) bresults.getValue( "stilton" );
QueryResults qresults = ( QueryResults ) bresults.getValue( "cheeses" );
In the above example multiple commands are executed, two of which populate the ExecutionResults
.
The query command defaults to use the same identifier as the query name, but it can also be mapped to a different identifier.
All commands support XML and jSON marshalling using XStream, as well as JAXB marshalling. This is covered in Drools commands.
2.2.4.7. StatelessKieSession
The StatelessKieSession
wraps the KieSession
, instead of extending it.
Its main focus is on the decision service type scenarios.
It avoids the need to call dispose()
.
Stateless sessions do not support iterative insertions and the method call fireAllRules()
from Java code; the act of calling execute()
is a single-shot method that will internally instantiate a KieSession
, add all the user data and execute user commands, call fireAllRules()
, and then call dispose()
.
While the main way to work with this class is via the BatchExecution
(a subinterface of Command
) as supported by the CommandExecutor
interface, two convenience methods are provided for when simple object insertion is all that’s required.
The CommandExecutor
and BatchExecution
are talked about in detail in their own section.
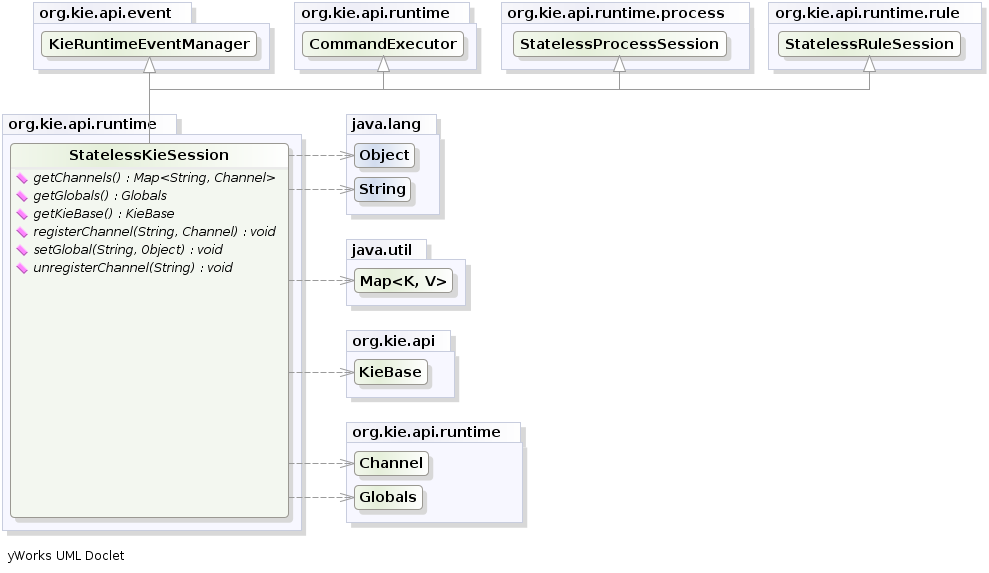
Our simple example shows a stateless session executing a given collection of Java objects using the convenience API. It will iterate the collection, inserting each element in turn.
StatelessKieSession ksession = kbase.newStatelessKieSession();
ksession.execute( collection );
If this was done as a single Command it would be as follows:
ksession.execute( CommandFactory.newInsertElements( collection ) );
If you wanted to insert the collection itself, and the collection’s individual elements, then CommandFactory.newInsert(collection)
would do the job.
Methods of the CommandFactory
create the supported commands, all of which can be marshalled using XStream and the BatchExecutionHelper
. BatchExecutionHelper
provides details on the XML format as well as how to use Drools Pipeline to automate the marshalling of BatchExecution
and ExecutionResults
.
StatelessKieSession
supports globals, scoped in a number of ways.
We cover the non-command way first, as commands are scoped to a specific execution call.
Globals can be resolved in three ways.
-
The StatelessKieSession method
getGlobals()
returns a Globals instance which provides access to the session’s globals. These are shared for all execution calls. Exercise caution regarding mutable globals because execution calls can be executing simultaneously in different threads.Example 26. Session scoped globalStatelessKieSession ksession = kbase.newStatelessKieSession(); // Set a global hbnSession, that can be used for DB interactions in the rules. ksession.setGlobal( "hbnSession", hibernateSession ); // Execute while being able to resolve the "hbnSession" identifier. ksession.execute( collection );
-
Using a delegate is another way of global resolution. Assigning a value to a global (with
setGlobal(String, Object)
) results in the value being stored in an internal collection mapping identifiers to values. Identifiers in this internal collection will have priority over any supplied delegate. Only if an identifier cannot be found in this internal collection, the delegate global (if any) will be used. -
The third way of resolving globals is to have execution scoped globals. Here, a
Command
to set a global is passed to theCommandExecutor
.
The CommandExecutor
interface also offers the ability to export data via "out" parameters.
Inserted facts, globals and query results can all be returned.
// Set up a list of commands
List cmds = new ArrayList();
cmds.add( CommandFactory.newSetGlobal( "list1", new ArrayList(), true ) );
cmds.add( CommandFactory.newInsert( new Person( "jon", 102 ), "person" ) );
cmds.add( CommandFactory.newQuery( "Get People" "getPeople" );
// Execute the list
ExecutionResults results =
ksession.execute( CommandFactory.newBatchExecution( cmds ) );
// Retrieve the ArrayList
results.getValue( "list1" );
// Retrieve the inserted Person fact
results.getValue( "person" );
// Retrieve the query as a QueryResults instance.
results.getValue( "Get People" );
2.2.4.8. Marshalling
The KieMarshallers
are used to marshal and unmarshal KieSessions.
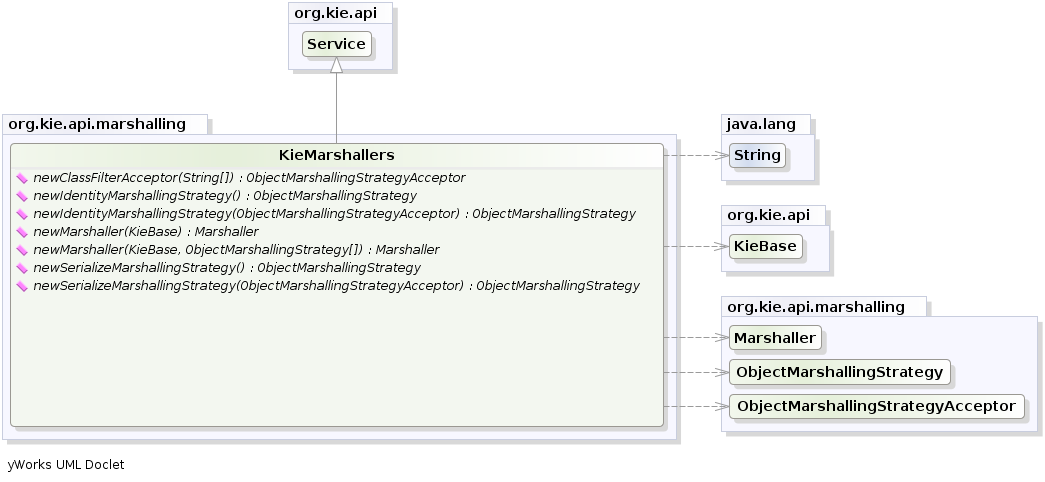
An instance of the KieMarshallers
can be retrieved from the KieServices
.
A simple example is shown below:
// ksession is the KieSession
// kbase is the KieBase
ByteArrayOutputStream baos = new ByteArrayOutputStream();
Marshaller marshaller = KieServices.Factory.get().getMarshallers().newMarshaller( kbase );
marshaller.marshall( baos, ksession );
baos.close();
However, with marshalling, you will need more flexibility when dealing with referenced user data.
To achieve this use the ObjectMarshallingStrategy
interface.
Two implementations are provided, but users can implement their own.
The two supplied strategies are IdentityMarshallingStrategy
and SerializeMarshallingStrategy
. SerializeMarshallingStrategy
is the default, as shown in the example above, and it just calls the Serializable
or Externalizable
methods on a user instance. IdentityMarshallingStrategy
creates an integer id for each user object and stores them in a Map, while the id is written to the stream.
When unmarshalling it accesses the IdentityMarshallingStrategy
map to retrieve the instance.
This means that if you use the IdentityMarshallingStrategy
, it is stateful for the life of the Marshaller instance and will create ids and keep references to all objects that it attempts to marshal.
Below is the code to use an Identity Marshalling Strategy.
ByteArrayOutputStream baos = new ByteArrayOutputStream();
KieMarshallers kMarshallers = KieServices.Factory.get().getMarshallers()
ObjectMarshallingStrategy oms = kMarshallers.newIdentityMarshallingStrategy()
Marshaller marshaller =
kMarshallers.newMarshaller( kbase, new ObjectMarshallingStrategy[]{ oms } );
marshaller.marshall( baos, ksession );
baos.close();
Im most cases, a single strategy is insufficient.
For added flexibility, the ObjectMarshallingStrategyAcceptor
interface can be used.
This Marshaller has a chain of strategies, and while reading or writing a user object it iterates the strategies asking if they accept responsibility for marshalling the user object.
One of the provided implementations is ClassFilterAcceptor
.
This allows strings and wild cards to be used to match class names.
The default is ".", so in the above example the Identity Marshalling Strategy is used which has a default "." acceptor.
Assuming that we want to serialize all classes except for one given package, where we will use identity lookup, we could do the following:
ByteArrayOutputStream baos = new ByteArrayOutputStream();
KieMarshallers kMarshallers = KieServices.Factory.get().getMarshallers()
ObjectMarshallingStrategyAcceptor identityAcceptor =
kMarshallers.newClassFilterAcceptor( new String[] { "org.domain.pkg1.*" } );
ObjectMarshallingStrategy identityStrategy =
kMarshallers.newIdentityMarshallingStrategy( identityAcceptor );
ObjectMarshallingStrategy sms = kMarshallers.newSerializeMarshallingStrategy();
Marshaller marshaller =
kMarshallers.newMarshaller( kbase,
new ObjectMarshallingStrategy[]{ identityStrategy, sms } );
marshaller.marshall( baos, ksession );
baos.close();
Note that the acceptance checking order is in the natural order of the supplied elements.
2.2.4.9. Persistence and Transactions
Longterm out of the box persistence with Java Persistence API (JPA) is possible with Drools. It is necessary to have some implementation of the Java Transaction API (JTA) installed. For development purposes the Bitronix Transaction Manager is suggested, as it’s simple to set up and works embedded, but for production use JBoss Transactions is recommended.
KieServices kieServices = KieServices.Factory.get();
Environment env = kieServices.newEnvironment();
env.set( EnvironmentName.ENTITY_MANAGER_FACTORY,
Persistence.createEntityManagerFactory( "emf-name" ) );
env.set( EnvironmentName.TRANSACTION_MANAGER,
TransactionManagerServices.getTransactionManager() );
// KieSessionConfiguration may be null, and a default will be used
KieSession ksession =
kieServices.getStoreServices().newKieSession( kbase, null, env );
int sessionId = ksession.getId();
UserTransaction ut =
(UserTransaction) new InitialContext().lookup( "java:comp/UserTransaction" );
ut.begin();
ksession.insert( data1 );
ksession.insert( data2 );
ksession.startProcess( "process1" );
ut.commit();
To use a JPA, the Environment must be set with both the EntityManagerFactory
and the TransactionManager
.
If rollback occurs the ksession state is also rolled back, hence it is possible to continue to use it after a rollback.
To load a previously persisted KieSession you’ll need the id, as shown below:
KieSession ksession =
kieServices.getStoreServices().loadKieSession( sessionId, kbase, null, env );
To enable persistence several classes must be added to your persistence.xml, as in the example below:
<persistence-unit name="org.drools.persistence.jpa" transaction-type="JTA">
<provider>org.hibernate.ejb.HibernatePersistence</provider>
<jta-data-source>jdbc/BitronixJTADataSource</jta-data-source>
<class>org.drools.persistence.info.SessionInfo</class>
<class>org.drools.persistence.info.WorkItemInfo</class>
<properties>
<property name="hibernate.dialect" value="org.hibernate.dialect.H2Dialect"/>
<property name="hibernate.max_fetch_depth" value="3"/>
<property name="hibernate.hbm2ddl.auto" value="update" />
<property name="hibernate.show_sql" value="true" />
<property name="hibernate.transaction.manager_lookup_class"
value="org.hibernate.transaction.BTMTransactionManagerLookup" />
</properties>
</persistence-unit>
The jdbc JTA data source would have to be configured first. Bitronix provides a number of ways of doing this, and its documentation should be consulted for details. For a quick start, here is the programmatic approach:
PoolingDataSource ds = new PoolingDataSource();
ds.setUniqueName( "jdbc/BitronixJTADataSource" );
ds.setClassName( "org.h2.jdbcx.JdbcDataSource" );
ds.setMaxPoolSize( 3 );
ds.setAllowLocalTransactions( true );
ds.getDriverProperties().put( "user", "sa" );
ds.getDriverProperties().put( "password", "sasa" );
ds.getDriverProperties().put( "URL", "jdbc:h2:mem:mydb" );
ds.init();
Bitronix also provides a simple embedded JNDI service, ideal for testing. To use it, add a jndi.properties file to your META-INF folder and add the following line to it:
java.naming.factory.initial=bitronix.tm.jndi.BitronixInitialContextFactory
2.2.5. Installation and Deployment Cheat Sheets
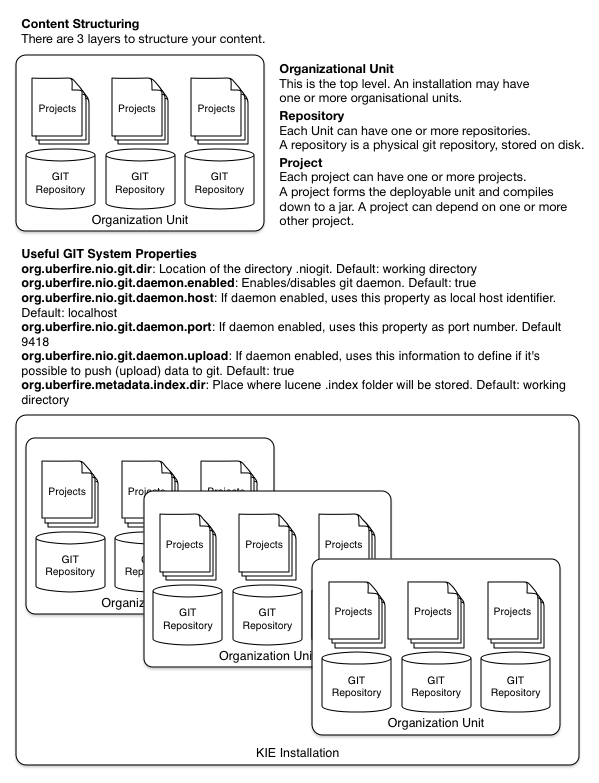
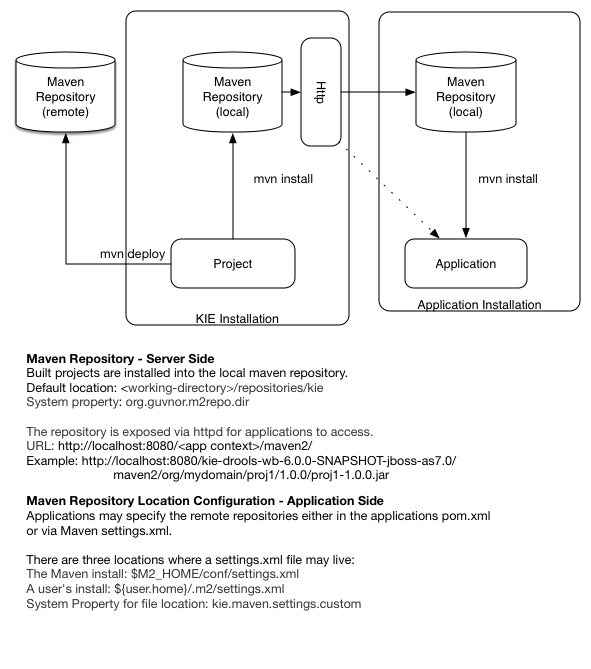
2.2.6. Build, Deploy and Utilize Examples
The best way to learn the new build system is by example. The source project "drools-examples-api" contains a number of examples, and can be found at GitHub:
Each example is described below, the order starts with the simplest (most of the options are defaulted) and working its way up to more complex use cases.
The Deploy use cases shown below all involve mvn install
.
Remote deployment of JARs in Maven is well covered in Maven literature.
Utilize refers to the initial act of loading the resources and providing access to the KIE runtimes.
Where as Run refers to the act of interacting with those runtimes.
2.2.6.1. Default KieSession
-
Project: default-kesession.
-
Summary: Empty kmodule.xml KieModule on the classpath that includes all resources in a single default KieBase. The example shows the retrieval of the default KieSession from the classpath.
An empty kmodule.xml will produce a single KieBase that includes all files found under resources path, be it DRL, BPMN2, XLS etc. That single KieBase is the default and also includes a single default KieSession. Default means they can be created without knowing their names.
<kmodule xmlns="http://www.drools.org/xsd/kmodule"> </kmodule>
mvn install
ks.getKieClasspathContainer() returns the KieContainer that contains the KieBases deployed onto the environment classpath. kContainer.newKieSession() creates the default KieSession. Notice that you no longer need to look up the KieBase, in order to create the KieSession. The KieSession knows which KieBase it’s associated with, and use that, which in this case is the default KieBase.
KieServices ks = KieServices.Factory.get();
KieContainer kContainer = ks.getKieClasspathContainer();
KieSession kSession = kContainer.newKieSession();
kSession.setGlobal("out", out);
kSession.insert(new Message("Dave", "Hello, HAL. Do you read me, HAL?"));
kSession.fireAllRules();
2.2.6.2. Named KieSession
-
Project: named-kiesession.
-
Summary: kmodule.xml that has one named KieBase and one named KieSession. The examples shows the retrieval of the named KieSession from the classpath.
kmodule.xml will produce a single named KieBase, 'kbase1' that includes all files found under resources path, be it DRL, BPMN2, XLS etc. KieSession 'ksession1' is associated with that KieBase and can be created by name.
<kmodule xmlns="http://www.drools.org/xsd/kmodule">
<kbase name="kbase1">
<ksession name="ksession1"/>
</kbase>
</kmodule>
mvn install
ks.getKieClasspathContainer() returns the KieContainer that contains the KieBases deployed onto the environment classpath. This time the KieSession uses the name 'ksession1'. You do not need to lookup the KieBase first, as it knows which KieBase 'ksession1' is assocaited with.
KieServices ks = KieServices.Factory.get();
KieContainer kContainer = ks.getKieClasspathContainer();
KieSession kSession = kContainer.newKieSession("ksession1");
kSession.setGlobal("out", out);
kSession.insert(new Message("Dave", "Hello, HAL. Do you read me, HAL?"));
kSession.fireAllRules();
2.2.6.3. KieBase Inheritence
-
Project: kiebase-inclusion.
-
Summary: 'kmodule.xml' demonstrates that one KieBase can include the resources from another KieBase, from another KieModule. In this case it inherits the named KieBase from the 'name-kiesession' example. The included KieBase can be from the current KieModule or any other KieModule that is in the pom.xml dependency list.
kmodule.xml will produce a single named KieBase, 'kbase2' that includes all files found under resources path, be it DRL, BPMN2, XLS etc. Further it will include all the resources found from the KieBase 'kbase1', due to the use of the 'includes' attribute. KieSession 'ksession2' is associated with that KieBase and can be created by name.
<kbase name="kbase2" includes="kbase1">
<ksession name="ksession2"/>
</kbase>
This example requires that the previous example, 'named-kiesession', is built and installed to the local Maven repository first. Once installed it can be included as a dependency, using the standard Maven <dependencies> element.
<project xmlns="http://maven.apache.org/POM/4.0.0"
xmlns:xsi="http://www.w3.org/2001/XMLSchema-instance"
xsi:schemaLocation="http://maven.apache.org/POM/4.0.0 http://maven.apache.org/xsd/maven-4.0.0.xsd">
<modelVersion>4.0.0</modelVersion>
<parent>
<groupId>org.drools</groupId>
<artifactId>drools-examples-api</artifactId>
<version>6.0.0/version>
</parent>
<artifactId>kiebase-inclusion</artifactId>
<name>Drools API examples - KieBase Inclusion</name>
<dependencies>
<dependency>
<groupId>org.drools</groupId>
<artifactId>drools-compiler</artifactId>
</dependency>
<dependency>
<groupId>org.drools</groupId>
<artifactId>named-kiesession</artifactId>
<version>6.0.0</version>
</dependency>
</dependencies>
</project>
Once 'named-kiesession' is built and installed this example can be built and installed as normal. Again the act of installing, will force the unit tests to run, demonstrating the use case.
mvn install
ks.getKieClasspathContainer() returns the KieContainer that contains the KieBases deployed onto the environment classpath. This time the KieSession uses the name 'ksession2'. You do not need to lookup the KieBase first, as it knows which KieBase 'ksession1' is assocaited with. Notice two rules fire this time, showing that KieBase 'kbase2' has included the resources from the dependency KieBase 'kbase1'.
KieServices ks = KieServices.Factory.get();
KieContainer kContainer = ks.getKieClasspathContainer();
KieSession kSession = kContainer.newKieSession("ksession2");
kSession.setGlobal("out", out);
kSession.insert(new Message("Dave", "Hello, HAL. Do you read me, HAL?"));
kSession.fireAllRules();
kSession.insert(new Message("Dave", "Open the pod bay doors, HAL."));
kSession.fireAllRules();
2.2.6.4. Multiple KieBases
-
Project: 'multiple-kbases.
-
Summary: Demonstrates that the 'kmodule.xml' can contain any number of KieBase or KieSession declarations. Introduces the 'packages' attribute to select the folders for the resources to be included in the KieBase.
kmodule.xml produces 6 different named KieBases. 'kbase1' includes all resources from the KieModule. The other KieBases include resources from other selected folders, via the 'packages' attribute. Note the use of wildcard '*', to select this package and all packages below it.
<kmodule xmlns="http://www.drools.org/xsd/kmodule">
<kbase name="kbase1">
<ksession name="ksession1"/>
</kbase>
<kbase name="kbase2" packages="org.some.pkg">
<ksession name="ksession2"/>
</kbase>
<kbase name="kbase3" includes="kbase2" packages="org.some.pkg2">
<ksession name="ksession3"/>
</kbase>
<kbase name="kbase4" packages="org.some.pkg, org.other.pkg">
<ksession name="ksession4"/>
</kbase>
<kbase name="kbase5" packages="org.*">
<ksession name="ksession5"/>
</kbase>
<kbase name="kbase6" packages="org.some.*">
<ksession name="ksession6"/>
</kbase>
</kmodule>
mvn install
Only part of the example is included below, as there is a test method per KieSession, but each one is a repetition of the other, with different list expectations.
@Test
public void testSimpleKieBase() {
List<Integer> list = useKieSession("ksession1");
// no packages imported means import everything
assertEquals(4, list.size());
assertTrue( list.containsAll( asList(0, 1, 2, 3) ) );
}
//.. other tests for ksession2 to ksession6 here
private List<Integer> useKieSession(String name) {
KieServices ks = KieServices.Factory.get();
KieContainer kContainer = ks.getKieClasspathContainer();
KieSession kSession = kContainer.newKieSession(name);
List<Integer> list = new ArrayList<Integer>();
kSession.setGlobal("list", list);
kSession.insert(1);
kSession.fireAllRules();
return list;
}
2.2.6.5. KieContainer from KieRepository
-
Project: kcontainer-from-repository
-
Summary: The project does not contain a kmodule.xml, nor does the pom.xml have any dependencies for other KieModules. Instead the Java code demonstrates the loading of a dynamic KieModule from a Maven repository.
The pom.xml must include kie-ci as a depdency, to ensure Maven is available at runtime. As this uses Maven under the hood you can also use the standard Maven settings.xml file.
<project xmlns="http://maven.apache.org/POM/4.0.0"
xmlns:xsi="http://www.w3.org/2001/XMLSchema-instance"
xsi:schemaLocation="http://maven.apache.org/POM/4.0.0 http://maven.apache.org/xsd/maven-4.0.0.xsd">
<modelVersion>4.0.0</modelVersion>
<parent>
<groupId>org.drools</groupId>
<artifactId>drools-examples-api</artifactId>
<version>6.0.0</version>
</parent>
<artifactId>kiecontainer-from-kierepo</artifactId>
<name>Drools API examples - KieContainer from KieRepo</name>
<dependencies>
<dependency>
<groupId>org.kie</groupId>
<artifactId>kie-ci</artifactId>
</dependency>
</dependencies>
</project>
mvn install
In the previous examples the classpath KieContainer used. This example creates a dynamic KieContainer as specified by the ReleaseId. The ReleaseId uses Maven conventions for group id, artifact id and version. It also obeys LATEST and SNAPSHOT for versions.
KieServices ks = KieServices.Factory.get();
// Install example1 in the local Maven repo before to do this
KieContainer kContainer = ks.newKieContainer(ks.newReleaseId("org.drools", "named-kiesession", "6.0.0-SNAPSHOT"));
KieSession kSession = kContainer.newKieSession("ksession1");
kSession.setGlobal("out", out);
Object msg1 = createMessage(kContainer, "Dave", "Hello, HAL. Do you read me, HAL?");
kSession.insert(msg1);
kSession.fireAllRules();
2.2.6.6. Default KieSession from File
-
Project: default-kiesession-from-file
-
Summary: Dynamic KieModules can also be loaded from any Resource location. The loaded KieModule provides default KieBase and KieSession definitions.
No kmodue.xml file exists. The project 'default-kiesession' must be built first, so that the resulting JAR, in the target folder, can be referenced as a File.
mvn install
Any KieModule can be loaded from a Resource location and added to the KieRepository. Once deployed in the KieRepository it can be resolved via its ReleaseId. Note neither Maven or kie-ci are needed here. It will not set up a transitive dependency parent classloader.
KieServices ks = KieServices.Factory.get();
KieRepository kr = ks.getRepository();
KieModule kModule = kr.addKieModule(ks.getResources().newFileSystemResource(getFile("default-kiesession")));
KieContainer kContainer = ks.newKieContainer(kModule.getReleaseId());
KieSession kSession = kContainer.newKieSession();
kSession.setGlobal("out", out);
Object msg1 = createMessage(kContainer, "Dave", "Hello, HAL. Do you read me, HAL?");
kSession.insert(msg1);
kSession.fireAllRules();
2.2.6.7. Named KieSession from File
-
Project: named-kiesession-from-file
-
Summary: Dynamic KieModules can also be loaded from any Resource location. The loaded KieModule provides named KieBase and KieSession definitions.
No kmodue.xml file exists. The project 'named-kiesession' must be built first, so that the resulting JAR, in the target folder, can be referenced as a File.
mvn install
Any KieModule can be loaded from a Resource location and added to the KieRepository. Once in the KieRepository it can be resolved via its ReleaseId. Note neither Maven or kie-ci are needed here. It will not setup a transitive dependency parent classloader.
KieServices ks = KieServices.Factory.get();
KieRepository kr = ks.getRepository();
KieModule kModule = kr.addKieModule(ks.getResources().newFileSystemResource(getFile("named-kiesession")));
KieContainer kContainer = ks.newKieContainer(kModule.getReleaseId());
KieSession kSession = kContainer.newKieSession("ksession1");
kSession.setGlobal("out", out);
Object msg1 = createMessage(kContainer, "Dave", "Hello, HAL. Do you read me, HAL?");
kSession.insert(msg1);
kSession.fireAllRules();
2.2.6.8. KieModule with Dependent KieModule
-
Project: kie-module-form-multiple-files
-
Summary: Programmatically provide the list of dependant KieModules, without using Maven to resolve anything.
No kmodue.xml file exists. The projects 'named-kiesession' and 'kiebase-include' must be built first, so that the resulting JARs, in the target folders, can be referenced as Files.
mvn install
Creates two resources. One is for the main KieModule 'exRes1' the other is for the dependency 'exRes2'. Even though kie-ci is not present and thus Maven is not available to resolve the dependencies, this shows how you can manually specify the dependent KieModules, for the vararg.
KieServices ks = KieServices.Factory.get();
KieRepository kr = ks.getRepository();
Resource ex1Res = ks.getResources().newFileSystemResource(getFile("kiebase-inclusion"));
Resource ex2Res = ks.getResources().newFileSystemResource(getFile("named-kiesession"));
KieModule kModule = kr.addKieModule(ex1Res, ex2Res);
KieContainer kContainer = ks.newKieContainer(kModule.getReleaseId());
KieSession kSession = kContainer.newKieSession("ksession2");
kSession.setGlobal("out", out);
Object msg1 = createMessage(kContainer, "Dave", "Hello, HAL. Do you read me, HAL?");
kSession.insert(msg1);
kSession.fireAllRules();
Object msg2 = createMessage(kContainer, "Dave", "Open the pod bay doors, HAL.");
kSession.insert(msg2);
kSession.fireAllRules();
2.2.6.9. Programmaticaly build a Simple KieModule with Defaults
-
Project: kiemoduelmodel-example
-
Summary: Programmaticaly buid a KieModule from just a single file. The POM and models are all defaulted. This is the quickest out of the box approach, but should not be added to a Maven repository.
mvn install
This programmatically builds a KieModule. It populates the model that represents the ReleaseId and kmodule.xml, and it adds the relevant resources. A pom.xml is generated from the ReleaseId.
KieServices ks = KieServices.Factory.get();
KieRepository kr = ks.getRepository();
KieFileSystem kfs = ks.newKieFileSystem();
kfs.write("src/main/resources/org/kie/example5/HAL5.drl", getRule());
KieBuilder kb = ks.newKieBuilder(kfs);
kb.buildAll(); // kieModule is automatically deployed to KieRepository if successfully built.
if (kb.getResults().hasMessages(Level.ERROR)) {
throw new RuntimeException("Build Errors:\n" + kb.getResults().toString());
}
KieContainer kContainer = ks.newKieContainer(kr.getDefaultReleaseId());
KieSession kSession = kContainer.newKieSession();
kSession.setGlobal("out", out);
kSession.insert(new Message("Dave", "Hello, HAL. Do you read me, HAL?"));
kSession.fireAllRules();
2.2.6.10. Programmaticaly build a KieModule using Meta Models
-
Project: kiemoduelmodel-example
-
Summary: Programmaticaly build a KieModule, by creating its kmodule.xml meta model resources.
mvn install
This programmatically builds a KieModule. It populates the model that represents the ReleaseId and kmodule.xml, as well as add the relevant resources. A pom.xml is generated from the ReleaseId.
KieServices ks = KieServices.Factory.get();
KieFileSystem kfs = ks.newKieFileSystem();
Resource ex1Res = ks.getResources().newFileSystemResource(getFile("named-kiesession"));
Resource ex2Res = ks.getResources().newFileSystemResource(getFile("kiebase-inclusion"));
ReleaseId rid = ks.newReleaseId("org.drools", "kiemodulemodel-example", "6.0.0-SNAPSHOT");
kfs.generateAndWritePomXML(rid);
KieModuleModel kModuleModel = ks.newKieModuleModel();
kModuleModel.newKieBaseModel("kiemodulemodel")
.addInclude("kiebase1")
.addInclude("kiebase2")
.newKieSessionModel("ksession6");
kfs.writeKModuleXML(kModuleModel.toXML());
kfs.write("src/main/resources/kiemodulemodel/HAL6.drl", getRule());
KieBuilder kb = ks.newKieBuilder(kfs);
kb.setDependencies(ex1Res, ex2Res);
kb.buildAll(); // kieModule is automatically deployed to KieRepository if successfully built.
if (kb.getResults().hasMessages(Level.ERROR)) {
throw new RuntimeException("Build Errors:\n" + kb.getResults().toString());
}
KieContainer kContainer = ks.newKieContainer(rid);
KieSession kSession = kContainer.newKieSession("ksession6");
kSession.setGlobal("out", out);
Object msg1 = createMessage(kContainer, "Dave", "Hello, HAL. Do you read me, HAL?");
kSession.insert(msg1);
kSession.fireAllRules();
Object msg2 = createMessage(kContainer, "Dave", "Open the pod bay doors, HAL.");
kSession.insert(msg2);
kSession.fireAllRules();
Object msg3 = createMessage(kContainer, "Dave", "What's the problem?");
kSession.insert(msg3);
kSession.fireAllRules();
2.3. Security
2.3.1. Security Manager
The KIE engine is a platform for the modelling and execution of business behavior, using a multitude of declarative abstractions and metaphores, like rules, processes, decision tables and etc.
Many times, the authoring of these metaphores is done by third party groups, be it a different group inside the same company, a group from a partner company, or even anonymous third parties on the internet.
Rules and Processes are designed to execute arbitrary code in order to do their job, but in such cases it might be necessary to constrain what they can do.
For instance, it is unlikely a rule should be allowed to create a classloader (what could open the system to an attack) and certainly it should not be allowed to make a call to System.exit()
.
The Java Platform provides a very comprehensive and well defined security framework that allows users to define policies for what a system can do. The KIE platform leverages that framework and allow application developers to define a specific policy to be applied to any execution of user provided code, be it in rules, processes, work item handlers and etc.
2.3.1.1. How to define a KIE Policy
Rules and processes can run with very restrict permissions, but the engine itself needs to perform many complex operations in order to work. Examples are: it needs to create classloaders, read system properties, access the file system, etc.
Once a security manager is installed, though, it will apply restrictions to all the code executing in the JVM according to the defined policy. For that reason, KIE allows the user to define two different policy files: one for the engine itself and one for the assets deployed into and executed by the engine.
One easy way to setup the enviroment is to give the engine itself a very permissive policy, while providing a constrained policy for rules and processes.
Policy files follow the standard policy file syntax as described in the Java documentation. For more details, see:
A permissive policy file for the engine can look like the following:
grant {
permission java.security.AllPermission;
}
An example security policy for rules could be:
grant {
permission java.util.PropertyPermission "*", "read";
permission java.lang.RuntimePermission "accessDeclaredMembers";
}
Please note that depending on what the rules and processes are supposed to do, many more permissions might need to be granted, like accessing files in the filesystem, databases, etc.
In order to use these policy files, all that is necessary is to execute the application with these files as parameters to the JVM. Three parameters are required:
Parameter | Meaning |
---|---|
-Djava.security.manager |
Enables the security manager |
-Djava.security.policy=<jvm_policy_file> |
Defines the global policy file to be applied to the whole application, including the engine |
-Dkie.security.policy=<kie_policy_file> |
Defines the policy file to be applied to rules and processes |
For instance:
java -Djava.security.manager -Djava.security.policy=global.policy -Dkie.security.policy=rules.policy
foo.bar.MyApp
When executing the engine inside a container, use your container’s documentation to find out how to configure the Security Manager and how to define the global security policy.
Define the kie security policy as described above and set the |
Please note that unless a Security Manager is configured, the |
A Security Manager has a high performance impact in the JVM. Applications with strict performance requirements are strongly discouraged of using a Security Manager. An alternative is the use of other security procedures like the auditing of rules/processes before testing and deployment to prevent malicious code from being deployed to the environment. |
Drools Runtime and Language
Drools is a powerful Hybrid Reasoning System.
3. Hybrid Reasoning
3.1. Artificial Intelligence
3.1.1. A Little History
Over the last few decades artificial intelligence (AI) became an unpopular term, with the well-known "AI Winter". There were large boasts from scientists and engineers looking for funding, which never lived up to expectations, resulting in many failed projects. Thinking Machines Corporation and the 5th Generation Computer (5GP) project probably exemplify best the problems at the time.
Thinking Machines Corporation was one of the leading AI firms in 1990, it had sales of nearly $65 million. Here is a quote from its brochure:
“Some day we will build a thinking machine. It will be a truly intelligent machine. One that can see and hear and speak. A machine that will be proud of us.”
Yet 5 years later it filed for bankruptcy protection under Chapter 11. The site inc.com has a fascinating article titled "The Rise and Fall of Thinking Machines". The article covers the growth of the industry and how a cosy relationship with Thinking Machines and DARPA over-heated the market, to the point of collapse. It explains how and why commerce moved away from AI and towards more practical number-crunching super computers.
The 5th Generation Computer project was a USD 400 million project in Japan to build a next generation computer. Valves (or Tubes) was the first generation, transistors the second, integrated circuits the third and finally microprocessors was the fourth. The fifth was intended to be a machine capable of effective Artificial Intelligence. This project spurred an "arms" race with the UK and USA, that caused much of the AI bubble. The 5GP would provide massive multi-cpu parallel processing hardware along with powerful knowledge representation and reasoning software via Prolog ; a type of [term]_ expert system_ . By 1992 the project was considered a failure and cancelled. It was the largest and most visible commercial venture for Prolog, and many of the failures are pinned on the problems of trying to run a logic based programming language concurrently on multi CPU hardware with effective results. Some believe that the failure of the 5GP project tainted Prolog and relegated it to academia, see "Whatever Happened to Prolog" by John C. Dvorak.
However while research funding dried up and the term AI became less used, many green shoots were planted and continued more quietly under discipline specific names: cognitive systems , machine learning , intelligent systems ,[term]_ knowledge representation and reasoning_ . Offshoots of these then made their way into commercial systems, such as expert systems in the Business Rules Management System (BRMS) market.
Imperative , system based languages, languages such as C, C++, Java and C#/.Net have dominated the last 20 years, enabled by the practicality of the languages and ability to run with good performance on commodity hardware. However many believe there is a renaissance underway in the field of AI, spurred by advances in hardware capabilities and AI research. In 2005 Heather Havenstein authored "Spring comes to AI winter" which outlines a case for this resurgence. Norvig and Russel dedicate several pages to what factors allowed the industry to overcome its problems and the research that came about as a result:
Recent years have seen a revolution in both the content and the methodology of work in artificial intelligence. It is now more common to build on existing theories than to propose brand-new ones, to base claims on rigorous theorems or hard experimental evidence rather than on intuition, and to show relevance to real-world applications rather than toy examples.
Computer vision , neural networks , machine learning and knowledge representation and reasoning (KRR) have made great strides towards becoming practical in commercial environments. For example, vision-based systems can now fully map out and navigate their environments with strong recognition skills. As a result we now have self-driving cars about to enter the commercial market. Ontological research, based around description logic, has provided very rich semantics to represent our world. Algorithms such as the tableaux algorithm have made it possible to use those rich semantics effectively in large complex ontologies. Early KRR systems, like Prolog in 5GP, were dogged by the limited semantic capabilities and memory restrictions on the size of those ontologies.
3.1.2. Knowledge Representation and Reasoning
In A Little History talks about AI as a broader subject and touches on Knowledge Representation and Reasoning (KRR) and also Expert Systems, I’ll come back to Expert Systems later.
KRR is about how we represent our knowledge in symbolic form, i.e. how we describe something. Reasoning is about how we go about the act of thinking using this knowledge. System based object-oriented languages, like C++, Java and C#, have data definitions called classes for describing the composition and behaviour of modeled entities. In Java we call exemplars of these described things beans or instances. However those classification systems are limited to ensure computational efficiency. Over the years researchers have developed increasingly sophisticated ways to represent our world. Many of you may already have heard of OWL (Web Ontology Language). There is always a gap between what can be theoretically represented and what can be used computationally in practically timely manner, which is why OWL has different sub-languages from Lite to Full. It is not believed that any reasoning system can support OWL Full. However, algorithmic advances continue to narrow that gap and improve the expressiveness available to reasoning engines.
There are also many approaches to how these systems go about thinking. You may have heard discussions comparing the merits of forward chaining, which is reactive and data driven, with backward chaining, which is passive and query driven. Many other types of reasoning techniques exist, each of which enlarges the scope of the problems we can tackle declaratively. To list just a few: imperfect reasoning (fuzzy logic, certainty factors), defeasible logic, belief systems, temporal reasoning and correlation. You don’t need to understand all these terms to understand and use Drools. They are just there to give an idea of the range of scope of research topics, which is actually far more extensive, and continues to grow as researchers push new boundaries.
KRR is often referred to as the core of Artificial Intelligence. Even when using biological approaches like neural networks, which model the brain and are more about pattern recognition than thinking, they still build on KRR theory. My first endeavours with Drools were engineering oriented, as I had no formal training or understanding of KRR. Learning KRR has allowed me to get a much wider theoretical background, and to better understand both what I’ve done and where I’m going, as it underpins nearly all of the theoretical side to our Drools R&D. It really is a vast and fascinating subject that will pay dividends for those who take the time to learn. I know it did and still does for me. Bracham and Levesque have written a seminal piece of work, called "Knowledge Representation and Reasoning" that is a must read for anyone wanting to build strong foundations. I would also recommend the Russel and Norvig book "Artificial Intelligence, a modern approach" which also covers KRR.
3.1.3. Rules Engines and Production Rule Systems (PRS)
We’ve now covered a brief history of AI and learnt that the core of AI is formed around KRR. We’ve shown than KRR is a vast and fascinating subject which forms the bulk of the theory driving Drools R&D.
The Drools engine is the computer program that delivers KRR functionality to the developer. At a high level it has three components:
-
Ontology
-
Rules
-
Data
As previously mentioned the ontology is the representation model we use for our "things". It could use records or Java classes or full-blown OWL based ontologies. The rules perform the reasoning, i.e., they facilitate "thinking". The distinction between rules and ontologies blurs a little with OWL based ontologies, whose richness is rule based.
The term "rules engine" is quite ambiguous in that it can be any system that uses rules, in any form, that can be applied to data to produce outcomes. This includes simple systems like form validation and dynamic expression engines. The book "How to Build a Business Rules Engine" (2004) by Malcolm Chisholm exemplifies this ambiguity. The book is actually about how to build and alter a database schema to hold validation rules. The book then shows how to generate Visual Basic code from those validation rules to validate data entry. While perfectly valid, this is very different to what we are talking about.
Drools started life as a specific type of rules engine called a Production Rule System (PRS) and was based around the Rete algorithm (usually pronounced as two syllables, e.g., REH-te or RAY-tay). The Rete algorithm, developed by Charles Forgy in 1974, forms the brain of a Production Rule System and is able to scale to a large number of rules and facts. A Production Rule is a two-part structure: the Drools engine matches facts and data against Production Rules - also called Productions or just Rules - to infer conclusions which result in actions.
when
<conditions>
then
<actions>;
The process of matching the new or existing facts against Production Rules is called pattern matching , which is performed by the inference engine . Actions execute in response to changes in data, like a database trigger; we say this is a data driven approach to reasoning. The actions themselves can change data, which in turn could match against other rules causing them to fire; this is referred to as forward chaining
Drools 5.x implements and extends the Rete algorithm. This extended Rete algorithm is named ReteOO , signifying that Drools has an enhanced and optimized implementation of the Rete algorithm for object oriented systems. Other Rete based engines also have marketing terms for their proprietary enhancements to Rete, like RetePlus and Rete III. The most common enhancements are covered in "Production Matching for Large Learning Systems" (1995) by Robert B. Doorenbos' thesis, which presents Rete/UL. Drools 6.x introduces a new lazy algorithm named PHREAK ; which is covered in more detail in the PHREAK algorithm section.
The Rules are stored in the Production Memory and the facts that the Inference Engine matches against are kept in the Working Memory. Facts are asserted into the Working Memory where they may then be modified or retracted. A system with a large number of rules and facts may result in many rules being true for the same fact assertion; these rules are said to be in conflict. The Agenda manages the execution order of these conflicting rules using a Conflict Resolution strategy.
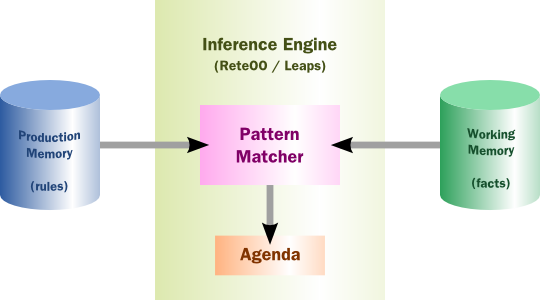
3.1.4. Hybrid Reasoning Systems (HRS)
You may have read discussions comparing the merits of forward chaining (reactive and data driven) or backward chaining (passive query). Here is a quick explanation of these two main types of reasoning.
Forward chaining is "data-driven" and thus reactionary, with facts being asserted into working memory, which results in one or more rules being concurrently true and scheduled for execution by the Agenda. In short, we start with a fact, it propagates through the rules, and we end in a conclusion.
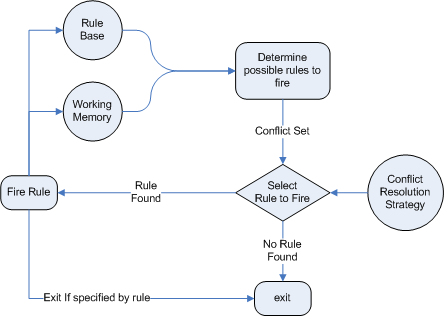
Backward chaining is "goal-driven", meaning that we start with a conclusion which the Drools engine tries to satisfy. If it can’t, then it searches for conclusions that it can satisfy. These are known as subgoals, that will help satisfy some unknown part of the current goal. It continues this process until either the initial conclusion is proven or there are no more subgoals. Prolog is an example of a Backward Chaining engine. Drools can also do backward chaining, which we refer to as derivation queries.
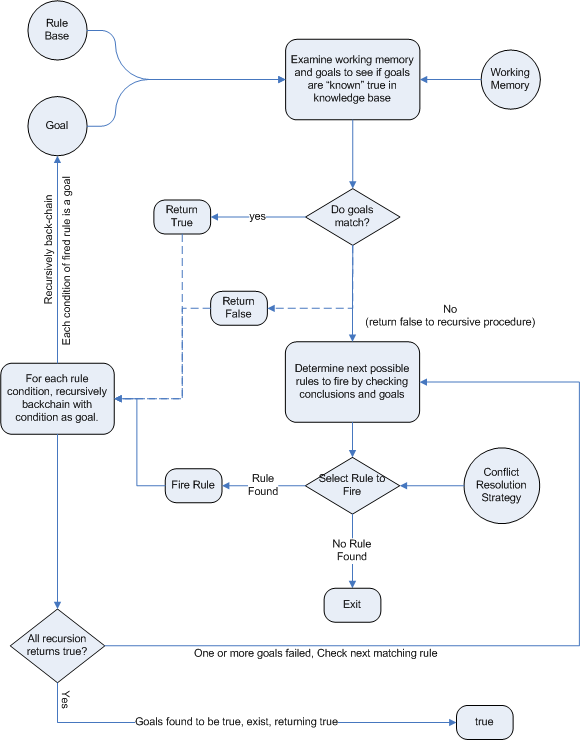
Historically you would have to make a choice between systems like OPS5 (forward) or Prolog (backward). Nowadays many modern systems provide both types of reasoning capabilities. There are also many other types of reasoning techniques, each of which enlarges the scope of the problems we can tackle declaratively. To list just a few: imperfect reasoning (fuzzy logic, certainty factors), defeasible logic, belief systems, temporal reasoning and correlation. Modern systems are merging these capabilities, and others not listed, to create hybrid reasoning systems (HRS).
While Drools started out as a PRS, 5.x introduced Prolog style backward chaining reasoning as well as some functional programming styles. For this reason we now prefer the term Hybrid Reasoning System when describing Drools.
Drools currently provides crisp reasoning, but imperfect reasoning is almost ready. Initially this will be imperfect reasoning with fuzzy logic; later we’ll add support for other types of uncertainty. Work is also under way to bring OWL based ontological reasoning, which will integrate with our traits system. We also continue to improve our functional programming capabilities.
3.1.5. Expert Systems
You will often hear the terms expert systems used to refer to production rule systems or Prolog -like systems. While this is normally acceptable, it’s technically incorrect as these are frameworks to build expert systems with, rather than expert systems themselves. It becomes an expert system once there is an ontological model to represent the domain and there are facilities for knowledge acquisition and explanation.
Mycin is the most famous expert system, built during the 70s. It is still heavily covered in academic literature, such as the recommended book "Expert Systems" by Peter Jackson.
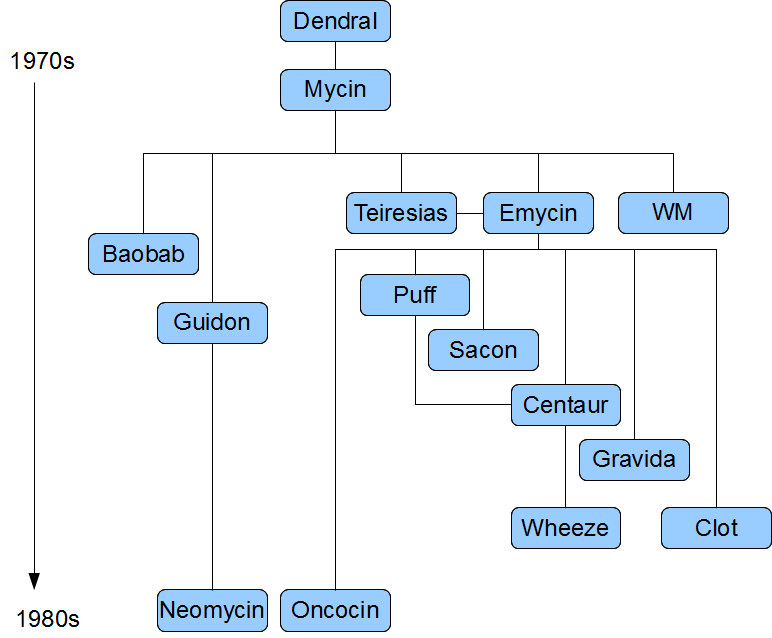
3.1.6. Recommended Reading
* General AI, KRR and Expert System Books*
For those wanting to get a strong theoretical background in KRR and expert systems, I’d strongly recommend the following books. "Artificial Intelligence: A Modern Approach" is a must have, for anyone’s bookshelf.
-
Introduction to Expert Systems
-
Peter Jackson
-
-
Expert Systems: Principles and Programming
-
Joseph C. Giarratano, Gary D. Riley
-
-
Knowledge Representation and Reasoning
-
Ronald J. Brachman, Hector J. Levesque
-
-
Artificial Intelligence : A Modern Approach.
-
Stuart Russell and Peter Norvig
-
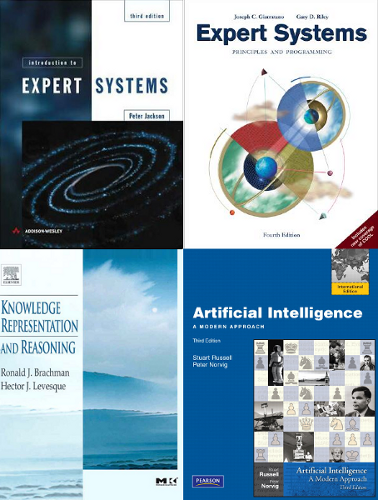
* Papers*
Here are some recommended papers that cover interesting areas in rules engine research:
-
Production Matching for Large Learning Systems: Rete/UL (1993)
-
Robert B. Doorenbos
-
-
Advances In Rete Pattern Matching
-
Marshall Schor, Timothy P. Daly, Ho Soo Lee, Beth R. Tibbitts (AAAI 1986)
-
-
Collection-Oriented Match
-
Anurag Acharya and Milind Tambe (1993)
-
-
The Leaps Algorithm
-
Don Batery (1990)
-
-
Gator: An Optimized Discrimination Network for Active Database Rule Condition Testing
-
Eric Hanson , Mohammed S. Hasan (1993)
-
* Drools Books*
There are currently three Drools books, all from Packt Publishing.
-
JBoss Drools Business Rules
-
Paul Browne
-
-
Drools JBoss Rules 5.0 Developers Guide
-
Michal Bali
-
-
Drools Developer’s Cookbook
-
Lucas Amador
-
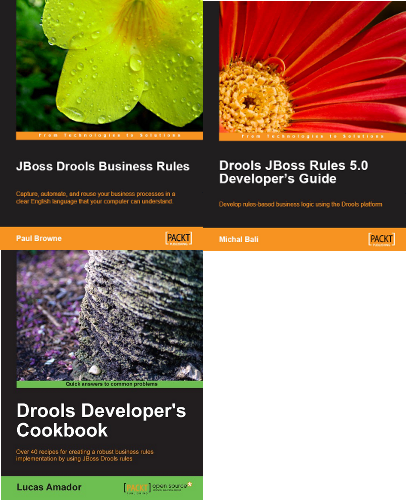
3.2. Rete Algorithm
The Rete algorithm was invented by Dr. Charles Forgy and documented in his PhD thesis in 1978-79. A simplified version of the paper was published in 1982 (http://citeseer.ist.psu.edu/context/505087/0). The Latin word "rete" means "net" or "network". The Rete algorithm can be broken into 2 parts: rule compilation and runtime execution.
The compilation algorithm describes how the Rules in the Production Memory are processed to generate an efficient discrimination network. In non-technical terms, a discrimination network is used to filter data as it propagates through the network. The nodes at the top of the network would have many matches, and as we go down the network, there would be fewer matches. At the very bottom of the network are the terminal nodes. In Dr. Forgy’s 1982 paper, he described 4 basic nodes: root, 1-input, 2-input and terminal.
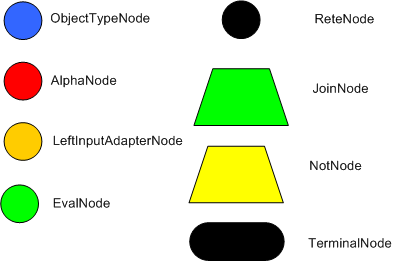
The root node is where all objects enter the network.
From there, it immediately goes to the ObjectTypeNode.
The purpose of the ObjectTypeNode is to make sure the Drools engine doesn’t do more work than it needs to.
For example, say we have 2 objects: Account and Order.
If the Drools engine tried to evaluate every single node against every object, it would waste a lot of cycles.
To make things efficient, the Drools engine should only pass the object to the nodes that match the object type.
The easiest way to do this is to create an ObjectTypeNode and have all 1-input and 2-input nodes descend from it.
This way, if an application asserts a new Account, it won’t propagate to the nodes for the Order object.
In Drools when an object is asserted it retrieves a list of valid ObjectTypesNodes via a lookup in a HashMap from the object’s Class; if this list doesn’t exist it scans all the ObjectTypeNodes finding valid matches which it caches in the list.
This enables Drools to match against any Class type that matches with an instanceof
check.
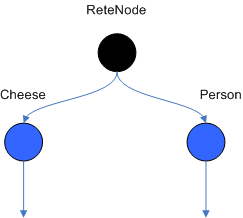
ObjectTypeNodes can propagate to AlphaNodes, LeftInputAdapterNodes and BetaNodes.
AlphaNodes are used to evaluate literal conditions.
Although the 1982 paper only covers equality conditions, many RETE implementations support other operations.
For example, Account.name == "Mr
Trout"
is a literal condition.
When a rule has multiple literal conditions for a single object type, they are linked together.
This means that if an application asserts an Account object, it must first satisfy the first literal condition before it can proceed to the next AlphaNode.
In Dr.
Forgy’s paper, he refers to these as IntraElement conditions.
The following diagram shows the AlphaNode combinations for Cheese( name == "cheddar", strength == "strong" ):
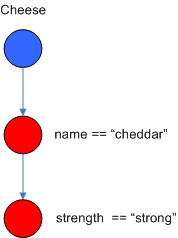
Drools extends Rete by optimizing the propagation from ObjectTypeNode to AlphaNode using hashing. Each time an AlphaNode is added to an ObjectTypeNode it adds the literal value as a key to the HashMap with the AlphaNode as the value. When a new instance enters the ObjectType node, rather than propagating to each AlphaNode, it can instead retrieve the correct AlphaNode from the HashMap, thereby avoiding unnecessary literal checks.
There are two two-input nodes, JoinNode and NotNode, and both are types of BetaNodes. BetaNodes are used to compare 2 objects, and their fields, to each other. The objects may be the same or different types. By convention we refer to the two inputs as left and right. The left input for a BetaNode is generally a list of objects; in Drools this is a Tuple. The right input is a single object. Two Nodes can be used to implement 'exists' checks. BetaNodes also have memory. The left input is called the Beta Memory and remembers all incoming tuples. The right input is called the Alpha Memory and remembers all incoming objects. Drools extends Rete by performing indexing on the BetaNodes. For instance, if we know that a BetaNode is performing a check on a String field, as each object enters we can do a hash lookup on that String value. This means when facts enter from the opposite side, instead of iterating over all the facts to find valid joins, we do a lookup returning potentially valid candidates. At any point a valid join is found the Tuple is joined with the Object; which is referred to as a partial match; and then propagated to the next node.
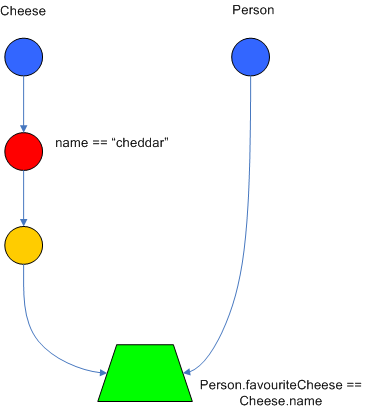
To enable the first Object, in the above case Cheese, to enter the network we use a LeftInputNodeAdapter - this takes an Object as an input and propagates a single Object Tuple.
Terminal nodes are used to indicate a single rule having matched all its conditions; at this point we say the rule has a full match. A rule with an 'or' conditional disjunctive connective results in subrule generation for each possible logical branch; thus one rule can have multiple terminal nodes.
Drools also performs node sharing. Many rules repeat the same patterns, and node sharing allows us to collapse those patterns so that they don’t have to be re-evaluated for every single instance. The following two rules share the first pattern, but not the last:
rule
when
Cheese( $cheddar : name == "cheddar" )
$person : Person( favouriteCheese == $cheddar )
then
System.out.println( $person.getName() + " likes cheddar" );
end
rule
when
Cheese( $cheddar : name == "cheddar" )
$person : Person( favouriteCheese != $cheddar )
then
System.out.println( $person.getName() + " does not like cheddar" );
end
As you can see below, the compiled Rete network shows that the alpha node is shared, but the beta nodes are not. Each beta node has its own TerminalNode. Had the second pattern been the same it would have also been shared.
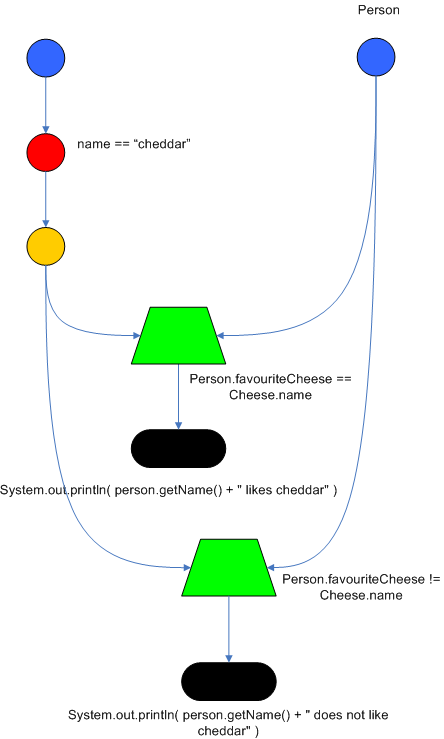
3.3. ReteOO Algorithm
The ReteOO was developed throughout the 3, 4 and 5 series releases. It takes the RETE algorithm and applies well known enhancements, all of which are covered by existing academic literature:
Node sharing
-
Sharing is applied to both the alpha and beta network. The beta network sharing is always from the root pattern.
Alpha indexing
-
Alpha Nodes with many children use a hash lookup mechanism to avoid testing each result.
Beta indexing
-
Join, Not and Exist nodes index their memories using a hash. This reduces the join attempts for equal checks. Recently range indexing was added to Not and Exists.
Tree based graphs
-
Join matches did not contain any references to their parent or children matches. Deletions would have to recalculate all join matches again, which involves recreating all those join match objects, to be able to find the parts of the network where the tuples should be deleted. This is called symmetrical propagation. A tree graph provides parent and children references, so a deletion is just a matter of following those references. This is asymmetrical propagation. The result is faster and makes less impact on the GC. It is also more robust because changes in values will not cause memory leaks if they happen without the Drools engine being notified.
Modify-in-place
-
Traditional RETE implements a modify as a delete + insert. This causes all join tuples to be GC’d, many of which are recreated again as part of the insert. Modify-in-place instead propagates as a single pass; every node is inspected.
Property reactive
-
Also called "new trigger condition". Allows more fine grained reactivity to updates. A Pattern can react to changes to specific properties and ignore others. This alleviates problems of recursion and also helps with performance.
Sub-networks
-
Not, Exists and Accumulate can each have nested conditional elements, which forms sub networks.
Backward Chaining
-
Prolog style derivation trees for backward chaining are supported. The implementation is stack based, so does not have method recursion issues for large graphs.
Lazy Truth Maintenance
-
Truth maintenance has a runtime cost which is incurred whether TMS is used or not. Lazy TMS enables it only on first use; further it is activated per object type, so irrelevant object types do not incur the runtime cost.
Heap based agenda
-
The agenda uses a binary heap queue to sort rule matches by salience, rather than any linear search or maintenance approach.
Dynamic Rules
-
Rules can be added and removed at runtime, while the Drools engine is still populated with data.
3.4. PHREAK Algorithm
Drools 6 introduces a new algorithm, that attempts to address some of the core issues of RETE. The algorithm is not a rewrite from scratch: it incorporates all of the existing code and enhancements from ReteOO. While PHREAK is an evolution of the RETE algorithm, it is no longer classified as a RETE implementation. Once an animal evolves beyond a certain point and key characteristics are changed, the animal becomes classified as a new species; in the same way PHREAK cannot be considered RETE. There are two key RETE characteristics that strongly identify any derivative strains, regardless of optimizations:
-
That it is an eager, data oriented algorithm.
-
All work is done using the insert, update or delete actions, eagerly producing all partial matches for all rules.
PHREAK in contrast is characterised as a lazy, goal oriented algorithm, where partial matching is aggressively delayed.
This eagerness of RETE can lead to a lot of churn in large systems, and much wasted work, where "wasted work" is defined as matching efforts that do not result in a rule firing.
PHREAK was heavily inspired by a number of algorithms, including (but not limited to) LEAPS, RETE/UL and Collection-Oriented Match. PHREAK has all enhancements listed in the ReteOO section. In addition it adds the following set of enhancements, which are explained in more detail in the following paragraphs.
-
Three layers of contextual memory; Node, Segment and Rule memories.
-
Rule, segment and node based linking.
-
Lazy (delayed) rule evaluation.
-
Isolated rule evaluation.
-
Set oriented propagations.
-
Stack based evaluations, with pause and resume.
When the PHREAK engine is started all rules are said to be unlinked; no rule evaluation can happen while rules are unlinked. The insert, update and delete actions are queued before entering the beta network. A simple heuristic, based on the rule most likely to result in firings, is used to select the next rule for evaluation; this delays the evaluation and firing of the other rules. Only once a rule has all right inputs populated will the rule be considered linked in, although no work is yet done. Instead a goal is created, that represents the rule, and placed into a priority queue; which is ordered by salience. Each queue itself is associated with an AgendaGroup. Only the active AgendaGroup will inspect its queue, popping the goal for the rule with the highest salience and submitting it for evaluation. So the work done shifts from the insert, update, delete phase to the fireAllRules phase. Only the rule for which the goal was created is evaluated, other potential rule evaluations from those facts are delayed. While individual rules are evaluated, node sharing is still achieved through the process of segmentation, which is explained later.
Each successful join attempt in RETE produces a tuple (or token, or partial match) that will be propagated to the child nodes. For this reason it is characterised as a tuple oriented algorithm. For each child node that it reaches it will attempt to join with the other side of the node; again each successful join attempt will be propagated straight away. This creates a descent recursion effect, thrashing the network of nodes as it ripples up and down, left and right from the point of entry into the beta network to all the reachable leaf nodes.
PHREAK propagation is set oriented (or collection-oriented), instead of tuple oriented. For the rule being evaluated it will visit the first node and process all queued inserts, updates and deletes. The results are added to a set and the set is propagated to the child node. In the child node all queued inserts, updates and deletes are processed, adding the results to the same set. Once finished that set is propagated to the next child node, and so on until the terminal node is reached. This creates a single pass, pipeline type effect that is isolated to the current rule being evaluated. This creates a batch process effect which can provide performance advantages for certain rule constructs, such as sub-networks with accumulates. In the future it will lend itself to being able to exploit multi-core machines in a number of ways.
The Linking and Unlinking uses a layered bit mask system, based on a network segmentation. When the rule network is built segments are created for nodes that are shared by the same set of rules. A rule itself is made up from a path of segments, although if there is no sharing that will be a single segment. A bit-mask offset is assigned to each node in the segment. Also another bit mask (the layering) is assigned to each segment in the rule’s path. When there is at least one input (data propagation) the node’s bit is set to on. When each node has its bit set to on the segment’s bit is also set to on. Conversely if any node’s bit is set to off, the segment is then also set to off. If each segment in the rule’s path is set to on, the rule is said to be linked in and a goal is created to schedule the rule for evaluation. The same bit-mask technique is used to also track dirty node, segments and rules; this allows for a rule already linked in to be scheduled for evaluation if it’s considered dirty since it was last evaluated.
This ensures that no rule will ever evaluate partial matches, if it’s impossible for it to result in rule instances because one of the joins has no data. This is possible in RETE and it will merrily churn away producing partial match attempts for all nodes, even if the last join is empty.
While the incremental rule evaluation always starts from the root node, the dirty bit masks are used to allow nodes and segments that are not dirty to be skipped.
Using the existence of at at least one items of data per node is a fairly basic heuristic. Future work would attempt to delay the linking even further, using techniques such as arc consistency to determine whether or not matching will result in rule instance firings.
Where as RETE has just a singe unit of memory, the node memory, PHREAK has 3 levels of memory. This allows for much more contextual understanding during evaluation of a Rule.
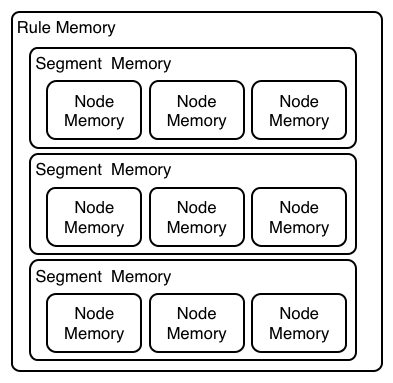
Example 1 shows a single rule with three patterns: A, B and C. It forms a single segment, with bits 1, 2 and 4 for the nodes. The single segment has a bit offset of 1.
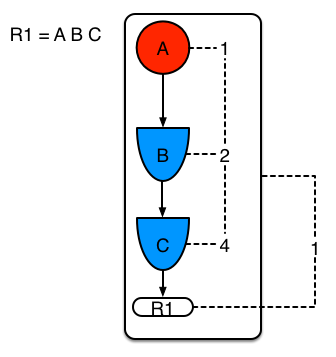
Example 2 demonstrates what happens when another rule is added that shares the pattern A. A is placed in its own segment, resulting in two segments per rule. Those two segments form a path for their respective rules. The first segment is shared by both paths. When A is linked the segment becomes linked. It then iterates each path the segment is shared by, setting the bit 1 to on. If B and C are later turned on, the second segment for path R1 is linked in; this causes bit 2 to be turned on for R1. With bit 1 and bit 2 set to on for R1, the rule is now linked and a goal created to schedule the rule for later evaluation and firing.
When a rule is evaluated it is the segments that allow the results of matching to be shared. Each segment has a staging memory to queue all inserts, updates and deletes for that segment. If R1 were to be evaluated it would process A and result in a set of tuples. The algorithm detects that there is a segmentation split and will create peered tuples for each insert, update and delete in the set and add them to R2’s staging memory. Those tuples will be merged with any existing staged tuples and wait for R2 to eventually be evaluated.
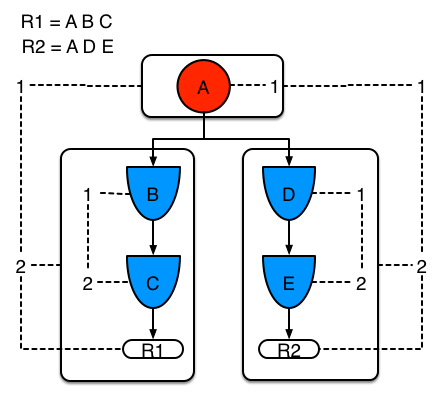
Example 3 adds a third rule and demonstrates what happens when A and B are shared. Only the bits for the segments are shown this time. Demonstrating that R4 has 3 segments, R3 has 3 segments and R1 has 2 segments. A and B are shared by R1, R3 and R4. While D is shared by R3 and R4.
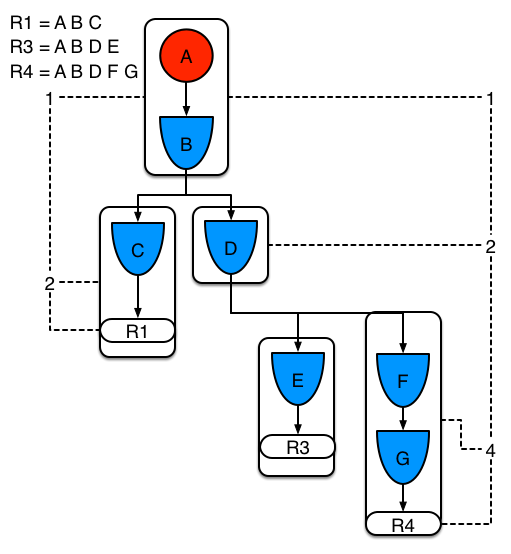
Sub-networks are formed when a Not, Exists or Accumulate node contains more than one element. In Example 4 "B not( C )" forms the sub network, note that "not( C )" is a single element which does not require a sub network and is therefore merged inside of the Not node.
The sub network gets its own segment. R1 still has a path of two segments. The sub network forms another "inner" path. When the sub network is linked in, it will link in the outer segment.
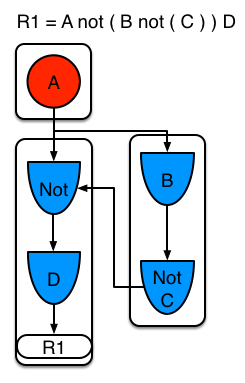
Example 5 shows that the sub-network nodes can be shared by a rule that does not have a sub-network. This results in the sub-network segment being split into two.
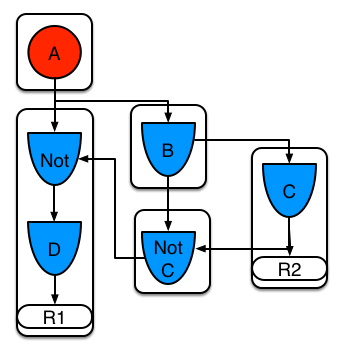
Constrained Not nodes and Accumulate nodes have special behaviour: these can never unlink a segment, and are always considered to have their bits on.
All rule evaluations are incremental, and will not waste work recomputing matches already produced.
The evaluation algorithm is stack, instead of method recursion, based. Evaluation can be paused and resumed at any time, via the use of a StackEntry to represent the node currently being evaluated.
When a rule evaluation reaches a sub-network a StackEntry is created for the outer path segment and the sub-network segment. The sub-network segment is evaluated first; when the set reaches the end of the sub-network path it is merged into a staging list for the outer node it feeds into. The previous StackEntry is then resumed and can now process the results of the sub network. This has the added benefit that all work is processed in a batch, before propagating to the child node; this is much more efficient for Accumulate nodes.
The same stack system can be used for efficient backward chaining. When a rule evaluation reaches a query node it again pauses the current evaluation, by placing it on the stack. The query is then evaluated which produces a result set, which is saved in a memory location for the resumed StackEntry to pick up and propagate to the child node. If the query itself called other queries the process would repeat, with the current query being paused and a new evaluation setup for the current query node.
One final point on performance: One single rule in general will not evaluate any faster with PHREAK than it does with RETE. For a given rule and same data set, which using a root context object to enable and disable matching, both attempt the same number of matches, produce the same number of rule instances and take roughly the same amount of time, except for the use case with subnetworks and Accumulates.
PHREAK can however be considered more forgiving that RETE for poorly written rule bases and with a more graceful degradation of performance as the number of rules and complexity increases.
RETE will also churn away producing partial matches for rules that do not have data in all the joins, whereas PHREAK will avoid this.
So it’s not that PHREAK is faster than RETE; it just won’t slow down as much as your system grows :)
AgendaGroups did not help in RETE performance, as all rules were evaluated at all times, regardless of the group. The same is true for salience, which is why root context objects are often used to limit matching attempts. PHREAK only evaluates rules for the active AgendaGroup, and within that group will attempt to avoid evaluation of rules (via salience) that do not result in rule instance firings.
With PHREAK AgendaGroups and salience now become useful performance tools. The root context objects are no longer needed and are potentially counterproductive to performance, as they force the flushing and recreation of matches for rules.
4. User Guide
4.1. The Basics
4.1.1. Stateless KIE session
So where do we get started? There are so many use cases and so much functionality in a rules engine such as Drools that it becomes beguiling. Have no fear my intrepid adventurer, the complexity is layered and you can ease yourself in with simple use cases.
Stateless session, not utilising inference, forms the simplest use case. A stateless session can be called like a function passing it some data and then receiving some results back. Some common use cases for stateless sessions are, but not limited to:
-
Validation
-
Is this person eligible for a mortgage?
-
-
Calculation
-
Compute a mortgage premium.
-
-
Routing and Filtering
-
Filter incoming messages, such as emails, into folders.
-
Send incoming messages to a destination.
-
So let’s start with a very simple example using a driving license application.
public class Applicant {
private String name;
private int age;
private boolean valid;
// getter and setter methods here
}
Now that we have our data model we can write our first rule. We assume that the application uses rules to reject invalid applications. As this is a simple validation use case we will add a single rule to disqualify any applicant younger than 18.
package com.company.license
rule "Is of valid age"
when
$a : Applicant( age < 18 )
then
$a.setValid( false );
end
To make the Drools engine aware of data, so it can be processed against the rules, we have to insert the data, much like with a database.
When the Applicant instance is inserted into the Drools engine it is evaluated against the constraints of the rules, in this case just two constraints for one rule.
We say two because the type Applicant is the first object type constraint, and age < 18
is the second field constraint.
An object type constraint plus its zero or more field constraints is referred to as a pattern.
When an inserted instance satisfies both the object type constraint and all the field constraints, it is said to be matched.
The $a
is a binding variable which permits us to reference the matched object in the consequence.
There its properties can be updated.
The dollar character ('$') is optional, but it helps to differentiate variable names from field names.
The process of matching patterns against the inserted data is, not surprisingly, often referred to as pattern matching.
To use this rule it is necessary to put it a Drools file, just a plain text file with .drl extension , short for "Drools Rule Language". Let’s call this file licenseApplication.drl, and store it in a Kie Project.
A Kie Project has the structure of a normal Maven project with an additional file (kmodule.xml) defining the KieBase
s and KieSession
s that can be created.
This file has to be placed in the resources/META-INF folder of the Maven project while all the other Drools artifacts, such as the licenseApplication.drl containing the former rule, must be stored in the resources folder or in any other subfolder under it.
Since meaningful defaults have been provided for all configuration aspects, the simplest kmodule.xml file can contain just an empty kmodule tag like the following:
<?xml version="1.0" encoding="UTF-8"?>
<kmodule xmlns="http://www.drools.org/xsd/kmodule"/>
At this point it is possible to create a KieContainer
that reads the files to be built, from the classpath.
KieServices kieServices = KieServices.Factory.get();
KieContainer kContainer = kieServices.getKieClasspathContainer();
The above code snippet compiles all the DRL files found on the classpath and put the result of this compilation, a KieModule
, in the KieContainer
.
If there are no errors, we are now ready to create our session from the KieContainer
and execute against some data:
StatelessKieSession kSession = kContainer.newStatelessKieSession();
Applicant applicant = new Applicant( "Mr John Smith", 16 );
assertTrue( applicant.isValid() );
ksession.execute( applicant );
assertFalse( applicant.isValid() );
The preceding code executes the data against the rules. Since the applicant is under the age of 18, the application is marked as invalid.
So far we’ve only used a single instance, but what if we want to use more than one? We can execute against any object implementing Iterable, such as a collection.
Let’s add another class called Application
, which has the date of the application, and we’ll also move the boolean valid field to the Application
class.
public class Applicant {
private String name;
private int age;
// getter and setter methods here
}
public class Application {
private Date dateApplied;
private boolean valid;
// getter and setter methods here
}
We will also add another rule to validate that the application was made within a period of time.
package com.company.license
rule "Is of valid age"
when
Applicant( age < 18 )
$a : Application()
then
$a.setValid( false );
end
rule "Application was made this year"
when
$a : Application( dateApplied > "01-jan-2009" )
then
$a.setValid( false );
end
Unfortunately a Java array does not implement the Iterable
interface, so we have to use the JDK converter method Arrays.asList(…)
.
The code shown below executes against an iterable list, where all collection elements are inserted before any matched rules are fired.
StatelessKieSession kSession = kContainer.newStatelessKieSession();
Applicant applicant = new Applicant( "Mr John Smith", 16 );
Application application = new Application();
assertTrue( application.isValid() );
ksession.execute( Arrays.asList( new Object[] { application, applicant } ) );
assertFalse( application.isValid() );
The two execute methods execute(Object object)
and execute(Iterable objects)
are actually convenience methods for the interface BatchExecutor
's method execute(Command command)
.
The KieCommands
commands factory, obtainable from the KieServices
like all other factories of the KIE API, is used to create commands, so that the following is equivalent to execute(Iterable it)
:
ksession.execute( kieServices.getCommands().newInsertElements( Arrays.asList( new Object[] { application, applicant } ) );
Batch Executor and Command Factory are particularly useful when working with multiple Commands and with output identifiers for obtaining results.
KieCommands kieCommands = kieServices.getCommands();
List<Command> cmds = new ArrayList<Command>();
cmds.add( kieCommands.newInsert( new Person( "Mr John Smith" ), "mrSmith", true, null ) );
cmds.add( kieCommands.newInsert( new Person( "Mr John Doe" ), "mrDoe", true, null ) );
BatchExecutionResults results = ksession.execute( kieCommands.newBatchExecution( cmds ) );
assertEquals( new Person( "Mr John Smith" ), results.getValue( "mrSmith" ) );
CommandFactory
supports many other Commands that can be used in the BatchExecutor
like StartProcess
, Query
, and SetGlobal
.
4.1.2. Stateful KIE session
Stateful Sessions are long lived and allow iterative changes over time. Some common use cases for Stateful Sessions are, but not limited to:
-
Monitoring
-
Stock market monitoring and analysis for semi-automatic buying.
-
-
Diagnostics
-
Fault finding, medical diagnostics
-
-
Logistics
-
Parcel tracking and delivery provisioning
-
-
Compliance
-
Validation of legality for market trades.
-
In contrast to a Stateless Session, the dispose()
method must be called afterwards to ensure there are no memory leaks, as the KieBase contains references to Stateful KIE sessions when they are created.
Since Stateful KIE session is the most commonly used session type it is just named KieSession
in the KIE API. KieSession
also supports the BatchExecutor
interface, like StatelessKieSession
, the only difference being that the FireAllRules
command is not automatically called at the end for a Stateful Session.
We illustrate the monitoring use case with an example for raising a fire alarm.
Using just four classes, we represent rooms in a house, each of which has one sprinkler.
If a fire starts in a room, we represent that with a single Fire
instance.
public class Room {
private String name
// getter and setter methods here
}
public class Sprinkler {
private Room room;
private boolean on;
// getter and setter methods here
}
public class Fire {
private Room room;
// getter and setter methods here
}
public class Alarm {
}
In the previous section on Stateless Sessions the concepts of inserting and matching against data were introduced. That example assumed that only a single instance of each object type was ever inserted and thus only used literal constraints. However, a house has many rooms, so rules must express relationships between objects, such as a sprinkler being in a certain room. This is best done by using a binding variable as a constraint in a pattern. This "join" process results in what is called cross products, which are covered in the next section.
When a fire occurs an instance of the Fire
class is created, for that room, and inserted into the session.
The rule uses a binding on the room
field of the Fire
object to constrain matching to the sprinkler for that room, which is currently off.
When this rule fires and the consequence is executed the sprinkler is turned on.
rule "When there is a fire turn on the sprinkler"
when
Fire($room : room)
$sprinkler : Sprinkler( room == $room, on == false )
then
modify( $sprinkler ) { setOn( true ) };
System.out.println( "Turn on the sprinkler for room " + $room.getName() );
end
Whereas the Stateless Session uses standard Java syntax to modify a field, in the above rule we use the modify
statement, which acts as a sort of "with" statement.
It may contain a series of comma separated Java expressions, i.e., calls to setters of the object selected by the modify
statement’s control expression.
This modifies the data, and makes the Drools engine aware of those changes so it can reason over them once more.
This process is called inference, and it’s essential for the working of a Stateful Session.
Stateless Sessions typically do not use inference, so the Drools engine does not need to be aware of changes to data.
Inference can also be turned off explicitly by using the sequential mode.
So far we have rules that tell us when matching data exists, but what about when it does not exist? How do we determine that a fire has been extinguished, i.e., that there isn’t a Fire
object any more? Previously the constraints have been sentences according to Propositional Logic, where the Drools engine is constraining against individual instances.
Drools also has support for First Order Logic that allows you to look at sets of data.
A pattern under the keyword not
matches when something does not exist.
The rule given below turns the sprinkler off as soon as the fire in that room has disappeared.
rule "When the fire is gone turn off the sprinkler"
when
$room : Room( )
$sprinkler : Sprinkler( room == $room, on == true )
not Fire( room == $room )
then
modify( $sprinkler ) { setOn( false ) };
System.out.println( "Turn off the sprinkler for room " + $room.getName() );
end
While there is one sprinkler per room, there is just a single alarm for the building.
An Alarm
object is created when a fire occurs, but only one Alarm
is needed for the entire building, no matter how many fires occur.
Previously not
was introduced to match the absence of a fact; now we use its complement exists
which matches for one or more instances of some category.
rule "Raise the alarm when we have one or more fires"
when
exists Fire()
then
insert( new Alarm() );
System.out.println( "Raise the alarm" );
end
Likewise, when there are no fires we want to remove the alarm, so the not
keyword can be used again.
rule "Cancel the alarm when all the fires have gone"
when
not Fire()
$alarm : Alarm()
then
delete( $alarm );
System.out.println( "Cancel the alarm" );
end
Finally there is a general health status message that is printed when the application first starts and after the alarm is removed and all sprinklers have been turned off.
rule "Status output when things are ok"
when
not Alarm()
not Sprinkler( on == true )
then
System.out.println( "Everything is ok" );
end
As we did in the Stateless Session example, the above rules should be placed in a single DRL file and saved into the resouces folder of your Maven project or any of its subfolder.
As before, we can then obtain a KieSession
from the KieContainer
.
The only difference is that this time we create a Stateful Session, whereas before we created a Stateless Session.
KieServices kieServices = KieServices.Factory.get();
KieContainer kContainer = kieServices.getKieClasspathContainer();
KieSession ksession = kContainer.newKieSession();
With the session created it is now possible to iteratively work with it over time.
Four Room
objects are created and inserted, as well as one Sprinkler
object for each room.
At this point the Drools engine has done all of its matching, but no rules have fired yet.
Calling ksession.fireAllRules()
allows the matched rules to fire, but without a fire that will just produce the health message.
String[] names = new String[]{"kitchen", "bedroom", "office", "livingroom"};
Map<String,Room> name2room = new HashMap<String,Room>();
for( String name: names ){
Room room = new Room( name );
name2room.put( name, room );
ksession.insert( room );
Sprinkler sprinkler = new Sprinkler( room );
ksession.insert( sprinkler );
}
ksession.fireAllRules();
> Everything is ok
We now create two fires and insert them; this time a reference is kept for the returned FactHandle
.
A Fact Handle is an internal engine reference to the inserted instance and allows instances to be retracted or modified at a later point in time.
With the fires now in the Drools engine, once fireAllRules()
is called, the alarm is raised and the respective sprinklers are turned on.
Fire kitchenFire = new Fire( name2room.get( "kitchen" ) );
Fire officeFire = new Fire( name2room.get( "office" ) );
FactHandle kitchenFireHandle = ksession.insert( kitchenFire );
FactHandle officeFireHandle = ksession.insert( officeFire );
ksession.fireAllRules();
> Raise the alarm
> Turn on the sprinkler for room kitchen
> Turn on the sprinkler for room office
After a while the fires will be put out and the Fire
instances are retracted.
This results in the sprinklers being turned off, the alarm being cancelled, and eventually the health message is printed again.
ksession.delete( kitchenFireHandle );
ksession.delete( officeFireHandle );
ksession.fireAllRules();
> Cancel the alarm
> Turn off the sprinkler for room office
> Turn off the sprinkler for room kitchen
> Everything is ok
Everyone still with me? That wasn’t so hard and already I’m hoping you can start to see the value and power of a declarative rule system.
4.1.3. Methods versus Rules
People often confuse methods and rules, and new rule users often ask, "How do I call a rule?" After the last section, you are now feeling like a rule expert and the answer to that is obvious, but let’s summarize the differences nonetheless.
public void helloWorld(Person person) {
if ( person.getName().equals( "Chuck" ) ) {
System.out.println( "Hello Chuck" );
}
}
-
Methods are called directly.
-
Specific instances are passed.
-
One call results in a single execution.
rule "Hello World" when
Person( name == "Chuck" )
then
System.out.println( "Hello Chuck" );
end
-
Rules execute by matching against any data as long it is inserted into the Drools engine.
-
Rules can never be called directly.
-
Specific instances cannot be passed to a rule.
-
Depending on the matches, a rule may fire once or several times, or not at all.
4.1.4. Cross Products
Earlier the term "cross product" was mentioned, which is the result of a join. Imagine for a moment that the data from the fire alarm example were used in combination with the following rule where there are no field constraints:
rule "Show Sprinklers" when
$room : Room()
$sprinkler : Sprinkler()
then
System.out.println( "room:" + $room.getName() +
" sprinkler:" + $sprinkler.getRoom().getName() );
end
In SQL terms this would be like doing select * from Room,
Sprinkler
and every row in the Room table would be joined with every row in the Sprinkler table resulting in the following output:
room:office sprinkler:office
room:office sprinkler:kitchen
room:office sprinkler:livingroom
room:office sprinkler:bedroom
room:kitchen sprinkler:office
room:kitchen sprinkler:kitchen
room:kitchen sprinkler:livingroom
room:kitchen sprinkler:bedroom
room:livingroom sprinkler:office
room:livingroom sprinkler:kitchen
room:livingroom sprinkler:livingroom
room:livingroom sprinkler:bedroom
room:bedroom sprinkler:office
room:bedroom sprinkler:kitchen
room:bedroom sprinkler:livingroom
room:bedroom sprinkler:bedroom
These cross products can obviously become huge, and they may very well contain spurious data. The size of cross products is often the source of performance problems for new rule authors. From this it can be seen that it’s always desirable to constrain the cross products, which is done with the variable constraint.
rule
when
$room : Room()
$sprinkler : Sprinkler( room == $room )
then
System.out.println( "room:" + $room.getName() +
" sprinkler:" + $sprinkler.getRoom().getName() );
end
This results in just four rows of data, with the correct Sprinkler for each Room.
In SQL (actually HQL) the corresponding query would be select * from Room, Sprinkler where Room ==
Sprinkler.room
.
room:office sprinkler:office
room:kitchen sprinkler:kitchen
room:livingroom sprinkler:livingroom
room:bedroom sprinkler:bedroom
4.2. Execution Control
4.2.1. Agenda
The Agenda is a Rete feature. It maintains set of rules that are able to execute, its job is to schedule that execution in a deterministic order.
During actions on the RuleRuntime
, rules may become fully matched and eligible for execution; a single Rule Runtime Action can result in multiple eligible rules.
When a rule is fully matched a Rule Match is created, referencing the rule and the matched facts, and placed onto the Agenda.
The Agenda controls the execution order of these Matches using a Conflict Resolution strategy.
The Drools engine cycles repeatedly through two phases:
-
Rule Runtime Actions. This is where most of the work takes place, either in the Consequence (the RHS itself) or the main Java application process. Once the Consequence has finished or the main Java application process calls
fireAllRules()
the Drools engine switches to the Agenda Evaluation phase. -
Agenda Evaluation. This attempts to select a rule to fire. If no rule is found it exits, otherwise it fires the found rule, switching the phase back to Rule Runtime Actions.
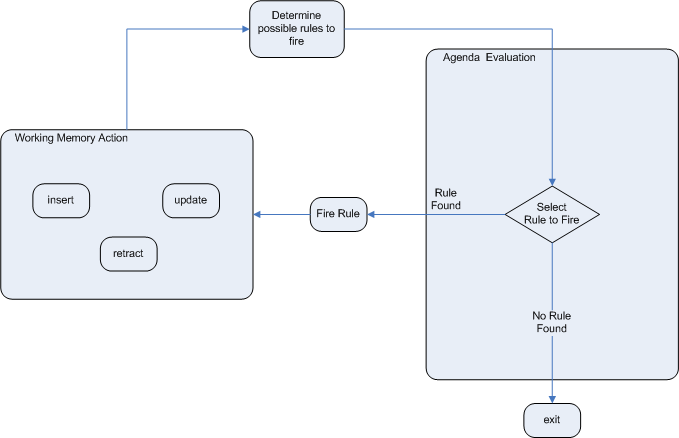
The process repeats until the agenda is clear, in which case control returns to the calling application. When Rule Runtime Actions are taking place, no rules are being fired.
4.2.2. Rule Matches and Conflict Sets.
4.2.2.1. Cashflow Example
So far the data and the matching process has been simple and small. To mix things up a bit a new example will be explored that handles cashflow calculations over date periods. The state of the Drools engine will be illustratively shown at key stages to help get a better understanding of what is actually going on under the hood. Three classes will be used, as shown below. This will help us grow our understanding of pattern matching and joins further. We will then use this to illustrate different techniques for execution control.
public class CashFlow {
private Date date;
private double amount;
private int type;
long accountNo;
// getter and setter methods here
}
public class Account {
private long accountNo;
private double balance;
// getter and setter methods here
}
public AccountPeriod {
private Date start;
private Date end;
// getter and setter methods here
}
By now you already know how to create KieBases and how to instantiate facts to populate the KieSession
, so tables will be used to show the state of the inserted data, as it makes things clearer for illustration purposes.
The tables below show that a single fact was inserted for the Account
.
Also inserted are a series of debits and credits as CashFlow
objects for that account, extending over two quarters.
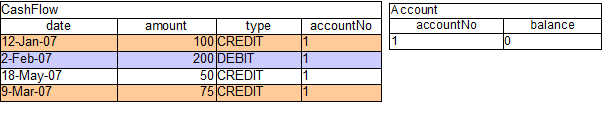
Two rules can be used to determine the debit and credit for that quarter and update the Account balance. The two rules below constrain the cashflows for an account for a given time period. Notice the "&&" which use short cut syntax to avoid repeating the field name twice.
|
|
Earlier we showed how rules would equate to SQL, which can often help people with an SQL background to understand rules. The two rules above can be represented with two views and a trigger for each view, as below:
|
|
|
|
If the AccountPeriod
is set to the first quarter we constrain the rule "increase balance for credits" to fire on two rows of data and "decrease balance for debits" to act on one row of data.
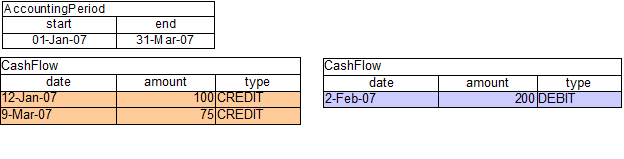
The two cashflow tables above represent the matched data for the two rules.
The data is matched during the insertion stage and, as you discovered in the previous chapter, does not fire straight away, but only after fireAllRules()
is called.
Meanwhile, the rule plus its matched data is placed on the Agenda and referred to as an RuIe Match or Rule Instance.
The Agenda is a table of Rule Matches that are able to fire and have their consequences executed, as soon as fireAllRules() is called.
Rule Matches on the Agenda are referred to as a conflict set
and their execution is determine by a conflict resolution strategy.
Notice that the order of execution so far is considered arbitrary.
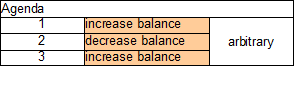
After all of the above activations are fired, the account has a balance of -25.
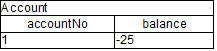
If the AccountPeriod
is updated to the second quarter, we have just a single matched row of data, and thus just a single Rule Match on the Agenda.
The firing of that Activation results in a balance of 25.
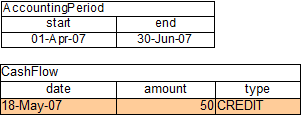
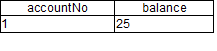
4.2.2.2. Conflict Resolution
What if you don’t want the order of rule execution to be arbitrary? When there is one or more Rule Match on the Agenda they are said to be in conflict, and a conflict resolution strategy is used to determine the order of execution. The Drools strategy is very simple and based around a salience value, which assigns a priority to a rule. Each rule has a default value of 0, the higher the value the higher the priority.
As a general rule, it is a good idea not to count on rules firing in any particular order, and to author the rules without worrying about a "flow". However when a flow is needed a number of possibilities exist beyond salience: agenda groups, rule flow groups, activation groups and control/semaphore facts.
As of Drools 6.0 rule definition order in the source file is used to set priority after salience.
4.2.2.3. Salience
To illustrate Salience we add a rule to print the account balance, where we want this rule to be executed after all the debits and credits have been applied for all accounts. We achieve this by assigning a negative salience to this rule so that it fires after all rules with the default salience 0.
|
The table below depicts the resulting Agenda. The three debit and credit rules are shown to be in arbitrary order, while the print rule is ranked last, to execute afterwards.
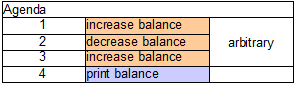
4.2.2.4. Agenda Groups
Agenda groups allow you to place rules into groups, and to place those groups onto a stack. The stack has push/pop bevaviour. Calling "setFocus" places the group onto the stack:
ksession.getAgenda().getAgendaGroup( "Group A" ).setFocus();
The agenda always evaluates the top of the stack. When all the rules have fired for a group, it is popped from the stack and the next group is evaluated.
|
|
First set the focus to the "report" group and then by placing the focus on "calculation" we ensure that group is evaluated first.
Agenda agenda = ksession.getAgenda();
agenda.getAgendaGroup( "report" ).setFocus();
agenda.getAgendaGroup( "calculation" ).setFocus();
ksession.fireAllRules();
4.2.2.5. Rule Flow
Drools also features ruleflow-group attributes which allows workflow diagrams to declaratively specify when rules are allowed to fire. The screenshot below is taken from Eclipse using the Drools plugin. It has two ruleflow-group nodes which ensures that the calculation rules are executed before the reporting rules.
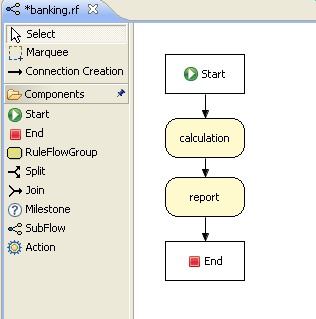
The use of the ruleflow-group attribute in a rule is shown below.
|
|
4.3. Inference
4.3.1. Bus Pass Example
Inference has a bad name these days, as something not relevant to business use cases and just too complicated to be useful. It is true that contrived and complicated examples occur with inference, but that should not detract from the fact that simple and useful ones exist too. But more than this, correct use of inference can crate more agile and less error prone business rules, which are easier to maintain.
So what is inference? Something is inferred when we gain knowledge of something from using previous knowledge. For example, given a Person fact with an age field and a rule that provides age policy control, we can infer whether a Person is an adult or a child and act on this.
rule "Infer Adult"
when
$p : Person( age >= 18 )
then
insert( new IsAdult( $p ) )
end
Due to the preceding rule, every Person who is 18 or over will have an instance of IsAdult inserted for them. This fact is special in that it is known as a relation. We can use this inferred relation in any rule:
$p : Person()
IsAdult( person == $p )
So now we know what inference is, and have a basic example, how does this facilitate good rule design and maintenance?
Let’s take a government department that are responsible for issuing ID cards when children become adults, henceforth referred to as ID department. They might have a decision table that includes logic like this, which says when an adult living in London is 18 or over, issue the card:
RuleTable ID Card |
|||
CONDITION |
CONDITION |
ACTION |
|
p : Person |
|||
location |
age >= $1 |
issueIdCard($1) |
|
Select Person |
Select Adults |
Issue ID Card |
|
Issue ID Card to Adults |
London |
18 |
p |
However the ID department does not set the policy on who an adult is. That’s done at a central government level. If the central government were to change that age to 21, this would initiate a change management process. Someone would have to liaise with the ID department and make sure their systems are updated, in time for the law going live.
This change management process and communication between departments is not ideal for an agile environment, and change becomes costly and error prone. Also the card department is managing more information than it needs to be aware of with its "monolithic" approach to rules management which is "leaking" information better placed elsewhere. By this I mean that it doesn’t care what explicit "age >= 18" information determines whether someone is an adult, only that they are an adult.
In contrast to this, let’s pursue an approach where we split (de-couple) the authoring responsibilities, so that both the central government and the ID department maintain their own rules.
It’s the central government’s job to determine who is an adult. If they change the law they just update their central repository with the new rules, which others use:
RuleTable Age Policy |
||
CONDITION |
ACTION |
|
p : Person |
||
age >= $1 |
insert($1) |
|
Adult Age Policy |
Add Adult Relation |
|
Infer Adult |
18 |
new IsAdult( p ) |
The IsAdult fact, as discussed previously, is inferred from the policy rules. It encapsulates the seemingly arbitrary piece of logic "age >= 18" and provides semantic abstractions for its meaning. Now if anyone uses the above rules, they no longer need to be aware of explicit information that determines whether someone is an adult or not. They can just use the inferred fact:
RuleTable ID Card |
|||
CONDITION |
CONDITION |
ACTION |
|
p : Person |
isAdult |
||
location |
person == $1 |
issueIdCard($1) |
|
Select Person |
Select Adults |
Issue ID Card |
|
Issue ID Card to Adults |
London |
p |
p |
While the example is very minimal and trivial it illustrates some important points. We started with a monolithic and leaky approach to our knowledge engineering. We created a single decision table that had all possible information in it and that leaks information from central government that the ID department did not care about and did not want to manage.
We first de-coupled the knowledge process so each department was responsible for only what it needed to know. We then encapsulated this leaky knowledge using an inferred fact IsAdult. The use of the term IsAdult also gave a semantic abstraction to the previously arbitrary logic "age >= 18".
So a general rule of thumb when doing your knowledge engineering is:
-
Bad
-
Monolithic
-
Leaky
-
-
Good
-
De-couple knowledge responsibilities
-
Encapsulate knowledge
-
Provide semantic abstractions for those encapsulations
-
4.4. Truth Maintenance with Logical Objects
4.4.1. Overview
After regular inserts you have to retract facts explicitly. With logical assertions, the fact that was asserted will be automatically retracted when the conditions that asserted it in the first place are no longer true. Actually, it’s even cleverer then that, because it will be retracted only if there isn’t any single condition that supports the logical assertion.
Normal insertions are said to be stated, i.e., just like the intuitive meaning of "stating a fact" implies.
Using a HashMap
and a counter, we track how many times a particular equality is stated; this means we count how many different instances are equal.
When we logically insert an object during a RHS execution we are said to justify it, and it is considered to be justified by the firing rule. For each logical insertion there can only be one equal object, and each subsequent equal logical insertion increases the justification counter for this logical assertion. A justification is removed by the LHS of the creating rule becoming untrue, and the counter is decreased accordingly. As soon as we have no more justifications the logical object is automatically retracted.
If we try to logically insert an object when there is an equal stated object, this will fail and return null.
If we state an object that has an existing equal object that is justified we override the Fact; how this override works depends on the configuration setting WM_BEHAVIOR_PRESERVE
.
When the property is set to discard we use the existing handle and replace the existing instance with the new Object, which is the default behavior; otherwise we override it to stated but we create an new FactHandle
.
This can be confusing on a first read, so hopefully the flow charts below help.
When it says that it returns a new FactHandle
, this also indicates the Object
was propagated through the network.
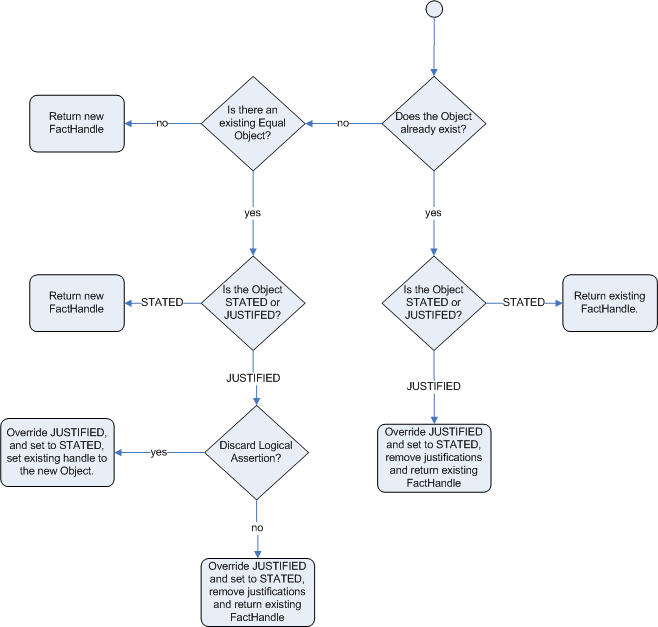
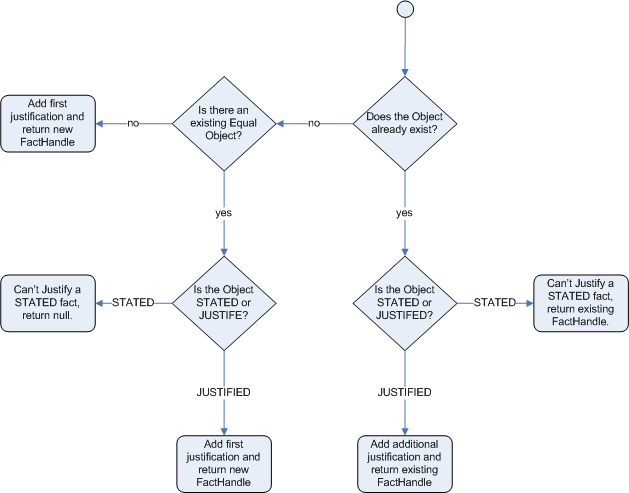
4.4.1.1. Bus Pass Example With Inference and TMS
The previous example was issuing ID cards to over 18s, in this example we now issue bus passes, either a child or adult pass.
rule "Issue Child Bus Pass" when
$p : Person( age < 16 )
then
insert(new ChildBusPass( $p ) );
end
rule "Issue Adult Bus Pass" when
$p : Person( age >= 16 )
then
insert(new AdultBusPass( $p ) );
end
As before the above example is considered monolithic, leaky and providing poor separation of concerns.
As before we can provide a more robust application with a separation of concerns using inference. Notice this time we don’t just insert the inferred object, we use "insertLogical":
rule "Infer Child" when
$p : Person( age < 16 )
then
insertLogical( new IsChild( $p ) )
end
rule "Infer Adult" when
$p : Person( age >= 16 )
then
insertLogical( new IsAdult( $p ) )
end
A "insertLogical" is part of the Drools Truth Maintenance System (TMS). When a fact is logically inserted, this fact is dependant on the truth of the "when" clause. It means that when the rule becomes false the fact is automatically retracted. This works particularly well as the two rules are mutually exclusive. So in the above rules if the person is under 16 it inserts an IsChild fact, once the person is 16 or over the IsChild fact is automatically retracted and the IsAdult fact inserted.
Returning to the code to issue bus passes, these two rules can + logically insert the ChildBusPass and AdultBusPass facts, as the TMS + supports chaining of logical insertions for a cascading set of retracts.
rule "Issue Child Bus Pass" when
$p : Person( )
IsChild( person == $p )
then
insertLogical(new ChildBusPass( $p ) );
end
rule "Issue Adult Bus Pass" when
$p : Person( age >= 16 )
IsAdult( person =$p )
then
insertLogical(new AdultBusPass( $p ) );
end
Now when a person changes from being 15 to 16, not only is the IsChild fact automatically retracted, so is the person’s ChildBusPass fact. For bonus points we can combine this with the 'not' conditional element to handle notifications, in this situation, a request for the returning of the pass. So when the TMS automatically retracts the ChildBusPass object, this rule triggers and sends a request to the person:
rule "Return ChildBusPass Request "when
$p : Person( )
not( ChildBusPass( person == $p ) )
then
requestChildBusPass( $p );
end
4.4.1.2. Important note: Equality for Java objects
It is important to note that for Truth Maintenance (and logical assertions) to work at all, your Fact objects (which may be JavaBeans) must override equals and hashCode methods (from java.lang.Object) correctly. As the truth maintenance system needs to know when two different physical objects are equal in value, both equals and hashCode must be overridden correctly, as per the Java standard.
Two objects are equal if and only if their equals methods return true for each other and if their hashCode methods return the same values. See the Java API for more details (but do keep in mind you MUST override both equals and hashCode).
TMS behaviour is not affected by theruntime configuration of Identity vs Equality, TMS is always equality.
4.4.1.3. Deleting stated or logically asserted facts from the working memory
By default when a fact is deleted from the working memory Drools attempts to remove it both from the set of stated facts and also from the Truth Maintenance System in case it has been logically asserted. However, using an overload of the delete method, it is also possible to remove it only from one of the 2. For instance invoking:
ksession.delete( factHandle, FactHandle.State.LOGICAL );
the fact is removed only if it has been logically asserted, but not if it is a stated fact. In this case, if the fact has been stated its deletion fails silently and it is ignored.
4.5. Logging
One way to illuminate the black box that is the Drools engine is to play with the logging level.
Everything is logged to SLF4J, which is a simple logging facade that can delegate any log to Logback, Apache Commons Logging, Log4j or java.util.logging. Add a dependency to the logging adaptor for your logging framework of choice. If you’re not using any logging framework yet, you can use Logback by adding this Maven dependency:
<dependency>
<groupId>ch.qos.logback</groupId>
<artifactId>logback-classic</artifactId>
<version>1.x</version>
</dependency>
If you’re developing for an ultra light environment, use |
Configure the logging level on the package org.drools
.
For example:
In Logback, configure it in your logback.xml file:
<configuration>
<logger name="org.drools" level="debug"/>
...
<configuration>
In Log4J, configure it in your log4j.xml file:
<log4j:configuration xmlns:log4j="http://jakarta.apache.org/log4j/">
<category name="org.drools">
<priority value="debug" />
</category>
...
</log4j:configuration>
5. Running
Ths sections extends the KIE Running section, which should be read first, with specifics for the Drools runtime.
5.1. KieRuntime
5.1.1. EntryPoint
The EntryPoint
provides the methods around inserting, updating and deleting facts.
The term "entry point" is related to the fact that we have multiple partitions in a Working Memory and you can choose which one you are inserting into.
The use of multiple entry points is more common in event processing use cases, but they can be used by pure rule applications as well.
The KieRuntime
interface provides the main interaction with the Drools engine.
It is available in rule consequences and process actions.
In this manual the focus is on the methods and interfaces related to rules, and the methods pertaining to processes will be ignored for now.
But you’ll notice that the KieRuntime
inherits methods from both the WorkingMemory
and the ProcessRuntime
, thereby providing a unified API to work with processes and rules.
When working with rules, three interfaces form the KieRuntime
: EntryPoint
, WorkingMemory
and the KieRuntime
itself.
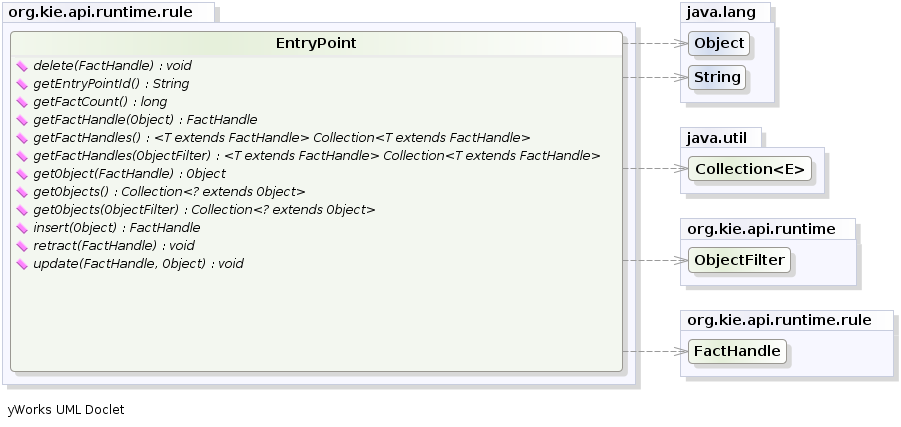
5.1.1.1. Insert
In order for a fact to be evaluated against the rules in a KieBase
, it has to be inserted into the session.
This is done by calling the method insert(yourObject)
.
When a fact is inserted into the session, some of its properties might be immediately evaluated (eager evaluation) and some might be deferred for later evaluation (lazy evaluation). The exact behaviour depends on the Drools engine algorithm being used.
Expert systems typically use the term assert or assertion to refer to facts made available to the system.
However, due to "assert" being a keyword in most languages, we have decided to use the |
When an Object is inserted it returns a FactHandle
.
This FactHandle
is the token used to represent your inserted object within the WorkingMemory
.
It is also used for interactions with the WorkingMemory
when you wish to delete or modify an object.
Cheese stilton = new Cheese("stilton");
FactHandle stiltonHandle = ksession.insert( stilton );
As mentioned in the KieBase section, a Working Memory may operate in two assertion modes: either equality or identity. Identity is the default.
Identity means that the Working Memory uses an IdentityHashMap
to store all asserted objects.
New instance assertions always result in the return of new FactHandle
, but if an instance is asserted again then it returns the original fact handle, i.e., it ignores repeated insertions for the same object.
Equality means that the Working Memory uses a HashMap
to store all asserted objects.
An object instance assertion will only return a new FactHandle
if the inserted object is not equal (according to its equal()/hashcode()
methods) to an already existing fact.
5.1.1.2. Delete
In order to remove a fact from the session, the method delete()
is used.
When a fact is deleted, any matches that are active and depend on that fact will be cancelled.
Note that it is possible to have rules that depend on the nonexistence of a fact, in which case deleting a fact may cause a rule to activate.
(See the not
and exists
keywords).
Expert systems typically use the term retract or retraction to refer to the operation of removing facts from the Working Memory.
Drools prefers the keyword |
Retraction may be done using the FactHandle
that was returned by the insert call.
On the right hand side of a rule the delete
statement is used, which works with a simple object reference.
Cheese stilton = new Cheese("stilton");
FactHandle stiltonHandle = ksession.insert( stilton );
....
ksession.delete( stiltonHandle );
5.1.1.3. Update
The Drools engine must be notified of modified facts, so that they can be reprocessed.
You must use the update()
method to notify the WorkingMemory
of changed objects for those objects that are not able to notify the WorkingMemory
themselves.
Notice that update()
always takes the modified object as a second parameter, which allows you to specify new instances for immutable objects.
On the right hand side of a rule the modify
statement is recommended, as it makes the changes and notifies the Drools engine in a single statement.
Alternatively, after changing a fact object’s field values through calls of setter methods you must invoke update
immediately, event before changing another fact, or you will cause problems with the indexing within the Drools engine.
The modify statement avoids this problem.
Cheese stilton = new Cheese("stilton");
FactHandle stiltonHandle = workingMemory.insert( stilton );
...
stilton.setPrice( 100 );
workingMemory.update( stiltonHandle, stilton );
5.1.2. RuleRuntime
The RuleRuntime provides access to the Agenda, permits query executions, and lets you access named Entry Points.
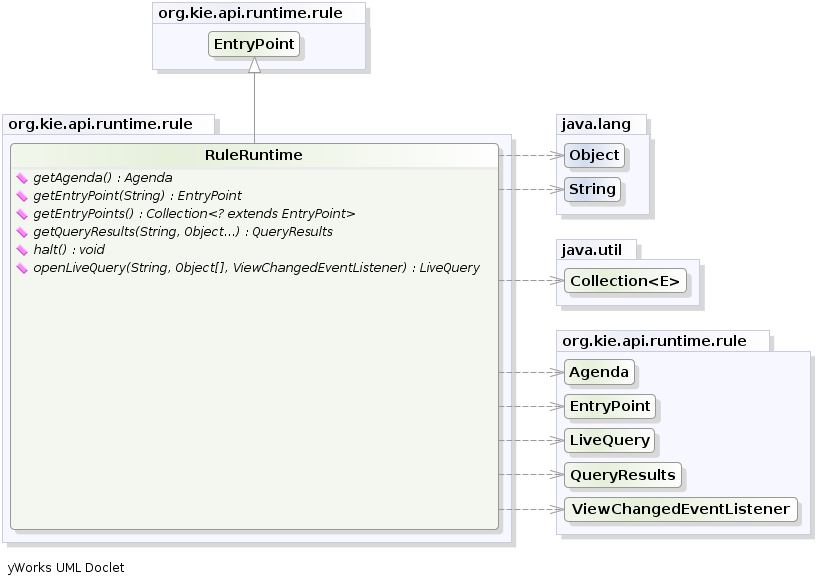
5.1.2.1. Query
Queries are used to retrieve fact sets based on patterns, as they are used in rules.
Patterns may make use of optional parameters.
Queries can be defined in the KIE base, from where they are called up to return the matching results.
While iterating over the result collection, any identifier bound in the query can be used to access the corresponding fact or fact field by calling the get
method with the binding variable’s name as its argument.
If the binding refers to a fact object, its FactHandle can be retrieved by calling getFactHandle
, again with the variable’s name as the parameter.
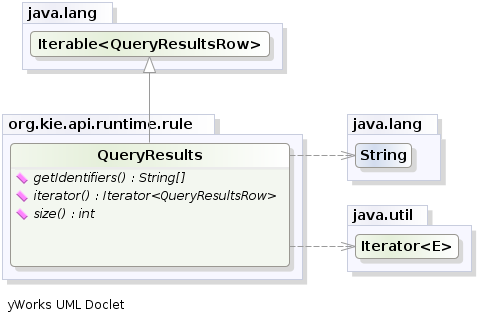
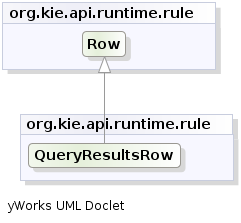
QueryResults results =
ksession.getQueryResults( "my query", new Object[] { "string" } );
for ( QueryResultsRow row : results ) {
System.out.println( row.get( "varName" ) );
}
5.1.2.2. Live Queries
Invoking queries and processing the results by iterating over the returned set is not a good way to monitor changes over time.
To alleviate this, Drools provides Live Queries, which have a listener attached instead of returning an iterable result set.
These live queries stay open by creating a view and publishing change events for the contents of this view.
To activate, you start your query with parameters and listen to changes in the resulting view.
The dispose
method terminates the query and discontinues this reactive scenario.
final List updated = new ArrayList();
final List removed = new ArrayList();
final List added = new ArrayList();
ViewChangedEventListener listener = new ViewChangedEventListener() {
public void rowUpdated(Row row) {
updated.add( row.get( "$price" ) );
}
public void rowRemoved(Row row) {
removed.add( row.get( "$price" ) );
}
public void rowAdded(Row row) {
added.add( row.get( "$price" ) );
}
};
// Open the LiveQuery
LiveQuery query = ksession.openLiveQuery( "cheeses",
new Object[] { "cheddar", "stilton" },
listener );
...
...
query.dispose() // calling dispose to terminate the live query
A Drools blog article contains an example of Glazed Lists integration for live queries:
5.1.3. StatefulRuleSession
The StatefulRuleSession
is inherited by the KieSession
and provides the rule related methods that are relevant from outside of the Drools engine.
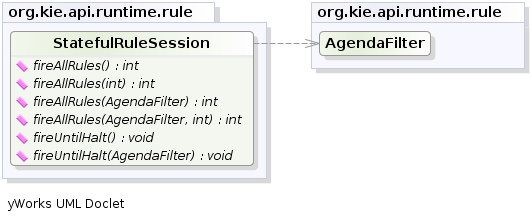
5.1.3.1. Agenda Filters
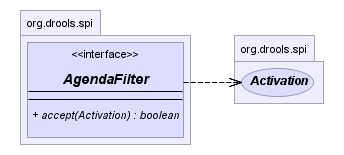
` AgendaFilter` objects are optional implementations of the filter interface which are used to allow or deny the firing of a match. What you filter on is entirely up to the implementation. Drools 4.0 used to supply some out of the box filters, which have not be exposed in drools 5.0 knowledge-api, but they are simple to implement and the Drools 4.0 code base can be referred to.
To use a filter specify it while calling fireAllRules()
.
The following example permits only rules ending in the string "Test"
.
All others will be filtered out.
ksession.fireAllRules( new RuleNameEndsWithAgendaFilter( "Test" ) );
5.2. Agenda
The Agenda is a Rete feature.
During actions on the WorkingMemory
, rules may become fully matched and eligible for execution; a single Working Memory Action can result in multiple eligible rules.
When a rule is fully matched a Match is created, referencing the rule and the matched facts, and placed onto the Agenda.
The Agenda controls the execution order of these Matches using a Conflict Resolution strategy.
The Drools engine cycles repeatedly through two phases:
-
Working Memory Actions. This is where most of the work takes place, either in the Consequence (the RHS itself) or the main Java application process. Once the Consequence has finished or the main Java application process calls
fireAllRules()
the Drools engine switches to the Agenda Evaluation phase. -
Agenda Evaluation. This attempts to select a rule to fire. If no rule is found it exits, otherwise it fires the found rule, switching the phase back to Working Memory Actions.
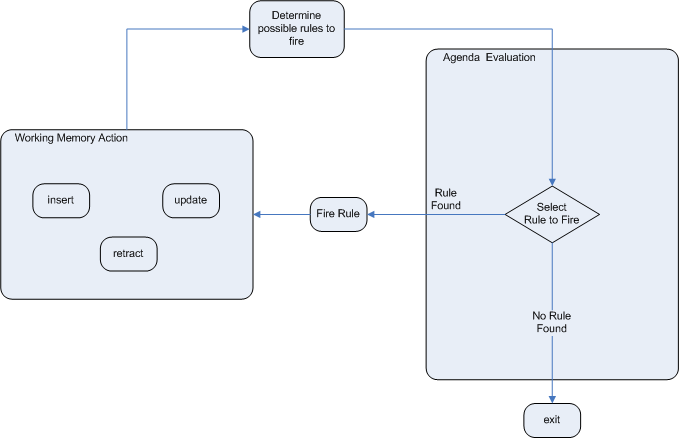
The process repeats until the agenda is clear, in which case control returns to the calling application. When Working Memory Actions are taking place, no rules are being fired.
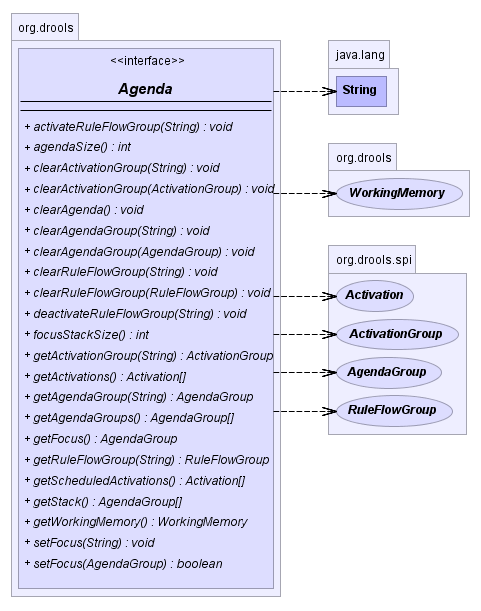
5.2.1. Conflict Resolution
Conflict resolution is required when there are multiple rules on the agenda. (The basics to this are covered in chapter "Quick Start".) As firing a rule may have side effects on the working memory, the Drools engine needs to know in what order the rules should fire (for instance, firing ruleA may cause ruleB to be removed from the agenda).
The default conflict resolution strategies employed by Drools are: Salience and LIFO (last in, first out).
The most visible one is salience (or priority), in which case a user can specify that a certain rule has a higher priority (by giving it a higher number) than other rules. In that case, the rule with higher salience will be preferred. LIFO priorities are based on the assigned Working Memory Action counter value, with all rules created during the same action receiving the same value. The execution order of a set of firings with the same priority value is arbitrary.
As a general rule, it is a good idea not to count on rules firing in any particular order, and to author the rules without worrying about a "flow". However when a flow is needed a number of possibilities exist, including but not limited to: agenda groups, rule flow groups, activation groups, control/semaphore facts. These are discussed in later sections.
Drools 4.0 supported custom conflict resolution strategies; while this capability still exists in Drools it has not yet been exposed to the end user via knowledge-api in Drools 5.0.
5.2.2. AgendaGroup
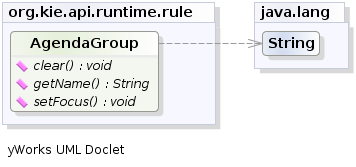
Agenda groups are a way to partition rules (matches, actually) on the agenda. At any one time, only one group has "focus" which means that matches for rules in that group only will take effect. You can also have rules with "auto focus" which means that the focus is taken for its agenda group when that rule’s conditions are true.
Agenda groups are known as "modules" in CLIPS terminology. While it best to design rules that do not need control flow, this is not always possible. Agenda groups provide a handy way to create a "flow" between grouped rules. You can switch the group which has focus either from within the Drools engine, or via the API. If your rules have a clear need for multiple "phases" or "sequences" of processing, consider using agenda-groups for this purpose.
Each time setFocus()
is called it pushes that Agenda Group onto a stack.
When the focus group is empty it is popped from the stack and the focus group that is now on top evaluates.
An Agenda Group can appear in multiple locations on the stack.
The default Agenda Group is "MAIN", with all rules which do not specify an Agenda Group being in this group.
It is also always the first group on the stack, given focus initially, by default.
ksession.getAgenda().getAgendaGroup( "Group A" ).setFocus();
The clear()
method can be used to cancel all the activations generated by the rules belonging to a given Agenda Group before one has had a chance to fire.
ksession.getAgenda().getAgendaGroup( "Group A" ).clear();
Note that, due to the lazy nature of the phreak algorithm used by Drools, the activations are by default materialized only at firing time, but it is possible to anticipate the evaluation and then the activation of a given rule at the moment when a fact is inserted into the session by annotating it with @Propagation(IMMEDIATE)
as explained in the Propagation modes section.
5.2.3. ActivationGroup
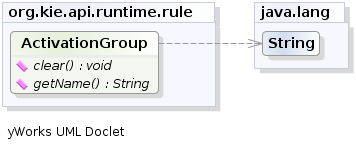
An activation group is a set of rules bound together by the same "activation-group" rule attribute.
In this group only one rule can fire, and after that rule has fired all the other rules are cancelled from the agenda.
The clear()
method can be called at any time, which cancels all of the activations before one has had a chance to fire.
ksession.getAgenda().getActivationGroup( "Group B" ).clear();
5.2.4. RuleFlowGroup
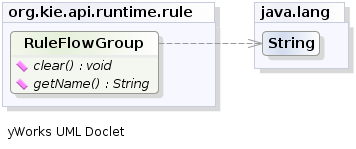
A rule flow group is a group of rules associated by the "ruleflow-group" rule attribute.
These rules can only fire when the group is activated.
The group itself can only become active when the elaboration of the ruleflow diagram reaches the node representing the group.
Here too, the clear()
method can be called at any time to cancels all matches still remaining on the Agenda.
ksession.getAgenda().getRuleFlowGroup( "Group C" ).clear();
5.3. Event Model
The event package provides means to be notified of Drools engine events, including rules firing, objects being asserted, etc. This allows you, for instance, to separate logging and auditing activities from the main part of your application (and the rules).
The WorkingMemoryEventManager
allows for listeners to be added and removed, so that events for the working memory and the agenda can be listened to.
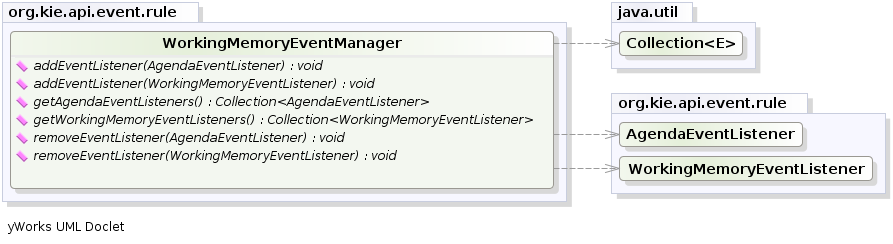
The following code snippet shows how a simple agenda listener is declared and attached to a session. It will print matches after they have fired.
ksession.addEventListener( new DefaultAgendaEventListener() {
public void afterMatchFired(AfterMatchFiredEvent event) {
super.afterMatchFired( event );
System.out.println( event );
}
});
Drools also provides DebugRuleRuntimeEventListener
and DebugAgendaEventListener
which implement each method with a debug print statement.
To print all Working Memory events, you add a listener like this:
ksession.addEventListener( new DebugRuleRuntimeEventListener() );
The events currently supported are:
-
MatchCreatedEvent
-
MatchCancelledEvent
-
BeforeMatchFiredEvent
-
AfterMatchFiredEvent
-
AgendaGroupPushedEvent
-
AgendaGroupPoppedEvent
-
ObjectInsertEvent
-
ObjectDeletedEvent
-
ObjectUpdatedEvent
-
ProcessCompletedEvent
-
ProcessNodeLeftEvent
-
ProcessNodeTriggeredEvent
-
ProcessStartEvent
5.4. StatelessKieSession
The StatelessKieSession
wraps the KieSession
, instead of extending it.
Its main focus is on decision service type scenarios.
It avoids the need to call dispose()
.
Stateless sessions do not support iterative insertions and the method call fireAllRules()
from Java code; the act of calling execute()
is a single-shot method that will internally instantiate a KieSession
, add all the user data and execute user commands, call fireAllRules()
, and then call dispose()
.
While the main way to work with this class is via the BatchExecution
(a subinterface of Command
) as supported by the CommandExecutor
interface, two convenience methods are provided for when simple object insertion is all that’s required.
The CommandExecutor
and BatchExecution
are talked about in detail in their own section.
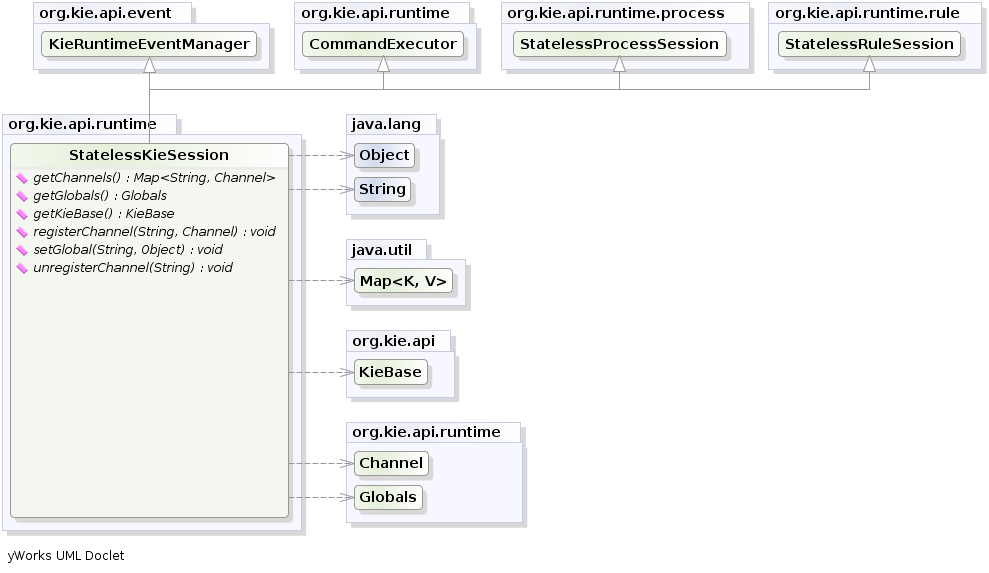
Our simple example shows a stateless session executing a given collection of Java objects using the convenience API. It will iterate the collection, inserting each element in turn.
StatelessKieSession ksession = kbase.newStatelessKieSession();
ksession.execute( collection );
If this was done as a single Command it would be as follows:
ksession.execute( CommandFactory.newInsertElements( collection ) );
If you wanted to insert the collection itself, and the collection’s individual elements, then CommandFactory.newInsert(collection)
would do the job.
Methods of the CommandFactory
create the supported commands, all of which can be marshalled using XStream and the BatchExecutionHelper
. BatchExecutionHelper
provides details on the XML format as well as how to use Drools Pipeline to automate the marshalling of BatchExecution
and ExecutionResults
.
StatelessKieSession
supports globals, scoped in a number of ways.
I’ll cover the non-command way first, as commands are scoped to a specific execution call.
Globals can be resolved in three ways.
-
The StatelessKieSession method
getGlobals()
returns a Globals instance which provides access to the session’s globals. These are shared for all execution calls. Exercise caution regarding mutable globals because execution calls can be executing simultaneously in different threads.Example 70. Session scoped globalStatelessKieSession ksession = kbase.newStatelessKieSession(); // Set a global hbnSession, that can be used for DB interactions in the rules. ksession.setGlobal( "hbnSession", hibernateSession ); // Execute while being able to resolve the "hbnSession" identifier. ksession.execute( collection );
-
Using a delegate is another way of global resolution. Assigning a value to a global (with
setGlobal(String, Object)
) results in the value being stored in an internal collection mapping identifiers to values. Identifiers in this internal collection will have priority over any supplied delegate. Only if an identifier cannot be found in this internal collection, the delegate global (if any) will be used. -
The third way of resolving globals is to have execution scoped globals. Here, a
Command
to set a global is passed to theCommandExecutor
.
The CommandExecutor
interface also offers the ability to export data via "out" parameters.
Inserted facts, globals and query results can all be returned.
// Set up a list of commands
List cmds = new ArrayList();
cmds.add( CommandFactory.newSetGlobal( "list1", new ArrayList(), true ) );
cmds.add( CommandFactory.newInsert( new Person( "jon", 102 ), "person" ) );
cmds.add( CommandFactory.newQuery( "Get People" "getPeople" );
// Execute the list
ExecutionResults results =
ksession.execute( CommandFactory.newBatchExecution( cmds ) );
// Retrieve the ArrayList
results.getValue( "list1" );
// Retrieve the inserted Person fact
results.getValue( "person" );
// Retrieve the query as a QueryResults instance.
results.getValue( "Get People" );
5.4.1. Sequential Mode
With Rete you have a stateful session where objects can be asserted and modified over time, and where rules can also be added and removed. Now what happens if we assume a stateless session, where after the initial data set no more data can be asserted or modified and rules cannot be added or removed? Certainly it won’t be necessary to re-evaluate rules, and the Drools engine will be able to operate in a simplified way.
-
Order the Rules by salience and position in the ruleset (by setting a sequence attribute on the rule terminal node).
-
Create an elements, one element for each possible rule match; element position indicates firing order.
-
Turn off all node memories, except the right-input Object memory.
-
Disconnect the Left Input Adapter Node propagation, and let the Object plus the Node be referenced in a Command object, which is added to a list on the Working Memory for later execution.
-
Assert all objects, and, when all assertions are finished and thus right-input node memories are populated, check the Command list and execute each in turn.
-
All resulting Matches should be placed in the elements, based upon the determined sequence number of the Rule. Record the first and last populated elements, to reduce the iteration range.
-
Iterate the elements of Matches, executing populated element in turn.
-
If we have a maximum number of allowed rule executions, we can exit our network evaluations early to fire all the rules in the elements.
The LeftInputAdapterNode
no longer creates a Tuple, adding the Object, and then propagate the Tuple – instead a Command object is created and added to a list in the Working Memory.
This Command object holds a reference to the LeftInputAdapterNode
and the propagated object.
This stops any left-input propagations at insertion time, so that we know that a right-input propagation will never need to attempt a join with the left-inputs (removing the need for left-input memory). All nodes have their memory turned off, including the left-input Tuple memory but excluding the right-input object memory, which means that the only node remembering an insertion propagation is the right-input object memory.
Once all the assertions are finished and all right-input memories populated, we can then iterate the list of LeftInputAdatperNode
Command objects calling each in turn.
They will propagate down the network attempting to join with the right-input objects, but they won’t be remembered in the left input as we know there will be no further object assertions and thus propagations into the right-input memory.
There is no longer an Agenda, with a priority queue to schedule the Tuples; instead, there is simply an elements for the number of rules.
The sequence number of the RuleTerminalNode
indicates the element within the elements where to place the Match.
Once all Command objects have finished we can iterate our elements, checking each element in turn, and firing the Matches if they exist.
To improve performance, we remember the first and the last populated cell in the elements.
The network is constructed, with each RuleTerminalNode
being given a sequence number based on a salience number and its order of being added to the network.
Typically the right-input node memories are Hash Maps, for fast object deletion; here, as we know there will be no object deletions, we can use a list when the values of the object are not indexed. For larger numbers of objects indexed Hash Maps provide a performance increase; if we know an object type has only a few instances, indexing is probably not advantageous, and a list can be used.
Sequential mode can only be used with a Stateless Session and is off by default.
To turn it on, either call RuleBaseConfiguration.setSequential(true)
, or set the rulebase configuration property drools.sequential
to true.
Sequential mode can fall back to a dynamic agenda by calling setSequentialAgenda
with SequentialAgenda.DYNAMIC
.
You may also set the "drools.sequential.agenda" property to "sequential" or "dynamic".
5.5. Rule Execution Modes
Drools provides two modes for rule execution - passive and active.
As a general guideline, Passive Mode is most suitable for Drools engine applications which need to explicitly control when the Drools engine shall evaluate and fire the rules, or for CEP applications making use of the Pseudo Clock. Active Mode is most effective for Drools engine applications which delegate control of when rules are evaluated and fired to the Drools engine, or for typical CEP application making use of the Real Time Clock.
5.5.1. Passive Mode
With Passive mode not only is the user responsible for working memory operations, such as insert()
, but also for when the rules are to evaluate the data and fire the resulting rule instantiations - using fireAllRules()
.
An example outline of Drools code for a CEP application making use of Passive Mode:
KieSessionConfiguration config = KieServices.Factory.get().newKieSessionConfiguration();
config.setOption( ClockTypeOption.get("pseudo") );
KieSession session = kbase.newKieSession( conf, null );
SessionPseudoClock clock = session.getSessionClock();
session.insert( tick1 );
session.fireAllRules();
clock.advanceTime(1, TimeUnit.SECONDS);
session.insert( tick2 );
session.fireAllRules();
clock.advanceTime(1, TimeUnit.SECONDS);
session.insert( tick3 );
session.fireAllRules();
session.dispose();
5.5.2. Active Mode
Drools offers a fireUntilHalt()
method, that starts the Drools engine in Active Mode, which is asynchronous in behavior, where rules will be continually evaluated and fired, until a halt()
call is made.
This is specially useful for CEP scenarios that require what is commonly known as "active queries".
Please note calling fireUntilHalt()
blocks the current thread, while the Drools engine will start and continue running asynchronously until the halt()
is called on the KieSession. It is suggested therefore to call fireUntilHalt()
from a dedicated thread, so the current thread does not get blocked indefinitely; this also enable the current thread to call halt()
at a later stage, ref. examples below.
An example outline of Drools code for a CEP application making use of Active Mode:
KieSessionConfiguration config = KieServices.Factory.get().newKieSessionConfiguration();
config.setOption( ClockTypeOption.get("realtime") );
KieSession session = kbase.newKieSession( conf, null );
new Thread( new Runnable() {
@Override
public void run() {
session.fireUntilHalt();
}
} ).start();
session.insert( tick1 );
... Thread.sleep( 1000L ); ...
session.insert( tick2 );
... Thread.sleep( 1000L ); ...
session.insert( tick3 );
session.halt();
session.dispose();
Generally, it is not recommended mixing fireAllRules() and fireUntilHalt() , especially from different threads. However the Drools engine is able to handle such situations safely, thanks to the internal state machine. If fireAllRules() is running and a call fireUntilHalt() is made, the Drools engine will wait until the fireAllRules() is finished and then start fireUntilHalt() . However if fireUntilHalt() is running and fireAllRules() is called, the later is ignored and will just return directly. For more details about thread-safety and the internal state machine, reference section "Improved multi-threading behaviour".
|
5.5.2.1. Performing KieSession operations atomically when in Active Mode
When in Active Mode, the Drools engine is in control of when the rule shall be evaluated and fired; therefore it is important that operations on the KieSession are performed in a thread-safe manner. Additionally, from a client-side perspective, there might be the need for more than one operations to be called on the KieSession in between rule evaluations, but for engine to consider these as an atomic operation: for example, inserting more than one Fact at a given time, but for the Drools engine to await until all the inserts are done, before evaluating the rules again.
Drools offers a submit()
method to group and perform operations on the KieSession as a thread-safe atomic action, while in Active Mode.
An example outline of Drools code to perform KieSession operations atomically when in Active Mode:
KieSession session = ...;
new Thread( new Runnable() {
@Override
public void run() {
session.fireUntilHalt();
}
} ).start();
final FactHandle fh = session.insert( fact_a );
... Thread.sleep( 1000L ); ...
session.submit( new KieSession.AtomicAction() {
@Override
public void execute( KieSession kieSession ) {
fact_a.setField("value");
kieSession.update( fh, fact_a );
kieSession.insert( fact_1 );
kieSession.insert( fact_2 );
kieSession.insert( fact_3 );
}
} );
... Thread.sleep( 1000L ); ...
session.insert( fact_z );
session.halt();
session.dispose();
As a reminder example, the fact handle could also be retrieved from the KieSession:
...
session.insert( fact_a );
... Thread.sleep( 1000L ); ...
session.submit( new KieSession.AtomicAction() {
@Override
public void execute( KieSession kieSession ) {
final FactHandle fh = kieSession.getFactHandle( fact_a );
fact_a.setField("value");
kieSession.update( fh, fact_a );
kieSession.insert( fact_1 );
kieSession.insert( fact_2 );
kieSession.insert( fact_3 );
}
} );
...
5.6. Propagation modes
The introduction of PHREAK as default algorithm for the Drools engine made the rules' evaluation lazy. This new Drools lazy behavior allowed a relevant performance boost but, in some very specific cases, breaks the semantic of a few Drools features.
More precisely in some circumstances it is necessary to propagate the insertion of new fact into th session immediately. For instance Drools allows a query to be executed in pull only (or passive) mode by prepending a '?' symbol to its invocation as in the following example:
query Q (Integer i)
String( this == i.toString() )
end
rule R when
$i : Integer()
?Q( $i; )
then
System.out.println( $i );
end
In this case, since the query is passive, it shouldn’t react to the insertion of a String matching the join condition in the query itself. In other words this sequence of commands
KieSession ksession = ...
ksession.insert(1);
ksession.insert("1");
ksession.fireAllRules();
shouldn’t cause the rule R to fire because the String satisfying the query condition has been inserted after the Integer and the passive query shouldn’t react to this insertion. Conversely the rule should fire if the insertion sequence is inverted because the insertion of the Integer, when the passive query can be satisfied by the presence of an already existing String, will trigger it.
Unfortunately the lazy nature of PHREAK doesn’t allow the Drools engine to make any distinction regarding the insertion sequence of the two facts, so the rule will fire in both cases. In circumstances like this it is necessary to evaluate the rule eagerly as done by the old RETEOO-based engine.
In other cases it is required that the propagation is eager, meaning that it is not immedate, but anyway has to happen before the Drools engine/agenda starts scheduled evaluations. For instance this is necessary when a rule has the no-loop or the lock-on-active attribute and in fact when this happens this propagation mode is automatically enforced by the Drools engine.
To cover these use cases, and in all other situations where an immediate or eager rule evaluation is required, it is possible to declaratively specify so by annotating the rule itself with @Propagation(Propagation.Type), where Propagation.Type is an enumeration with 3 possible values:
-
IMMEDIATE means that the propagation is performed immediately.
-
EAGER means that the propagation is performed lazily but eagerly evaluated before scheduled evaluations.
-
LAZY means that the propagation is totally lazy and this is default PHREAK behaviour
This means that the following drl:
query Q (Integer i)
String( this == i.toString() )
end
rule R @Propagation(IMMEDIATE) when
$i : Integer()
?Q( $i; )
then
System.out.println( $i );
end
will make the rule R to fire if and only if the Integer is inserted after the String, thus behaving in accordance with the semantic of the passive query.
5.7. Commands and the CommandExecutor
The CommandFactory
allows for commands to be executed on those sessions, the only difference being that the Stateless KIE session executes fireAllRules()
at the end before disposing the session.
The currently supported commands are:
-
FireAllRules
-
GetGlobal
-
SetGlobal
-
InsertObject
-
InsertElements
-
Query
-
StartProcess
-
BatchExecution
`
InsertObject` will insert a single object, with an optional "out" identifier. InsertElements
will iterate an Iterable, inserting each of the elements.
What this means is that a Stateless KIE session is no longer limited to just inserting objects, it can now start processes or execute queries, and do this in any order.
StatelessKieSession ksession = kbase.newStatelessKieSession();
ExecutionResults bresults =
ksession.execute( CommandFactory.newInsert( new Cheese( "stilton" ), "stilton_id" ) );
Stilton stilton = bresults.getValue( "stilton_id" );
The execute method always returns an ExecutionResults
instance, which allows access to any command results if they specify an out identifier such as the "stilton_id" above.
StatelessKieSession ksession = kbase.newStatelessKieSession();
Command cmd = CommandFactory.newInsertElements( Arrays.asList( Object[] {
new Cheese( "stilton" ),
new Cheese( "brie" ),
new Cheese( "cheddar" ),
});
ExecutionResults bresults = ksession.execute( cmd );
The execute method only allows for a single command.
That’s where BatchExecution
comes in, which represents a composite command, created from a list of commands.
Now, execute will iterate over the list and execute each command in turn.
This means you can insert some objects, start a process, call fireAllRules and execute a query, all in a single execute(…)
call, which is quite powerful.
As mentioned previosly, the StatelessKieSession will execute fireAllRules()
automatically at the end.
However the keen-eyed reader probably has already noticed the FireAllRules
command and wondered how that works with a StatelessKieSession.
The FireAllRules
command is allowed, and using it will disable the automatic execution at the end; think of using it as a sort of manual override function.
A custom XStream marshaller can be used with the Drools Pipeline to achieve XML scripting, which is perfect for services.
Here are two simple XML samples, one for the BatchExecution and one for the ExecutionResults
.
<batch-execution>
<insert out-identifier='outStilton'>
<org.drools.compiler.Cheese>
<type>stilton</type>
<price>25</price>
<oldPrice>0</oldPrice>
</org.drools.compiler.Cheese>
</insert>
</batch-execution>
<execution-results>
<result identifier='outStilton'>
<org.drools.compiler.Cheese>
<type>stilton</type>
<oldPrice>25</oldPrice>
<price>30</price>
</org.drools.compiler.Cheese>
</result>
</execution-results>
Spring and Camel, covered in the integrations book, facilitate declarative services.
<batch-execution>
<insert out-identifier="stilton">
<org.drools.compiler.Cheese>
<type>stilton</type>
<price>1</price>
<oldPrice>0</oldPrice>
</org.drools.compiler.Cheese>
</insert>
<query out-identifier='cheeses2' name='cheesesWithParams'>
<string>stilton</string>
<string>cheddar</string>
</query>
</batch-execution>
The CommandExecutor
returns an ExecutionResults
, and this is handled by the pipeline code snippet as well.
A similar output for the <batch-execution> XML sample above would be:
<execution-results>
<result identifier="stilton">
<org.drools.compiler.Cheese>
<type>stilton</type>
<price>2</price>
</org.drools.compiler.Cheese>
</result>
<result identifier='cheeses2'>
<query-results>
<identifiers>
<identifier>cheese</identifier>
</identifiers>
<row>
<org.drools.compiler.Cheese>
<type>cheddar</type>
<price>2</price>
<oldPrice>0</oldPrice>
</org.drools.compiler.Cheese>
</row>
<row>
<org.drools.compiler.Cheese>
<type>cheddar</type>
<price>1</price>
<oldPrice>0</oldPrice>
</org.drools.compiler.Cheese>
</row>
</query-results>
</result>
</execution-results>
The BatchExecutionHelper
provides a configured XStream instance to support the marshalling of Batch Executions, where the resulting XML can be used as a message format, as shown above.
Configured converters only exist for the commands supported via the Command Factory.
The user may add other converters for their user objects.
This is very useful for scripting stateless or stateful KIE sessions, especially when services are involved.
There is currently no XML schema to support schema validation.
The basic format is outlined here, and the drools-pipeline module has an illustrative unit test in the XStreamBatchExecutionTest
unit test.
The root element is <batch-execution> and it can contain zero or more commands elements.
<batch-execution>
...
</batch-execution>
This contains a list of elements that represent commands, the supported commands is limited to those Commands provided by the Command Factory. The most basic of these is the <insert> element, which inserts objects. The contents of the insert element is the user object, as dictated by XStream.
<batch-execution>
<insert>
...<!-- any user object -->
</insert>
</batch-execution>
The insert element features an "out-identifier" attribute, demanding that the inserted object will also be returned as part of the result payload.
<batch-execution>
<insert out-identifier='userVar'>
...
</insert>
</batch-execution>
It’s also possible to insert a collection of objects using the <insert-elements> element.
This command does not support an out-identifier.
The org.domain.UserClass
is just an illustrative user object that XStream would serialize.
<batch-execution>
<insert-elements>
<org.domain.UserClass>
...
</org.domain.UserClass>
<org.domain.UserClass>
...
</org.domain.UserClass>
<org.domain.UserClass>
...
</org.domain.UserClass>
</insert-elements>
</batch-execution>
While the out
attribute is useful in returning specific instances as a result payload, we often wish to run actual queries.
Both parameter and parameterless queries are supported.
The name
attribute is the name of the query to be called, and the out-identifier
is the identifier to be used for the query results in the <execution-results>
payload.
<batch-execution>
<query out-identifier='cheeses' name='cheeses'/>
<query out-identifier='cheeses2' name='cheesesWithParams'>
<string>stilton</string>
<string>cheddar</string>
</query>
</batch-execution>
5.8. KieSessions pool
In high volume use cases KieSession`s get created and disposed with a very high frequency. In general this operation is not
extremely time consuming, but when repeated millions of times can become a bottleneck and also requires a huge GC effort.
It is possible to greatly alleviate this problem by using a pool of `KieSession`s. To obtain such a pool from a `KieContainer
is enough to invoke on it the method
KieContainerSessionsPool KieContainer.newKieSessionsPool(int initialSize)
where initialSize
is the number of the KieSession`s that will be initially created in the pool. However, if required by the running
application, the number of `KieSession`s in the pool will dynamically grow beyond that value. At this point you can create
`KieSession`s from that pool as you would normally do from a `KieContainer
:
KieContainerSessionsPool pool = kContainer.newKieSessionsPool(10);
KieSession kSession = pool.newKieSession();
Now you can use the KieSession as per normal, and when you call dispose()
on it, instead of being destroyed, it just
gets resetted and pushed back into the pool. Note that using this pool will also affect the case when you have one or more
StatelessKieSession`s and you keep reusing them with multiple call to the `execute()
method. In fact a StatelessKieSession
created directly from a KieContainer
will keep to internally create a new KieSession
for each execute()
invocation.
Conversely if you create the StatelessKieSession
from the pool it will internally uses the KieSession`s provided by the
pool itself. In other words even if you asked a `StatelessKieSession
to the pool, what is actually pooled are the `KieSession`s
that are wrapped by it.
Once you’re done with the pool it is required that you call the shutdown()
method on it to avoid memory leaks. Alternatively
calling dispose()
on the whole KieContainer
will also automatically shutdown all the pools eventually created from it.
5.9. Rule units
You can use rule units to partition a rule set into smaller units, bind different data sources to those units, and then execute the individual unit. A rule unit consists of data sources, global variables, and rules.
You can define a rule unit by implementing the RuleUnit
interface as shown in the following example:
package org.mypackage.myunit;
public static class AdultUnit implements RuleUnit {
private int adultAge;
private DataSource<Person> persons;
public AdultUnit( ) { }
public AdultUnit( DataSource<Person> persons, int age ) {
this.persons = persons;
this.age = age;
}
// A DataSource of Persons in this rule unit
public DataSource<Person> getPersons() {
return persons;
}
// A global variable valid in this rule unit
public int getAdultAge() {
return adultAge;
}
// --- life cycle methods
@Override
public void onStart() {
System.out.println("AdultUnit started.");
}
@Override
public void onEnd() {
System.out.println("AdultUnit ended.");
}
}
In this example, persons
is a source of facts of type Person
. It represents that part of the working memory, which is related to that specific
entry-point used when the rule unit is evaluated. The adultAge
global variable is accessible from all the rules belonging to this rule unit. The last two methods are part of the rule unit life cycle and are invoked by the Drools engine.
The lifecycle of a rule unit can be monitored overriding the following methods:
Method | Invoked when |
---|---|
onStart() |
the Drools engine starts evaluating the unit |
onEnd() |
the evaluation of this unit terminates |
onSuspend() |
the execution of unit is suspended (only for runUntilHalt) |
onResume() |
the execution of unit is resumed (only for runUntilHalt) |
onYield(RuleUnit other) |
the consequence of rule in a given rule unit triggers the execution of a different unit |
All of these methods have an empty default implementation inside the RuleUnit
interface, so their implementation is optional. At this point, it is possible to add one or more rules to this rule unit. By default all the rules in a DRL file are automatically associated to a rule unit following a naming convention of the name of the DRL file itself. If the DRL file is in the same package and has the same name as a class implementing the RuleUnit
interface, then all of the rules in that DRL file will implicitly belong to that unit.
For example, all the rules in the DRL file named AdultUnit.drl
in the package org.mypackage.myunit
are automatically part of the rule unit org.mypackage.myunit.AdultUnit
.
You can avoid using this naming convention and explicitly declare the unit to which the rules in a DRL file belong by using the new unit
keyword. The unit declaration must always immediately follow the package declaration and contain the name of the class in that package of which the rules in the DRL file are part.
package org.mypackage.myunit
unit AdultUnit
rule Adult when
$p : Person(age >= adultAge) from persons
then
System.out.println($p.getName() + " is adult and greater than " + adultAge);
end
You cannot mix rules with and without a rule unit in the same KIE base. |
Additionally, you can rewrite the same pattern in a more convenient way using the oopath notation introduced in drools 6, as shown in the following example:
package org.mypackage.myunit
unit AdultUnit
rule Adult when
$p : /persons[age >= adultAge]
then
System.out.println($p.getName() + " is adult and greater than " + adultAge);
end
In this example, the persons matched by the left-hand side of the rule is retrieved from the DataSource
contained in the rule unit class with
the same name. The adultAge
variable is used in both the left-hand side and right-hand side of the rule in the same way that a global variable is defined at the DRL level. The persons
DataSource acts as a specific entry point, feeding the working memory.
The easiest way to create a DataSource
is using a fixed set of data, as shown in the following example:
DataSource<Person> persons = DataSource.create( new Person( "Mario", 42 ),
new Person( "Marilena", 44 ),
new Person( "Sofia", 4 ) );
To execute one or more rule units defined in a given KIE base, create a new RuleUnitExecutor
and bind it to the KIE base:
KieBase kbase = kieContainer.getKieBase();
RuleUnitExecutor executor = RuleUnitExecutor.create().bind( kbase );
At this point, create the AdultUnit
by passing the persons DataSource
to it and running it on the RuleUnitExecutor
:
RuleUnit adultUnit = new AdultUnit(persons, 18);
executor.run( adultUnit );
This produces the following output:
org.mypackage.myunit.AdultUnit started.
Marilena is adult and greater than 18
Mario is adult and greater than 18
org.mypackage.myunit.AdultUnit ended.
Instead of explicitly creating the rule unit instance, you can pass to the executor the rule unit class that you want to run and let the executor create an instance of it. You can then set the DataSource
and other variables before running it. In order to do so, ensure that you have previously registered those variables on the executor so that the following code produces exactly the same result as the former example:
executor.bindVariable( "persons", persons );
.bindVariable( "adultAge", 18 );
executor.run( AdultUnit.class );
The name passed to the RuleUnitExecutor.bindVariable()
method is used at run time to bind the variable to the field of the rule unit class with the same name. For example, in the previous example the RuleUnitExecutor
inserts into the new rule unit the data source formerly bound to the "persons"
name and the value 18
bound to the String "adultAge"
to the fields with the corresponding names inside the AdultUnit
class.
You can override this default and explicitly define a logical binding name for each field of the rule unit class using the @UnitVar
annotation. For example, the field binding in the following class can be redefined with alternative names:
package org.mypackage.myunit;
public static class AdultUnit implements RuleUnit {
@UnitVar("minAge")
private int adultAge = 18;
@UnitVar("data")
private DataSource<Person> persons;
}
You can then bind the variables to the executor using those alternative names and run the unit:
executor.bindVariable( "data", persons );
.bindVariable( "minAge", 18 );
executor.run( AdultUnit.class );
You can execute a rule unit in passive mode as shown in the previous example (equivalent to invoking fireAllRules
on an entire KIE session)
or in active mode using the runUntilHalt
(equivalent to the KIE session fireUntilHalt
).
As for the fireUntilHalt
, the runUntilHalt
is blocking and therefore has to be issued on a separated thread:
new Thread( () -> executor.runUntilHalt( adultUnit ) ).start();
5.9.1. Data sources
A DataSource
is a source of the data processed by a given rule unit. A rule unit can have zero or more data sources and
to each DataSource declared inside a rule unit corresponds a different entry-point into the rule unit executor. A DataSource
can be shared by different units, but in this case there will be many different entry-points, one for each unit, through which
the same objects will be inserted.
In other terms the DataSource
represents the entry-point of the rule unit, so it is possible to insert a new fact into it:
Person mario = new Person( "Mario", 42 );
FactHandle marioFh = persons.insert( mario );
Modify the fact, optionally specifying the set of properties that have been modified in order to leverage property reactivity:
mario.setAge( 43 );
persons.update( marioFh, mario, "age" );
or delete it
persons.delete( marioFh );
5.9.2. Imperatively running and declaratively guarding a RuleUnit
As anticipated, you can define multiple rule units in the same KIE base and these units can work in a coordinated way by invoking or guarding the execution of each other. To demonstrate this let’s suppose having the following 2 drl files each of them containing a rule belonging to a distinct rule unit.
package org.mypackage.myunit
unit AdultUnit
rule Adult when
Person(age >= 18, $name : name) from persons
then
System.out.println($name + " is adult");
end
package org.mypackage.myunit
unit NotAdultUnit
rule NotAdult when
$p : Person(age < 18, $name : name) from persons
then
System.out.println($name + " is NOT adult");
modify($p) { setAge(18); }
drools.run( AdultUnit.class );
end
Also suppose to have a RuleUnitExecutor
created from the KieBase
built out of these rules and a DataSource
of Persons
bound to it.
RuleUnitExecutor executor = RuleUnitExecutor.create().bind( kbase );
DataSource<Person> persons = executor.newDataSource( "persons",
new Person( "Mario", 42 ),
new Person( "Marilena", 44 ),
new Person( "Sofia", 4 ) );
Note that in this case we are creating the DataSource
directly out of the RuleUnitExecutor
and binding it to the
"persons" variable in a single statement.
At this point trying to execute the NotAdultUnit unit we obtain the following output:
Sofia is NOT adult
Mario is adult
Marilena is adult
Sofia is adult
In fact the NotAdult rule finds a match when evaluating the person "Sofia" who has an age lower than 18. Then it modifies
her age to 18 and with the statement drools.run( AdultUnit.class )
triggers the execution of the other unit which has a
rule that now can fire for all the 3 persons in the DataSource
. This means that the drools.run()
statement inside a
consequence is the way to imperatively interrupt the execution of a rule unit and cede the control to a different rule unit.
Conversely the drools.guard()
statement allows to declaratively schedule the execution of another rule unit when the
condition in the LHS of the rule containing that statement is met. More precisely, using this mechanism a rule in a given
rule unit acts as a guard for a different unit. This means that, when the Drools engine produces at least one match for the LHS
of the guarding rule, the guarded RuleUnit is considered active. Of course a RuleUnit can have more than one guarding rule.
Let’s see how this works with another practical example. Suppose of having a simple BoxOffice
class
public class BoxOffice {
private boolean open;
public BoxOffice( boolean open ) {
this.open = open;
}
public boolean isOpen() {
return open;
}
public void setOpen( boolean open ) {
this.open = open;
}
}
and a BoxOfficeUnit
with a data source of box offices.
public class BoxOfficeUnit implements RuleUnit {
private DataSource<BoxOffice> boxOffices;
public DataSource<BoxOffice> getBoxOffices() {
return boxOffices;
}
}
We introduce now the requirement to keep selling tickets for the event as long as there is at least one opened box office.
To achieve this let’s define a second unit with a DataSource
of person and a second one of tickets.
public class TicketIssuerUnit implements RuleUnit {
private DataSource<Person> persons;
private DataSource<AdultTicket> tickets;
private List<String> results;
public TicketIssuerUnit() { }
public TicketIssuerUnit( DataSource<Person> persons, DataSource<AdultTicket> tickets ) {
this.persons = persons;
this.tickets = tickets;
}
public DataSource<Person> getPersons() {
return persons;
}
public DataSource<AdultTicket> getTickets() {
return tickets;
}
public List<String> getResults() {
return results;
}
}
Then we can define a first rule in the BoxOfficeUnit that guards for this second unit.
package org.mypackage.myunit;
unit BoxOfficeUnit;
rule BoxOfficeIsOpen when
$box: /boxOffices[ open ]
then
drools.guard( TicketIssuerUnit.class );
end
In this way we achieved what we have anticipated: by running the BoxOfficeUnit at some point it will also evaluates the rules in the TicketIssuerUnit defined as
package org.mypackage.myunit;
unit TicketIssuerUnit;
rule IssueAdultTicket when
$p: /persons[ age >= 18 ]
then
tickets.insert(new AdultTicket($p));
end
rule RegisterAdultTicket when
$t: /tickets
then
results.add( $t.getPerson().getName() );
end
that is guarded by the BoxOfficeIsOpen rule, until there will exist at least a set of facts satisfying the LHS patterns of that rule. In other terms the existence of at least one open box office will keep the guarding rule and in turn its guarded unit active as it is evident in the following use case.
DataSource<Person> persons = executor.newDataSource( "persons" );
DataSource<BoxOffice> boxOffices = executor.newDataSource( "boxOffices" );
DataSource<AdultTicket> tickets = executor.newDataSource( "tickets" );
List<String> list = new ArrayList<>();
executor.bindVariable( "results", list );
// two open box offices
BoxOffice office1 = new BoxOffice(true);
FactHandle officeFH1 = boxOffices.insert( office1 );
BoxOffice office2 = new BoxOffice(true);
FactHandle officeFH2 = boxOffices.insert( office2 );
persons.insert(new Person("Mario", 40));
// fire BoxOfficeIsOpen -> run TicketIssuerUnit -> fire RegisterAdultTicket
executor.run(BoxOfficeUnit.class);
assertEquals( 1, list.size() );
assertEquals( "Mario", list.get(0) );
list.clear();
persons.insert(new Person("Matteo", 30));
executor.run(BoxOfficeUnit.class); // fire RegisterAdultTicket
assertEquals( 1, list.size() );
assertEquals( "Matteo", list.get(0) );
list.clear();
// close one box office, the other is still open
office1.setOpen(false);
boxOffices.update(officeFH1, office1);
persons.insert(new Person("Mark", 35));
executor.run(BoxOfficeUnit.class);
assertEquals( 1, list.size() );
assertEquals( "Mark", list.get(0) );
list.clear();
// all box offices, are now closed
office2.setOpen(false);
boxOffices.update(officeFH2, office2); // guarding rule no longer true
persons.insert(new Person("Edson", 35));
executor.run(BoxOfficeUnit.class); // no fire
assertEquals( 0, list.size() );
5.9.3. RuleUnit identity
Since a rule can guard multiple rule units and at the same time a unit can be guarded and then activated by multiple rules,
it is necessary to clearly define what is the identity of a given unit. By the default the identity of a unit is simply the
rule unit class. This is encoded in the getUnitIdentity()
default method of the RuleUnit
interface
default Identity getUnitIdentity() {
return new Identity( getClass() );
}
and implies that each unit is threated as a singleton by the RuleUnitExecutor
. To demonstrate this let’s suppose of
having a simple RuleUnit
class with only a DataSource
accepting any kind of object
public class Unit0 implements RuleUnit {
private DataSource<Object> input;
public DataSource<Object> getInput() {
return input;
}
}
together with a rule belonging to this unit that guards another unit using 2 different conditions.
package org.mypackage.myunit
unit Unit0
rule GuardAgeCheck when
$i: /input#Integer
$s: /input#String
then
drools.guard( new AgeCheckUnit($i) );
drools.guard( new AgeCheckUnit($s.length()) );
end
This second RuleUnit
is intended to check the age of a set of persons. Then it has a DataSource
of the persons to check,
a minAge variable against which doing this check and a list were accumulating the results
public class AgeCheckUnit implements RuleUnit {
private final int minAge;
private DataSource<Person> persons;
private List<String> results;
public AgeCheckUnit( int minAge ) {
this.minAge = minAge;
}
public DataSource<Person> getPersons() {
return persons;
}
public int getMinAge() {
return minAge;
}
public List<String> getResults() {
return results;
}
}
while the corresponding rule actually performing the check of the persons in the DataSource
is the following:
package org.mypackage.myunit
unit AgeCheckUnit
rule CheckAge when
$p : /persons{ age > minAge }
then
results.add($p.getName() + ">" + minAge);
end
At this point we can create a RuleUnitExecutor
, bind it to the KIE base containing these 2 units and also create
the 2 DataSource
s to feed the same units.
RuleUnitExecutor executor = RuleUnitExecutor.create().bind( kbase );
DataSource<Object> input = executor.newDataSource( "input" );
DataSource<Person> persons = executor.newDataSource( "persons",
new Person( "Mario", 42 ),
new Person( "Sofia", 4 ) );
List<String> results = new ArrayList<>();
executor.bindVariable( "results", results );
We are now ready to insert some objects into the input data source and execute the Unit0.
ds.insert("test");
ds.insert(3);
ds.insert(4);
executor.run(Unit0.class);
As outcome of this execution the results list will contain the following:
[Sofia>3, Mario>3]
As anticipated the rule unit named AgeCheckUnit is seen as a singleton and then executed only once, this time with minAge
equals to 3 (but this is not deterministic). Both the String "test" and the Integer 4 inserted into the input data source
could also trigger a second execution with minAge
set to 4, but this is not happening because another unit with the same
identity has been already evaluated. To fix this problem it is enough to override the getUnitIdentity()
method in the
AgeCheckUnit
class to also include the variable minAge in its identity.
public class AgeCheckUnit implements RuleUnit {
...
@Override
public Identity getUnitIdentity() {
return new Identity(getClass(), minAge);
}
}
Having done so, the units with minAge 3 and 4 are considered two different units and then both evaluated, so trying to rerun the former example the result list will now contain
[Mario>4, Sofia>3, Mario>3]
6. Rule Language Reference
6.1. Overview
Drools has a "native" rule language. This format is very light in terms of punctuation, and supports natural and domain specific languages via "expanders" that allow the language to morph to your problem domain. This chapter is mostly concerted with this native rule format. The diagrams used to present the syntax are known as "railroad" diagrams, and they are basically flow charts for the language terms. The technically very keen may also refer to DRL.g which is the Antlr3 grammar for the rule language. If you use Business Central, a lot of the rule structure is done for you with content assistance, for example, type "ru" and press ctrl+space, and it will build the rule structure for you.
6.1.1. A rule file
A rule file is typically a file with a .drl extension. In a DRL file you can have multiple rules, queries and functions, as well as some resource declarations like imports, globals and attributes that are assigned and used by your rules and queries. However, you are also able to spread your rules across multiple rule files (in that case, the extension .rule is suggested, but not required) - spreading rules across files can help with managing large numbers of rules. A DRL file is simply a text file.
The overall structure of a rule file is:
package package-name
imports
globals
functions
queries
rules
The order in which the elements are declared is not important, except for the package name that, if declared, must be the first element in the rules file. All elements are optional, so you will use only those you need. We will discuss each of them in the following sections.
6.1.2. What makes a rule
For the impatient, just as an early view, a rule has the following rough structure:
rule "name"
attributes
when
LHS
then
RHS
end
It’s really that simple. Mostly punctuation is not needed, even the double quotes for "name" are optional, as are newlines. Attributes are simple (always optional) hints to how the rule should behave. LHS is the conditional parts of the rule, which follows a certain syntax which is covered below. RHS is basically a block that allows dialect specific semantic code to be executed.
It is important to note that white space is not important, except in the case of domain specific languages, where lines are processed one by one and spaces may be significant to the domain language.
6.2. Keywords
Drools 5 introduces the concept of hard and soft keywords.
Hard keywords are reserved, you cannot use any hard keyword when naming your domain objects, properties, methods, functions and other elements that are used in the rule text.
Here is the list of hard keywords that must be avoided as identifiers when writing rules:
-
true
-
false
-
null
Soft keywords are just recognized in their context, enabling you to use these words in any other place if you wish, although, it is still recommended to avoid them, to avoid confusions, if possible. Here is a list of the soft keywords:
-
lock-on-active
-
date-effective
-
date-expires
-
no-loop
-
auto-focus
-
activation-group
-
agenda-group
-
ruleflow-group
-
entry-point
-
duration
-
package
-
import
-
dialect
-
salience
-
enabled
-
attributes
-
rule
-
extend
-
when
-
then
-
template
-
query
-
declare
-
function
-
global
-
eval
-
not
-
in
-
or
-
and
-
exists
-
forall
-
accumulate
-
collect
-
from
-
action
-
reverse
-
result
-
end
-
over
-
init
Of course, you can have these (hard and soft) words as part of a method name in camel case, like notSomething() or accumulateSomething() - there are no issues with that scenario.
Although the 3 hard keywords above are unlikely to be used in your existing domain models, if you absolutely need to use them as identifiers instead of keywords, the DRL language provides the ability to escape hard keywords on rule text. To escape a word, simply enclose it in grave accents, like this:
Holiday( `true` == "yes" ) // please note that Drools will resolve that reference to the method Holiday.isTrue()
6.3. Comments
Comments are sections of text that are ignored by the Drools engine. They are stripped out when they are encountered, except inside semantic code blocks, like the RHS of a rule.
6.3.1. Single line comment
To create single line comments, you can use '//'. The parser will ignore anything in the line after the comment symbol. Example:
rule "Testing Comments"
when
// this is a single line comment
eval( true ) // this is a comment in the same line of a pattern
then
// this is a comment inside a semantic code block
end
'#' for comments has been removed. |
6.3.2. Multi-line comment

Multi-line comments are used to comment blocks of text, both in and outside semantic code blocks. Example:
rule "Test Multi-line Comments"
when
/* this is a multi-line comment
in the left hand side of a rule */
eval( true )
then
/* and this is a multi-line comment
in the right hand side of a rule */
end
6.4. Error Messages
Drools 5 introduces standardized error messages. This standardization aims to help users to find and resolve problems in a easier and faster way. In this section you will learn how to identify and interpret those error messages, and you will also receive some tips on how to solve the problems associated with them.
6.4.1. Message format
The standardization includes the error message format and to better explain this format, let’s use the following example:

1st Block: This area identifies the error code.
2nd Block: Line and column information.
3rd Block: Some text describing the problem.
4th Block: This is the first context. Usually indicates the rule, function, template or query where the error occurred. This block is not mandatory.
5th Block: Identifies the pattern where the error occurred. This block is not mandatory.
6.4.2. Error Messages Description
6.4.2.1. 101: No viable alternative
Indicates the most common errors, where the parser came to a decision point but couldn’t identify an alternative. Here are some examples:
1: rule one
2: when
3: exists Foo()
4: exits Bar() // "exits"
5: then
6: end
The above example generates this message:
-
[ERR 101] Line 4:4 no viable alternative at input 'exits' in rule one
At first glance this seems to be valid syntax, but it is not (exits != exists). Let’s take a look at next example:
1: package org.drools.examples;
2: rule
3: when
4: Object()
5: then
6: System.out.println("A RHS");
7: end
Now the above code generates this message:
-
[ERR 101] Line 3:2 no viable alternative at input 'WHEN'
This message means that the parser encountered the token WHEN, actually a hard keyword, but it’s in the wrong place since the rule name is missing.
The error "no viable alternative" also occurs when you make a simple lexical mistake. Here is a sample of a lexical problem:
1: rule simple_rule
2: when
3: Student( name == "Andy )
4: then
5: end
The above code misses to close the quotes and because of this the parser generates this error message:
-
[ERR 101] Line 0:-1 no viable alternative at input '<eof>' in rule simple_rule in pattern Student
Usually the Line and Column information are accurate, but in some cases (like unclosed quotes), the parser generates a 0:-1 position. In this case you should check whether you didn’t forget to close quotes, apostrophes or parentheses. |
6.4.2.2. 102: Mismatched input
This error indicates that the parser was looking for a particular symbol that it didn’t find at the current input position. Here are some samples:
1: rule simple_rule
2: when
3: foo3 : Bar(
The above example generates this message:
-
[ERR 102] Line 0:-1 mismatched input '<eof>' expecting ')' in rule simple_rule in pattern Bar
To fix this problem, it is necessary to complete the rule statement.
Usually when you get a 0:-1 position, it means that parser reached the end of source. |
The following code generates more than one error message:
1: package org.drools.examples;
2:
3: rule "Avoid NPE on wrong syntax"
4: when
5: not( Cheese( ( type == "stilton", price == 10 ) || ( type == "brie", price == 15 ) ) from $cheeseList )
6: then
7: System.out.println("OK");
8: end
These are the errors associated with this source:
-
[ERR 102] Line 5:36 mismatched input ',' expecting ')' in rule "Avoid NPE on wrong syntax" in pattern Cheese
-
[ERR 101] Line 5:57 no viable alternative at input 'type' in rule "Avoid NPE on wrong syntax"
-
[ERR 102] Line 5:106 mismatched input ')' expecting 'then' in rule "Avoid NPE on wrong syntax"
Note that the second problem is related to the first. To fix it, just replace the commas (',') by AND operator ('&&').
In some situations you can get more than one error message. Try to fix one by one, starting at the first one. Some error messages are generated merely as consequences of other errors. |
6.4.2.3. 103: Failed predicate
A validating semantic predicate evaluated to false. Usually these semantic predicates are used to identify soft keywords. This sample shows exactly this situation:
1: package nesting;
2: dialect "mvel"
3:
4: import org.drools.compiler.Person
5: import org.drools.compiler.Address
6:
7: fdsfdsfds
8:
9: rule "test something"
10: when
11: p: Person( name=="Michael" )
12: then
13: p.name = "other";
14: System.out.println(p.name);
15: end
With this sample, we get this error message:
-
[ERR 103] Line 7:0 rule 'rule_key' failed predicate: {(validateIdentifierKey(DroolsSoftKeywords.RULE))}? in rule
The fdsfdsfds text is invalid and the parser couldn’t identify it as the soft keyword rule
.
This error is very similar to 102: Mismatched input, but usually involves soft keywords. |
6.4.2.4. 104: Trailing semi-colon not allowed
This error is associated with the eval
clause, where its expression may not be terminated with a semicolon.
Check this example:
1: rule simple_rule
2: when
3: eval(abc();)
4: then
5: end
Due to the trailing semicolon within eval, we get this error message:
-
[ERR 104] Line 3:4 trailing semi-colon not allowed in rule simple_rule
This problem is simple to fix: just remove the semi-colon.
6.4.2.5. 105: Early Exit
The recognizer came to a subrule in the grammar that must match an alternative at least once, but the subrule did not match anything. Simply put: the parser has entered a branch from where there is no way out. This example illustrates it:
1: template test_error
2: aa s 11;
3: end
This is the message associated to the above sample:
-
[ERR 105] Line 2:2 required (…)+ loop did not match anything at input 'aa' in template test_error
To fix this problem it is necessary to remove the numeric value as it is neither a valid data type which might begin a new template slot nor a possible start for any other rule file construct.
6.4.3. Other Messages
Any other message means that something bad has happened, so please contact the development team.
6.5. Package
A package is a collection of rules and other related constructs, such as imports and globals. The package members are typically related to each other - perhaps HR rules, for instance. A package represents a namespace, which ideally is kept unique for a given grouping of rules. The package name itself is the namespace, and is not related to files or folders in any way.
It is possible to assemble rules from multiple rule sources, and have one top level package configuration that all the rules are kept under (when the rules are assembled). Although, it is not possible to merge into the same package resources declared under different names. A single Rulebase may, however, contain multiple packages built on it. A common structure is to have all the rules for a package in the same file as the package declaration (so that is it entirely self-contained).
The following railroad diagram shows all the components that may make up a package.
Note that a package must have a namespace and be declared using standard Java conventions for package names; i.e., no spaces, unlike rule names which allow spaces.
In terms of the order of elements, they can appear in any order in the rule file, with the exception of the package
statement, which must be at the top of the file.
In all cases, the semicolons are optional.
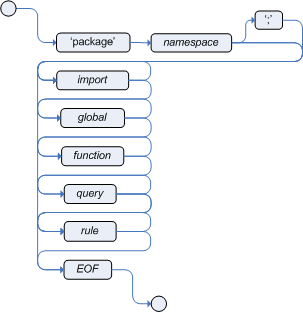
Notice that any rule attribute (as described the section Rule Attributes) may also be written at package level, superseding the attribute’s default value. The modified default may still be replaced by an attribute setting within a rule.
6.5.1. import

Import statements work like import statements in Java.
You need to specify the fully qualified paths and type names for any objects you want to use in the rules.
Drools automatically imports classes from the Java package of the same name, and also from the package java.lang
.
6.5.2. global

With global
you define global variables.
They are used to make application objects available to the rules.
Typically, they are used to provide data or services that the rules use, especially application services used in rule consequences, and to return data from the rules, like logs or values added in rule consequences, or for the rules to interact with the application, doing callbacks.
Globals are not inserted into the Working Memory, and therefore a global should never be used to establish conditions in rules except when it has a constant immutable value.
The Drools engine cannot be notified about value changes of globals and does not track their changes.
Incorrect use of globals in constraints may yield surprising results - surprising in a bad way.
If multiple packages declare globals with the same identifier they must be of the same type and all of them will reference the same global value.
In order to use globals you must:
-
Declare your global variable in your rules file and use it in rules. For example:
global java.util.List myGlobalList; rule "Using a global" when eval( true ) then myGlobalList.add( "Hello World" ); end
-
Set the global value on your working memory. It is a best practice to set all global values before asserting any fact to the working memory. Example:
List list = new ArrayList(); KieSession kieSession = kiebase.newKieSession(); kieSession.setGlobal( "myGlobalList", list );
Note that these are just named instances of objects that you pass in from your application to the working memory.
This means you can pass in any object you want: you could pass in a service locator, or perhaps a service itself.
With the new from
element it is now common to pass a Hibernate session as a global, to allow from
to pull data from a named Hibernate query.
One example may be an instance of a Email service. In your integration code that is calling the Drools engine, you obtain your emailService object, and then set it in the working memory. In the DRL, you declare that you have a global of type EmailService, and give it the name "email". Then in your rule consequences, you can use things like email.sendSMS(number, message).
Globals are not designed to share data between rules and they should never be used for that purpose. Rules always reason and react to the working memory state, so if you want to pass data from rule to rule, assert the data as facts into the working memory.
Care must be taken when changing data held by globals because the Drools engine is not aware of those changes, hence cannot react to them.
6.6. Function
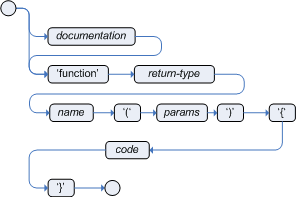
Functions are a way to put semantic code in your rule source file, as opposed to in normal Java classes.
They can’t do anything more than what you can do with helper classes.
(In fact, the compiler generates the helper class for you behind the scenes.) The main advantage of using functions in a rule is that you can keep the logic all in one place, and you can change the functions as needed (which can be a good or a bad thing). Functions are most useful for invoking actions on the consequence (then
) part of a rule, especially if that particular action is used over and over again, perhaps with only differing parameters for each rule.
A typical function declaration looks like:
function String hello(String name) {
return "Hello "+name+"!";
}
Note that the function
keyword is used, even though its not really part of Java.
Parameters to the function are defined as for a method, and you don’t have to have parameters if they are not needed.
The return type is defined just like in a regular method.
Alternatively, you could use a static method in a helper class, e.g., Foo.hello()
.
Drools supports the use of function imports, so all you would need to do is:
import function my.package.Foo.hello
Irrespective of the way the function is defined or imported, you use a function by calling it by its name, in the consequence or inside a semantic code block. Example:
rule "using a static function"
when
eval( true )
then
System.out.println( hello( "Bob" ) );
end
6.7. Type Declaration
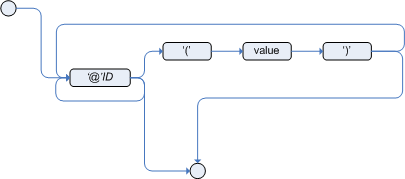
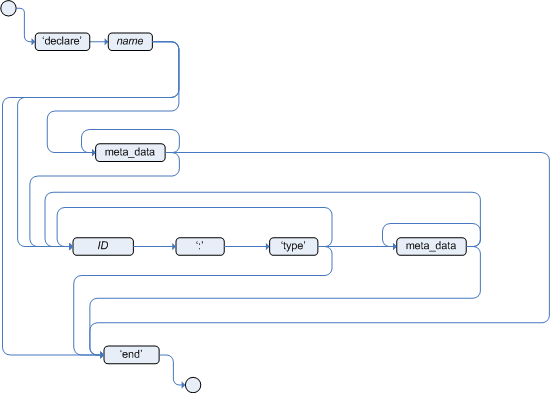
Type declarations have two main goals in the Drools engine: to allow the declaration of new types, and to allow the declaration of metadata for types.
-
Declaring new types: Drools works out of the box with plain Java objects as facts. Sometimes, however, users may want to define the model directly to the Drools engine, without worrying about creating models in a lower level language like Java. At other times, there is a domain model already built, but eventually the user wants or needs to complement this model with additional entities that are used mainly during the reasoning process.
-
Declaring metadata: facts may have meta information associated to them. Examples of meta information include any kind of data that is not represented by the fact attributes and is consistent among all instances of that fact type. This meta information may be queried at runtime by the Drools engine and used in the reasoning process.
6.7.1. Declaring New Types
To declare a new type, all you need to do is use the keyword declare
, followed by the list of fields, and the keyword end
.
A new fact must have a list of fields, otherwise the Drools engine will look for an existing fact class in the classpath and raise an error if not found.
declare Address
number : int
streetName : String
city : String
end
The previous example declares a new fact type called Address
.
This fact type will have three attributes: number
, streetName
and city
.
Each attribute has a type that can be any valid Java type, including any other class created by the user or even other fact types previously declared.
For instance, we may want to declare another fact type Person
:
declare Person
name : String
dateOfBirth : java.util.Date
address : Address
end
As we can see on the previous example, dateOfBirth
is of type java.util.Date
, from the Java API, while address
is of the previously defined fact type Address.
You may avoid having to write the fully qualified name of a class every time you write it by using the import
clause, as previously discussed.
import java.util.Date
declare Person
name : String
dateOfBirth : Date
address : Address
end
When you declare a new fact type, Drools will, at compile time, generate bytecode that implements a Java class representing the fact type. The generated Java class will be a one-to-one Java Bean mapping of the type definition. So, for the previous example, the generated Java class would be:
public class Person implements Serializable {
private String name;
private java.util.Date dateOfBirth;
private Address address;
// empty constructor
public Person() {...}
// constructor with all fields
public Person( String name, Date dateOfBirth, Address address ) {...}
// if keys are defined, constructor with keys
public Person( ...keys... ) {...}
// getters and setters
// equals/hashCode
// toString
}
Since the generated class is a simple Java class, it can be used transparently in the rules, like any other fact.
rule "Using a declared Type"
when<
$p : Person( name == "Bob" )
then
// Insert Mark, who is Bob's mate.
Person mark = new Person();
mark.setName( "Mark" );
insert( mark );
end
6.7.1.1. Declaring enumerative types
DRL also supports the declaration of enumerative types. Such type declarations require the additional keyword enum, followed by a comma separated list of admissible values terminated by a semicolon.
rule "Using a declared Type"
when
$p : Person( name == "Bob" )
then
// Insert Mark, who is Bob's mate.
Person mark = new Person();
mark.setName( "Mark" );
insert( mark );
end
The compiler will generate a valid Java enum, with static methods valueOf() and values(), as well as instance methods ordinal(), compareTo() and name().
Complex enums are also partially supported, declaring the internal fields similarly to a regular type declaration. Notice that as of version 6.x, enum fields do NOT support other declared types or enums
declare enum DaysOfWeek
SUN("Sunday"),MON("Monday"),TUE("Tuesday"),WED("Wednesday"),THU("Thursday"),FRI("Friday"),SAT("Saturday");
fullName : String
end
Enumeratives can then be used in rules
rule "Using a declared Enum"
when
$p : Employee( dayOff == DaysOfWeek.MONDAY )
then
...
end
6.7.2. Declaring Metadata
Metadata may be assigned to several different constructions in Drools: fact types, fact attributes and rules. Drools uses the at sign ('@') to introduce metadata, and it always uses the form:
@metadata_key( metadata_value )
The parenthesized metadata_value is optional.
For instance, if you want to declare a metadata attribute like author
, whose value is Bob, you could simply write:
@author( Bob )
Drools allows the declaration of any arbitrary metadata attribute, but some will have special meaning to the Drools engine, while others are simply available for querying at runtime. Drools allows the declaration of metadata both for fact types and for fact attributes. Any metadata that is declared before the attributes of a fact type are assigned to the fact type, while metadata declared after an attribute are assigned to that particular attribute.
import java.util.Date
declare Person
@author( Bob )
@dateOfCreation( 01-Feb-2009 )
name : String @key @maxLength( 30 )
dateOfBirth : Date
address : Address
end
In the previous example, there are two metadata items declared for the fact type (@author
and @dateOfCreation
) and two more defined for the name attribute (@key
and @maxLength
). Please note that the @key
metadata has no required value, and so the parentheses and the value were omitted.:
6.7.2.1. Predefined class level annotations
Some annotations have predefined semantics that are interpreted by the Drools engine. The following is a list of some of these predefined annotations and their meaning.
@role( <fact | event> )
The @role annotation defines how the Drools engine should handle instances of that type: either as regular facts or as events. It accepts two possible values:
-
fact : this is the default, declares that the type is to be handled as a regular fact.
-
event : declares that the type is to be handled as an event.
The following example declares that the fact type StockTick in a stock broker application is to be handled as an event.
import some.package.StockTick
declare StockTick
@role( event )
end
The same applies to facts declared inline. If StockTick was a fact type declared in the DRL itself, instead of a previously existing class, the code would be:
declare StockTick
@role( event )
datetime : java.util.Date
symbol : String
price : double
end
@typesafe( <boolean> )
By default all type declarations are compiled with type safety enabled; @typesafe( false ) provides a means to override this behaviour by permitting a fall-back, to type unsafe evaluation where all constraints are generated as MVEL constraints and executed dynamically. This can be important when dealing with collections that do not have any generics or mixed type collections.
@timestamp( <attribute name> )
Every event has an associated timestamp assigned to it. By default, the timestamp for a given event is read from the Session Clock and assigned to the event at the time the event is inserted into the working memory. Although, sometimes, the event has the timestamp as one of its own attributes. In this case, the user may tell the Drools engine to use the timestamp from the event’s attribute instead of reading it from the Session Clock.
@timestamp( <attributeName> )
To tell the Drools engine what attribute to use as the source of the event’s timestamp, just list the attribute name as a parameter to the @timestamp tag.
declare VoiceCall
@role( event )
@timestamp( callDateTime )
end
@duration( <attribute name> )
Drools supports both event semantics: point-in-time events and interval-based events. A point-in-time event is represented as an interval-based event whose duration is zero. By default, all events have duration zero. The user may attribute a different duration for an event by declaring which attribute in the event type contains the duration of the event.
@duration( <attributeName> )
So, for our VoiceCall fact type, the declaration would be:
declare VoiceCall
@role( event )
@timestamp( callDateTime )
@duration( callDuration )
end
@expires( <time interval> )
This tag is only considered when running the Drools engine in STREAM mode. Also, additional discussion on the effects of using this tag is made on the Memory Management section. It is included here for completeness. |
Events may be automatically expired after some time in the working memory. Typically this happens when, based on the existing rules in the KIE base, the event can no longer match and activate any rules. Although, it is possible to explicitly define when an event should expire.
@expires( <timeOffset> )
The value of timeOffset is a temporal interval in the form:
[#d][#h][#m][#s][#[ms]]
Where [ ] means an optional parameter and \# means a numeric value.
So, to declare that the VoiceCall facts should be expired after 1 hour and 35 minutes after they are inserted into the working memory, the user would write:
declare VoiceCall
@role( event )
@timestamp( callDateTime )
@duration( callDuration )
@expires( 1h35m )
end
The @expires policy will take precedence and override the implicit expiration offset calculated from temporal constraints and sliding windows in the KIE base.
@propertyChangeSupport
Facts that implement support for property changes as defined in the Javabean(tm) spec, now can be annotated so that the Drools engine register itself to listen for changes on fact properties. The boolean parameter that was used in the insert() method in the Drools 4 API is deprecated and does not exist in the drools-api module.
declare Person
@propertyChangeSupport
end
@propertyReactive
Make this type property reactive. See Fine grained property change listeners section for details.
@serialVersionUID
To improve the compatibility of serialized KieSession, it has been introduced the possibility to specify the serialVersionUID on the classes generated from the declared types through an annotation like the following:
declare MyClass
@serialVersionUID( 42 )
name : String
end
6.7.2.2. Predefined attribute level annotations
As noted before, Drools also supports annotations in type attributes. Here is a list of predefined attribute annotations.
@key
Declaring an attribute as a key attribute has 2 major effects on generated types:
-
The attribute will be used as a key identifier for the type, and as so, the generated class will implement the equals() and hashCode() methods taking the attribute into account when comparing instances of this type.
-
Drools will generate a constructor using all the key attributes as parameters.
For instance:
declare Person
firstName : String @key
lastName : String @key
age : int
end
For the previous example, Drools will generate equals() and hashCode() methods that will check the firstName and lastName attributes to determine if two instances of Person are equal to each other, but will not check the age attribute. It will also generate a constructor taking firstName and lastName as parameters, allowing one to create instances with a code like this:
Person person = new Person( "John", "Doe" );
@position
Patterns support positional arguments on type declarations.
Positional arguments are ones where you don’t need to specify the field name, as the position maps to a known named field. i.e. Person( name == "mark" ) can be rewritten as Person( "mark"; ). The semicolon ';' is important so that the Drools engine knows that everything before it is a positional argument. Otherwise we might assume it was a boolean expression, which is how it could be interpreted after the semicolon. You can mix positional and named arguments on a pattern by using the semicolon ';' to separate them. Any variables used in a positional that have not yet been bound will be bound to the field that maps to that position.
declare Cheese
name : String
shop : String
price : int
end
The default order is the declared order, but this can be overridden using @position
declare Cheese
name : String @position(1)
shop : String @position(2)
price : int @position(0)
end
The @Position annotation, in the org.drools.definition.type package, can be used to annotate original pojos on the classpath. Currently only fields on classes can be annotated. Inheritance of classes is supported, but not interfaces of methods yet.
Example patterns, with two constraints and a binding. Remember semicolon ';' is used to differentiate the positional section from the named argument section. Variables and literals and expressions using just literals are supported in positional arguments, but not variables.
Cheese( "stilton", "Cheese Shop", p; )
Cheese( "stilton", "Cheese Shop"; p : price )
Cheese( "stilton"; shop == "Cheese Shop", p : price )
Cheese( name == "stilton"; shop == "Cheese Shop", p : price )
@Position is inherited when beans extend each other; while not recommended, two fields may have the same @position value, and not all consecutive values need be declared. If a @position is repeated, the conflict is solved using inheritance (fields in the superclass have the precedence) and the declaration order. If a @position value is missing, the first field without an explicit @position (if any) is selected to fill the gap. As always, conflicts are resolved by inheritance and declaration order.
declare Cheese
name : String
shop : String @position(2)
price : int @position(0)
end
declare SeasonedCheese extends Cheese
year : Date @position(0)
origin : String @position(6)
country : String
end
In the example, the field order would be : price (@position 0 in the superclass), year (@position 0 in the subclass), name (first field with no @position), shop (@position 2), country (second field without @position), origin.
6.7.3. Declaring Metadata for Existing Types
Drools allows the declaration of metadata attributes for existing types in the same way as when declaring metadata attributes for new fact types. The only difference is that there are no fields in that declaration.
For instance, if there is a class org.drools.examples.Person, and one wants to declare metadata for it, it’s possible to write the following code:
import org.drools.examples.Person
declare Person
@author( Bob )
@dateOfCreation( 01-Feb-2009 )
end
Instead of using the import, it is also possible to reference the class by its fully qualified name, but since the class will also be referenced in the rules, it is usually shorter to add the import and use the short class name everywhere.
declare org.drools.examples.Person
@author( Bob )
@dateOfCreation( 01-Feb-2009 )
end
6.7.4. Parametrized constructors for declared types
Generate constructors with parameters for declared types.
Example: for a declared type like the following:
declare Person
firstName : String @key
lastName : String @key
age : int
end
The compiler will implicitly generate 3 constructors: one without parameters, one with the @key fields, and one with all fields.
Person() // parameterless constructor
Person( String firstName, String lastName )
Person( String firstName, String lastName, int age )
6.7.5. Non Typesafe Classes
@typesafe( <boolean>) has been added to type declarations. By default all type declarations are compiled with type safety enabled; @typesafe( false ) provides a means to override this behaviour by permitting a fall-back, to type unsafe evaluation where all constraints are generated as MVEL constraints and executed dynamically. This can be important when dealing with collections that do not have any generics or mixed type collections.
6.7.6. Accessing Declared Types from the Application Code
Declared types are usually used inside rules files, while Java models are used when sharing the model between rules and applications. Although, sometimes, the application may need to access and handle facts from the declared types, especially when the application is wrapping the Drools engine and providing higher level, domain specific user interfaces for rules management.
In such cases, the generated classes can be handled as usual with the Java Reflection API, but, as we know, that usually requires a lot of work for small results. Therefore, Drools provides a simplified API for the most common fact handling the application may want to do.
The first important thing to realize is that a declared fact will belong to the package where it was declared.
So, for instance, in the example below, Person
will belong to the org.drools.examples
package, and so the fully qualified name of the generated class will be org.drools.examples.Person
.
package org.drools.examples
import java.util.Date
declare Person
name : String
dateOfBirth : Date
address : Address
end
Declared types, as discussed previously, are generated at KIE base compilation time, i.e., the application will only have access to them at application run time. Therefore, these classes are not available for direct reference from the application.
Drools then provides an interface through which users can handle declared types from the application code: org.drools.definition.type.FactType
.
Through this interface, the user can instantiate, read and write fields in the declared fact types.
// get a reference to a KIE base with a declared type:
KieBase kbase = ...
// get the declared FactType
FactType personType = kbase.getFactType( "org.drools.examples",
"Person" );
// handle the type as necessary:
// create instances:
Object bob = personType.newInstance();
// set attributes values
personType.set( bob,
"name",
"Bob" );
personType.set( bob,
"age",
42 );
// insert fact into a session
KieSession ksession = ...
ksession.insert( bob );
ksession.fireAllRules();
// read attributes
String name = personType.get( bob, "name" );
int age = personType.get( bob, "age" );
The API also includes other helpful methods, like setting all the attributes at once, reading values from a Map, or reading all attributes at once, into a Map.
Although the API is similar to Java reflection (yet much simpler to use), it does not use reflection underneath, relying on much more performant accessors implemented with generated bytecode.
6.7.7. Type Declaration 'extends'
Type declarations now support 'extends' keyword for inheritance
In order to extend a type declared in Java by a DRL declared subtype, repeat the supertype in a declare statement without any fields.
import org.people.Person
declare Person end
declare Student extends Person
school : String
end
declare LongTermStudent extends Student
years : int
course : String
end
6.8. Rule
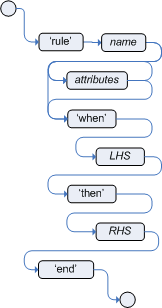
A rule specifies that when a particular set of conditions occur, specified in the Left Hand Side (LHS), then do what queryis specified as a list of actions in the Right Hand Side (RHS). A common question from users is "Why use when instead of if?" "When" was chosen over "if" because "if" is normally part of a procedural execution flow, where, at a specific point in time, a condition is to be checked.
In contrast, "when" indicates that the condition evaluation is not tied to a specific evaluation sequence or point in time, but that it happens continually, at any time during the life time of the Drools engine; whenever the condition is met, the actions are executed.
A rule must have a name, unique within its rule package. If you define a rule twice in the same DRL it produces an error while loading. If you add a DRL that includes a rule name already in the package, it replaces the previous rule. If a rule name is to have spaces, then it will need to be enclosed in double quotes (it is best to always use double quotes).
Attributes - described below - are optional. They are best written one per line.
The LHS of the rule follows the when
keyword (ideally on a new line), similarly the RHS follows
the then
keyword (again, ideally on a newline). The rule is terminated by the keyword end
.
Rules cannot be nested.
rule "<name>"
<attribute>*
when
<conditional element>*
then
<action>*
end
rule "Approve if not rejected"
salience -100
agenda-group "approval"
when
not Rejection()
p : Policy(approved == false, policyState:status)
exists Driver(age > 25)
Process(status == policyState)
then
log("APPROVED: due to no objections.");
p.setApproved(true);
end
6.8.1. Rule Attributes
Rule attributes provide a declarative way to influence the behavior of the rule. Some are quite simple, while others are part of complex subsystems such as ruleflow. To get the most from Drools you should make sure you have a proper understanding of each attribute.
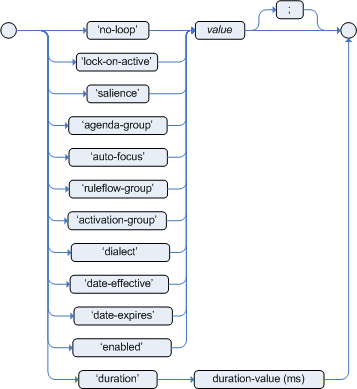
no-loop
-
default value:
false
type: Boolean
When a rule’s consequence modifies a fact it may cause the rule to activate again, causing an infinite loop. Setting no-loop to true will skip the creation of another Activation for the rule with the current set of facts.
ruleflow-group
-
default value: N/A
type: String
Ruleflow is a Drools feature that lets you exercise control over the firing of rules. Rules that are assembled by the same ruleflow-group identifier fire only when their group is active.
lock-on-active
-
default value:
false
type: Boolean
Whenever a ruleflow-group becomes active or an agenda-group receives the focus, any rule within that group that has lock-on-active set to true will not be activated any more; irrespective of the origin of the update, the activation of a matching rule is discarded. This is a stronger version of
no-loop
, because the change could now be caused not only by the rule itself. It’s ideal for calculation rules where you have a number of rules that modify a fact and you don’t want any rule re-matching and firing again. Only when the ruleflow-group is no longer active or the agenda-group loses the focus those rules with lock-on-active set to true become eligible again for their activations to be placed onto the agenda. salience
-
default value:
0
type: integer
Each rule has an integer salience attribute which defaults to zero and can be negative or positive. Salience is a form of priority where rules with higher salience values are given higher priority when ordered in the Activation queue.
Drools also supports dynamic salience where you can use an expression involving bound variables.
rule "Fire in rank order 1,2,.."
salience( -$rank )
when
Element( $rank : rank,... )
then
...
end
agenda-group
-
default value: MAIN
type: String
Agenda groups allow the user to partition the Agenda providing more execution control. Only rules in the agenda group that has acquired the focus are allowed to fire.
auto-focus
-
default value:
false
type: Boolean
When a rule is activated where the
auto-focus
value is true and the rule’s agenda group does not have focus yet, then it is given focus, allowing the rule to potentially fire. activation-group
-
default value: N/A
type: String
Rules that belong to the same activation-group, identified by this attribute’s string value, will only fire exclusively. More precisely, the first rule in an activation-group to fire will cancel all pending activations of all rules in the group, i.e., stop them from firing.
Note: This used to be called Xor group, but technically it’s not quite an Xor. You may still hear people mention Xor group; just swap that term in your mind with activation-group.
dialect
-
default value: as specified by the package
type: String
possible values: "java" or "mvel"
The dialect species the language to be used for any code expressions in the LHS or the RHS code block. Currently two dialects are available, Java and MVEL. While the dialect can be specified at the package level, this attribute allows the package definition to be overridden for a rule.
date-effective
-
default value: N/A
type: String, containing a date and time definition
A rule can only activate if the current date and time is after date-effective attribute.
date-expires
-
default value: N/A
type: String, containing a date and time definition
A rule cannot activate if the current date and time is after the date-expires attribute.
duration
-
default value: no default value
type: long
The duration dictates that the rule will fire after a specified duration, if it is still true.
enabled
-
default value:
true
type: boolean
If false, the rule is not fired even if the condition is met. Note that the rule is still evaluated so it could affect performance even if it’s false.
rule "my rule"
salience 42
agenda-group "number 1"
when ...
6.8.2. Timers and Calendars
Rules now support both interval and cron based timers, which replace the now deprecated duration attribute.
timer ( int: <initial delay> <repeat interval>? )
timer ( int: 30s )
timer ( int: 30s 5m )
timer ( cron: <cron expression> )
timer ( cron:* 0/15 * * * ? )
Interval (indicated by "int:") timers follow the semantics of java.util.Timer objects, with an initial delay and an optional repeat interval. Cron (indicated by "cron:") timers follow standard Unix cron expressions:
rule "Send SMS every 15 minutes"
timer (cron:* 0/15 * * * ?)
when
$a : Alarm( on == true )
then
channels[ "sms" ].insert( new Sms( $a.mobileNumber, "The alarm is still on" );
end
A rule controlled by a timer becomes active when it matches, and once for each individual match. Its consequence is executed repeatedly, according to the timer’s settings. This stops as soon as the condition doesn’t match any more.
Consequences are executed even after control returns from a call to fireUntilHalt. Moreover, the Drools engine remains reactive to any changes made to the Working Memory. For instance, removing a fact that was involved in triggering the timer rule’s execution causes the repeated execution to terminate, or inserting a fact so that some rule matches will cause that rule to fire. But the Drools engine is not continually active, only after a rule fires, for whatever reason. Thus, reactions to an insertion done asynchronously will not happen until the next execution of a timer-controlled rule. Disposing a session puts an end to all timer activity.
Conversely when the Drools engine runs in passive mode (i.e.: using fireAllRules instead of fireUntilHalt) by default it doesn’t fire consequences of timed rules unless fireAllRules isn’t invoked again.
However it is possible to change this default behavior by configuring the KieSession with a TimedRuleExecutionOption
as shown in the following example.
KieSessionConfiguration ksconf = KieServices.Factory.get().newKieSessionConfiguration();
ksconf.setOption( TimedRuleExecutionOption.YES );
KSession ksession = kbase.newKieSession(ksconf, null);
It is also possible to have a finer grained control on the timed rules that have to be automatically executed.
To do this it is necessary to set a FILTERED
TimedRuleExecutionOption
that allows to define a
callback to filter those rules, as done in the next example.
KieSessionConfiguration ksconf = KieServices.Factory.get().newKieSessionConfiguration();
conf.setOption( new TimedRuleExecutionOption.FILTERED(new TimedRuleExecutionFilter() {
public boolean accept(Rule[] rules) {
return rules[0].getName().equals("MyRule");
}
}) );
For what regards interval timers it is also possible to define both the delay and interval as an expression instead of a fixed value. To do that it is necessary to use an expression timer (indicated by "expr:") as in the following example:
declare Bean
delay : String = "30s"
period : long = 60000
end
rule "Expression timer"
timer( expr: $d, $p )
when
Bean( $d : delay, $p : period )
then
end
The expressions, $d
and $p
in this case, can use any variable defined in the pattern matching part
of the rule and can be any String that can be parsed in a time duration or any numeric value that
will be internally converted in a long representing a duration expressed in milliseconds.
Both interval and expression timers can have 3 optional parameters named "start", "end" and "repeat-limit". When one or more of these parameters are used the first part of the timer definition must be followed by a semicolon ';' and the parameters have to be separated by a comma ',' as in the following example:
timer (int: 30s 10s; start=3-JAN-2010, end=5-JAN-2010)
The value for start and end parameters can be a Date, a String representing a Date or a long, or more in general any Number, that will be transformed in a Java Date applying the following conversion:
new Date( ((Number) n).longValue() )
Conversely the repeat-limit can be only an integer and it defines the maximum number of repetitions allowed by the timer. If both the end and the repeat-limit parameters are set the timer will stop when the first of the two will be matched.
The using of the start parameter implies the definition of a phase for the timer, where the beginning of the phase is given by the start itself plus the eventual delay. In other words in this case the timed rule will then be scheduled at times:
start + delay + n*period
for up to repeat-limit times and no later than the end timestamp (whichever first). For instance the rule having the following interval timer
timer ( int: 30s 1m; start="3-JAN-2010" )
will be scheduled at the 30th second of every minute after the midnight of the 3-JAN-2010. This also means that if for example you turn the system on at midnight of the 3-FEB-2010 it won’t be scheduled immediately but will preserve the phase defined by the timer and so it will be scheduled for the first time 30 seconds after the midnight.
If for some reason the system is paused (e.g. the session is serialized and then deserialized after a while) the rule will be scheduled only once to recover from missing activations (regardless of how many activations we missed) and subsequently it will be scheduled again in phase with the timer.
Calendars are used to control when rules can fire. The Calendar API is modelled on Quartz:
Calendar weekDayCal = QuartzHelper.quartzCalendarAdapter(org.quartz.Calendar quartzCal)
Calendars are registered with the KieSession
:
ksession.getCalendars().set( "weekday", weekDayCal );
They can be used in conjunction with normal rules and rules including timers. The rule attribute "calendars" may contain one or more comma-separated calendar names written as string literals.
rule "weekdays are high priority"
calendars "weekday"
timer (int:0 1h)
when
Alarm()
then
send( "priority high - we have an alarm" );
end
rule "weekend are low priority"
calendars "weekend"
timer (int:0 4h)
when
Alarm()
then
send( "priority low - we have an alarm" );
end
6.8.3. Left Hand Side (when) syntax
6.8.3.1. What is the Left Hand Side?
The Left Hand Side (LHS) is a common name for the conditional part of the rule. It consists of zero or more Conditional Elements. If the LHS is empty, it will be considered as a condition element that is always true and it will be activated once, when a new WorkingMemory session is created.

rule "no CEs"
when
// empty
then
... // actions (executed once)
end
// The above rule is internally rewritten as:
rule "eval(true)"
when
eval( true )
then
... // actions (executed once)
end
Conditional elements work on one or more patterns (which are described below). The most common
conditional element is " and"
. Therefore it is implicit when you have multiple patterns in the
LHS of a rule that are not connected in any way:
rule "2 unconnected patterns"
when
Pattern1()
Pattern2()
then
... // actions
end
// The above rule is internally rewritten as:
rule "2 and connected patterns"
when
Pattern1()
and Pattern2()
then
... // actions
end
An “and” cannot have a leading declaration binding (unlike for example
|
6.8.3.2. Pattern (conditional element)
What is a pattern?
A pattern element is the most important Conditional Element. It can potentially match on each fact that is inserted in the working memory.
A pattern contains of zero or more constraints and has an optional pattern binding. The railroad diagram below shows the syntax for this.

In its simplest form, with no constraints, a pattern matches against a fact of the given type.
In the following case the type is Cheese
, which means that the pattern will match against all Person
objects in the Working Memory:
Person()
The type need not be the actual class of some fact object. Patterns may refer to superclasses or even interfaces, thereby potentially matching facts from many different classes.
Object() // matches all objects in the working memory
Inside of the pattern parenthesis is where all the action happens: it defines the constraints for that pattern. For example, with a age related constraint:
Person( age == 100 )
For backwards compatibility reasons it’s allowed to suffix patterns with the |
Pattern binding
For referring to the matched object, use a pattern binding variable such as $p
.
rule ...
when
$p : Person()
then
System.out.println( "Person " + $p );
end
The prefixed dollar symbol ($
) is just a convention; it can be useful in complex rules where it helps to easily differentiate between variables and fields, but it is not mandatory.
6.8.3.3. Constraint (part of a pattern)
What is a constraint?
A constraint is an expression that returns true
or false
.
This example has a constraint that states 5 is smaller than
6:
Person( 5 < 6 ) // just an example, as constraints like this would be useless in a real pattern
In essence, it’s a Java expression with some enhancements (such as property access) and a few differences (such as equals()
semantics for ==
). Let’s take a deeper look.
Property access on Java Beans (POJO’s)
Any bean property can be used directly.
A bean property is exposed using a standard Java bean getter: a method getMyProperty()
(or isMyProperty()
for a primitive boolean) which takes no arguments and return something.
For example: the age property is written as age
in DRL instead of the getter getAge()
:
Person( age == 50 )
// this is the same as:
Person( getAge() == 50 )
Drools uses the standard JDK Introspector
class to do this mapping, so it follows the standard Java bean specification.
We recommend using property access ( |
Property accessors must not change the state of the object in a way that may effect the rules. Remember that the Drools engine effectively caches the results of its matching in between invocations to make it faster.
To solve this latter case, insert a fact that wraps the current date into working memory and update that fact between |
The following fallback applies: if the getter of a property cannot be found, the compiler will resort to using the property name as a method name and without arguments:
|
Nested property access is also supported:
Person( address.houseNumber == 50 )
// this is the same as:
Person( getAddress().getHouseNumber() == 50 )
Nested properties are also indexed.
In a stateful session, care should be taken when using nested accessors as the Working Memory is not aware of any of the nested values, and does not know when they change.
Either consider them immutable while any of their parent references are inserted into the Working Memory.
Or, instead, if you wish to modify a nested value you should mark all of the outer facts as updated.
In the above example, when the |
Java expression
You can use any Java expression that returns a boolean
as a constraint inside the parentheses of a pattern.
Java expressions can be mixed with other expression enhancements, such as property access:
Person( age == 50 )
It is possible to change the evaluation priority by using parentheses, as in any logic or mathematical expression:
Person( age > 100 && ( age % 10 == 0 ) )
It is possible to reuse Java methods:
Person( Math.round( weight / ( height * height ) ) < 25.0 )
As for property accessors, methods must not change the state of the object in a way that may affect the rules. Any method executed on a fact in the LHS should be a read only method.
|
The state of a fact should not change between rule invocations (unless those facts are marked as updated to the working memory on every change):
|
Normal Java operator precedence applies, see the operator precedence list below.
All operators have normal Java semantics except for The
The
|
Type coercion is always attempted if the field and the value are of different types; exceptions will be thrown if a bad coercion is attempted. For instance, if "ten" is provided as a string in a numeric evaluator, an exception is thrown, whereas "10" would coerce to a numeric 10. Coercion is always in favor of the field type and not the value type:
Person( age == "10" ) // "10" is coerced to 10
Comma separated AND
The comma character (‘`,`’) is used to separate constraint groups. It has implicit AND connective semantics.
// Person is at least 50 and weighs at least 80 kg
Person( age > 50, weight > 80 )
// Person is at least 50, weighs at least 80 kg and is taller than 2 meter.
Person( age > 50, weight > 80, height > 2 )
Although the The comma operator should be preferred at the top level constraint, as it makes constraints easier to read and the Drools engine will often be able to optimize them better. |
The comma (,
) operator cannot be embedded in a composite constraint expression, such as parentheses:
Person( ( age > 50, weight > 80 ) || height > 2 ) // Do NOT do this: compile error
// Use this instead
Person( ( age > 50 && weight > 80 ) || height > 2 )
Binding variables
A property can be bound to a variable:
// 2 persons of the same age
Person( $firstAge : age ) // binding
Person( age == $firstAge ) // constraint expression
The prefixed dollar symbol ($
) is just a convention; it can be useful in complex rules where it helps to easily differentiate between variables and fields.
For backwards compatibility reasons, It’s allowed (but not recommended) to mix a constraint binding and constraint expressions as such:
|
Bound variable restrictions using the operator ==
provide for very fast execution as it use hash indexing to improve performance.
Unification
Drools does not allow bindings to the same declaration. However this is an important aspect to derivation query unification. While positional arguments are always processed with unification a special unification symbol, ':=', was introduced for named arguments named arguments. The following "unifies" the age argument across two people.
Person( $age := age )
Person( $age := age)
In essence unification will declare a binding for the first occurrence and constrain to the same value of the bound field for sequence occurrences.
Grouped accessors for nested objects
Often it happens that it is necessary to access multiple properties of a nested object as in the following example
Person( name == "mark", address.city == "london", address.country == "uk" )
These accessors to nested objects can be grouped with a '.(…)' syntax providing more readable rules as in
Person( name == "mark", address.( city == "london", country == "uk") )
Note the '.' prefix, this is necessary to differentiate the nested object constraints from a method call.
Inline casts and coercion
When dealing with nested objects, it also quite common the need to cast to a subtype. It is possible to do that via the # symbol as in:
Person( name == "mark", address#LongAddress.country == "uk" )
This example casts Address to LongAddress, making its getters available. If the cast is not possible (instanceof returns false), the evaluation will be considered false. Also fully qualified names are supported:
Person( name == "mark", address#org.domain.LongAddress.country == "uk" )
It is possible to use multiple inline casts in the same expression:
Person( name == "mark", address#LongAddress.country#DetailedCountry.population > 10000000 )
moreover, since we also support the instanceof operator, if that is used we will infer its results for further uses of that field, within that pattern:
Person( name == "mark", address instanceof LongAddress, address.country == "uk" )
Special literal support
Besides normal Java literals (including Java 5 enums), this literal is also supported:
Date literal
The date format dd-MMM-yyyy
is supported by default.
You can customize this by providing an alternative date format mask as the system property named drools.dateformat
. This system property can also contain a time format mask part (e.g.drools.dateformat="dd-MMM-yyyy HH:mm"
).
Another possibility to customize the date format is to change the language locale with drools.defaultlanguage
and drools.defaultcountry
system properties (e.g. locale of Thailand set as drools.defaultlanguage=th
and drools.defaultcountry=TH
).
Cheese( bestBefore < "27-Oct-2009" )
List and Map access
It’s possible to directly access a List
value by index:
// Same as childList(0).getAge() == 18
Person( childList[0].age == 18 )
It’s also possible to directly access a Map
value by key:
// Same as credentialMap.get("jsmith").isValid()
Person( credentialMap["jsmith"].valid )
Abbreviated combined relation condition
This allows you to place more than one restriction on a field using the restriction connectives &&
or ||
.
Grouping via parentheses is permitted, resulting in a recursive syntax pattern.
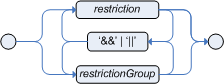

// Simple abbreviated combined relation condition using a single &&
Person( age > 30 && < 40 )
// Complex abbreviated combined relation using groupings
Person( age ( (> 30 && < 40) ||
(> 20 && < 25) ) )
// Mixing abbreviated combined relation with constraint connectives
Person( age > 30 && < 40 || location == "london" )
Special DRL operators

Coercion to the correct value for the evaluator and the field will be attempted.
The operators < ⇐ > >=
These operators can be used on properties with natural ordering.
For example, for Date fields, <
means before, for String
fields, it means alphabetically lower.
Person( firstName < $otherFirstName )
Person( birthDate < $otherBirthDate )
Only applies on Comparable
properties.
Null-safe dereferencing operator
The !. operator allows to derefencing in a null-safe way. More in details the matching algorithm requires the value to the left of the !. operator to be not null in order to give a positive result for pattern matching itself. In other words the pattern:
Person( $streetName : address!.street )
will be internally translated in:
Person( address != null, $streetName : address.street )
The operator matches
Matches a field against any valid Java Regular Expression. Typically that regexp is a string literal, but variables that resolve to a valid regexp are also allowed.
Cheese( type matches "(Buffalo)?\\S*Mozzarella" )
Like in Java, regular expressions written as string literals need to escape '\\'. |
Only applies on String
properties.
Using matches
against a null
value always evaluates to false.
The operator not matches
The operator returns true if the String does not match the regular expression.
The same rules apply as for the matches
operator.
Example:
Cheese( type not matches "(Buffalo)?\\S*Mozzarella" )
Only applies on String
properties.
Using not matches
against a null
value always evaluates to true.
The operator contains
The operator contains
is used to check whether a field that is a
Collection or elements contains the specified value.
CheeseCounter( cheeses contains "stilton" ) // contains with a String literal
CheeseCounter( cheeses contains $var ) // contains with a variable
Only applies on Collection
properties.
The operator contains
can also be used in place of String.contains()
constraints checks.
Cheese( name contains "tilto" )
Person( fullName contains "Jr" )
String( this contains "foo" )
The operator not contains
The operator not contains
is used to check whether a field that is a
Collection or elements does not contain the specified value.
CheeseCounter( cheeses not contains "cheddar" ) // not contains with a String literal
CheeseCounter( cheeses not contains $var ) // not contains with a variable
Only applies on Collection
properties.
For backward compatibility, the |
The operator not contains
can also be used in place of the logical negation of String.contains()
for constraints checks - i.e.: ! String.contains()
Cheese( name not contains "tilto" )
Person( fullName not contains "Jr" )
String( this not contains "foo" )
The operator memberOf
The operator memberOf
is used to check whether a field is a member of a collection or elements; that collection must be a variable.
CheeseCounter( cheese memberOf $matureCheeses )
The operator not memberOf
The operator not memberOf
is used to check whether a field is not a member of a collection or elements; that collection must be a variable.
CheeseCounter( cheese not memberOf $matureCheeses )
The operator soundslike
This operator is similar to matches
, but it checks whether a word has almost the same sound (using English pronunciation) as the given value.
This is based on the Soundex algorithm (see http://en.wikipedia.org/wiki/Soundex
).
// match cheese "fubar" or "foobar"
Cheese( name soundslike 'foobar' )
The operator str
This operator str
is used to check whether a field that is a String
starts with or ends with a certain value.
It can also be used to check the length of the String.
Message( routingValue str[startsWith] "R1" )
Message( routingValue str[endsWith] "R2" )
Message( routingValue str[length] 17 )
The operators in
and notin
(compound value restriction)
The compound value restriction is used where there is more than one possible value to match.
Currently only the in
and not in
evaluators support this.
The second operand of this operator must be a comma-separated list of values, enclosed in parentheses.
Values may be given as variables, literals, return values or qualified identifiers.
Both evaluators are actually syntactic
sugar, internally rewritten as a list of multiple restrictions using the operators !=
and ==
.
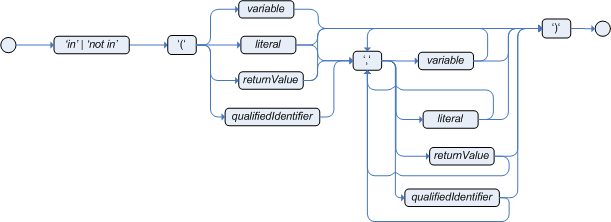
Person( $cheese : favouriteCheese )
Cheese( type in ( "stilton", "cheddar", $cheese ) )
Inline eval operator (deprecated)

An inline eval constraint can use any valid dialect expression as long as it results to a primitive boolean. The expression must be constant over time. Any previously bound variable, from the current or previous pattern, can be used; autovivification is also used to auto-create field binding variables. When an identifier is found that is not a current variable, the builder looks to see if the identifier is a field on the current object type, if it is, the field binding is auto-created as a variable of the same name. This is called autovivification of field variables inside of inline eval’s.
This example will find all male-female pairs where the male is 2 years older than the female; the variable age
is auto-created in the second pattern by the autovivification process.
Person( girlAge : age, sex = "F" )
Person( eval( age == girlAge + 2 ), sex = 'M' ) // eval() is actually obsolete in this example
Inline eval’s are effectively obsolete as their inner syntax is now directly supported. It’s recommended not to use them. Simply write the expression without wrapping eval() around it. |
Operator precedence
The operators are evaluated in this precedence:
Operator type | Operators | Notes |
---|---|---|
(nested / null safe) property access |
|
Not normal Java semantics |
List/Map access |
|
Not normal Java semantics |
constraint binding |
|
Not normal Java semantics |
multiplicative |
|
|
additive |
|
|
shift |
|
|
relational |
|
|
equality |
|
Does not use normal Java (not) same semantics: uses (not) equals semantics instead. |
non-short circuiting AND |
|
|
non-short circuiting exclusive OR |
|
|
non-short circuiting inclusive OR |
|
|
logical AND |
|
|
logical OR |
|
|
ternary |
|
|
Comma separated AND |
|
Not normal Java semantics |
6.8.3.4. Positional Arguments
Patterns now support positional arguments on type declarations.
Positional arguments are ones where you don’t need to specify the field name, as the position maps to a known named field. i.e. Person( name == "mark" ) can be rewritten as Person( "mark"; ). The semicolon ';' is important so that the Drools engine knows that everything before it is a positional argument. Otherwise we might assume it was a boolean expression, which is how it could be interpreted after the semicolon. You can mix positional and named arguments on a pattern by using the semicolon ';' to separate them. Any variables used in a positional that have not yet been bound will be bound to the field that maps to that position.
declare Cheese
name : String
shop : String
price : int
end
Example patterns, with two constraints and a binding. Remember semicolon ';' is used to differentiate the positional section from the named argument section. Variables and literals and expressions using just literals are supported in positional arguments, but not variables. Positional arguments are always resolved using unification.
Cheese( "stilton", "Cheese Shop", p; )
Cheese( "stilton", "Cheese Shop"; p : price )
Cheese( "stilton"; shop == "Cheese Shop", p : price )
Cheese( name == "stilton"; shop == "Cheese Shop", p : price )
Positional arguments that are given a previously declared binding will constrain against that using unification; these are referred to as input arguments. If the binding does not yet exist, it will create the declaration binding it to the field represented by the position argument; these are referred to as output arguments.
6.8.3.5. Fine grained property change listeners
When you call modify() (see the modify statement section) on a given object it will trigger a revaluation of all patterns of the matching object type in the KIE base. This can can lead to unwanted and useless evaluations and in the worst cases to infinite recursions. The only workaround to avoid it was to split up your objects into smaller ones having a 1 to 1 relationship with the original object.
This has been introduced to provide an easier and more consistent way to overcome this problem. In fact it allows the pattern matching to only react to modification of properties actually constrained or bound inside of a given pattern. That will help with performance and recursion and avoid artificial object splitting.
This feature is enabled by default, but in case you need or want to dectivate it on a specific bean you can annotate it with @classReactive. This annotation works both on DRL type declarations:
declare Person
@classReactive
firstName : String
lastName : String
end
and on Java classes:
@ClassReactive
public static class Person {
private String firstName;
private String lastName;
}
By using this feature, for instance, if you have a rule like the following:
rule "Every person named Mario is a male" when
$person : Person( firstName == "Mario" )
then
modify ( $person ) { setMale( true ) }
end
you won’t have to add the no-loop attribute to it in order to avoid an infinite recursion because the Drools engine recognizes that the pattern matching is done on the 'firstName' property while the RHS of the rule modifies the 'male' one. Note that this feature does not work for update(), and this is one of the reasons why we promote modify() since it encapsulates the field changes within the statement. Moreover, on Java classes, you can also annotate any method to say that its invocation actually modifies other properties. For instance in the former Person class you could have a method like:
@Modifies( { "firstName", "lastName" } )
public void setName(String name) {
String[] names = name.split("\\s");
this.firstName = names[0];
this.lastName = names[1];
}
That means that if a rule has a RHS like the following:
modify($person) { setName("Mario Fusco") }
it will correctly recognize that the values of both properties 'firstName' and 'lastName' could have potentially been modified and act accordingly, not missing of reevaluating the patterns constrained on them. At the moment the usage of @Modifies is not allowed on fields but only on methods. This is coherent with the most common scenario where the @Modifies will be used for methods that are not related with a class field as in the Person.setName() in the former example. Also note that @Modifies is not transitive, meaning that if another method internally invokes the Person.setName() one it won’t be enough to annotate it with @Modifies( { "name" } ), but it is necessary to use @Modifies( { "firstName", "lastName" } ) even on it. Very likely @Modifies transitivity will be implemented in the next release.
For what regards nested accessors, the Drools engine will be notified only for top level fields. In other words a pattern matching like:
Person ( address.city.name == "London )
will be revaluated only for modification of the 'address' property of a Person object. In the same way the constraints analysis is currently strictly limited to what there is inside a pattern. Another example could help to clarify this. An LHS like the following:
$p : Person( )
Car( owner = $p.name )
will not listen on modifications of the person’s name, while this one will do:
Person( $name : name )
Car( owner = $name )
To overcome this problem it is possible to annotate a pattern with @watch as it follows:
$p : Person( ) @watch ( name )
Car( owner = $p.name )
Indeed, annotating a pattern with @watch allows you to modify the inferred set of properties for which that pattern will react. Note that the properties named in the @watch annotation are actually added to the ones automatically inferred, but it is also possible to explicitly exclude one or more of them prepending their name with a ! and to make the pattern to listen for all or none of the properties of the type used in the pattern respectively with the wildcrds * and !*. So, for example, you can annotate a pattern in the LHS of a rule like:
// listens for changes on both firstName (inferred) and lastName
Person( firstName == $expectedFirstName ) @watch( lastName )
// listens for all the properties of the Person bean
Person( firstName == $expectedFirstName ) @watch( * )
// listens for changes on lastName and explicitly exclude firstName
Person( firstName == $expectedFirstName ) @watch( lastName, !firstName )
// listens for changes on all the properties except the age one
Person( firstName == $expectedFirstName ) @watch( *, !age )
Since it doesn’t make sense to use this annotation on a pattern using a type annotated with @ClassReactive the rule compiler will raise a compilation error if you try to do so. Also the duplicated usage of the same property in @watch (for example like in: @watch( firstName, ! firstName ) ) will end up in a compilation error. In a next release we will make the automatic detection of the properties to be listened smarter by doing analysis even outside of the pattern.
It is also possible to enable this feature only on specific types of your model or to completely disallow it by using on option of the KnowledgeBuilderConfiguration. In particular this new PropertySpecificOption can have one of the following 3 values:
- DISABLED => the feature is turned off and all the other related annotations are just ignored
- ALLOWED => types are not property reactive unless they are not annotated with @PropertyReactive (which is the dual of @ClassReactive)
- ALWAYS => all types are property reactive. This is the default behavior
So, for example, to have a KnowledgeBuilder for which property reactivity is disabled by default:
KnowledgeBuilderConfiguration config = KnowledgeBuilderFactory.newKnowledgeBuilderConfiguration();
config.setOption(PropertySpecificOption.ALLOWED);
KnowledgeBuilder kbuilder = KnowledgeBuilderFactory.newKnowledgeBuilder(config);
In this last case it will be possible to reenable the property reactivity feature on a specific type by annotating it with @PropertyReactive.
It is important to notice that property reactivity is automatically available only for modifications performed inside the consequence of a rule. Conversely a programmatic update is unaware of the object’s properties that have been changed, so it is unable of using this feature.
To workaround this limitation it is possible to optionally specify in an update statement the names of the properties that have been changed in the modified object as in the following example:
Person me = new Person("me", 40);
FactHandle meHandle = ksession.insert( me );
me.setAge(41);
me.setAddress("California Avenue");
ksession.update( meHandle, me, "age", "address" );
6.8.3.6. Basic conditional elements
Conditional Element and
The Conditional Element "and"
is used to group other Conditional Elements into a logical conjunction.
Drools supports both prefix and
and infix and
.

Traditional infix and
is supported:
//infixAnd
Cheese( cheeseType : type ) and Person( favouriteCheese == cheeseType )
Explicit grouping with parentheses is also supported:
//infixAnd with grouping
( Cheese( cheeseType : type ) and
( Person( favouriteCheese == cheeseType ) or
Person( favouriteCheese == cheeseType ) )
The symbol |

Prefix and
is also supported:
(and Cheese( cheeseType : type )
Person( favouriteCheese == cheeseType ) )
The root element of the LHS is an implicit prefix and
and doesn’t need to be specified:
when
Cheese( cheeseType : type )
Person( favouriteCheese == cheeseType )
then
...
Conditional Element or
The Conditional Element or
is used to group other Conditional Elements into a logical disjunction.
Drools supports both prefix or
and infix or
.

Traditional infix or
is supported:
//infixOr
Cheese( cheeseType : type ) or Person( favouriteCheese == cheeseType )
Explicit grouping with parentheses is also supported:
//infixOr with grouping
( Cheese( cheeseType : type ) or
( Person( favouriteCheese == cheeseType ) and
Person( favouriteCheese == cheeseType ) )
The symbol |

Prefix or
is also supported:
(or Person( sex == "f", age > 60 )
Person( sex == "m", age > 65 )
)
The behavior of the Conditional Element |
The Conditional Element or
also allows for optional pattern binding.
This means that each resulting subrule will bind its pattern to the pattern binding.
Each pattern must be bound separately, using eponymous variables:
pensioner : ( Person( sex == "f", age > 60 ) or Person( sex == "m", age > 65 ) )
(or pensioner : Person( sex == "f", age > 60 )
pensioner : Person( sex == "m", age > 65 ) )
Since the conditional element or
results in multiple subrule generation, one for each possible logically outcome, the example above would result in the internal generation of two rules.
These two rules work independently within the Working Memory, which means both can match, activate and fire - there is no shortcutting.
The best way to think of the conditional element or
is as a shortcut for generating two or more similar rules.
When you think of it that way, it’s clear that for a single rule there could be multiple activations if two or more terms of the disjunction are true.
Conditional Element not

The CE not
is first order logic’s non-existential quantifier and checks for the non-existence of something in the Working Memory.
Think of "not" as meaning "there must be none of…".
The keyword not
may be followed by parentheses around the CEs that it applies to.
In the simplest case of a single pattern (like below) you may optionally omit the parentheses.
not Bus()
// Brackets are optional:
not Bus(color == "red")
// Brackets are optional:
not ( Bus(color == "red", number == 42) )
// "not" with nested infix and - two patterns,
// brackets are requires:
not ( Bus(color == "red") and
Bus(color == "blue") )
Conditional Element exists

The CE exists
is first order logic’s existential quantifier and checks for the existence of something in the Working Memory.
Think of "exists" as meaning "there is at least one..". It is different from just having the pattern on its own, which is more like saying "for each one of…". If you use exists
with a pattern, the rule will only activate at most once, regardless of how much data there is in working memory that matches the condition inside of the exists
pattern.
Since only the existence matters, no bindings will be established.
The keyword exists
must be followed by parentheses around the CEs that it applies to.
In the simplest case of a single pattern (like below) you may omit the parentheses.
exists Bus()
exists Bus(color == "red")
// brackets are optional:
exists ( Bus(color == "red", number == 42) )
// "exists" with nested infix and,
// brackets are required:
exists ( Bus(color == "red") and
Bus(color == "blue") )
6.8.3.7. Advanced conditional elements
Conditional Element forall

The Conditional Element forall
completes the First Order Logic support in Drools.
The Conditional Element forall
evaluates to true when all facts that match the first pattern match all the remaining patterns.
Example:
rule "All English buses are red"
when
forall( $bus : Bus( type == 'english')
Bus( this == $bus, color = 'red' ) )
then
// all English buses are red
end
In the above rule, we "select" all Bus objects whose type is "english". Then, for each fact that matches this pattern we evaluate the following patterns and if they match, the forall CE will evaluate to true.
To state that all facts of a given type in the working memory must match a set of constraints, forall
can be written with a single pattern for simplicity.
Example:
rule "All Buses are Red"
when
forall( Bus( color == 'red' ) )
then
// all Bus facts are red
end
Another example shows multiple patterns inside the forall
:
rule "all employees have health and dental care programs"
when
forall( $emp : Employee()
HealthCare( employee == $emp )
DentalCare( employee == $emp )
)
then
// all employees have health and dental care
end
Forall can be nested inside other CEs.
For instance, forall
can be used inside a not
CE.
Note that only single patterns have optional parentheses, so that with a nested forall
parentheses must be used:
rule "not all employees have health and dental care"
when
not ( forall( $emp : Employee()
HealthCare( employee == $emp )
DentalCare( employee == $emp ) )
)
then
// not all employees have health and dental care
end
As a side note, forall( p1 p2 p3…)
is equivalent to writing:
not(p1 and not(and p2 p3...))
Also, it is important to note that forall
is a scope delimiter.
Therefore, it can use any previously bound variable, but no variable bound inside it will be available for use outside of it.
Conditional Element from

The Conditional Element from
enables users to specify an arbitrary source for data to be matched by LHS patterns.
This allows the Drools engine to reason over data not in the Working Memory.
The data source could be a sub-field on a bound variable or the results of a method call.
It is a powerful construction that allows out of the box integration with other application components and frameworks.
One common example is the integration with data retrieved on-demand from databases using hibernate named queries.
The expression used to define the object source is any expression that follows regular MVEL syntax. Therefore, it allows you to easily use object property navigation, execute method calls and access maps and collections elements.
Here is a simple example of reasoning and binding on another pattern sub-field:
rule "validate zipcode"
when
Person( $personAddress : address )
Address( zipcode == "23920W") from $personAddress
then
// zip code is ok
end
With all the flexibility from the new expressiveness in the Drools engine you can slice and dice this problem many ways. This is the same but shows how you can use a graph notation with the 'from':
rule "validate zipcode"
when
$p : Person( )
$a : Address( zipcode == "23920W") from $p.address
then
// zip code is ok
end
Previous examples were evaluations using a single pattern.
The CE from
also support object sources that return a collection of objects.
In that case, from
will iterate over all objects in the collection and try to match each of them individually.
For instance, if we want a rule that applies 10% discount to each item in an order, we could do:
rule "apply 10% discount to all items over US$ 100,00 in an order"
when
$order : Order()
$item : OrderItem( value > 100 ) from $order.items
then
// apply discount to $item
end
The above example will cause the rule to fire once for each item whose value is greater than 100 for each given order.
You must take caution, however, when using from
, especially in conjunction with the lock-on-active
rule attribute as it may produce unexpected results.
Consider the example provided earlier, but now slightly modified as follows:
rule "Assign people in North Carolina (NC) to sales region 1"
ruleflow-group "test"
lock-on-active true
when
$p : Person( )
$a : Address( state == "NC") from $p.address
then
modify ($p) {} // Assign person to sales region 1 in a modify block
end
rule "Apply a discount to people in the city of Raleigh"
ruleflow-group "test"
lock-on-active true
when
$p : Person( )
$a : Address( city == "Raleigh") from $p.address
then
modify ($p) {} // Apply discount to person in a modify block
end
In the above example, persons in Raleigh, NC should be assigned to sales region 1 and receive a discount; i.e., you would expect both rules to activate and fire. Instead you will find that only the second rule fires.
If you were to turn on the audit log, you would also see that when the second rule fires, it deactivates the first rule.
Since the rule attribute lock-on-active
prevents a rule from creating new activations when a set of facts change, the first rule fails to reactivate.
Though the set of facts have not changed, the use of from
returns a new fact for all intents and purposes each time it is evaluated.
First, it’s important to review why you would use the above pattern.
You may have many rules across different rule-flow groups.
When rules modify working memory and other rules downstream of your RuleFlow (in different rule-flow groups) need to be reevaluated, the use of modify
is critical.
You don’t, however, want other rules in the same rule-flow group to place activations on one another recursively.
In this case, the no-loop
attribute is ineffective, as it would only prevent a rule from activating itself recursively.
Hence, you resort to lock-on-active
.
There are several ways to address this issue:
-
Avoid the use of
from
when you can assert all facts into working memory or use nested object references in your constraint expressions (shown below). -
Place the variable assigned used in the modify block as the last sentence in your condition (LHS).
-
Avoid the use of
lock-on-active
when you can explicitly manage how rules within the same rule-flow group place activations on one another (explained below).
The preferred solution is to minimize use of from
when you can assert all your facts into working memory directly.
In the example above, both the Person and Address instance can be asserted into working memory.
In this case, because the graph is fairly simple, an even easier solution is to modify your rules as follows:
rule "Assign people in North Carolina (NC) to sales region 1"
ruleflow-group "test"
lock-on-active true
when
$p : Person(address.state == "NC" )
then
modify ($p) {} // Assign person to sales region 1 in a modify block
end
rule "Apply a discount to people in the city of Raleigh"
ruleflow-group "test"
lock-on-active true
when
$p : Person(address.city == "Raleigh" )
then
modify ($p) {} //Apply discount to person in a modify block
end
Now, you will find that both rules fire as expected.
However, it is not always possible to access nested facts as above.
Consider an example where a Person holds one or more Addresses and you wish to use an existential quantifier to match people with at least one address that meets certain conditions.
In this case, you would have to resort to the use of from
to reason over the collection.
There are several ways to use from
to achieve this and not all of them exhibit an issue with the use of lock-on-active
.
For example, the following use of from
causes both rules to fire as expected:
rule "Assign people in North Carolina (NC) to sales region 1"
ruleflow-group "test"
lock-on-active true
when
$p : Person($addresses : addresses)
exists (Address(state == "NC") from $addresses)
then
modify ($p) {} // Assign person to sales region 1 in a modify block
end
rule "Apply a discount to people in the city of Raleigh"
ruleflow-group "test"
lock-on-active true
when
$p : Person($addresses : addresses)
exists (Address(city == "Raleigh") from $addresses)
then
modify ($p) {} // Apply discount to person in a modify block
end
However, the following slightly different approach does exhibit the problem:
rule "Assign people in North Carolina (NC) to sales region 1"
ruleflow-group "test"
lock-on-active true
when
$assessment : Assessment()
$p : Person()
$addresses : List() from $p.addresses
exists (Address( state == "NC") from $addresses)
then
modify ($assessment) {} // Modify assessment in a modify block
end
rule "Apply a discount to people in the city of Raleigh"
ruleflow-group "test"
lock-on-active true
when
$assessment : Assessment()
$p : Person()
$addresses : List() from $p.addresses
exists (Address( city == "Raleigh") from $addresses)
then
modify ($assessment) {} // Modify assessment in a modify block
end
In the above example, the $addresses variable is returned from the use of from
.
The example also introduces a new object, assessment, to highlight one possible solution in this case.
If the $assessment variable assigned in the condition (LHS) is moved to the last condition in each rule, both rules fire as expected.
Though the above examples demonstrate how to combine the use of from
with lock-on-active
where no loss of rule activations occurs, they carry the drawback of placing a dependency on the order of conditions on the LHS.
In addition, the solutions present greater complexity for the rule author in terms of keeping track of which conditions may create issues.
A better alternative is to assert more facts into working memory.
In this case, a person’s addresses may be asserted into working memory and the use of from
would not be necessary.
There are cases, however, where asserting all data into working memory is not practical and we need to find other solutions.
Another option is to reevaluate the need for lock-on-active
.
An alternative to lock-on-active
is to directly manage how rules within the same rule-flow group activate one another by including conditions in each rule that prevent rules from activating each other recursively when working memory is modified.
For example, in the case above where a discount is applied to citizens of Raleigh, a condition may be added to the rule that checks whether the discount has already been applied.
If so, the rule does not activate.
The pattern containing a from clause cannot be followed by another pattern starting with a parenthesis as in the following example
This is because in that case the DRL parser reads the from expression as "from $l (String() or Number())" and it is impossible to disambiguate this expression from a function call. The straightforward fix to this is wrapping also the from clause in parenthesis as it follows:
|
Conditional Element collect
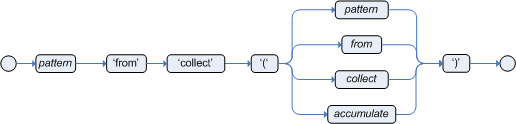
The Conditional Element collect
allows rules to reason over a collection of objects obtained from the given source or from the working memory.
In First Oder Logic terms this is the cardinality quantifier.
A simple example:
import java.util.ArrayList
rule "Raise priority if system has more than 3 pending alarms"
when
$system : System()
$alarms : ArrayList( size >= 3 )
from collect( Alarm( system == $system, status == 'pending' ) )
then
// Raise priority, because system $system has
// 3 or more alarms pending. The pending alarms
// are $alarms.
end
In the above example, the rule will look for all pending alarms in the working memory for each given system and group them in ArrayLists. If 3 or more alarms are found for a given system, the rule will fire.
The result pattern of collect
can be any concrete class that implements the java.util.Collection
interface and provides a default no-arg public constructor.
This means that you can use Java collections like ArrayList, LinkedList, HashSet, etc., or your own class, as long as it implements the java.util.Collection
interface and provide a default no-arg public constructor.
Both source and result patterns can be constrained as any other pattern.
Variables bound before the collect
CE are in the scope of both source and result patterns and therefore you can use them to constrain both your source and result patterns.
But note that collect
is a scope delimiter for bindings, so that any binding made inside of it is not available for use outside of it.
Collect accepts nested from
CEs.
The following example is a valid use of "collect":
import java.util.LinkedList;
rule "Send a message to all mothers"
when
$town : Town( name == 'Paris' )
$mothers : LinkedList()
from collect( Person( gender == 'F', children > 0 )
from $town.getPeople()
)
then
// send a message to all mothers
end
Conditional Element accumulate
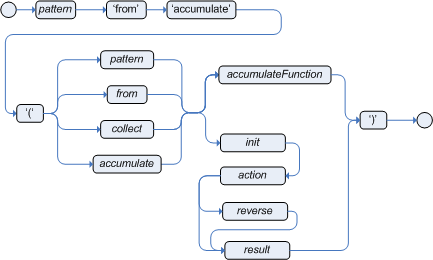
The Conditional Element accumulate
is a more flexible and powerful form of collect
, in the sense that it can be used to do what collect
does and also achieve results that the CE collect
is not capable of achieving.
Accumulate allows a rule to iterate over a collection of objects, executing custom actions for each of the elements, and at the end, it returns a result object.
Accumulate supports both the use of pre-defined accumulate functions, or the use of inline custom code. Inline custom code should be avoided though, as it is harder for rule authors to maintain, and frequently leads to code duplication. Accumulate functions are easier to test and reuse.
The Accumulate CE also supports multiple different syntaxes. The preferred syntax is the top level accumulate, as noted bellow, but all other syntaxes are supported for backward compatibility.
Accumulate CE (preferred syntax)
The top level accumulate syntax is the most compact and flexible syntax. The simplified syntax is as follows:
accumulate( <source pattern>; <functions> [;<constraints>] )
For instance, a rule to calculate the minimum, maximum and average temperature reading for a given sensor and that raises an alarm if the minimum temperature is under 20C degrees and the average is over 70C degrees could be written in the following way, using Accumulate:
The DRL language defines “`acc`” as a synonym of “`accumulate`”. The author might prefer to use “`acc`” as a less verbose keyword or the full keyword “`accumulate`” for legibility. |
rule "Raise alarm"
when
$s : Sensor()
accumulate( Reading( sensor == $s, $temp : temperature );
$min : min( $temp ),
$max : max( $temp ),
$avg : average( $temp );
$min < 20, $avg > 70 )
then
// raise the alarm
end
In the above example, min, max and average are Accumulate Functions and will calculate the minimum, maximum and average temperature values over all the readings for each sensor.
Drools ships with several built-in accumulate functions, including:
-
average
-
min
-
max
-
count
-
sum
-
variance
-
standardDeviation
-
collectList
-
collectSet
These common functions accept any expression as input. For instance, if someone wants to calculate the average profit on all items of an order, a rule could be written using the average function:
rule "Average profit"
when
$order : Order()
accumulate( OrderItem( order == $order, $cost : cost, $price : price );
$avgProfit : average( 1 - $cost / $price ) )
then
// average profit for $order is $avgProfit
end
Accumulate Functions are all pluggable.
That means that if needed, custom, domain specific functions can easily be added to the Drools engine and rules can start to use them without any restrictions.
To implement a new Accumulate Function all one needs to do is to create a Java class that implements the org.kie.api.runtime.rule.AccumulateFunction
interface.
As an example of an Accumulate Function implementation, the following is the implementation of the average
function:
/**
* An implementation of an accumulator capable of calculating average values
*/
public class AverageAccumulateFunction implements org.kie.api.runtime.rule.AccumulateFunction<AverageAccumulateFunction.AverageData> {
public void readExternal(ObjectInput in) throws IOException, ClassNotFoundException {
}
public void writeExternal(ObjectOutput out) throws IOException {
}
public static class AverageData implements Externalizable {
public int count = 0;
public double total = 0;
public AverageData() {}
public void readExternal(ObjectInput in) throws IOException, ClassNotFoundException {
count = in.readInt();
total = in.readDouble();
}
public void writeExternal(ObjectOutput out) throws IOException {
out.writeInt(count);
out.writeDouble(total);
}
}
/* (non-Javadoc)
* @see org.kie.api.runtime.rule.AccumulateFunction#createContext()
*/
public AverageData createContext() {
return new AverageData();
}
/* (non-Javadoc)
* @see org.kie.api.runtime.rule.AccumulateFunction#init(java.io.Serializable)
*/
public void init(AverageData context) {
context.count = 0;
context.total = 0;
}
/* (non-Javadoc)
* @see org.kie.api.runtime.rule.AccumulateFunction#accumulate(java.io.Serializable, java.lang.Object)
*/
public void accumulate(AverageData context,
Object value) {
context.count++;
context.total += ((Number) value).doubleValue();
}
/* (non-Javadoc)
* @see org.kie.api.runtime.rule.AccumulateFunction#reverse(java.io.Serializable, java.lang.Object)
*/
public void reverse(AverageData context, Object value) {
context.count--;
context.total -= ((Number) value).doubleValue();
}
/* (non-Javadoc)
* @see org.kie.api.runtime.rule.AccumulateFunction#getResult(java.io.Serializable)
*/
public Object getResult(AverageData context) {
return new Double( context.count == 0 ? 0 : context.total / context.count );
}
/* (non-Javadoc)
* @see org.kie.api.runtime.rule.AccumulateFunction#supportsReverse()
*/
public boolean supportsReverse() {
return true;
}
/* (non-Javadoc)
* @see org.kie.api.runtime.rule.AccumulateFunction#getResultType()
*/
public Class< ? > getResultType() {
return Number.class;
}
}
The code for the function is very simple, as we could expect, as all the "dirty" integration work is done by the Drools engine. Finally, to use the function in the rules, the author can import it using the "import accumulate" statement:
import accumulate <class_name> <function_name>
For instance, if one implements the class some.package.VarianceFunction
function that implements the variance
function and wants to use it in the rules, he would do the following:
import accumulate some.package.VarianceFunction variance
rule "Calculate Variance"
when
accumulate( Test( $s : score ), $v : variance( $s ) )
then
// the variance of the test scores is $v
end
The built in functions (sum, average, etc) are imported automatically by the Drools engine. Only user-defined custom accumulate functions need to be explicitly imported. |
For backward compatibility, Drools still supports the configuration of accumulate functions through configuration files and system properties, but this is a deprecated method. In order to configure the variance function from the previous example using the configuration file or system property, the user would set a property like this:
Please note that " |
Alternate Syntax: single function with return type
The accumulate syntax evolved over time with the goal of becoming more compact and expressive. Nevertheless, Drools still supports previous syntaxes for backward compatibility purposes.
In case the rule is using a single accumulate function on a given accumulate, the author may add a pattern for the result object and use the "from" keyword to link it to the accumulate result. Example: a rule to apply a 10% discount on orders over $100 could be written in the following way:
rule "Apply 10% discount to orders over US$ 100,00"
when
$order : Order()
$total : Number( doubleValue > 100 )
from accumulate( OrderItem( order == $order, $value : value ),
sum( $value ) )
then
// apply discount to $order
end
In the above example, the accumulate element is using only one function (sum), and so, the rules author opted to explicitly write a pattern for the result type of the accumulate function (Number) and write the constraints inside it. There are no problems in using this syntax over the compact syntax presented before, except that is is a bit more verbose. Also note that it is not allowed to use both the return type and the functions binding in the same accumulate statement.
Compile-time checks are performed in order to ensure the pattern used with the "from
" keyword is assignable from the result of the accumulate function used.
With this syntax, the " |
In the above example, "$total
" is bound to the result returned by the accumulate sum() function.
As another example however, if the result of the accumulate function is a collection, "from
" still binds to the single result and it does not iterate:
rule "Person names"
when
$x : Object() from accumulate(MyPerson( $val : name );
collectList( $val ) )
then
// $x is a List
end
The binded "$x : Object()
" is the List itself, returned by the collectList accumulate function used.
This is an important distinction to highlight, as the "from
" keyword can also be used separately of accumulate, to iterate over the elements of a collection:
rule "Iterate the numbers"
when
$xs : List()
$x : Integer() from $xs
then
// $x matches and binds to each Integer in the collection
end
While this syntax is still supported for backward compatibility purposes, for this and other reasons we encourage rule authors to make use instead of the Accumulate CE preferred syntax (described in the previous chapter), so to avoid any potential pitfalls, as described by these examples.
Accumulate with inline custom code
The use of accumulate with inline custom code is not a good practice for several reasons, including difficulties on maintaining and testing rules that use them, as well as the inability of reusing that code. Implementing your own accumulate functions is very simple and straightforward, they are easy to unit test and to use. This form of accumulate is supported for backward compatibility only. |
Another possible syntax for the accumulate is to define inline custom code, instead of using accumulate functions. As noted on the previous warned, this is discouraged though for the stated reasons.
The general syntax of the accumulate
CE with inline custom code is:
<result pattern> from accumulate( <source pattern>,
init( <init code> ),
action( <action code> ),
reverse( <reverse code> ),
result( <result expression> ) )
The meaning of each of the elements is the following:
-
<source pattern>: the source pattern is a regular pattern that the Drools engine will try to match against each of the source objects.
-
<init code>: this is a semantic block of code in the selected dialect that will be executed once for each tuple, before iterating over the source objects.
-
<action code>: this is a semantic block of code in the selected dialect that will be executed for each of the source objects.
-
<reverse code>: this is an optional semantic block of code in the selected dialect that if present will be executed for each source object that no longer matches the source pattern. The objective of this code block is to undo any calculation done in the <action code> block, so that the Drools engine can do decremental calculation when a source object is modified or deleted, hugely improving performance of these operations.
-
<result expression>: this is a semantic expression in the selected dialect that is executed after all source objects are iterated.
-
<result pattern>: this is a regular pattern that the Drools engine tries to match against the object returned from the <result expression>. If it matches, the
accumulate
conditional element evaluates to true and the Drools engine proceeds with the evaluation of the next CE in the rule. If it does not matches, theaccumulate
CE evaluates to false and the Drools engine stops evaluating CEs for that rule.
It is easier to understand if we look at an example:
rule "Apply 10% discount to orders over US$ 100,00"
when
$order : Order()
$total : Number( doubleValue > 100 )
from accumulate( OrderItem( order == $order, $value : value ),
init( double total = 0; ),
action( total += $value; ),
reverse( total -= $value; ),
result( total ) )
then
// apply discount to $order
end
In the above example, for each Order
in the Working Memory, the Drools engine will execute the init
code initializing the total variable to zero.
Then it will iterate over all OrderItem
objects for that order, executing the action for each one (in the example, it will sum the value of all items into the total variable). After iterating over all OrderItem
objects, it will return the value corresponding to the result
expression (in the above example, the value of variable total
). Finally, the Drools engine will try to match the result with the Number
pattern, and if the double value is greater than 100, the rule will fire.
The example used Java as the semantic dialect, and as such, note that the usage of the semicolon as statement delimiter is mandatory in the init, action and reverse code blocks. The result is an expression and, as such, it does not admit ';'. If the user uses any other dialect, he must comply to that dialect’s specific syntax.
As mentioned before, the reverse code is optional, but it is strongly recommended that the user writes it in order to benefit from the improved performance on update and delete.
The accumulate
CE can be used to execute any action on source objects.
The following example instantiates and populates a custom object:
rule "Accumulate using custom objects"
when
$person : Person( $likes : likes )
$cheesery : Cheesery( totalAmount > 100 )
from accumulate( $cheese : Cheese( type == $likes ),
init( Cheesery cheesery = new Cheesery(); ),
action( cheesery.addCheese( $cheese ); ),
reverse( cheesery.removeCheese( $cheese ); ),
result( cheesery ) );
then
// do something
end
6.8.3.8. Conditional Element eval

The conditional element eval
is essentially a catch-all which allows any semantic code (that returns a primitive boolean) to be executed.
This code can refer to variables that were bound in the LHS of the rule, and functions in the rule package.
Overuse of eval reduces the declarativeness of your rules and can result in a poorly performing engine.
While eval
can be used anywhere in the patterns, the best practice is to add it as the last conditional element in the LHS of a rule.
Evals cannot be indexed and thus are not as efficient as Field Constraints. However this makes them ideal for being used when functions return values that change over time, which is not allowed within Field Constraints.
For folks who are familiar with Drools 2.x lineage, the old Drools parameter and condition tags are equivalent to binding a variable to an appropriate type, and then using it in an eval node.
p1 : Parameter()
p2 : Parameter()
eval( p1.getList().containsKey( p2.getItem() ) )
p1 : Parameter()
p2 : Parameter()
// call function isValid in the LHS
eval( isValid( p1, p2 ) )
6.8.4. The Right Hand Side (then)
6.8.4.1. Usage
The Right Hand Side (RHS) is a common name for the consequence or action part of the rule; this part should contain a list of actions to be executed. It is bad practice to use imperative or conditional code in the RHS of a rule; as a rule should be atomic in nature - "when this, then do this", not "when this, maybe do this". The RHS part of a rule should also be kept small, thus keeping it declarative and readable. If you find you need imperative and/or conditional code in the RHS, then maybe you should be breaking that rule down into multiple rules. The main purpose of the RHS is to insert, delete or modify working memory data. To assist with that there are a few convenience methods you can use to modify working memory; without having to first reference a working memory instance.
update(
object,
handle);
will tell the Drools engine that an object has changed (one that has been bound to something on the LHS) and rules may need to be reconsidered.
update(
object);
can also be used; here the Knowledge Helper will look up the facthandle for you, via an identity check, for the passed object.
(Note that if you provide Property Change Listeners to your Java beans that you are inserting into the Drools engine, you can avoid the need to call update()
when the object changes.). After a fact’s field values have changed you must call update before changing another fact, or you will cause problems with the indexing within the Drools engine.
The modify keyword avoids this problem.
insert(new
Something());
will place a new object of your creation into the Working Memory.
insertLogical(new
Something());
is similar to insert, but the object will be automatically deleted when there are no more facts to support the truth of the currently firing rule.
delete(
handle);
removes an object from Working Memory.
These convenience methods are basically macros that provide short cuts to the KnowledgeHelper
instance that lets you access your Working Memory from rules files.
The predefined variable drools
of type KnowledgeHelper
lets you call several other useful methods.
(Refer to the KnowledgeHelper
interface documentation for more advanced operations).
-
The call
drools.halt()
terminates rule execution immediately. This is required for returning control to the point whence the current session was put to work withfireUntilHalt()
. -
Methods
insert(Object o)
,update(Object o)
anddelete(Object o)
can be called ondrools
as well, but due to their frequent use they can be called without the object reference. -
drools.getWorkingMemory()
returns theWorkingMemory
object. -
drools.setFocus( String s)
sets the focus to the specified agenda group. -
drools.getRule().getName()
, called from a rule’s RHS, returns the name of the rule. -
drools.getTuple()
returns the Tuple that matches the currently executing rule, anddrools.getActivation()
delivers the corresponding Activation. (These calls are useful for logging and debugging purposes.)
The full Knowledge Runtime API is exposed through another predefined variable, kcontext
, of type KieContext
.
Its method getKieRuntime()
delivers an object of type KieRuntime
, which, in turn, provides access to a wealth of methods, many of which are quite useful for coding RHS logic.
-
The call
kcontext.getKieRuntime().halt()
terminates rule execution immediately. -
The accessor
getAgenda()
returns a reference to this session’sAgenda
, which in turn provides access to the various rule groups: activation groups, agenda groups, and rule flow groups. A fairly common paradigm is the activation of some agenda group, which could be done with the lengthy call:// give focus to the agenda group CleanUp kcontext.getKieRuntime().getAgenda().getAgendaGroup( "CleanUp" ).setFocus();
(You can achieve the same using
drools.setFocus( "CleanUp" )
.) -
To run a query, you call
getQueryResults(String query)
, whereupon you may process the results, as explained in section Query. Usingkcontext.getKieRuntime().getQueryResults()
or usingdrools.getKieRuntime().getQueryResults()
is the proper method of running a query from a rule’s RHS, and the only supported way. -
A set of methods dealing with event management lets you, among other things, add and remove event listeners for the Working Memory and the Agenda.
-
Method
getKieBase()
returns theKieBase
object, the backbone of all the Knowledge in your system, and the originator of the current session. -
You can manage globals with
setGlobal(…)
,getGlobal(…)
andgetGlobals()
. -
Method
getEnvironment()
returns the runtime’sEnvironment
which works much like what you know as your operating system’s environment.
6.8.4.2. The modify
Statement
This language extension provides a structured approach to fact updates.
It combines the update operation with a number of setter calls to change the object’s fields.
This is the syntax schema for the modify
statement:
modify ( <fact-expression> ) {
<expression> [ , <expression> ]*
}
The parenthesized <fact-expression> must yield a fact object reference. The expression list in the block should consist of setter calls for the given object, to be written without the usual object reference, which is automatically prepended by the compiler.
The example illustrates a simple fact modification.
rule "modify stilton"
when
$stilton : Cheese(type == "stilton")
then
modify( $stilton ){
setPrice( 20 ),
setAge( "overripe" )
}
end
The advantages in using the modify statment are particularly clear when used in conjuction with fine grained property change listeners. See the corresponding section for more details.
6.8.5. Conditional named consequences
Sometimes the constraint of having one single consequence for each rule can be somewhat limiting and leads to verbose and difficult to be maintained repetitions like in the following example:
rule "Give 10% discount to customers older than 60"
when
$customer : Customer( age > 60 )
then
modify($customer) { setDiscount( 0.1 ) };
end
rule "Give free parking to customers older than 60"
when
$customer : Customer( age > 60 )
$car : Car ( owner == $customer )
then
modify($car) { setFreeParking( true ) };
end
It is already possible to partially overcome this problem by making the second rule extending the first one like in:
rule "Give 10% discount to customers older than 60"
when
$customer : Customer( age > 60 )
then
modify($customer) { setDiscount( 0.1 ) };
end
rule "Give free parking to customers older than 60"
extends "Give 10% discount to customers older than 60"
when
$car : Car ( owner == $customer )
then
modify($car) { setFreeParking( true ) };
end
Anyway this feature makes it possible to define more labelled consequences other than the default one in a single rule, so, for example, the 2 former rules can be compacted in only one like it follows:
rule "Give 10% discount and free parking to customers older than 60"
when
$customer : Customer( age > 60 )
do[giveDiscount]
$car : Car ( owner == $customer )
then
modify($car) { setFreeParking( true ) };
then[giveDiscount]
modify($customer) { setDiscount( 0.1 ) };
end
This last rule has 2 consequences, the usual default one, plus another one named "giveDiscount" that is activated, using the keyword do, as soon as a customer older than 60 is found in the KIE base, regardless of the fact that he owns a car or not. The activation of a named consequence can be also guarded by an additional condition like in this further example:
rule "Give free parking to customers older than 60 and 10% discount to golden ones among them"
when
$customer : Customer( age > 60 )
if ( type == "Golden" ) do[giveDiscount]
$car : Car ( owner == $customer )
then
modify($car) { setFreeParking( true ) };
then[giveDiscount]
modify($customer) { setDiscount( 0.1 ) };
end
The condition in the if statement is always evaluated on the pattern immediately preceding it. In the end this last, a bit more complicated, example shows how it is possible to switch over different conditions using a nested if/else statement:
rule "Give free parking and 10% discount to over 60 Golden customer and 5% to Silver ones"
when
$customer : Customer( age > 60 )
if ( type == "Golden" ) do[giveDiscount10]
else if ( type == "Silver" ) break[giveDiscount5]
$car : Car ( owner == $customer )
then
modify($car) { setFreeParking( true ) };
then[giveDiscount10]
modify($customer) { setDiscount( 0.1 ) };
then[giveDiscount5]
modify($customer) { setDiscount( 0.05 ) };
end
Here the purpose is to give a 10% discount AND a free parking to Golden customers over 60, but only a 5% discount (without free parking) to the Silver ones. This result is achieved by activating the consequence named "giveDiscount5" using the keyword break instead of do. In fact do just schedules a consequence in the agenda, allowing the remaining part of the LHS to continue of being evaluated as per normal, while break also blocks any further pattern matching evaluation. Note, of course, that the activation of a named consequence not guarded by any condition with break doesn’t make sense (and generates a compile time error) since otherwise the LHS part following it would be never reachable.
6.8.6. A Note on Auto-boxing and Primitive Types
Drools attempts to preserve numbers in their primitive or object wrapper form, so a variable bound to an int primitive when used in a code block or expression will no longer need manual unboxing; unlike Drools 3.0 where all primitives were autoboxed, requiring manual unboxing. A variable bound to an object wrapper will remain as an object; the existing JDK 1.5 and JDK 5 rules to handle auto-boxing and unboxing apply in this case. When evaluating field constraints, the system attempts to coerce one of the values into a comparable format; so a primitive is comparable to an object wrapper.
6.9. Query
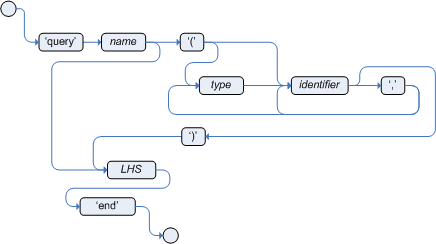
A query is a simple way to search the working memory for facts that match the stated conditions. Therefore, it contains only the structure of the LHS of a rule, so that you specify neither "when" nor "then". A query has an optional set of parameters, each of which can be optionally typed. If the type is not given, the type Object is assumed. The Drools engine will attempt to coerce the values as needed. Query names are global to the KieBase; so do not add queries of the same name to different packages for the same RuleBase.
To return the results use ksession.getQueryResults("name")
, where "name" is the query’s name.
This returns a list of query results, which allow you to retrieve the objects that matched the query.
The first example presents a simple query for all the people over the age of 30. The second one, using parameters, combines the age limit with a location.
query "people over the age of 30"
person : Person( age > 30 )
end
query "people over the age of x" (int x, String y)
person : Person( age > x, location == y )
end
We iterate over the returned QueryResults using a standard "for" loop. Each element is a QueryResultsRow which we can use to access each of the columns in the tuple. These columns can be accessed by bound declaration name or index position.
QueryResults results = ksession.getQueryResults( "people over the age of 30" );
System.out.println( "we have " + results.size() + " people over the age of 30" );
System.out.println( "These people are are over 30:" );
for ( QueryResultsRow row : results ) {
Person person = ( Person ) row.get( "person" );
System.out.println( person.getName() + "\n" );
}
Support for positional syntax has been added for more compact code. By default the declared type order in the type declaration matches the argument position. But it possible to override these using the @position annotation. This allows patterns to be used with positional arguments, instead of the more verbose named arguments.
declare Cheese
name : String @position(1)
shop : String @position(2)
price : int @position(0)
end
The @Position annotation, in the org.drools.definition.type package, can be used to annotate original pojos on the classpath.
Currently only fields on classes can be annotated.
Inheritance of classes is supported, but not interfaces or methods.
The isContainedIn query below demonstrates the use of positional arguments in a pattern; Location(x, y;)
instead of Location( thing == x, location == y).
Queries can now call other queries, this combined with optional query arguments provides derivation query style backward chaining. Positional and named syntax is supported for arguments. It is also possible to mix both positional and named, but positional must come first, separated by a semi colon. Literal expressions can be passed as query arguments, but at this stage you cannot mix expressions with variables. Here is an example of a query that calls another query. Note that 'z' here will always be an 'out' variable. The '?' symbol means the query is pull only, once the results are returned you will not receive further results as the underlying data changes.
declare Location
thing : String
location : String
end
query isContainedIn( String x, String y )
Location(x, y;)
or
( Location(z, y;) and ?isContainedIn(x, z;) )
end
As previously mentioned you can use live "open" queries to reactively receive changes over time from the query results, as the underlying data it queries against changes. Notice the "look" rule calls the query without using '?'.
query isContainedIn( String x, String y )
Location(x, y;)
or
( Location(z, y;) and isContainedIn(x, z;) )
end
rule look when
Person( $l : likes )
isContainedIn( $l, 'office'; )
then
insertLogical( $l 'is in the office' );
end
Drools supports unification for derivation queries, in short this means that arguments are optional. It is possible to call queries from Java leaving arguments unspecified using the static field org.drools.core.runtime.rule.Variable.v - note you must use 'v' and not an alternative instance of Variable. These are referred to as 'out' arguments. Note that the query itself does not declare at compile time whether an argument is in or an out, this can be defined purely at runtime on each use. The following example will return all objects contained in the office.
results = ksession.getQueryResults( "isContainedIn", new Object[] { Variable.v, "office" } );
l = new ArrayList<List<String>>();
for ( QueryResultsRow r : results ) {
l.add( Arrays.asList( new String[] { (String) r.get( "x" ), (String) r.get( "y" ) } ) );
}
The algorithm uses stacks to handle recursion, so the method stack will not blow up.
It is also possible to use as input argument for a query both the field of a fact as in:
query contains(String $s, String $c)
$s := String( this.contains( $c ) )
end
rule PersonNamesWithA when
$p : Person()
contains( $p.name, "a"; )
then
end
and more in general any kind of valid expression like in:
query checkLength(String $s, int $l)
$s := String( length == $l )
end
rule CheckPersonNameLength when
$i : Integer()
$p : Person()
checkLength( $p.name, 1 + $i + $p.age; )
then
end
The following is not yet supported:
-
List and Map unification
-
Expression unification - pred( X, X + 1, X * Y / 7 )
6.10. Domain Specific Languages
Domain Specific Languages (or DSLs) are a way of creating a rule language that is dedicated to your problem domain. A set of DSL definitions consists of transformations from DSL "sentences" to DRL constructs, which lets you use of all the underlying rule language and engine features. Given a DSL, you write rules in DSL rule (or DSLR) files, which will be translated into DRL files.
DSL and DSLR files are plain text files, and you can use any text editor to create and modify them. But there are also DSL and DSLR editors, both in the IDE as well as in the web based BRMS, and you can use those as well, although they may not provide you with the full DSL functionality.
6.10.1. When to Use a DSL
DSLs can serve as a layer of separation between rule authoring (and rule authors) and the technical intricacies resulting from the modelling of domain object and the Drools engine’s native language and methods. If your rules need to be read and validated by domain experts (such as business analysts, for instance) who are not programmers, you should consider using a DSL; it hides implementation details and focuses on the rule logic proper. DSL sentences can also act as "templates" for conditional elements and consequence actions that are used repeatedly in your rules, possibly with minor variations. You may define DSL sentences as being mapped to these repeated phrases, with parameters providing a means for accommodating those variations.
DSLs have no impact on the Drools engine at runtime, they are just a compile time feature, requiring a special parser and transformer.
6.10.2. DSL Basics
The Drools DSL mechanism allows you to customise conditional expressions and consequence actions. A global substitution mechanism ("keyword") is also available.
[when]Something is {colour}=Something(colour=="{colour}")
In the preceding example, [when]
indicates the scope of the expression, i.e., whether it is valid for the LHS or the RHS of a rule.
The part after the bracketed keyword is the expression that you use in the rule; typically a natural language expression, but it doesn’t have to be.
The part to the right of the equal sign ("=") is the mapping of the expression into the rule language.
The form of this string depends on its destination, RHS or LHS.
If it is for the LHS, then it ought to be a term according to the regular LHS syntax; if it is for the RHS then it might be a Java statement.
Whenever the DSL parser matches a line from the rule file written in the DSL with an expression in the DSL definition, it performs three steps of string manipulation.
First, it extracts the string values appearing where the expression contains variable names in braces (here: {colour}
). Then, the values obtained from these captures are then interpolated wherever that name, again enclosed in braces, occurs on the right hand side of the mapping.
Finally, the interpolated string replaces whatever was matched by the entire expression in the line of the DSL rule file.
Note that the expressions (i.e., the strings on the left hand side of the equal sign) are used as regular expressions in a pattern matching operation against a line of the DSL rule file, matching all or part of a line. This means you can use (for instance) a '?' to indicate that the preceding character is optional. One good reason to use this is to overcome variations in natural language phrases of your DSL. But, given that these expressions are regular expression patterns, this also means that all "magic" characters of Java’s pattern syntax have to be escaped with a preceding backslash ('\').
It is important to note that the compiler transforms DSL rule files line by line. In the above example, all the text after "Something is " to the end of the line is captured as the replacement value for "{colour}", and this is used for interpolating the target string. This may not be exactly what you want. For instance, when you intend to merge different DSL expressions to generate a composite DRL pattern, you need to transform a DSLR line in several independent operations. The best way to achieve this is to ensure that the captures are surrounded by characteristic text - words or even single characters. As a result, the matching operation done by the parser plucks out a substring from somewhere within the line. In the example below, quotes are used as distinctive characters. Note that the characters that surround the capture are not included during interpolation, just the contents between them.
As a rule of thumb, use quotes for textual data that a rule editor may want to enter.
You can also enclose the capture with words to ensure that the text is correctly matched.
Both is illustrated by the following example.
Note that a single line such as Something is "green" and
another solid thing
is now correctly expanded.
[when]something is "{colour}"=Something(colour=="{colour}")
[when]another {state} thing=OtherThing(state=="{state})"
It is a good idea to avoid punctuation (other than quotes or apostrophes) in your DSL expressions as much as possible. The main reason is that punctuation is easy to forget for rule authors using your DSL. Another reason is that parentheses, the period and the question mark are magic characters, requiring escaping in the DSL definition.
In a DSL mapping, the braces "{" and "}" should only be used to enclose a variable definition or reference, resulting in a capture. If they should occur literally, either in the expression or within the replacement text on the right hand side, they must be escaped with a preceding backslash ("\"):
[then]do something= if (foo) \{ doSomething(); \}
If braces "{" and "}" should appear in the replacement string of a DSL definition, escape them with a backslash ('\'). |
# This is a comment to be ignored.
[when]There is a person with name of "{name}"=Person(name=="{name}")
[when]Person is at least {age} years old and lives in "{location}"=
Person(age >= {age}, location=="{location}")
[then]Log "{message}"=System.out.println("{message}");
[when]And = and
Given the above DSL examples, the following examples show the expansion of various DSLR snippets:
There is a person with name of "Kitty"
==> Person(name="Kitty")
Person is at least 42 years old and lives in "Atlanta"
==> Person(age >= 42, location="Atlanta")
Log "boo"
==> System.out.println("boo");
There is a person with name of "Bob" And Person is at least 30 years old and lives in "Utah"
==> Person(name="Bob") and Person(age >= 30, location="Utah")
Don’t forget that if you are capturing plain text from a DSL rule line and want to use it as a string literal in the expansion, you must provide the quotes on the right hand side of the mapping. |
You can chain DSL expressions together on one line, as long as it is clear to the parser where one ends and the next one begins and where the text representing a parameter ends. (Otherwise you risk getting all the text until the end of the line as a parameter value.) The DSL expressions are tried, one after the other, according to their order in the DSL definition file. After any match, all remaining DSL expressions are investigated, too.
The resulting DRL text may consist of more than one line.
Line ends are in the replacement text are written as \n
.
6.10.3. Adding Constraints to Facts
A common requirement when writing rule conditions is to be able to add an arbitrary combination of constraints to a pattern. Given that a fact type may have many fields, having to provide an individual DSL statement for each combination would be plain folly.
The DSL facility allows you to add constraints to a pattern by a simple convention: if your DSL expression starts with a hyphen (minus character, "-") it is assumed to be a field constraint and, consequently, is is added to the last pattern line preceding it.
For an example, lets take look at class Cheese
, with the following fields: type, price, age and country.
We can express some LHS condition in normal DRL like the following
Cheese(age < 5, price == 20, type=="stilton", country=="ch")
The DSL definitions given below result in three DSL phrases which may be used to create any combination of constraint involving these fields.
[when]There is a Cheese with=Cheese()
[when]- age is less than {age}=age<{age}
[when]- type is '{type}'=type=='{type}'
[when]- country equal to '{country}'=country=='{country}'
You can then write rules with conditions like the following:
There is a Cheese with
- age is less than 42
- type is 'stilton'
The parser will pick up a line beginning with "-" and add it as a constraint to the preceding pattern, inserting a comma when it is required. For the preceding example, the resulting DRL is:
Cheese(age<42, type=='stilton')
Combining all numeric fields with all relational operators (according to the DSL expression "age is less than…" in the preceding example) produces an unwieldy amount of DSL entries. But you can define DSL phrases for the various operators and even a generic expression that handles any field constraint, as shown below. (Notice that the expression definition contains a regular expression in addition to the variable name.)
[when][]is less than or equal to=<=
[when][]is less than=<
[when][]is greater than or equal to=>=
[when][]is greater than=>
[when][]is equal to===
[when][]equals===
[when][]There is a Cheese with=Cheese()
[when][]- {field:\w*} {operator} {value:\d*}={field} {operator} {value}
Given these DSL definitions, you can write rules with conditions such as:
There is a Cheese with
- age is less than 42
- rating is greater than 50
- type equals 'stilton'
In this specific case, a phrase such as "is less than" is replaced by <
, and then the line matches the last DSL entry.
This removes the hyphen, but the final result is still added as a constraint to the preceding pattern.
After processing all of the lines, the resulting DRL text is:
Cheese(age<42, rating > 50, type=='stilton')
The order of the entries in the DSL is important if separate DSL expressions are intended to match the same line, one after the other. |
6.10.4. Developing a DSL
A good way to get started is to write representative samples of the rules your application requires, and to test them as you develop. This will provide you with a stable framework of conditional elements and their constraints. Rules, both in DRL and in DSLR, refer to entities according to the data model representing the application data that should be subject to the reasoning process defined in rules. Notice that writing rules is generally easier if most of the data model’s types are facts.
Given an initial set of rules, it should be possible to identify recurring or similar code snippets and to mark variable parts as parameters. This provides reliable leads as to what might be a handy DSL entry. Also, make sure you have a full grasp of the jargon the domain experts are using, and base your DSL phrases on this vocabulary.
You may postpone implementation decisions concerning conditions and actions during this first design phase by leaving certain conditional elements and actions in their DRL form by prefixing a line with a greater sign (">"). (This is also handy for inserting debugging statements.)
During the next development phase, you should find that the DSL configuration stabilizes pretty quickly. New rules can be written by reusing the existing DSL definitions, or by adding a parameter to an existing condition or consequence entry.
Try to keep the number of DSL entries small. Using parameters lets you apply the same DSL sentence for similar rule patterns or constraints. But do not exaggerate: authors using the DSL should still be able to identify DSL phrases by some fixed text.
6.10.5. DSL and DSLR Reference
A DSL file is a text file in a line-oriented format. Its entries are used for transforming a DSLR file into a file according to DRL syntax.
-
A line starting with "" or "//" (with or without preceding white space) is treated as a comment. A comment line starting with "/" is scanned for words requesting a debug option, see below.
-
Any line starting with an opening bracket ("[") is assumed to be the first line of a DSL entry definition.
-
Any other line is appended to the preceding DSL entry definition, with the line end replaced by a space.
A DSL entry consists of the following four parts:
-
A scope definition, written as one of the keywords "when" or "condition", "then" or "consequence", "*" and "keyword", enclosed in brackets ("[" and "]"). This indicates whether the DSL entry is valid for the condition or the consequence of a rule, or both. A scope indication of "keyword" means that the entry has global significance, i.e., it is recognized anywhere in a DSLR file.
-
A type definition, written as a Java class name, enclosed in brackets. This part is optional unless the next part begins with an opening bracket. An empty pair of brackets is valid, too.
-
A DSL expression consists of a (Java) regular expression, with any number of embedded variable definitions, terminated by an equal sign ("="). A variable definition is enclosed in braces ("{" and "}"). It consists of a variable name and two optional attachments, separated by colons (":"). If there is one attachment, it is a regular expression for matching text that is to be assigned to the variable; if there are two attachments, the first one is a hint for the GUI editor and the second one the regular expression.
Note that all characters that are "magic" in regular expressions must be escaped with a preceding backslash ("\") if they should occur literally within the expression.
-
The remaining part of the line after the delimiting equal sign is the replacement text for any DSLR text matching the regular expression. It may contain variable references, i.e., a variable name enclosed in braces. Optionally, the variable name may be followed by an exclamation mark ("!") and a transformation function, see below.
Note that braces ("{" and "}") must be escaped with a preceding backslash ("\") if they should occur literally within the replacement string.
Debugging of DSL expansion can be turned on, selectively, by using a comment line starting with "#/" which may contain one or more words from the table presented below. The resulting output is written to standard output.
Word | Description |
---|---|
result |
Prints the resulting DRL text, with line numbers. |
steps |
Prints each expansion step of condition and consequence lines. |
keyword |
Dumps the internal representation of all DSL entries with scope "keyword". |
when |
Dumps the internal representation of all DSL entries with scope "when" or "*". |
then |
Dumps the internal representation of all DSL entries with scope "then" or "*". |
usage |
Displays a usage statistic of all DSL entries. |
Below are some sample DSL definitions, with comments describing the language features they illustrate.
# Comment: DSL examples
#/ debug: display result and usage
# keyword definition: replaces "regula" by "rule"
[keyword][]regula=rule
# conditional element: "T" or "t", "a" or "an", convert matched word
[when][][Tt]here is an? {entity:\w+}=
${entity!lc}: {entity!ucfirst} ()
# consequence statement: convert matched word, literal braces
[then][]update {entity:\w+}=modify( ${entity!lc} )\{ \}
The transformation of a DSLR file proceeds as follows:
-
The text is read into memory.
-
Each of the "keyword" entries is applied to the entire text. First, the regular expression from the keyword definition is modified by replacing white space sequences with a pattern matching any number of white space characters, and by replacing variable definitions with a capture made from the regular expression provided with the definition, or with the default (".*?"). Then, the DSLR text is searched exhaustively for occurrences of strings matching the modified regular expression. Substrings of a matching string corresponding to variable captures are extracted and replace variable references in the corresponding replacement text, and this text replaces the matching string in the DSLR text.
-
Sections of the DSLR text between "when" and "then", and "then" and "end", respectively, are located and processed in a uniform manner, line by line, as described below.
For a line, each DSL entry pertaining to the line’s section is taken in turn, in the order it appears in the DSL file. Its regular expression part is modified: white space is replaced by a pattern matching any number of white space characters; variable definitions with a regular expression are replaced by a capture with this regular expression, its default being ".*?". If the resulting regular expression matches all or part of the line, the matched part is replaced by the suitably modified replacement text.
Modification of the replacement text is done by replacing variable references with the text corresponding to the regular expression capture. This text may be modified according to the string transformation function given in the variable reference; see below for details.
If there is a variable reference naming a variable that is not defined in the same entry, the expander substitutes a value bound to a variable of that name, provided it was defined in one of the preceding lines of the current rule.
-
If a DSLR line in a condition is written with a leading hyphen, the expanded result is inserted into the last line, which should contain a pattern CE, i.e., a type name followed by a pair of parentheses. if this pair is empty, the expanded line (which should contain a valid constraint) is simply inserted, otherwise a comma (",") is inserted beforehand.
If a DSLR line in a consequence is written with a leading hyphen, the expanded result is inserted into the last line, which should contain a "modify" statement, ending in a pair of braces ("{" and "}"). If this pair is empty, the expanded line (which should contain a valid method call) is simply inserted, otherwise a comma (",") is inserted beforehand.
It is currently not possible to use a line with a leading hyphen to insert text into other conditional element forms (e.g., "accumulate") or it may only work for the first insertion (e.g., "eval"). |
All string transformation functions are described in the following table.
Name | Description |
---|---|
uc |
Converts all letters to upper case. |
lc |
Converts all letters to lower case. |
ucfirst |
Converts the first letter to upper case, and all other letters to lower case. |
num |
Extracts all digits and "-" from the string. If the last two digits in the original string are preceded by "." or ",", a decimal period is inserted in the corresponding position. |
a?b/c |
Compares the string with string a, and if they are equal, replaces it with b, otherwise with c. But c can be another triplet a, b, c, so that the entire structure is, in fact, a translation table. |
The following DSL examples show how to use string transformation functions.
# definitions for conditions
[when][]There is an? {entity}=${entity!lc}: {entity!ucfirst}()
[when][]- with an? {attr} greater than {amount}={attr} <= {amount!num}
[when][]- with a {what} {attr}={attr} {what!positive?>0/negative?%lt;0/zero?==0/ERROR}
A file containing a DSL definition has to be put under the resources folder or any of its subfolders like any other drools artifact.
It must have the extension .dsl
, or alternatively be marked with type ResourceType.DSL
.
when programmatically added to a KieFileSystem
.
For a file using DSL definition, the extension .dslr
should be used, while it can be added to a KieFileSystem
with type ResourceType.DSLR
.
For parsing and expanding a DSLR file the DSL configuration is read and supplied to the parser. Thus, the parser can "recognize" the DSL expressions and transform them into native rule language expressions.
7. Complex Event Processing
7.1. Complex Event Processing
There is no broadly accepted definition on the term Complex Event Processing. The term Event by itself is frequently overloaded and used to refer to several different things, depending on the context it is used. Defining terms is not the goal of this guide and as so, lets adopt a loose definition that, although not formal, will allow us to proceed with a common understanding.
So, in the scope of this guide:
Event, is a record of a significant change of state in the application domain at a given point in time. |
For instance, on a Stock Broker application, when a sale operation is executed, it causes a change of state in the domain. This change of state can be observed on several entities in the domain, like the price of the securities that changed to match the value of the operation, the ownership of the traded assets that changed from the seller to the buyer, the balance of the accounts from both seller and buyer that are credited and debited, etc. Depending on how the domain is modelled, this change of state may be represented by a single event, multiple atomic events or even hierarchies of correlated events. In any case, in the context of this guide, Event is the record of the change of a particular piece of data in the domain.
Events are processed by computer systems since they were invented, and throughout the history, systems responsible for that were given different names and different methodologies were employed. It wasn’t until the 90’s though, that a more focused work started on EDA (Event Driven Architecture) with a more formal definition on the requirements and goals for event processing. Old messaging systems started to change to address such requirements and new systems started to be developed with the single purpose of event processing. Two trends were born under the names of Event Stream Processing and Complex Event Processing.
In the very beginnings, Event Stream Processing was focused on the capabilities of processing streams of events in (near) real time, while the main focus of Complex Event Processing was on the correlation and composition of atomic events into complex (compound) events. An important (maybe the most important) milestone was the publishing of Dr. David Luckham’s book "The Power of Events" in 2002. In the book, Dr Luckham introduces the concept of Complex Event Processing and how it can be used to enhance systems that deal with events. Over the years, both trends converged to a common understanding and today these systems are all referred to as CEP systems.
This is a very simplistic explanation to a really complex and fertile field of research, but sets a high level and common understanding of the concepts that this guide will introduce.
The current understanding of what Complex Event Processing is may be briefly described as the following quote from Wikipedia:
— http://en.wikipedia.org/wiki/Complex_event_processing
|
In other words, CEP is about detecting and selecting the interesting events (and only them) from an event cloud, finding their relationships and inferring new data from them and their relationships.
For the remaining of this guide, we will use the terms Complex Event Processing and CEP as a broad reference for any of the related technologies and techniques, including but not limited to, CEP, Complex Event Processing, ESP, Event Stream Processing and Event Processing in general. |
7.2. Drools Fusion
Event Processing use cases, in general, share several requirements and goals with Business Rules use cases. These overlaps happen both on the business side and on the technical side.
On the Business side:
-
Business rules are frequently defined based on the occurrence of scenarios triggered by events. Examples could be:
-
On an algorithmic trading application: take an action if the security price increases X% compared to the day opening price, where the price increases are usually denoted by events on a Stock Trade application.
-
On a monitoring application: take an action if the temperature on the server room increases X degrees in Y minutes, where sensor readings are usually denoted by events.
-
-
Both business rules and event processing queries change frequently and require immediate response for the business to adapt itself to new market conditions, new regulations and new enterprise policies.
From a technical perspective:
-
Both require seamless integration with the enterprise infrastructure and applications, specially on autonomous governance, including, but not limited to, lifecycle management, auditing, security, etc.
-
Both have functional requirements like pattern matching and non-functional requirements like response time and query/rule explanation.
Even sharing requirements and goals, historically, both fields were born appart and although the industry evolved and one can find good products on the market, they either focus on event processing or on business rules management. That is due not only because of historical reasons but also because, even overlapping in part, use cases do have some different requirements.
Drools was also born as a rules engine several years ago, but following the vision of becoming a single platform for behavioral modelling, it soon realized that it could only achieve this goal by crediting the same importance to the three complementary business modelling techniques:
|
In this context, Drools Fusion is the module responsible for adding event processing capabilities into the platform.
Supporting Complex Event Processing, though, is much more than simply understanding what an event is. CEP scenarios share several common and distinguishing characteristics:
-
Usually required to process huge volumes of events, but only a small percentage of the events are of real interest.
-
Events are usually immutable, since they are a record of state change.
-
Usually the rules and queries on events must run in reactive modes, i.e., react to the detection of event patterns.
-
Usually there are strong temporal relationships between related events.
-
Individual events are usually not important. The system is concerned about patterns of related events and their relationships.
-
Usually, the system is required to perform composition and aggregation of events.
Based on this general common characteristics, Drools Fusion defined a set of goals to be achieved in order to support Complex Event Processing appropriately:
-
Support Events, with their proper semantics, as first class citizens.
-
Allow detection, correlation, aggregation and composition of events.
-
Support processing of Streams of events.
-
Support temporal constraints in order to model the temporal relationships between events.
-
Support sliding windows of interesting events.
-
Support a session scoped unified clock.
-
Support the required volumes of events for CEP use cases.
-
Support to (re)active rules.
-
Support adapters for event input into the Drools engine (pipeline).
The above list of goals are based on the requirements not covered by Drools Expert itself, since in a unified platform, all features of one module are leveraged by the other modules. This way, Drools Fusion is born with enterprise grade features like Pattern Matching, that is paramount to a CEP product, but that is already provided by Drools Expert. In the same way, all features provided by Drools Fusion are leveraged by Drools Flow (and vice-versa) making process management aware of event processing and vice-versa.
For the remaining of this guide, we will go through each of the features Drools Fusion adds to the platform. All these features are available to support different use cases in the CEP world, and the user is free to select and use the ones that will help him model his business use case.
7.3. Event Semantics
An event is a fact that present a few distinguishing characteristics:
-
Usually immutables: since, by the previously discussed definition, events are a record of a state change in the application domain, i.e., a record of something that already happened, and the past can not be "changed", events are immutables. This constraint is an important requirement for the development of several optimizations and for the specification of the event lifecycle. This does not mean that the Java object representing the object must be immutable. Quite the contrary, the Drools engine does not enforce immutability of the object model, because one of the most common use cases for rules is event data enrichment.
As a best practice, the application is allowed to populate un-populated event attributes (to enrich the event with inferred data), but already populated attributes should never be changed.
-
Strong temporal constraints: rules involving events usually require the correlation of multiple events, specially temporal correlations where events are said to happen at some point in time relative to other events.
-
Managed lifecycle: due to their immutable nature and the temporal constraints, events usually will only match other events and facts during a limited window of time, making it possible for the Drools engine to manage the lifecycle of the events automatically. In other words, one an event is inserted into the working memory, it is possible for the Drools engine to find out when an event can no longer match other facts and automatically delete it, releasing its associated resources.
-
Use of sliding windows: since all events have timestamps associated to them, it is possible to define and use sliding windows over them, allowing the creation of rules on aggregations of values over a period of time. Example: average of an event value over 60 minutes.
Drools supports the declaration and usage of events with both semantics: point-in-time events and interval-based events.
A simplistic way to understand the unitification of the semantics is to consider a point-in-time event as an interval-based event whose duration is zero. |
7.4. Event Processing Modes
Rules engines in general have a well known way of processing data and rules and provide the application with the results. Also, there is not many requirements on how facts should be presented to the rules engine, specially because in general, the processing itself is time independent. That is a good assumption for most scenarios, but not for all of them. When the requirements include the processing of real time or near real time events, time becomes and important variable of the reasoning process.
The following sections will explain the impact of time on rules reasoning and the two modes provided by Drools for the reasoning process.
7.4.1. Cloud Mode
The CLOUD processing mode is the default processing mode. Users of rules engines are familiar with this mode because it behaves in exactly the same way as any pure forward chaining rules engine, including previous versions of Drools.
When running in CLOUD mode, the Drools engine sees all facts in the working memory, does not matter if they are regular facts or events, as a whole. There is no notion of flow of time, although events have a timestamp as usual. In other words, although the Drools engine knows that a given event was created, for instance, on January 1st 2009, at 09:35:40.767, it is not possible for the Drools engine to determine how "old" the event is, because there is no concept of "now".
In this mode, the Drools engine will apply its usual many-to-many pattern matching algorithm, using the rules constraints to find the matching tuples, activate and fire rules as usual.
This mode does not impose any kind of additional requirements on facts. So for instance:
-
There is no notion of time. No requirements clock synchronization.
-
There is no requirement on event ordering. The Drools engine looks at the events as an unordered cloud against which the Drools engine tries to match rules.
On the other hand, since there is no requirements, some benefits are not available either. For instance, in CLOUD mode, it is not possible to use sliding windows, because sliding windows are based on the concept of "now" and there is no concept of "now" in CLOUD mode.
Since there is no ordering requirement on events, it is not possible for the Drools engine to determine when events can no longer match and as so, there is no automatic life-cycle management for events. I.e., the application must explicitly delete events when they are no longer necessary, in the same way the application does with regular facts.
Cloud mode is the default execution mode for Drools, but in any case, as any other configuration in Drools, it is possible to change this behavior either by setting a system property, using configuration property files or using the API. The corresponding property is:
KieBaseConfiguration config = KieServices.Factory.get().newKieBaseConfiguration();
config.setOption( EventProcessingOption.CLOUD );
The equivalent property is:
drools.eventProcessingMode = cloud
7.4.2. Stream Mode
The STREAM processing mode is the mode of choice when the application needs to process streams of events. It adds a few common requirements to the regular processing, but enables a whole lot of features that make stream event processing a lot simpler.
The main requirements to use STREAM mode are:
-
Events in each stream must be time-ordered. I.e., inside a given stream, events that happened first must be inserted first into the Drools engine.
-
The Drools engine will force synchronization between streams through the use of the session clock, so, although the application does not need to enforce time ordering between streams, the use of non-time-synchronized streams may result in some unexpected results.
Given that the above requirements are met, the application may enable the STREAM mode using the following API:
KieBaseConfiguration config = KieServices.Factory.get().newKieBaseConfiguration();
config.setOption( EventProcessingOption.STREAM );
Or, the equivalent property:
drools.eventProcessingMode = stream
When using the STREAM, the Drools engine knows the concept of flow of time and the concept of "now", i.e., the Drools engine understands how old events are based on the current timestamp read from the Session Clock. This characteristic allows the Drools engine to provide the following additional features to the application:
-
Sliding Window support
-
Automatic Event Lifecycle Management
-
Automatic Rule Delaying when using Negative Patterns
All these features are explained in the following sections.
7.4.2.1. Role of Session Clock in Stream mode
When running the Drools engine in CLOUD mode, the session clock is used only to time stamp the arriving events that don’t have a previously defined timestamp attribute. Although, in STREAM mode, the Session Clock assumes an even more important role.
In STREAM mode, the session clock is responsible for keeping the current timestamp, and based on it, the Drools engine does all the temporal calculations on event’s aging, synchronizes streams from multiple sources, schedules future tasks and so on.
Check the documentation on the Session Clock section to know how to configure and use different session clock implementations.
7.4.2.2. Negative Patterns in Stream Mode
Negative patterns behave different in STREAM mode when compared to CLOUD mode. In CLOUD mode, the Drools engine assumes that all facts and events are known in advance (there is no concept of flow of time) and so, negative patterns are evaluated immediately.
When running in STREAM mode, negative patterns with temporal constraints may require the Drools engine to wait for a time period before activating a rule. The time period is automatically calculated by the Drools engine in a way that the user does not need to use any tricks to achieve the desired result.
For instance:
rule "Sound the alarm"
when
$f : FireDetected( )
not( SprinklerActivated( ) )
then
// sound the alarm
end
The above rule has no temporal constraints that would require delaying the rule, and so, the rule activates immediately. The following rule on the other hand, must wait for 10 seconds before activating, since it may take up to 10 seconds for the sprinklers to activate:
rule "Sound the alarm"
when
$f : FireDetected( )
not( SprinklerActivated( this after[0s,10s] $f ) )
then
// sound the alarm
end
This behaviour allows the Drools engine to keep consistency when dealing with negative patterns and temporal constraints at the same time. The above would be the same as writing the rule as below, but does not burden the user to calculate and explicitly write the appropriate duration parameter:
rule "Sound the alarm"
duration( 10s )
when
$f : FireDetected( )
not( SprinklerActivated( this after[0s,10s] $f ) )
then
// sound the alarm
end
The following rule expects every 10 seconds at least one “Heartbeat” event, if not the rule fires. The special case in this rule is that we use the same type of the object in the first pattern and in the negative pattern. The negative pattern has the temporal constraint to wait between 0 to 10 seconds before firing and it excludes the Heartbeat bound to $h. Excluding the bound Heartbeat is important since the temporal constraint [0s, …] does not exclude by itself the bound event $h from being matched again, thus preventing the rule to fire.
rule "Sound the alarm"
when
$h: Heartbeat( ) from entry-point "MonitoringStream"
not( Heartbeat( this != $h, this after[0s,10s] $h ) from entry-point "MonitoringStream" )
then
// Sound the alarm
end
7.5. Session Clock
Reasoning over time requires a reference clock. Just to mention one example, if a rule reasons over the average price of a given stock over the last 60 minutes, how the Drools engine knows what stock price changes happened over the last 60 minutes in order to calculate the average? The obvious response is: by comparing the timestamp of the events with the "current time". How the Drools engine knows what time is now? Again, obviously, by querying the Session Clock.
The session clock implements a strategy pattern, allowing different types of clocks to be plugged and used by the Drools engine. This is very important because the Drools engine may be running in an elements of different scenarios that may require different clock implementations. Just to mention a few:
-
Rules testing: testing always requires a controlled environment, and when the tests include rules with temporal constraints, it is necessary to not only control the input rules and facts, but also the flow of time.
-
Regular execution: usually, when running rules in production, the application will require a real time clock that allows the Drools engine to react immediately to the time progression.
-
Special environments: specific environments may have specific requirements on time control. Cluster environments may require clock synchronization through heart beats, or JEE environments may require the use of an AppServer provided clock, etc.
-
Rules replay or simulation: to replay scenarios or simulate scenarios it is necessary that the application also controls the flow of time.
7.5.1. Available Clock Implementations
Drools 5 provides 2 clock implementations out of the box. The default real time clock, based on the system clock, and an optional pseudo clock, controlled by the application.
7.5.1.1. Real Time Clock
By default, Drools uses a real time clock implementation that internally uses the system clock to determine the current timestamp.
To explicitly configure the Drools engine to use the real time clock, just set the session configuration parameter to real time:
KieSessionConfiguration config = KieServices.Factory.get().newKieSessionConfiguration();
config.setOption( ClockTypeOption.get("realtime") );
7.5.1.2. Pseudo Clock
Drools also offers out of the box an implementation of a clock that is controlled by the application that is called Pseudo Clock. This clock is specially useful for unit testing temporal rules since it can be controlled by the application and so the results become deterministic.
To configure the pseudo session clock, do:
KieSessionConfiguration config = KieServices.Factory.get().newKieSessionConfiguration();
config.setOption( ClockTypeOption.get("pseudo") );
As an example of how to control the pseudo session clock:
KieSessionConfiguration config = KieServices.Factory.get().newKieSessionConfiguration();
conf.setOption( ClockTypeOption.get( "pseudo" ) );
KieSession session = kbase.newKieSession( conf, null );
SessionPseudoClock clock = session.getSessionClock();
// then, while inserting facts, advance the clock as necessary:
FactHandle handle1 = session.insert( tick1 );
clock.advanceTime( 10, TimeUnit.SECONDS );
FactHandle handle2 = session.insert( tick2 );
clock.advanceTime( 30, TimeUnit.SECONDS );
FactHandle handle3 = session.insert( tick3 );
7.6. Sliding Windows
Sliding Windows are a way to scope the events of interest by defining a window that is constantly moving. The two most common types of sliding window implementations are time based windows and length based windows.
The next sections will detail each of them.
Sliding Windows are only available when running the Drools engine in STREAM mode. Check the Event Processing Mode section for details on how the STREAM mode works. |
Sliding windows start to match immediately and defining a sliding window does not imply that the rule has to wait for the sliding window to be "full" in order to match. For instance, a rule that calculates the average of an event property on a window:length(10) will start calculating the average immediately, and it will start at 0 (zero) for no-events, and will update the average as events arrive one by one. |
7.6.1. Sliding Time Windows
Sliding Time Windows allow the user to write rules that will only match events occurring in the last X time units.
For instance, if the user wants to consider only the Stock Ticks that happened in the last 2 minutes, the pattern would look like this:
StockTick() over window:time( 2m )
Drools uses the "over" keyword to associate windows to patterns.
On a more elaborate example, if the user wants to sound an alarm in case the average temperature over the last 10 minutes read from a sensor is above the threshold value, the rule would look like:
rule "Sound the alarm in case temperature rises above threshold"
when
TemperatureThreshold( $max : max )
Number( doubleValue > $max ) from accumulate(
SensorReading( $temp : temperature ) over window:time( 10m ),
average( $temp ) )
then
// sound the alarm
end
The Drools engine will automatically disregard any SensorReading older than 10 minutes and keep the calculated average consistent.
Please note that time based windows are considered when calculating the interval an event remains in the working memory before being expired, but an event falling off a sliding window does not mean by itself that the event will be discarded from the working memory, as there might be other rules that depend on that event. The Drools engine will discard events only when no other rules depend on that event and the expiration policy for that event type is fulfilled. |
7.6.2. Sliding Length Windows
Sliding Length Windows work the same way as Time Windows, but consider events based on order of their insertion into the session instead of flow of time.
For instance, if the user wants to consider only the last 10 RHT Stock Ticks, independent of how old they are, the pattern would look like this:
StockTick( company == "RHT" ) over window:length( 10 )
As you can see, the pattern is similar to the one presented in the previous section, but instead of using window:time to define the sliding window, it uses window:length.
Using a similar example to the one in the previous section, if the user wants to sound an alarm in case the average temperature over the last 100 readings from a sensor is above the threshold value, the rule would look like:
rule "Sound the alarm in case temperature rises above threshold"
when
TemperatureThreshold( $max : max )
Number( doubleValue > $max ) from accumulate(
SensorReading( $temp : temperature ) over window:length( 100 ),
average( $temp ) )
then
// sound the alarm
end
The Drools engine will consider only the last 100 readings to calculate the average temperature.
Please note that falling off a length based window is not criteria for event expiration in the session. The Drools engine disregards events that fall off a window when calculating that window, but does not remove the event from the session based on that condition alone as there might be other rules that depend on that event. |
Please note that length based windows do not define temporal constraints for event expiration from the session, and the Drools engine will not consider them. If events have no other rules defining temporal constraints and no explicit expiration policy, the Drools engine will keep them in the session indefinitely. |
When using a sliding window, alpha constraints are evaluated before the window is considered, but beta (join) constraints are evaluated afterwards. This usually doesn’t make a difference when time windows are concerned, but it’s important when using a length window. For example this pattern:
StockTick( company == "RHT" ) over window:length( 10 )
defines a window of (at most) 10 StockTicks all having company equal to "RHT", while the following one:
$s : String()
StockTick( company == $s ) over window:length( 10 )
first creates a window of (at most) 10 StockTicks regardless of the value of their company attribute and then filters among them only the ones having the company equal to the String selected from the working memory.
7.6.3. Window Declaration
The Drools engine also supports the declaration of Windows. This promotes a clear separation between what are the filters applied to the window and what are the constraints applied to the result of window. It also allows easy reuse of windows among multiple rules.
Another benefit is a new implementation of the basic window support in the Drools engine, increasing the overall performance of the rules that use sliding windows.
The simplified EBNF to declare a window is:
windowDeclaration := DECLARE WINDOW ID annotation* lhsPatternBind END
For example a window containing only the last 10 stock ticks from a given source can be defined like:
declare window Ticks
StockTick( source == "NYSE" )
over window:length( 10 )
from entry-point STStream
end
Rules can then use this declared window by using it as a source for a FROM as in:
rule "RHT ticks in the window"
when
accumulate( StockTick( company == "RHT" ) from window Ticks,
$cnt : count(1) )
then
// there has been $cnt RHT ticks over the last 10 ticks
end
Note that this example also demonstrates how the window declaration allows to separate the constraints applied to the window (only the StockTicks having "NYSE" as source are among the 10 events included into window) and the constraints applied to the window result (among the last 10 events having "NYSE" as source only the ones with company equal to "RHT" are selected).
7.7. Streams Support
Most CEP use cases have to deal with streams of events. The streams can be provided to the application in various forms, from JMS queues to flat text files, from database tables to raw sockets or even through web service calls. In any case, the streams share a common set of characteristics:
-
events in the stream are ordered by a timestamp. The timestamp may have different semantics for different streams but they are always ordered internally.
-
volumes of events are usually high.
-
atomic events are rarely useful by themselves. Usually meaning is extracted from the correlation between multiple events from the stream and also from other sources.
-
streams may be homogeneous, i.e. contain a single type of events, or heterogeneous, i.e. contain multiple types of events.
Drools generalized the concept of a stream as an "entry point" into the Drools engine. An entry point is for drools a gate from which facts come. The facts may be regular facts or special facts like events.
In Drools, facts from one entry point (stream) may join with facts from any other entry point or event with facts from the working memory. Although, they never mix, i.e., they never lose the reference to the entry point through which they entered the Drools engine. This is important because one may have the same type of facts coming into the Drools engine through several entry points, but one fact that is inserted into the Drools engine through entry point A will never match a pattern from a entry point B, for example.
7.7.1. Declaring and Using Entry Points
Entry points are declared implicitly in Drools by directly making use of them in rules. I.e. referencing an entry point in a rule will make the Drools engine, at compile time, to identify and create the proper internal structures to support that entry point.
So, for instance, lets imagine a banking application, where transactions are fed into the system coming from streams. One of the streams contains all the transactions executed in ATM machines. So, if one of the rules says: a withdraw is authorized if and only if the account balance is over the requested withdraw amount, the rule would look like:
rule "authorize withdraw"
when
WithdrawRequest( $ai : accountId, $am : amount ) from entry-point "ATM Stream"
CheckingAccount( accountId == $ai, balance > $am )
then
// authorize withdraw
end
In the previous example, the Drools engine compiler will identify that the pattern is tied to the entry point "ATM Stream" and will both create all the necessary structures for the rulebase to support the "ATM Stream" and will only match WithdrawRequests coming from the "ATM Stream". In the previous example, the rule is also joining the event from the stream with a fact from the main working memory (CheckingAccount).
Now, lets imagine a second rule that states that a fee of $2 must be applied to any account for which a withdraw request is placed at a bank branch:
rule "apply fee on withdraws on branches"
when
WithdrawRequest( $ai : accountId, processed == true ) from entry-point "Branch Stream"
CheckingAccount( accountId == $ai )
then
// apply a $2 fee on the account
end
The previous rule will match events of the exact same type as the first rule (WithdrawRequest), but from two different streams, so an event inserted into "ATM Stream" will never be evaluated against the pattern on the second rule, because the rule states that it is only interested in patterns coming from the "Branch Stream".
So, entry points, besides being a proper abstraction for streams, are also a way to scope facts in the working memory, and a valuable tool for reducing cross products explosions. But that is a subject for another time.
Inserting events into an entry point is equally simple. Instead of inserting events directly into the working memory, insert them into the entry point as shown in the example below:
// create your rulebase and your session as usual
KieSession session = ...
// get a reference to the entry point
EntryPoint atmStream = session.getEntryPoint( "ATM Stream" );
// and start inserting your facts into the entry point
atmStream.insert( aWithdrawRequest );
The previous example shows how to manually insert facts into a given entry point. Although, usually, the application will use one of the many adapters to plug a stream end point, like a JMS queue, directly into the Drools engine entry point, without coding the inserts manually. The Drools pipeline API has several adapters and helpers to do that as well as examples on how to do it.
7.8. Memory Management for Events
The automatic memory management for events is only performed when running the Drools engine in STREAM mode. Check the Event Processing Mode section for details on how the STREAM mode works. |
One of the benefits of running the Drools engine in STREAM mode is that the Drools engine can detect when an event can no longer match any rule due to its temporal constraints. When that happens, the Drools engine can safely delete the event from the session without side effects and release any resources used by that event.
There are basically 2 ways for the Drools engine to calculate the matching window for a given event:
-
explicitly, using the expiration policy
-
implicitly, analyzing the temporal constraints on events
7.8.1. Explicit expiration offset
The first way of allowing the Drools engine to calculate the window of interest for a given event type is by explicitly setting it. To do that, just use the declare statement and define an expiration for the fact type:
declare StockTick
@expires( 30m )
end
The above example declares an expiration offset of 30 minutes for StockTick events. After that time, assuming no rule still needs the event, the Drools engine will expire and remove the event from the session automatically.
An explicit expiration policy for a given event type overrides any inferred expiration offset for that same type. |
7.8.2. Inferred expiration offset
Another way for the Drools engine to calculate the expiration offset for a given event is implicitly, by analyzing the temporal constraints in the rules. For instance, given the following rule:
rule "correlate orders"
when
$bo : BuyOrderEvent( $id : id )
$ae : AckEvent( id == $id, this after[0,10s] $bo )
then
// do something
end
Analyzing the above rule, the Drools engine automatically calculates that whenever a BuyOrderEvent matches, it needs to store it for up to 10 seconds to wait for matching AckEvent’s. So, the implicit expiration offset for BuyOrderEvent will be 10 seconds. AckEvent, on the other hand, can only match existing BuyOrderEvent’s, and so its expiration offset will be zero seconds.
The Drools engine will make this analysis for the whole rulebase and find the offset for every event type.
An explicit expiration policy for a given event type overrides any inferred expiration offset for that same type. |
7.9. Temporal Reasoning
Temporal reasoning is another requirement of any CEP system. As discussed previously, one of the distinguishing characteristics of events is their strong temporal relationships.
Temporal reasoning is an extensive field of research, from its roots on Temporal Modal Logic to its more practical applications in business systems. There are hundreds of papers and thesis written and approaches are described for several applications. Drools once more takes a pragmatic and simple approach based on several sources, but specially worth noting the following papers:
-
[ALLEN81] Allen, J.F.. An Interval-based Representation of Temporal Knowledge. 1981.
-
[ALLEN83] Allen, J.F.. Maintaining knowledge about temporal intervals. 1983.
-
[BENNE00] Bennet, Brandon and Galton, Antony P.. A Unifying Semantics for Time and Events. 2005.
-
[YONEK05] Yoneki, Eiko and Bacon, Jean. Unified Semantics for Event Correlation Over Time and Space in Hybrid Network Environments. 2005.
Drools implements the Interval-based Time Event Semantics described by Allen, and represents Point-in-Time Events as Interval-based evens with duration 0 (zero).
For all temporal operator intervals, the "" (star) symbol is used to indicate positive infinity and the "-" (minus star) is used to indicate negative infinity. |
7.9.1. Temporal Operators
Drools implements all 13 operators defined by Allen and also their logical complement (negation). This section details each of the operators and their parameters.
7.9.1.1. After
The after evaluator correlates two events and matches when the temporal distance from the current event to the event being correlated belongs to the distance range declared for the operator.
Lets look at an example:
$eventA : EventA( this after[ 3m30s, 4m ] $eventB )
The previous pattern will match if and only if the temporal distance between the time when $eventB finished and the time when $eventA started is between ( 3 minutes and 30 seconds ) and ( 4 minutes ). In other words:
3m30s <= $eventA.startTimestamp - $eventB.endTimeStamp <= 4m
The temporal distance interval for the after operator is optional:
-
If two values are defined (like in the example below), the interval starts on the first value and finishes on the second.
-
If only one value is defined, the interval starts on the value and finishes on the positive infinity.
-
If no value is defined, it is assumed that the initial value is 1ms and the final value is the positive infinity.
It is possible to define negative distances for this operator. Example:
|
If the first value is greater than the second value, the Drools engine automatically reverses them, as there is no reason to have the first value greater than the second value. Example: the following two patterns are considered to have the same semantics:
|
The after, before and coincides operators can be used to define constraints between events, java.util.Date attributes, and long attributes (interpreted as timestamps since epoch) in any combination. Example:
|
7.9.1.2. Before
The before evaluator correlates two events and matches when the temporal distance from the event being correlated to the current correlated belongs to the distance range declared for the operator.
Lets look at an example:
$eventA : EventA( this before[ 3m30s, 4m ] $eventB )
The previous pattern will match if and only if the temporal distance between the time when $eventA finished and the time when $eventB started is between ( 3 minutes and 30 seconds ) and ( 4 minutes ). In other words:
3m30s <= $eventB.startTimestamp - $eventA.endTimeStamp <= 4m
The temporal distance interval for the before operator is optional:
-
If two values are defined (like in the example below), the interval starts on the first value and finishes on the second.
-
If only one value is defined, then the interval starts on the value and finishes on the positive infinity.
-
If no value is defined, it is assumed that the initial value is 1ms and the final value is the positive infinity.
It is possible to define negative distances for this operator. Example:
|
If the first value is greater than the second value, the Drools engine automatically reverses them, as there is no reason to have the first value greater than the second value. Example: the following two patterns are considered to have the same semantics:
|
The after, before and coincides operators can be used to define constraints between events, java.util.Date attributes, and long attributes (interpreted as timestamps since epoch) in any combination. Example:
|
7.9.1.3. Coincides
The coincides evaluator correlates two events and matches when both happen at the same time. Optionally, the evaluator accept thresholds for the distance between events' start and finish timestamps.
Lets look at an example:
$eventA : EventA( this coincides $eventB )
The previous pattern will match if and only if the start timestamps of both $eventA and $eventB are the same AND the end timestamp of both $eventA and $eventB also are the same.
Optionally, this operator accepts one or two parameters. These parameters are the thresholds for the distance between matching timestamps.
-
If only one parameter is given, it is used for both start and end timestamps.
-
If two parameters are given, then the first is used as a threshold for the start timestamp and the second one is used as a threshold for the end timestamp.
In other words:
$eventA : EventA( this coincides[15s, 10s] $eventB )
Above pattern will match if and only if:
abs( $eventA.startTimestamp - $eventB.startTimestamp ) <= 15s &&
abs( $eventA.endTimestamp - $eventB.endTimestamp ) <= 10s
It makes no sense to use negative interval values for the parameters and the Drools engine will raise an error if that happens. |
The after, before and coincides operators can be used to define constraints between events, java.util.Date attributes, and long attributes (interpreted as timestamps since epoch) in any combination. Example:
|
7.9.1.4. During
The during evaluator correlates two events and matches when the current event happens during the occurrence of the event being correlated.
Lets look at an example:
$eventA : EventA( this during $eventB )
The previous pattern will match if and only if the $eventA starts after $eventB starts and finishes before $eventB finishes.
In other words:
$eventB.startTimestamp < $eventA.startTimestamp <= $eventA.endTimestamp < $eventB.endTimestamp
The during operator accepts 1, 2 or 4 optional parameters as follow:
-
If one value is defined, this will be the maximum distance between the start timestamp of both event and the maximum distance between the end timestamp of both events in order to operator match. Example:
$eventA : EventA( this during[ 5s ] $eventB )
Will match if and only if:
0 < $eventA.startTimestamp - $eventB.startTimestamp <= 5s && 0 < $eventB.endTimestamp - $eventA.endTimestamp <= 5s
-
If two values are defined, the first value will be the minimum distance between the timestamps of both events, while the second value will be the maximum distance between the timestamps of both events. Example:
$eventA : EventA( this during[ 5s, 10s ] $eventB )
Will match if and only if:
5s <= $eventA.startTimestamp - $eventB.startTimestamp <= 10s && 5s <= $eventB.endTimestamp - $eventA.endTimestamp <= 10s
-
If four values are defined, the first two values will be the minimum and maximum distances between the start timestamp of both events, while the last two values will be the minimum and maximum distances between the end timestamp of both events. Example:
$eventA : EventA( this during[ 2s, 6s, 4s, 10s ] $eventB )
Will match if and only if:
2s <= $eventA.startTimestamp - $eventB.startTimestamp <= 6s && 4s <= $eventB.endTimestamp - $eventA.endTimestamp <= 10s
7.9.1.5. Finishes
The finishes evaluator correlates two events and matches when the current event’s start timestamp happens after the correlated event’s start timestamp, but both end timestamps occur at the same time.
Lets look at an example:
$eventA : EventA( this finishes $eventB )
The previous pattern will match if and only if the $eventA starts after $eventB starts and finishes at the same time $eventB finishes.
In other words:
$eventB.startTimestamp < $eventA.startTimestamp &&
$eventA.endTimestamp == $eventB.endTimestamp
The finishes evaluator accepts one optional parameter. If it is defined, it determines the maximum distance between the end timestamp of both events in order for the operator to match. Example:
$eventA : EventA( this finishes[ 5s ] $eventB )
Will match if and only if:
$eventB.startTimestamp < $eventA.startTimestamp &&
abs( $eventA.endTimestamp - $eventB.endTimestamp ) <= 5s
It makes no sense to use a negative interval value for the parameter and the Drools engine will raise an exception if that happens. |
7.9.1.6. Finished By
The finishedby evaluator correlates two events and matches when the current event start timestamp happens before the correlated event start timestamp, but both end timestamps occur at the same time. This is the symmetrical opposite of finishes evaluator.
Lets look at an example:
$eventA : EventA( this finishedby $eventB )
The previous pattern will match if and only if the $eventA starts before $eventB starts and finishes at the same time $eventB finishes.
In other words:
$eventA.startTimestamp < $eventB.startTimestamp &&
$eventA.endTimestamp == $eventB.endTimestamp
The finishedby evaluator accepts one optional parameter. If it is defined, it determines the maximum distance between the end timestamp of both events in order for the operator to match. Example:
$eventA : EventA( this finishedby[ 5s ] $eventB )
Will match if and only if:
$eventA.startTimestamp < $eventB.startTimestamp &&
abs( $eventA.endTimestamp - $eventB.endTimestamp ) <= 5s
It makes no sense to use a negative interval value for the parameter and the Drools engine will raise an exception if that happens. |
7.9.1.7. Includes
The includes evaluator correlates two events and matches when the event being correlated happens during the current event. It is the symmetrical opposite of during evaluator.
Lets look at an example:
$eventA : EventA( this includes $eventB )
The previous pattern will match if and only if the $eventB starts after $eventA starts and finishes before $eventA finishes.
In other words:
$eventA.startTimestamp < $eventB.startTimestamp <= $eventB.endTimestamp < $eventA.endTimestamp
The includes operator accepts 1, 2 or 4 optional parameters as follow:
-
If one value is defined, this will be the maximum distance between the start timestamp of both event and the maximum distance between the end timestamp of both events in order to operator match. Example:
$eventA : EventA( this includes[ 5s ] $eventB )
Will match if and only if:
0 < $eventB.startTimestamp - $eventA.startTimestamp <= 5s && 0 < $eventA.endTimestamp - $eventB.endTimestamp <= 5s
-
If two values are defined, the first value will be the minimum distance between the timestamps of both events, while the second value will be the maximum distance between the timestamps of both events. Example:
$eventA : EventA( this includes[ 5s, 10s ] $eventB )
Will match if and only if:
5s <= $eventB.startTimestamp - $eventA.startTimestamp <= 10s && 5s <= $eventA.endTimestamp - $eventB.endTimestamp <= 10s
-
If four values are defined, the first two values will be the minimum and maximum distances between the start timestamp of both events, while the last two values will be the minimum and maximum distances between the end timestamp of both events. Example:
$eventA : EventA( this includes[ 2s, 6s, 4s, 10s ] $eventB )
Will match if and only if:
2s <= $eventB.startTimestamp - $eventA.startTimestamp <= 6s && 4s <= $eventA.endTimestamp - $eventB.endTimestamp <= 10s
7.9.1.8. Meets
The meets evaluator correlates two events and matches when the current event’s end timestamp happens at the same time as the correlated event’s start timestamp.
Lets look at an example:
$eventA : EventA( this meets $eventB )
The previous pattern will match if and only if the $eventA finishes at the same time $eventB starts.
In other words:
abs( $eventB.startTimestamp - $eventA.endTimestamp ) == 0
The meets evaluator accepts one optional parameter. If it is defined, it determines the maximum distance between the end timestamp of current event and the start timestamp of the correlated event in order for the operator to match. Example:
$eventA : EventA( this meets[ 5s ] $eventB )
Will match if and only if:
abs( $eventB.startTimestamp - $eventA.endTimestamp) <= 5s
It makes no sense to use a negative interval value for the parameter and the Drools engine will raise an exception if that happens. |
7.9.1.9. Met By
The metby evaluator correlates two events and matches when the current event’s start timestamp happens at the same time as the correlated event’s end timestamp.
Lets look at an example:
$eventA : EventA( this metby $eventB )
The previous pattern will match if and only if the $eventA starts at the same time $eventB finishes.
In other words:
abs( $eventA.startTimestamp - $eventB.endTimestamp ) == 0
The metby evaluator accepts one optional parameter. If it is defined, it determines the maximum distance between the end timestamp of the correlated event and the start timestamp of the current event in order for the operator to match. Example:
$eventA : EventA( this metby[ 5s ] $eventB )
Will match if and only if:
abs( $eventA.startTimestamp - $eventB.endTimestamp) <= 5s
It makes no sense to use a negative interval value for the parameter and the Drools engine will raise an exception if that happens. |
7.9.1.10. Overlaps
The overlaps evaluator correlates two events and matches when the current event starts before the correlated event starts and finishes after the correlated event starts, but before the correlated event finishes. In other words, both events have an overlapping period.
Lets look at an example:
$eventA : EventA( this overlaps $eventB )
The previous pattern will match if and only if:
$eventA.startTimestamp < $eventB.startTimestamp < $eventA.endTimestamp < $eventB.endTimestamp
The overlaps operator accepts 1 or 2 optional parameters as follow:
-
If one parameter is defined, this will be the maximum distance between the start timestamp of the correlated event and the end timestamp of the current event. Example:
$eventA : EventA( this overlaps[ 5s ] $eventB )
Will match if and only if:
$eventA.startTimestamp < $eventB.startTimestamp < $eventA.endTimestamp < $eventB.endTimestamp && 0 <= $eventA.endTimestamp - $eventB.startTimestamp <= 5s
-
If two values are defined, the first value will be the minimum distance and the second value will be the maximum distance between the start timestamp of the correlated event and the end timestamp of the current event. Example:
$eventA : EventA( this overlaps[ 5s, 10s ] $eventB )
Will match if and only if:
$eventA.startTimestamp < $eventB.startTimestamp < $eventA.endTimestamp < $eventB.endTimestamp && 5s <= $eventA.endTimestamp - $eventB.startTimestamp <= 10s
7.9.1.11. Overlapped By
The overlappedby evaluator correlates two events and matches when the correlated event starts before the current event starts and finishes after the current event starts, but before the current event finishes. In other words, both events have an overlapping period.
Lets look at an example:
$eventA : EventA( this overlappedby $eventB )
The previous pattern will match if and only if:
$eventB.startTimestamp < $eventA.startTimestamp < $eventB.endTimestamp < $eventA.endTimestamp
The overlappedby operator accepts 1 or 2 optional parameters as follow:
-
If one parameter is defined, this will be the maximum distance between the start timestamp of the current event and the end timestamp of the correlated event. Example:
$eventA : EventA( this overlappedby[ 5s ] $eventB )
Will match if and only if:
$eventB.startTimestamp < $eventA.startTimestamp < $eventB.endTimestamp < $eventA.endTimestamp && 0 <= $eventB.endTimestamp - $eventA.startTimestamp <= 5s
-
If two values are defined, the first value will be the minimum distance and the second value will be the maximum distance between the start timestamp of the current event and the end timestamp of the correlated event. Example:
$eventA : EventA( this overlappedby[ 5s, 10s ] $eventB )
Will match if and only if:
$eventB.startTimestamp < $eventA.startTimestamp < $eventB.endTimestamp < $eventA.endTimestamp && 5s <= $eventB.endTimestamp - $eventA.startTimestamp <= 10s
7.9.1.12. Starts
The starts evaluator correlates two events and matches when the current event’s end timestamp happens before the correlated event’s end timestamp, but both start timestamps occur at the same time.
Lets look at an example:
$eventA : EventA( this starts $eventB )
The previous pattern will match if and only if the $eventA finishes before $eventB finishes and starts at the same time $eventB starts.
In other words:
$eventA.startTimestamp == $eventB.startTimestamp &&
$eventA.endTimestamp < $eventB.endTimestamp
The starts evaluator accepts one optional parameter. If it is defined, it determines the maximum distance between the start timestamp of both events in order for the operator to match. Example:
$eventA : EventA( this starts[ 5s ] $eventB )
Will match if and only if:
abs( $eventA.startTimestamp - $eventB.startTimestamp ) <= 5s &&
$eventA.endTimestamp < $eventB.endTimestamp
It makes no sense to use a negative interval value for the parameter and the Drools engine will raise an exception if that happens. |
7.9.1.13. Started By
The startedby evaluator correlates two events and matches when the correlating event’s end timestamp happens before the current event’s end timestamp, but both start timestamps occur at the same time. Lets look at an example:
$eventA : EventA( this startedby $eventB )
The previous pattern will match if and only if the $eventB finishes before $eventA finishes and starts at the same time $eventB starts.
In other words:
$eventA.startTimestamp == $eventB.startTimestamp &&
$eventA.endTimestamp > $eventB.endTimestamp
The startedby evaluator accepts one optional parameter. If it is defined, it determines the maximum distance between the start timestamp of both events in order for the operator to match. Example:
$eventA : EventA( this starts[ 5s ] $eventB )
Will match if and only if:
abs( $eventA.startTimestamp - $eventB.startTimestamp ) <= 5s &&
$eventA.endTimestamp > $eventB.endTimestamp
It makes no sense to use a negative interval value for the parameter and the Drools engine will raise an exception if that happens. |
8. Decision Model and Notation (DMN)
8.1. Decision Model and Notation (DMN)
Decision Model and Notation (DMN) is a standard established by the Object Management Group (OMG) for describing and modeling operational decisions. DMN defines an XML schema that enables DMN models to be shared between DMN-compliant platforms and across organizations so that business analysts and business rules developers can collaborate in designing and implementing DMN decision services. The DMN standard is similar to and can be used together with the Business Process Model and Notation (BPMN) standard for designing and modeling business processes.
For more information about the background and applications of DMN, see the OMG Decision Model and Notation specification.
8.1.1. DMN conformance levels
The DMN specification defines three incremental levels of conformance in a software implementation. A product that claims compliance at one level must also be compliant with any preceding levels. For example, a conformance level 3 implementation must also include the supported components in conformance levels 1 and 2. For the formal definitions of each conformance level, see the OMG Decision Model and Notation specification.
The following are summaries of the three DMN conformance levels:
- Conformance level 1
-
A DMN conformance level 1 implementation supports decision requirement diagrams (DRDs), decision logic, and decision tables, but decision models are not executable. Any language can be used to define the expressions, including natural, unstructured languages.
- Conformance level 2
-
A DMN conformance level 2 implementation includes the requirements in conformance level 1, and supports Simplified Friendly Enough Expression Language (S-FEEL) expressions and fully executable decision models.
- Conformance level 3
-
A DMN conformance level 3 implementation includes the requirements in conformance levels 1 and 2, and supports Friendly Enough Expression Language (FEEL) expressions, the full set of boxed expressions, and fully executable decision models.
Drools provides design and runtime support for DMN 1.2 models at conformance level 3. You can design your DMN models directly in Business Central or import existing DMN models into your Drools projects for deployment and execution.
8.1.2. DMN decision requirements diagram (DRD) components
A decision requirements diagram (DRD) is a visual representation of your DMN model. This diagram consists of one or more decision requirements graphs (DRGs) that represent a particular domain of an overall DRD. The DRGs trace business decisions using decision nodes, business knowledge models, sources of business knowledge, input data, and decision services.
The following table summarizes the components in a DRD:
Component | Description | Notation | |
---|---|---|---|
Elements |
Decision |
Node where one or more input elements determine an output based on defined decision logic. |
![]() |
Business knowledge model |
Reusable function with one or more decision elements. Decisions that have the same logic but depend on different sub-input data or sub-decisions use business knowledge models to determine which procedure to follow. |
![]() |
|
Knowledge source |
External authorities, documents, committees, or policies that regulate a decision or business knowledge model. Knowledge sources are references to real-world factors rather than executable business rules. |
![]() |
|
Input data |
Information used in a decision node or a business knowledge model. Input data usually includes business-level concepts or objects relevant to the business, such as loan applicant data used in a lending strategy. |
![]() |
|
Decision service |
Top-level decision containing a set of reusable decisions published as a service for invocation. A decision service can be invoked from an external application or a BPMN business process. |
![]() |
|
Requirement connectors |
Information requirement |
Connection from an input data node or decision node to another decision node that requires the information. |
![]() |
Knowledge requirement |
Connection from a business knowledge model to a decision node or to another business knowledge model that invokes the decision logic. |
![]() |
|
Authority requirement |
Connection from an input data node or a decision node to a dependent knowledge source or from a knowledge source to a decision node, business knowledge model, or another knowledge source. |
![]() |
|
Artifacts |
Text annotation |
Explanatory note associated with an input data node, decision node, business knowledge model, or knowledge source. |
![]() |
Association |
Connection from an input data node, decision node, business knowledge model, or knowledge source to a text annotation. |
![]() |
The following table summarizes the permitted connectors between DRD elements:
Starts from | Connects to | Connection type | Example |
---|---|---|---|
Decision |
Decision |
Information requirement |
![]() |
Business knowledge model |
Decision |
Knowledge requirement |
![]() |
Business knowledge model |
![]() |
||
Decision service |
Decision |
Knowledge requirement |
![]() |
Business knowledge model |
![]() |
||
Input data |
Decision |
Information requirement |
![]() |
Knowledge source |
Authority requirement |
![]() |
|
Knowledge source |
Decision |
Authority requirement |
![]() |
Business knowledge model |
![]() |
||
Knowledge source |
![]() |
||
Decision |
Text annotation |
Association |
![]() |
Business knowledge model |
![]() |
||
Knowledge source |
![]() |
||
Input data |
![]() |
The following example DRD illustrates some of these DMN components in practice:
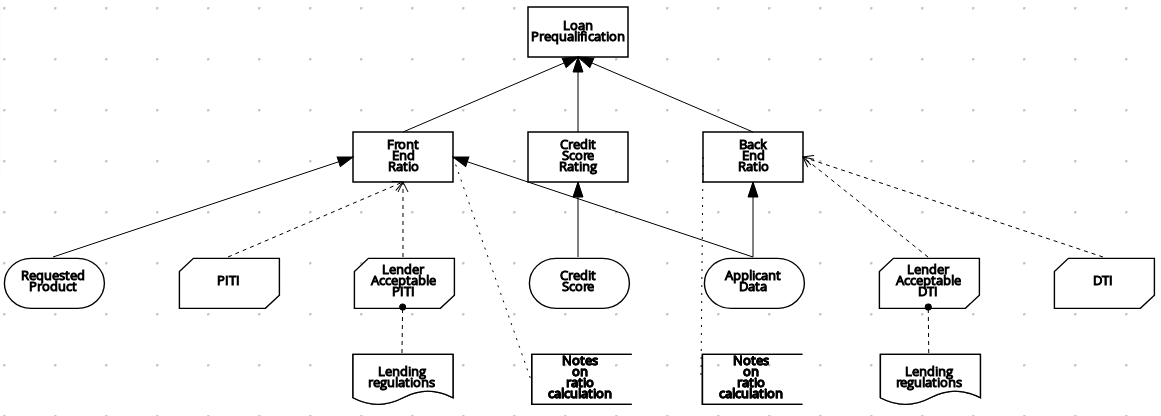
The following example DRD illustrates DMN components that are part of a reusable decision service:
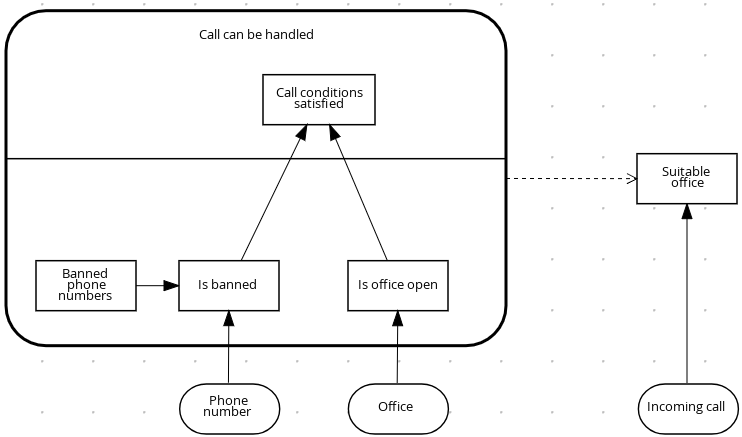
In a DMN decision service node, the decision nodes in the bottom segment incorporate input data from outside of the decision service to arrive at a final decision in the top segment of the decision service node. The resulting top-level decisions from the decision service are then implemented in any subsequent decisions or business knowledge requirements of the DMN model. You can reuse DMN decision services in other DMN models to apply the same decision logic with different input data and different outgoing connections.
8.1.3. Rule expressions in FEEL
Friendly Enough Expression Language (FEEL) is an expression language defined by the Object Management Group (OMG) DMN specification. FEEL expressions define the logic of a decision in a DMN model. FEEL is designed to facilitate both decision modeling and execution by assigning semantics to the decision model constructs. FEEL expressions in decision requirements diagrams (DRDs) occupy table cells in boxed expressions for decision nodes and business knowledge models.
For more information about FEEL in DMN, see the OMG Decision Model and Notation specification.
8.1.3.1. Variable and function names in FEEL
Unlike many traditional expression languages, Friendly Enough Expression Language (FEEL) supports spaces and a few special characters as part of variable and function names. A FEEL name must start with a letter
, ?
, or _
element. The unicode letter characters are also allowed. Variable names cannot start with a language keyword, such as and
, true
, or every
. The remaining characters in a variable name can be any of the starting characters, as well as digits
, white spaces, and special characters such as +
, -
, /
, *
, '
, and .
.
For example, the following names are all valid FEEL names:
-
Age
-
Birth Date
-
Flight 234 pre-check procedure
Several limitations apply to variable and function names in FEEL:
- Ambiguity
-
The use of spaces, keywords, and other special characters as part of names can make FEEL ambiguous. The ambiguities are resolved in the context of the expression, matching names from left to right. The parser resolves the variable name as the longest name matched in scope. You can use
( )
to disambiguate names if necessary. - Spaces in names
-
The DMN specification limits the use of spaces in FEEL names. According to the DMN specification, names can contain multiple spaces but not two consecutive spaces.
In order to make the language easier to use and avoid common errors due to spaces, Drools removes the limitation on the use of consecutive spaces. Drools supports variable names with any number of consecutive spaces, but normalizes them into a single space. For example, the variable references
First Name
with one space andFirst Name
with two spaces are both acceptable in Drools.Drools also normalizes the use of other white spaces, like the non-breakable white space that is common in web pages, tabs, and line breaks. From a Drools FEEL engine perspective, all of these characters are normalized into a single white space before processing.
- The keyword
in
-
The keyword
in
is the only keyword in the language that cannot be used as part of a variable name. Although the specifications allow the use of keywords in the middle of variable names, the use ofin
in variable names conflicts with the grammar definition offor
,every
andsome
expression constructs.
8.1.3.2. Data types in FEEL
Friendly Enough Expression Language (FEEL) supports the following data types:
-
Numbers
-
Strings
-
Boolean values
-
Dates
-
Time
-
Date and time
-
Days and time duration
-
Years and months duration
-
Functions
-
Contexts
-
Ranges (or intervals)
-
Lists
The DMN specification currently does not provide an explicit way of declaring a variable as a function , context , range , or list , but Drools extends the DMN built-in types to support variables of these types.
|
The following are descriptions of each data type:
- Numbers
-
Numbers in FEEL are based on the IEEE 754-2008 Decimal 128 format, with 34 digits of precision. Internally, numbers are represented in Java as
BigDecimals
withMathContext DECIMAL128
. FEEL supports only one number data type, so the same type is used to represent both integers and floating point numbers.FEEL numbers use a dot (
.
) as a decimal separator. FEEL does not support-INF
,+INF
, orNaN
. FEEL usesnull
to represent invalid numbers.Drools extends the DMN specification and supports additional number notations:
-
Scientific: You can use scientific notation with the suffix
e<exp>
orE<exp>
. For example,1.2e3
is the same as writing the expression1.2*10**3
, but is a literal instead of an expression. -
Hexadecimal: You can use hexadecimal numbers with the prefix
0x
. For example,0xff
is the same as the decimal number255
. Both uppercase and lowercase letters are supported. For example,0XFF
is the same as0xff
. -
Type suffixes: You can use the type suffixes
f
,F
,d
,D
,l
, andL
. These suffixes are ignored.
-
- Strings
-
Strings in FEEL are any sequence of characters delimited by double quotation marks.
Example:
"John Doe"
- Boolean values
-
FEEL uses three-valued boolean logic, so a boolean logic expression may have values
true
,false
, ornull
. - Dates
-
Date literals are not supported in FEEL, but you can use the built-in
date()
function to construct date values. Date strings in FEEL follow the format defined in the XML Schema Part 2: Datatypes document. The format is"YYYY-MM-DD"
whereYYYY
is the year with four digits,MM
is the number of the month with two digits, andDD
is the number of the day.Example:
date( "2017-06-23" )
Date objects have time equal to
"00:00:00"
, which is midnight. The dates are considered to be local, without a timezone. - Time
-
Time literals are not supported in FEEL, but you can use the built-in
time()
function to construct time values. Time strings in FEEL follow the format defined in the XML Schema Part 2: Datatypes document. The format is"hh:mm:ss[.uuu][(+-)hh:mm]"
wherehh
is the hour of the day (from00
to23
),mm
is the minutes in the hour, andss
is the number of seconds in the minute. Optionally, the string may define the number of milliseconds (uuu
) within the second and contain a positive (+
) or negative (-
) offset from UTC time to define its timezone. Instead of using an offset, you can use the letterz
to represent the UTC time, which is the same as an offset of-00:00
. If no offset is defined, the time is considered to be local.Examples:
time( "04:25:12" ) time( "14:10:00+02:00" ) time( "22:35:40.345-05:00" ) time( "15:00:30z" )
Time values that define an offset or a timezone cannot be compared to local times that do not define an offset or a timezone.
- Date and time
-
Date and time literals are not supported in FEEL, but you can use the built-in
date and time()
function to construct date and time values. Date and time strings in FEEL follow the format defined in the XML Schema Part 2: Datatypes document. The format is"<date>T<time>"
, where<date>
and<time>
follow the prescribed XML schema formatting, conjoined byT
.Examples:
date and time( "2017-10-22T23:59:00" ) date and time( "2017-06-13T14:10:00+02:00" ) date and time( "2017-02-05T22:35:40.345-05:00" ) date and time( "2017-06-13T15:00:30z" )
Date and time values that define an offset or a timezone cannot be compared to local date and time values that do not define an offset or a timezone.
If your implementation of the DMN specification does not support spaces in the XML schema, use the keyword dateTime
as a synonym ofdate and time
. - Days and time duration
-
Days and time duration literals are not supported in FEEL, but you can use the built-in
duration()
function to construct days and time duration values. Days and time duration strings in FEEL follow the format defined in the XML Schema Part 2: Datatypes document, but are restricted to only days, hours, minutes and seconds. Months and years are not supported.Examples:
duration( "P1DT23H12M30S" ) duration( "P23D" ) duration( "PT12H" ) duration( "PT35M" )
If your implementation of the DMN specification does not support spaces in the XML schema, use the keyword dayTimeDuration
as a synonym ofdays and time duration
. - Years and months duration
-
Years and months duration literals are not supported in FEEL, but you can use the built-in
duration()
function to construct days and time duration values. Years and months duration strings in FEEL follow the format defined in the XML Schema Part 2: Datatypes document, but are restricted to only years and months. Days, hours, minutes, or seconds are not supported.Examples:
duration( "P3Y5M" ) duration( "P2Y" ) duration( "P10M" ) duration( "P25M" )
If your implementation of the DMN specification does not support spaces in the XML schema, use the keyword yearMonthDuration
as a synonym ofyears and months duration
. - Functions
-
FEEL has
function
literals (or anonymous functions) that you can use to create functions. The DMN specification currently does not provide an explicit way of declaring a variable as afunction
, but Drools extends the DMN built-in types to support variables of functions.Example:
function(a, b) a + b
In this example, the FEEL expression creates a function that adds the parameters
a
andb
and returns the result. - Contexts
-
FEEL has
context
literals that you can use to create contexts. Acontext
in FEEL is a list of key and value pairs, similar to maps in languages like Java. The DMN specification currently does not provide an explicit way of declaring a variable as acontext
, but Drools extends the DMN built-in types to support variables of contexts.Example:
{ x : 5, y : 3 }
In this example, the expression creates a context with two entries,
x
andy
, representing a coordinate in a chart.In DMN 1.2, another way to create contexts is to create an item definition that contains the list of keys as attributes, and then declare the variable as having that item definition type.
The Drools DMN API supports DMN
ItemDefinition
structural types in aDMNContext
represented in two ways:-
User-defined Java type: Must be a valid JavaBeans object defining properties and getters for each of the components in the DMN
ItemDefinition
. If necessary, you can also use the@FEELProperty
annotation for those getters representing a component name which would result in an invalid Java identifier. -
java.util.Map
interface: The map needs to define the appropriate entries, with the keys corresponding to the component name in the DMNItemDefinition
.
-
- Ranges (or intervals)
-
FEEL has
range
literals that you can use to create ranges or intervals. Arange
in FEEL is a value that defines a lower and an upper bound, where either can be open or closed. The DMN specification currently does not provide an explicit way of declaring a variable as arange
, but Drools extends the DMN built-in types to support variables of ranges.The syntax of a range is defined in the following formats:
range := interval_start endpoint '..' endpoint interval_end interval_start := open_start | closed_start open_start := '(' | ']' closed_start := '[' interval_end := open_end | closed_end open_end := ')' | '[' closed_end := ']' endpoint := expression
The expression for the endpoint must return a comparable value, and the lower bound endpoint must be lower than the upper bound endpoint.
For example, the following literal expression defines an interval between
1
and10
, including the boundaries (a closed interval on both endpoints):[ 1 .. 10 ]
The following literal expression defines an interval between 1 hour and 12 hours, including the lower boundary (a closed interval), but excluding the upper boundary (an open interval):
[ duration("PT1H") .. duration("PT12H") ]
You can use ranges in decision tables to test for ranges of values, or use ranges in simple literal expressions. For example, the following literal expression returns
true
if the value of a variablex
is between0
and100
:x in [ 1 .. 100 ]
- Lists
-
FEEL has
list
literals that you can use to create lists of items. Alist
in FEEL is represented by a comma-separated list of values enclosed in square brackets. The DMN specification currently does not provide an explicit way of declaring a variable as alist
, but Drools extends the DMN built-in types to support variables of lists.Example:
[ 2, 3, 4, 5 ]
All lists in FEEL contain elements of the same type and are immutable. Elements in a list can be accessed by index, where the first element is
1
. Negative indexes can access elements starting from the end of the list so that-1
is the last element.For example, the following expression returns the second element of a list
x
:x[2]
The following expression returns the second-to-last element of a list
x
:x[-2]
8.1.4. DMN decision logic in boxed expressions
Boxed expressions in DMN are tables that you use to define the underlying logic of decision nodes and business knowledge models in a decision requirements diagram (DRD) or decision requirements graph (DRG). Some boxed expressions can contain other boxed expressions, but the top-level boxed expression corresponds to the decision logic of a single DRD artifact. While DRDs with one or more DRGs represent the flow of a DMN decision model, boxed expressions define the actual decision logic of individual nodes. DRDs and boxed expressions together form a complete and functional DMN decision model.
The following are the types of DMN boxed expressions:
-
Decision tables
-
Literal expressions
-
Contexts
-
Relations
-
Functions
-
Invocations
-
Lists
Drools does not provide boxed list expressions in Business Central, but supports a FEEL list data type that you can use in boxed literal expressions. For more information about the list data type and other FEEL data types in Drools, see Data types in FEEL.
|
All Friendly Enough Expression Language (FEEL) expressions that you use in your boxed expressions must conform to the FEEL syntax requirements in the OMG Decision Model and Notation specification.
8.1.4.1. DMN decision tables
A decision table in DMN is a visual representation of one or more business rules in a tabular format. You use decision tables to define rules for a decision node that applies those rules at a given point in the decision model. Each rule consists of a single row in the table, and includes columns that define the conditions (input) and outcome (output) for that particular row. The definition of each row is precise enough to derive the outcome using the values of the conditions. Input and output values can be FEEL expressions or defined data type values.
For example, the following decision table determines credit score ratings based on a defined range of a loan applicant’s credit score:
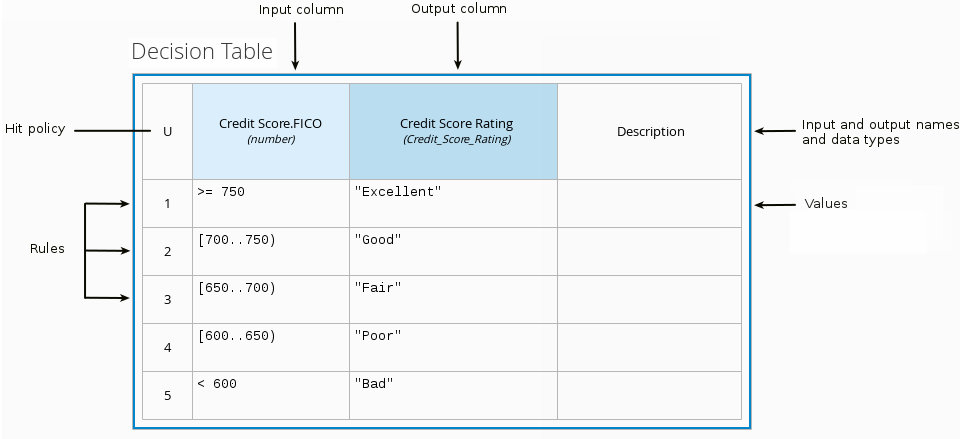
The following decision table determines the next step in a lending strategy for applicants depending on applicant loan eligibility and the bureau call type:
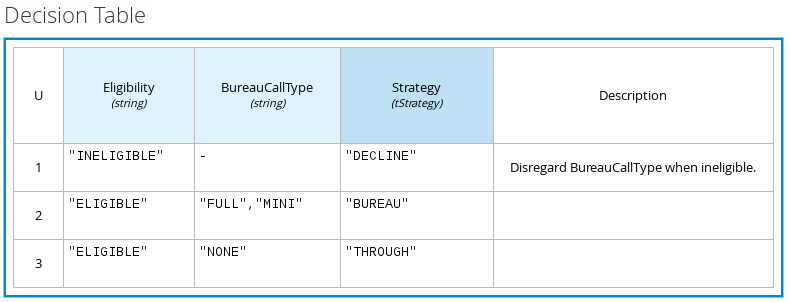
The following decision table determines applicant qualification for a loan as the concluding decision node in a loan prequalification decision model:
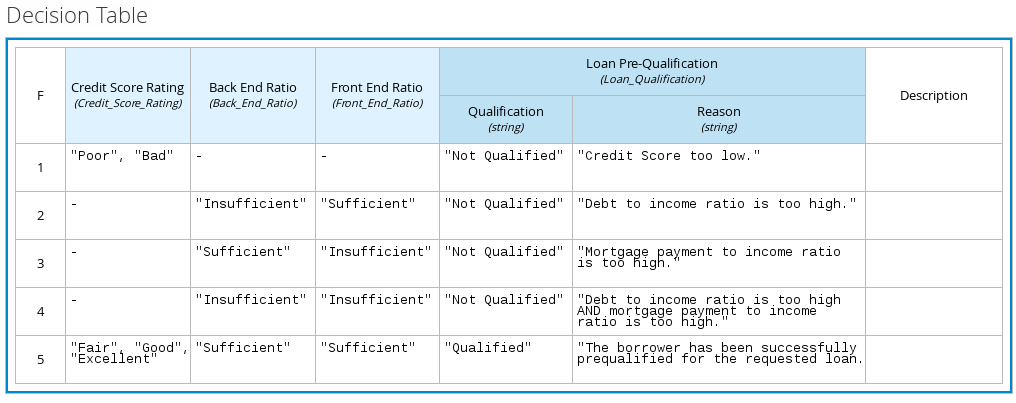
Decision tables are a popular way of modeling rules and decision logic, and are used in many methodologies (such as DMN) and implementation frameworks (such as Drools).
Drools supports both DMN decision tables and Drools-native decision tables, but they are different types of assets with different syntax requirements and are not interchangeable. For more information about Drools-native decision tables in Drools, see Spreadsheet decision tables. |
Hit policies in DMN decision tables
Hit policies determine how to reach an outcome when multiple rules in a decision table match the provided input values. For example, if one rule in a decision table applies a sales discount to military personnel and another rule applies a discount to students, then when a customer is both a student and in the military, the decision table hit policy must indicate whether to apply one discount or the other (Unique, First) or both discounts (Collect Sum). You specify the single character of the hit policy (U, F, C+) in the upper-left corner of the decision table.
The following are supported DMN decision table hit policies:
-
Unique (U): Permits only one rule to match. Any overlap raises an error.
-
Any (A): Permits multiple rules to match, but they must all have the same output. If multiple matching rules do not have the same output, an error is raised.
-
Priority (P): Permits multiple rules to match, with different outputs. The output that comes first in the output values list is selected.
-
First (F): Uses the first match in rule order.
-
Collect (C+, C>, C<, C#): Aggregates output from multiple rules based on an aggregation function.
-
Collect ( C ): Aggregates values in an arbitrary list.
-
Collect Sum (C+): Outputs the sum of all collected values. Values must be numeric.
-
Collect Min (C<): Outputs the minimum value among the matches. The resulting values must be comparable, such as numbers, dates, or text (lexicographic order).
-
Collect Max (C>): Outputs the maximum value among the matches. The resulting values must be comparable, such as numbers, dates or text (lexicographic order).
-
Collect Count (C#): Outputs the number of matching rules.
-
8.1.4.2. Boxed literal expressions
A boxed literal expression in DMN is a literal FEEL expression as text in a table cell, typically with a labeled column and an assigned data type. You use boxed literal expressions to define simple or complex node logic or decision data directly in FEEL for a particular node in a decision. Literal FEEL expressions must conform to FEEL syntax requirements in the OMG Decision Model and Notation specification.
For example, the following boxed literal expression defines the minimum acceptable PITI calculation (principal, interest, taxes, and insurance) in a lending decision, where acceptable rate
is a variable defined in the DMN model:
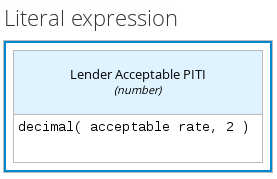
The following boxed literal expression sorts a list of possible dating candidates (soul mates) in an online dating application based on their score on criteria such as age, location, and interests:
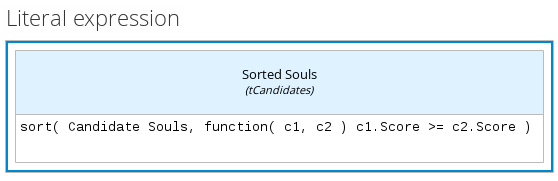
8.1.4.3. Boxed context expressions
A boxed context expression in DMN is a set of variable names and values with a result value. Each name-value pair is a context entry. You use context expressions to represent data definitions in decision logic and set a value for a desired decision element within the DMN decision model. A value in a boxed context expression can be a data type value or FEEL expression, or can contain a nested sub-expression of any type, such as a decision table, a literal expression, or another context expression.
For example, the following boxed context expression defines the factors for sorting delayed passengers in a flight-rebooking decision model, based on defined data types (tPassengerTable
, tFlightNumberList
):
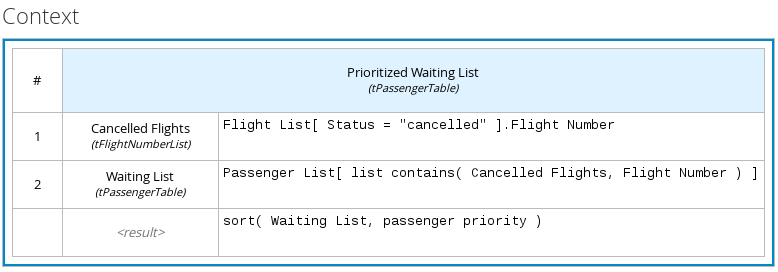
The following boxed context expression defines the factors that determine whether a loan applicant can meet minimum mortgage payments based on principal, interest, taxes, and insurance (PITI), represented as a front-end ratio calculation with a sub-context expression:
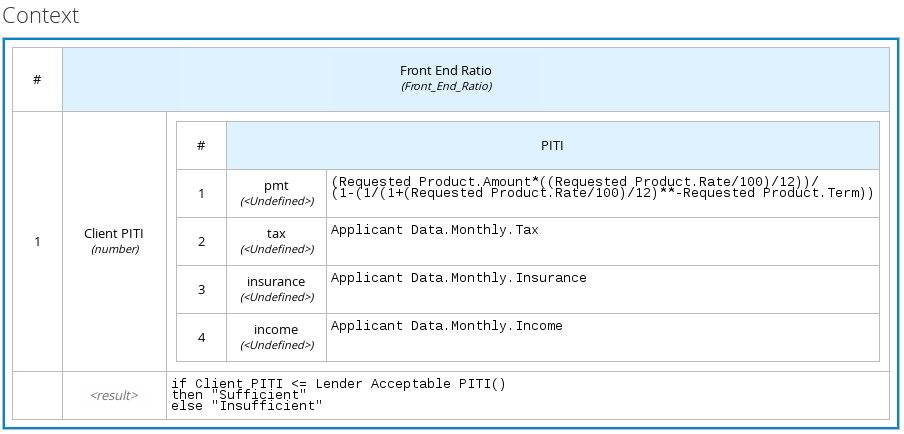
8.1.4.4. Boxed relation expressions
A boxed relation expression in DMN is a traditional data table with information about given entities, listed as rows. You use boxed relation tables to define decision data for relevant entities in a decision at a particular node. Boxed relation expressions are similar to context expressions in that they set variable names and values, but relation expressions contain no result value and list all variable values based on a single defined variable in each column.
For example, the following boxed relation expression provides information about employees in an employee rostering decision:
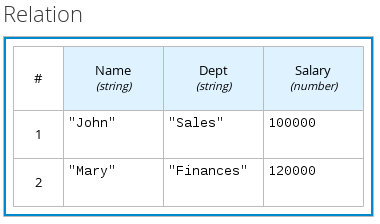
8.1.4.5. Boxed function expressions
A boxed function expression in DMN is a parameterized boxed expression containing a literal FEEL expression, a nested context expression of an external JAVA or PMML function, or a nested boxed expression of any type. By default, all business knowledge models are defined as boxed function expressions. You use boxed function expressions to call functions on your decision logic and to define all business knowledge models.
For example, the following boxed function expression determines airline flight capacity in a flight-rebooking decision model:
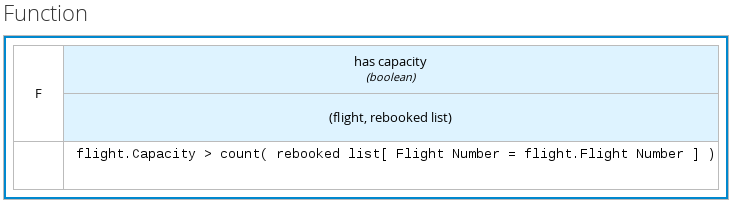
The following boxed function expression contains a basic Java function as a context expression for determining absolute value in a decision model calculation:
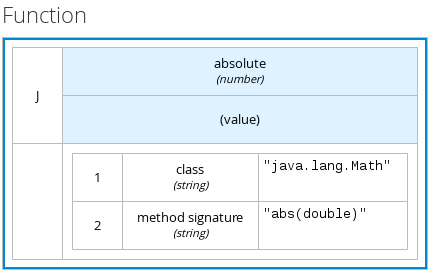
The following boxed function expression determines a monthly mortgage installment as a business knowledge model in a lending decision, with the function value defined as a nested context expression:
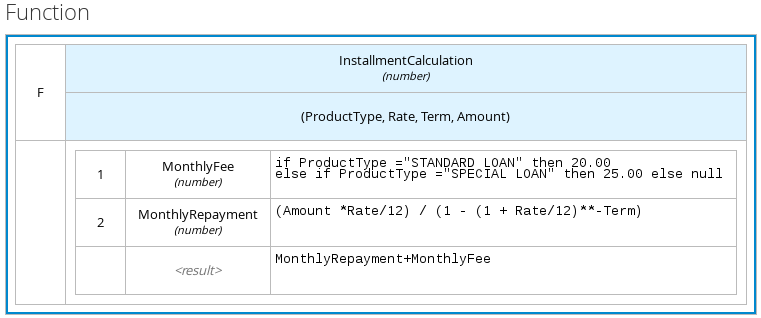
8.1.4.6. Boxed invocation expressions
A boxed invocation expression in DMN is a boxed expression that invokes a business knowledge model. A boxed invocation expression contains the name of the business knowledge model to be invoked and a list of parameter bindings. Each binding is represented by two boxed expressions on a row: The box on the left contains the name of a parameter and the box on the right contains the binding expression whose value is assigned to the parameter to evaluate the invoked business knowledge model. You use boxed invocations to invoke at a particular decision node a business knowledge model defined in the decision model.
For example, the following boxed invocation expression invokes a reassign next passenger
business knowledge model as the concluding decision node in a flight-rebooking decision model:
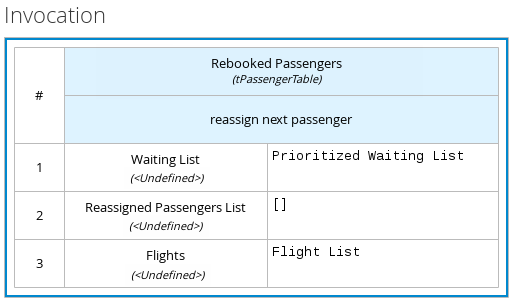
The following boxed invocation expression invokes an InstallmentCalculation
business knowledge model to calculate a monthly installment amount for a loan before proceeding to affordability decisions:
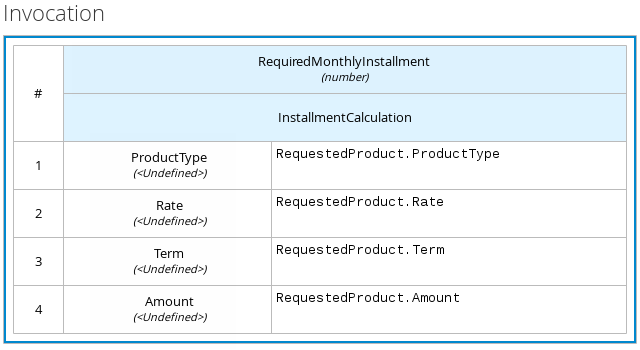
8.1.5. DMN model example
The following is a real-world DMN model example that demonstrates how you can use decision modeling to reach a decision based on input data, circumstances, and company guidelines. In this scenario, a flight from San Diego to New York is canceled, requiring the affected airline to find alternate arrangements for its inconvenienced passengers.
First, the airline collects the information necessary to determine how best to get the travelers to their destinations:
- Input data
-
-
List of flights
-
List of passengers
-
- Decisions
-
-
Prioritize the passengers who will get seats on a new flight
-
Determine which flights those passengers will be offered
-
- Business knowledge models
-
-
The company process for determining passenger priority
-
Any flights that have space available
-
Company rules for determining how best to reassign inconvenienced passengers
-
The airline then uses the DMN standard to model its decision process in the following decision requirements diagram (DRD) for determining the best rebooking solution:
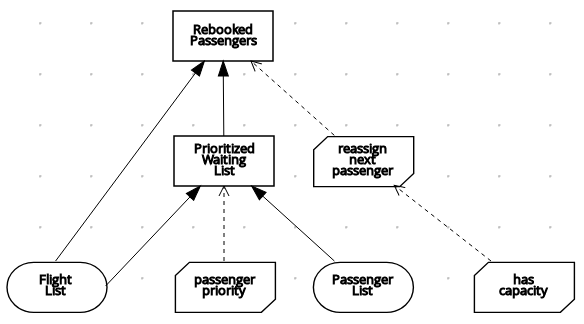
Similar to flowcharts, DRDs use shapes to represent the different elements in a process. Ovals contain the two necessary input data, rectangles contain the decision points in the model, and rectangles with clipped corners (business knowledge models) contain reusable logic that can be repeatedly invoked.
The DRD draws logic for each element from boxed expressions that provide variable definitions using FEEL expressions or data type values.
Some boxed expressions are basic, such as the following decision for establishing a prioritized waiting list:
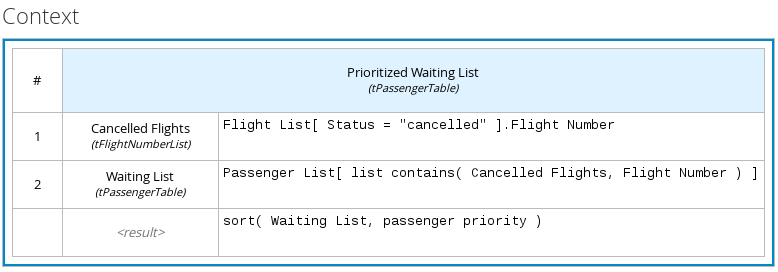
Some boxed expressions are more complex with greater detail and calculation, such as the following business knowledge model for reassigning the next delayed passenger:
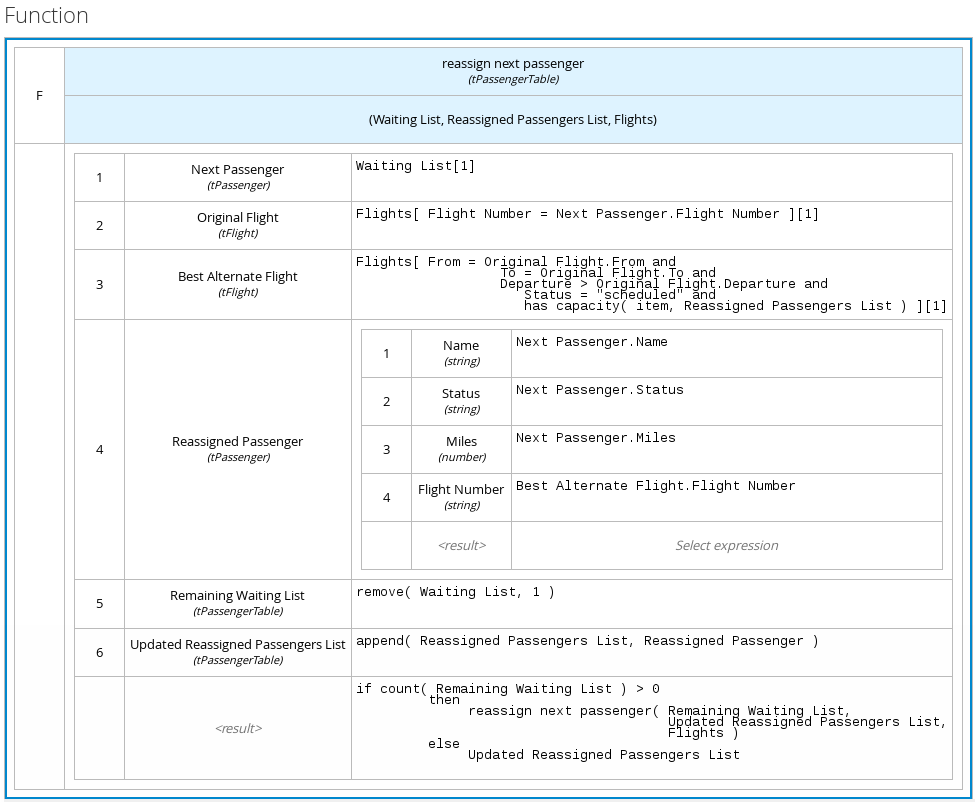
The following is the DMN source file for this decision model:
<definitions xmlns="http://www.omg.org/spec/DMN/20151101/dmn.xsd" xmlns:kie="https://www.drools.org/kie-dmn" xmlns:feel="http://www.omg.org/spec/FEEL/20140401" id="_0019_flight_rebooking" name="0019-flight-rebooking" namespace="https://www.drools.org/kie-dmn">
<itemDefinition id="_tFlight" name="tFlight">
<itemComponent id="_tFlight_Flight" name="Flight Number">
<typeRef>feel:string</typeRef>
</itemComponent>
<itemComponent id="_tFlight_From" name="From">
<typeRef>feel:string</typeRef>
</itemComponent>
<itemComponent id="_tFlight_To" name="To">
<typeRef>feel:string</typeRef>
</itemComponent>
<itemComponent id="_tFlight_Dep" name="Departure">
<typeRef>feel:dateTime</typeRef>
</itemComponent>
<itemComponent id="_tFlight_Arr" name="Arrival">
<typeRef>feel:dateTime</typeRef>
</itemComponent>
<itemComponent id="_tFlight_Capacity" name="Capacity">
<typeRef>feel:number</typeRef>
</itemComponent>
<itemComponent id="_tFlight_Status" name="Status">
<typeRef>feel:string</typeRef>
</itemComponent>
</itemDefinition>
<itemDefinition id="_tFlightTable" isCollection="true" name="tFlightTable">
<typeRef>kie:tFlight</typeRef>
</itemDefinition>
<itemDefinition id="_tPassenger" name="tPassenger">
<itemComponent id="_tPassenger_Name" name="Name">
<typeRef>feel:string</typeRef>
</itemComponent>
<itemComponent id="_tPassenger_Status" name="Status">
<typeRef>feel:string</typeRef>
</itemComponent>
<itemComponent id="_tPassenger_Miles" name="Miles">
<typeRef>feel:number</typeRef>
</itemComponent>
<itemComponent id="_tPassenger_Flight" name="Flight Number">
<typeRef>feel:string</typeRef>
</itemComponent>
</itemDefinition>
<itemDefinition id="_tPassengerTable" isCollection="true" name="tPassengerTable">
<typeRef>kie:tPassenger</typeRef>
</itemDefinition>
<itemDefinition id="_tFlightNumberList" isCollection="true" name="tFlightNumberList">
<typeRef>feel:string</typeRef>
</itemDefinition>
<inputData id="i_Flight_List" name="Flight List">
<variable name="Flight List" typeRef="kie:tFlightTable"/>
</inputData>
<inputData id="i_Passenger_List" name="Passenger List">
<variable name="Passenger List" typeRef="kie:tPassengerTable"/>
</inputData>
<decision name="Prioritized Waiting List" id="d_PrioritizedWaitingList">
<variable name="Prioritized Waiting List" typeRef="kie:tPassengerTable"/>
<informationRequirement>
<requiredInput href="#i_Passenger_List"/>
</informationRequirement>
<informationRequirement>
<requiredInput href="#i_Flight_List"/>
</informationRequirement>
<knowledgeRequirement>
<requiredKnowledge href="#b_PassengerPriority"/>
</knowledgeRequirement>
<context>
<contextEntry>
<variable name="Cancelled Flights" typeRef="kie:tFlightNumberList"/>
<literalExpression>
<text>Flight List[ Status = "cancelled" ].Flight Number</text>
</literalExpression>
</contextEntry>
<contextEntry>
<variable name="Waiting List" typeRef="kie:tPassengerTable"/>
<literalExpression>
<text>Passenger List[ list contains( Cancelled Flights, Flight Number ) ]</text>
</literalExpression>
</contextEntry>
<contextEntry>
<literalExpression>
<text>sort( Waiting List, passenger priority )</text>
</literalExpression>
</contextEntry>
</context>
</decision>
<decision name="Rebooked Passengers" id="d_RebookedPassengers">
<variable name="Rebooked Passengers" typeRef="kie:tPassengerTable"/>
<informationRequirement>
<requiredDecision href="#d_PrioritizedWaitingList"/>
</informationRequirement>
<informationRequirement>
<requiredInput href="#i_Flight_List"/>
</informationRequirement>
<knowledgeRequirement>
<requiredKnowledge href="#b_ReassignNextPassenger"/>
</knowledgeRequirement>
<invocation>
<literalExpression>
<text>reassign next passenger</text>
</literalExpression>
<binding>
<parameter name="Waiting List"/>
<literalExpression>
<text>Prioritized Waiting List</text>
</literalExpression>
</binding>
<binding>
<parameter name="Reassigned Passengers List"/>
<literalExpression>
<text>[]</text>
</literalExpression>
</binding>
<binding>
<parameter name="Flights"/>
<literalExpression>
<text>Flight List</text>
</literalExpression>
</binding>
</invocation>
</decision>
<businessKnowledgeModel id="b_PassengerPriority" name="passenger priority">
<encapsulatedLogic>
<formalParameter name="Passenger1" typeRef="kie:tPassenger"/>
<formalParameter name="Passenger2" typeRef="kie:tPassenger"/>
<decisionTable hitPolicy="UNIQUE">
<input id="b_Passenger_Priority_dt_i_P1_Status" label="Passenger1.Status">
<inputExpression typeRef="feel:string">
<text>Passenger1.Status</text>
</inputExpression>
<inputValues>
<text>"gold", "silver", "bronze"</text>
</inputValues>
</input>
<input id="b_Passenger_Priority_dt_i_P2_Status" label="Passenger2.Status">
<inputExpression typeRef="feel:string">
<text>Passenger2.Status</text>
</inputExpression>
<inputValues>
<text>"gold", "silver", "bronze"</text>
</inputValues>
</input>
<input id="b_Passenger_Priority_dt_i_P1_Miles" label="Passenger1.Miles">
<inputExpression typeRef="feel:string">
<text>Passenger1.Miles</text>
</inputExpression>
</input>
<output id="b_Status_Priority_dt_o" label="Passenger1 has priority">
<outputValues>
<text>true, false</text>
</outputValues>
<defaultOutputEntry>
<text>false</text>
</defaultOutputEntry>
</output>
<rule id="b_Passenger_Priority_dt_r1">
<inputEntry id="b_Passenger_Priority_dt_r1_i1">
<text>"gold"</text>
</inputEntry>
<inputEntry id="b_Passenger_Priority_dt_r1_i2">
<text>"gold"</text>
</inputEntry>
<inputEntry id="b_Passenger_Priority_dt_r1_i3">
<text>>= Passenger2.Miles</text>
</inputEntry>
<outputEntry id="b_Passenger_Priority_dt_r1_o1">
<text>true</text>
</outputEntry>
</rule>
<rule id="b_Passenger_Priority_dt_r2">
<inputEntry id="b_Passenger_Priority_dt_r2_i1">
<text>"gold"</text>
</inputEntry>
<inputEntry id="b_Passenger_Priority_dt_r2_i2">
<text>"silver","bronze"</text>
</inputEntry>
<inputEntry id="b_Passenger_Priority_dt_r2_i3">
<text>-</text>
</inputEntry>
<outputEntry id="b_Passenger_Priority_dt_r2_o1">
<text>true</text>
</outputEntry>
</rule>
<rule id="b_Passenger_Priority_dt_r3">
<inputEntry id="b_Passenger_Priority_dt_r3_i1">
<text>"silver"</text>
</inputEntry>
<inputEntry id="b_Passenger_Priority_dt_r3_i2">
<text>"silver"</text>
</inputEntry>
<inputEntry id="b_Passenger_Priority_dt_r3_i3">
<text>>= Passenger2.Miles</text>
</inputEntry>
<outputEntry id="b_Passenger_Priority_dt_r3_o1">
<text>true</text>
</outputEntry>
</rule>
<rule id="b_Passenger_Priority_dt_r4">
<inputEntry id="b_Passenger_Priority_dt_r4_i1">
<text>"silver"</text>
</inputEntry>
<inputEntry id="b_Passenger_Priority_dt_r4_i2">
<text>"bronze"</text>
</inputEntry>
<inputEntry id="b_Passenger_Priority_dt_r4_i3">
<text>-</text>
</inputEntry>
<outputEntry id="b_Passenger_Priority_dt_r4_o1">
<text>true</text>
</outputEntry>
</rule>
<rule id="b_Passenger_Priority_dt_r5">
<inputEntry id="b_Passenger_Priority_dt_r5_i1">
<text>"bronze"</text>
</inputEntry>
<inputEntry id="b_Passenger_Priority_dt_r5_i2">
<text>"bronze"</text>
</inputEntry>
<inputEntry id="b_Passenger_Priority_dt_r5_i3">
<text>>= Passenger2.Miles</text>
</inputEntry>
<outputEntry id="b_Passenger_Priority_dt_r5_o1">
<text>true</text>
</outputEntry>
</rule>
</decisionTable>
</encapsulatedLogic>
<variable name="passenger priority" typeRef="feel:boolean"/>
</businessKnowledgeModel>
<businessKnowledgeModel id="b_ReassignNextPassenger" name="reassign next passenger">
<encapsulatedLogic>
<formalParameter name="Waiting List" typeRef="kie:tPassengerTable"/>
<formalParameter name="Reassigned Passengers List" typeRef="kie:tPassengerTable"/>
<formalParameter name="Flights" typeRef="kie:tFlightTable"/>
<context>
<contextEntry>
<variable name="Next Passenger" typeRef="kie:tPassenger"/>
<literalExpression>
<text>Waiting List[1]</text>
</literalExpression>
</contextEntry>
<contextEntry>
<variable name="Original Flight" typeRef="kie:tFlight"/>
<literalExpression>
<text>Flights[ Flight Number = Next Passenger.Flight Number ][1]</text>
</literalExpression>
</contextEntry>
<contextEntry>
<variable name="Best Alternate Flight" typeRef="kie:tFlight"/>
<literalExpression>
<text>Flights[ From = Original Flight.From and To = Original Flight.To and Departure > Original Flight.Departure and Status = "scheduled" and has capacity( item, Reassigned Passengers List ) ][1]</text>
</literalExpression>
</contextEntry>
<contextEntry>
<variable name="Reassigned Passenger" typeRef="kie:tPassenger"/>
<context>
<contextEntry>
<variable name="Name" typeRef="feel:string"/>
<literalExpression>
<text>Next Passenger.Name</text>
</literalExpression>
</contextEntry>
<contextEntry>
<variable name="Status" typeRef="feel:string"/>
<literalExpression>
<text>Next Passenger.Status</text>
</literalExpression>
</contextEntry>
<contextEntry>
<variable name="Miles" typeRef="feel:number"/>
<literalExpression>
<text>Next Passenger.Miles</text>
</literalExpression>
</contextEntry>
<contextEntry>
<variable name="Flight Number" typeRef="feel:string"/>
<literalExpression>
<text>Best Alternate Flight.Flight Number</text>
</literalExpression>
</contextEntry>
</context>
</contextEntry>
<contextEntry>
<variable name="Remaining Waiting List" typeRef="kie:tPassengerTable"/>
<literalExpression>
<text>remove( Waiting List, 1 )</text>
</literalExpression>
</contextEntry>
<contextEntry>
<variable name="Updated Reassigned Passengers List" typeRef="kie:tPassengerTable"/>
<literalExpression>
<text>append( Reassigned Passengers List, Reassigned Passenger )</text>
</literalExpression>
</contextEntry>
<contextEntry>
<literalExpression>
<text>if count( Remaining Waiting List ) > 0 then reassign next passenger( Remaining Waiting List, Updated Reassigned Passengers List, Flights ) else Updated Reassigned Passengers List</text>
</literalExpression>
</contextEntry>
</context>
</encapsulatedLogic>
<variable name="reassign next passenger" typeRef="kie:tPassengerTable"/>
<knowledgeRequirement>
<requiredKnowledge href="#b_HasCapacity"/>
</knowledgeRequirement>
</businessKnowledgeModel>
<businessKnowledgeModel id="b_HasCapacity" name="has capacity">
<encapsulatedLogic>
<formalParameter name="flight" typeRef="kie:tFlight"/>
<formalParameter name="rebooked list" typeRef="kie:tPassengerTable"/>
<literalExpression>
<text>flight.Capacity > count( rebooked list[ Flight Number = flight.Flight Number ] )</text>
</literalExpression>
</encapsulatedLogic>
<variable name="has capacity" typeRef="feel:boolean"/>
</businessKnowledgeModel>
</definitions>
8.2. DMN support in Drools
Drools provides design and runtime support for DMN 1.2 models at conformance level 3. You can integrate DMN models with your Drools decision services in several ways:
-
Design your DMN models directly in Business Central using the DMN designer
-
Import DMN files into your project in Business Central (Menu → Design → Projects → Import Asset)
-
Package DMN files as part of your project knowledge JAR (KJAR) file without Business Central
In addition to all DMN conformance level 3 requirements, Drools also includes enhancements and fixes to FEEL and DMN model components to optimize the experience of implementing DMN decision services with Drools. From a platform perspective, DMN models are like any other business asset in Drools, such as DRL files or spreadsheet decision tables, that you can include in your Drools project and deploy to KIE Server in order to start your DMN decision services.
For more information about including external DMN files with your Drools project packaging and deployment method, see Build, Deploy, Utilize and Run.
8.2.1. FEEL enhancements in Drools
Drools includes the following enhancements and other changes to FEEL in the current DMN implementation:
-
Space Sensitivity: This DMN implementation of the FEEL language is space insensitive. The goal is to avoid non-deterministic behavior based on the context and differences in behavior based on invisible characters, such as white spaces. This means that for this implementation, a variable named
first name
with one space is exactly the same asfirst name
with two spaces in it. -
List functions
or()
andand()
: The specification defines two list functions namedor()
andand()
. However, according to the FEEL grammar, these are not valid function names, asand
andor
are reserved keywords. This implementation renames these functions toany()
andall()
respectively, in anticipation for DMN 1.2. -
Keyword
in
cannot be used in variable names: The specification defines that any keyword can be reused as part of a variable name, but the ambiguities caused with thefor … in … return
loop prevent the reuse of thein
keyword. All other keywords are supported as part of variable names. -
Keywords are not supported in attributes of anonymous types: FEEL is not a strongly typed language and the parser must resolve ambiguity in name parts of an attribute of an anonymous type. The parser supports reusable keywords as part of a variable name defined in the scope, but the parser does not support keywords in attributes of an anonymous type. For example,
for item in Order.items return Federal Tax for Item( item )
is a valid and supported FEEL expression, where a function namedFederal Tax for Item(…)
can be defined and invoked correctly in the scope. However, the expressionfor i in [ {x and y : true, n : 1}, {x and y : false, n: 2} ] return i.x and y
is not supported because anonymous types are defined in the iteration context of thefor
expression and the parser cannot resolve the ambiguity. -
Support for date and time literals on ranges: According to the grammar rules #8, #18, #19, #34 and #62,
date and time
literals are supported in ranges (pages 110-111). Chapter 10.3.2.7 on page 114, on the other hand, contradicts the grammar and says they are not supported. This implementation chose to follow the grammar and supportdate and time
literals on ranges, as well as extend the specification to support any arbitrary expression (see extensions below). -
Invalid time syntax: Chapter 10.3.2.3.4 on page 112 and bullet point about
time
on page 131 both state that thetime
string lexical representation follows the XML Schema Datatypes specification as well as ISO 8601. According to the XML Schema specification (https://www.w3.org/TR/xmlschema-2/#time), the lexical representation of a time follows the patternhh:mm:ss.sss
without any leading character. The DMN specification uses a leading "T" in several examples, that we understand is a typo and not in accordance with the standard. -
Support for scientific and hexadecimal notations: This implementation supports scientific and hexadecimal notation for numbers. For example,
1.2e5
(scientific notation),0xD5
(hexadecimal notation). -
Support for expressions as end points in ranges: This implementation supports expressions as endpoints for ranges. For example,
[date("2016-11-24")..date("2016-11-27")]
-
Support for additional types: The specification only defines the following as basic types of the language:
-
number
-
string
-
boolean
-
days and time duration
-
years and month duration
-
time
-
date and time
For completeness and orthogonality, this implementation also supports the following types:
-
context
-
list
-
range
-
function
-
unary test
-
-
Support for unary tests: For completeness and orthogonality, unary tests are supported as first class citizens in the language. They are functions with an implicit single parameter and can be invoked in the same way as functions. For example,
UnaryTestAsFunction.feel{ is minor : < 18, Bob is minor : is minor( bob.age ) }
-
Support for additional built-in functions: The following additional functions are supported:
-
now()
: Returns the current local date and time. -
today()
: Returns the current local date. -
decision table()
: Returns a decision table function, although the specification mentions a decision table. The function on page 114 is not implementable as defined. -
string( mask, p… )
: Returns a string formatted as per the mask. See Java String.format() for details on the mask syntax. For example,string( "%4.2f", 7.1298 )
returns the string"7.12"
.
-
-
Support for additional date and time arithmetics: Subtracting two dates returns a day and time duration with the number of days between the two dates, ignoring daylight savings. For example,
DateArithmetic.feeldate( "2017-05-12" ) - date( "2017-04-25" ) = duration( "P17D" )
8.2.2. DMN model enhancements in Drools
Drools includes the following enhancements to DMN model support in the current DMN implementation:
-
Support for types with spaces on names: The DMN XML schema defines type refs such as QNames. The QNames do not allow spaces. Therefore, it is not possible to use types like FEEL
date and time
,days and time duration
oryears and months duration
. This implementation does parse such typerefs as strings and allows type names with spaces. However, in order to comply with the XML schema, it also adds the following aliases to such types that can be used instead:-
date and time
=dateTime
-
days and time duration
=duration
ordayTimeDuration
-
years and months duration
=duration
oryearMonthDuration
Note that, for the "duration" types, the user can simply use
duration
and the Drools engine will infer the proper duration, eitherdays and time duration
oryears and months duration
.
-
-
Lists support heterogeneous element types: Currently this implementation supports lists with heterogeneous element types. This is an experimental extension and does limit the functionality of some functions and filters. This decision will be re-evaluated in the future.
-
TypeRef link between Decision Tables and Item Definitions: On decision tables/input clause, if no values list is defined, the Drools engine automatically checks the type reference and applies the allowed values check if it is defined.
8.2.3. Configurable DMN properties in Drools
Drools provides the following DMN properties that you can configure when you execute your DMN models on KIE Server or on your client application:
- org.kie.dmn.strictConformance
-
When enabled, this property disables by default any extensions or profiles provided beyond the DMN standard, such as some helper functions or enhanced features of DMN 1.2 backported into DMN 1.1. You can use this property to configure the Drools engine to support only pure DMN features, such as when running the DMN Technology Compatibility Kit (TCK).
Default value:
false
-Dorg.kie.dmn.strictConformance=true
- org.kie.dmn.runtime.typecheck
-
When enabled, this property enables verification of actual values conforming to their declared types in the DMN model, as input or output of DRD elements. You can use this property to verify whether data supplied to the DMN model or produced by the DMN model is compliant with what is specified in the model.
Default value:
false
-Dorg.kie.dmn.runtime.typecheck=true
- org.kie.dmn.decisionservice.coercesingleton
-
By default, this property makes the result of a decision service defining a single output decision be the single value of the output decision value. When disabled, this property makes the result of a decision service defining a single output decision be a
context
with the single entry for that decision. You can use this property to adjust your decision service outputs according to your project requirements.Default value:
true
-Dorg.kie.dmn.decisionservice.coercesingleton=false
- org.kie.dmn.profiles.$PROFILE_NAME
-
When valorized with a Java fully qualified name, this property loads a DMN profile onto the Drools engine at start time. You can use this property to implement a predefined DMN profile with supported features different from or beyond the DMN standard. For example, if you are creating DMN models using the Signavio DMN modeller, use this property to implement features from the Signavio DMN profile into your DMN decision service.
-Dorg.kie.dmn.profiles.signavio=org.kie.dmn.signavio.KieDMNSignavioProfile
- org.kie.dmn.compiler.execmodel
-
When enabled, this property enables DMN decision table logic to be compiled into executable rule models during run time. You can use this property to evaluate DMN decision table logic more efficiently. This property is helpful when the executable model compilation was not originally performed during project compile time. Enabling this property may result in added compile time during the first evaluation by the Drools engine, but subsequent compilations are more efficient.
Default value:
false
-Dorg.kie.dmn.compiler.execmodel=true
8.3. Creating and editing DMN models in Business Central
You can use the DMN designer in Business Central to design DMN decision requirements diagrams (DRDs) and define decision logic for a complete and functional DMN decision model. Drools provides design and runtime support for DMN 1.2 models at conformance level 3, and includes enhancements and fixes to FEEL and DMN model components to optimize the experience of implementing DMN decision services with Drools.
-
In Business Central, go to Menu → Design → Projects and click the project name.
-
Create or import a DMN file in your Business Central project.
To create a DMN file, click Add Asset → DMN, enter an informative DMN model name, select the appropriate Package, and click Ok.
To import an existing DMN file, click Import Asset, enter the DMN model name, select the appropriate Package, select the DMN file to upload, and click Ok.
The new DMN file is now listed in the DMN panel of the Project Explorer, and the DMN decision requirements diagram (DRD) canvas appears.
If you imported a DMN file that does not contain layout information, the imported decision requirements diagram (DRD) is formatted automatically in the DMN designer. Click Save in the DMN designer to save the DRD layout. -
Begin adding components to your new or imported DMN decision requirements diagram (DRD) by clicking and dragging one of the DMN nodes from the left toolbar.
The following DRD components are available:
-
Decision: Use this node for a DMN decision, where one or more input elements determine an output based on defined decision logic.
-
Business knowledge model: Use this node for reusable functions with one or more decision elements. Decisions that have the same logic but depend on different sub-input data or sub-decisions use business knowledge models to determine which procedure to follow.
-
Knowledge source: Use this node for external authorities, documents, committees, or policies that regulate a decision or business knowledge model. Knowledge sources are references to real-world factors rather than executable business rules.
-
Input data: Use this node for information used in a decision node or a business knowledge model. Input data usually includes business-level concepts or objects relevant to the business, such as loan applicant data used in a lending strategy.
-
Text annotation: Use this node for explanatory notes associated with an input data node, decision node, business knowledge model, or knowledge source.
-
Decision service: Use this node to enclose a set of reusable decisions implemented as a decision service for invocation. A decision service can be used in other DMN models and can be invoked from an external application or a BPMN business process.
-
-
In the DMN designer canvas, double-click the new DRD node to enter an informative node name.
-
If the node is a decision or business knowledge model, select the node to display the node options and click the Edit icon to open the DMN boxed expression designer to define the decision logic for the node:
Figure 118. Opening a new decision node boxed expressionFigure 119. Opening a new business knowledge model boxed expressionBy default, all business knowledge models are defined as boxed function expressions containing a literal FEEL expression, a nested context expression of an external JAVA or PMML function, or a nested boxed expression of any type.
For decision nodes, you click the undefined table to select the type of boxed expression you want to use, such as a boxed literal expression, boxed context expression, decision table, or other DMN boxed expression.
For business knowledge models, you click the top-left function cell to select the function type, or right-click the function value cell, select Clear, and select a boxed expression of another type.
-
In the selected boxed expression designer for either a decision node (any expression type) or business knowledge model (function expression), click the applicable table cells to define the table name, variable data types, variable names and values, function parameters and bindings, or FEEL expressions to include in the decision logic.
You can right-click cells for additional actions where applicable, such as inserting or removing table rows and columns or clearing table contents.
The following is an example decision table for a decision node that determines credit score ratings based on a defined range of a loan applicant’s credit score:
Figure 120. Decision node decision table for credit score ratingThe following is an example boxed function expression for a business knowledge model that calculates mortgage payments based on principal, interest, taxes, and insurance (PITI) as a literal expression:
Figure 121. Business knowledge model function for PITI calculation -
After you define the decision logic for the selected node, click Back to "<NODE_NAME>" to return to the DRD view.
-
For the selected DRD node, use the available connection options to create and connect to the next node in the DRD, or click and drag a new node onto the DRD canvas from the left toolbar.
The node type determines which connection options are supported. For example, an Input data node can connect to a decision node, knowledge source, or text annotation using the applicable connection type, whereas a Knowledge source node can connect to any DRD element. A Decision node can connect only to another decision or a text annotation.
The following connection types are available, depending on the node type:
-
Information requirement: Use this connection from an input data node or decision node to another decision node that requires the information.
-
Knowledge requirement: Use this connection from a business knowledge model to a decision node or to another business knowledge model that invokes the decision logic.
-
Authority requirement: Use this connection from an input data node or a decision node to a dependent knowledge source or from a knowledge source to a decision node, business knowledge model, or another knowledge source.
-
Association: Use this connection from an input data node, decision node, business knowledge model, or knowledge source to a text annotation.
Figure 122. Connecting credit score input to credit score rating decision -
-
Continue adding and defining the remaining DRD components of your decision model. Periodically click Save in the DMN designer to save your work.
-
After you add and define all components of the DRD, click Save to save and validate the completed DRD.
The following is an example DRD for a loan prequalification decision model:
Figure 123. Completed DRD for loan prequalificationThe following is an example DRD for a phone call handling decision model using a reusable decision service:
Figure 124. Completed DRD for phone call handling with a decision serviceIn a DMN decision service node, the decision nodes in the bottom segment incorporate input data from outside of the decision service to arrive at a final decision in the top segment of the decision service node. The resulting top-level decisions from the decision service are then implemented in any subsequent decisions or business knowledge requirements of the DMN model. You can reuse DMN decision services in other DMN models to apply the same decision logic with different input data and different outgoing connections.
8.3.1. Defining DMN decision logic in boxed expressions in Business Central
Boxed expressions in DMN are tables that you use to define the underlying logic of decision nodes and business knowledge models in a decision requirements diagram (DRD) or decision requirements graph (DRG). Some boxed expressions can contain other boxed expressions, but the top-level boxed expression corresponds to the decision logic of a single DRD artifact. While DRDs with one or more DRGs represent the flow of a DMN decision model, boxed expressions define the actual decision logic of individual nodes. DRDs and boxed expressions together form a complete and functional DMN decision model.
You can use the DMN designer in Business Central to define decision logic for your DRD components using built-in boxed expressions.
-
You have created or imported a DMN file in Business Central.
-
In Business Central, go to Menu → Design → Projects, click the project name, and select the DMN file you want to modify.
-
In the DMN designer canvas, select a decision node or business knowledge model that you want to define and click the Edit icon to open the DMN boxed expression designer:
Figure 125. Opening a new decision node boxed expressionFigure 126. Opening a new business knowledge model boxed expressionBy default, all business knowledge models are defined as boxed function expressions containing a literal FEEL expression, a nested context expression of an external JAVA or PMML function, or a nested boxed expression of any type.
For decision nodes, you click the undefined table to select the type of boxed expression you want to use, such as a boxed literal expression, boxed context expression, decision table, or other DMN boxed expression.
For business knowledge models, you click the top-left function cell to select the function type, or right-click the function value cell, select Clear, and select a boxed expression of another type.
-
For this example, use a decision node and select Decision Table as the boxed expression type.
A decision table in DMN is a visual representation of one or more rules in a tabular format. Each rule consists of a single row in the table, and includes columns that define the conditions (input) and outcome (output) for that particular row.
-
Click the input column header to define the name and data type for the input condition. For example, name the input column Credit Score.FICO with a
number
data type. This column specifies numeric credit score values or ranges of loan applicants. -
Click the output column header to define the name and data type for the output values. For example, name the output column Credit Score Rating and next to the Data Type option, click Manage to go to the Data Types page where you can create a custom data type with score ratings as constraints.
-
On the Data Types page, click Add and create a Credit_Score_Rating data type as a
string
. -
Click Constraints, select Enumeration from the drop-down options, and add the following constraints:
-
"Excellent"
-
"Good"
-
"Fair"
-
"Poor"
-
"Bad"
For information about constraint types and syntax requirements for the specified data type, see the Decision Model and Notation specification.
-
-
Click Ok to save the constraints and click Save to save the data type.
-
Return to the Credit Score Rating decision table, click the Credit Score Rating column header, and set the data type to this new custom data type.
-
Use the Credit Score.FICO input column to define credit score values or ranges of values, and use the Credit Score Rating column to specify one of the corresponding ratings you defined in the Credit_Score_Rating data type.
Right-click any value cell to insert or delete rows (rules) or columns (clauses).
Figure 127. Decision node decision table for credit score rating -
After you define all rules, click the top-left corner of the decision table to define the rule Hit Policy and Builtin Aggregator (for COLLECT hit policy only).
The hit policy determines how to reach an outcome when multiple rules in a decision table match the provided input values. The built-in aggregator determines how to aggregate rule values when you use the COLLECT hit policy.
The following example is a more complex decision table that determines applicant qualification for a loan as the concluding decision node in the same loan prequalification decision model:
Figure 128. Decision table for loan prequalification
For boxed expression types other than decision tables, you follow these guidelines similarly to navigate the boxed expression tables and define variables and parameters for decision logic, but according to the requirements of the boxed expression type. Some boxed expressions, such as boxed literal expressions, can be single-column tables, while other boxed expressions, such as function, context, and invocation expressions, can be multi-column tables with nested boxed expressions of other types.
For example, the following boxed context expression defines the parameters that determine whether a loan applicant can meet minimum mortgage payments based on principal, interest, taxes, and insurance (PITI), represented as a front-end ratio calculation with a sub-context expression:
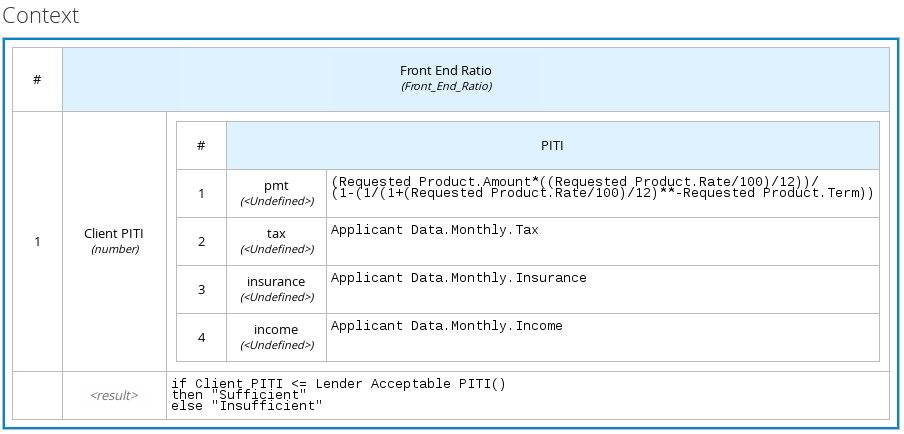
The following boxed function expression determines a monthly mortgage installment as a business knowledge model in a lending decision, with the function value defined as a nested context expression:
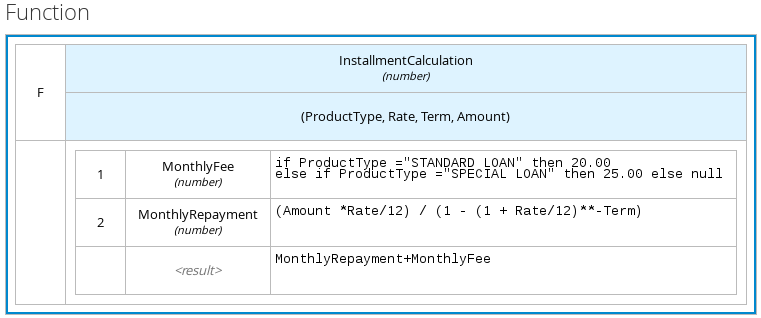
For more information and examples of each boxed expression type, see DMN decision logic in boxed expressions.
8.3.2. Creating custom data types for DMN boxed expressions in Business Central
In DMN boxed expressions in Business Central, data types determine the structure of the data that you use within an associated table, column, or field in the boxed expression. You can use default DMN data types (such as String, Number, Boolean) or you can create custom data types to specify additional fields and constraints that you want to implement for the boxed expression values.
Custom data types that you create for a boxed expression can be simple or structured:
-
Simple data types have only a name and a type assignment. Example:
Age (number)
. -
Structured data types contain multiple fields associated with a parent data type. Example: A single type
Person
containing the fieldsName (string)
,Age (number)
,Email (string)
.
-
You have created or imported a DMN file in Business Central.
-
In Business Central, go to Menu → Design → Projects, click the project name, and select the DMN file you want to modify.
-
In the DMN designer canvas, select a decision node or business knowledge model for which you want to define the data types and click the Edit icon to open the DMN boxed expression designer.
-
If the boxed expression is for a decision node that is not yet defined, click the undefined table to select the type of boxed expression you want to use, such as a boxed literal expression, boxed context expression, decision table, or other DMN boxed expression.
-
Click the cell for the table header, column header, or parameter field (depending on the boxed expression type) for which you want to define the data type and click Manage to go to the Data Types page where you can create a custom data type.
You can also set and manage custom output data types for a specified decision node or business knowledge model node by selecting the Diagram properties icon in the upper-right corner of the DMN designer:
The data type that you define for a specified cell in a boxed expression determines the structure of the data that you use within that associated table, column, or field in the boxed expression.
In this example, an output column Credit Score Rating for a DMN decision table defines a set of custom credit score ratings based on an applicant’s credit score.
-
On the Data Types page, click Add and create a Credit_Score_Rating data type as a
string
.If the data type requires a list of items, enable the List setting.
-
Click Constraints, select Enumeration from the drop-down options, and add the following constraints:
-
"Excellent"
-
"Good"
-
"Fair"
-
"Poor"
-
"Bad"
For information about constraint types and syntax requirements for the specified data type, see the Decision Model and Notation specification.
-
-
Click Ok to save the constraints and click Save to save the data type.
-
Return to the Credit Score Rating decision table, click the Credit Score Rating column header, set the data type to this new custom data type, and define the rule values for that column with the rating constraints that you specified.
Figure 131. Decision table for credit score ratingIn the DMN decision model for this scenario, the Credit Score Rating decision flows into the following Loan Prequalification decision that also requires custom data types:
-
Continuing with this example, return to the Data Types window, click Add, and create a Loan_Qualification data type as a
Structure
with no constraints. -
Next to the Loan_Qualification data type, select the settings icon (three vertical dots) and select Insert nested field to insert sub-fields within this parent data type.
You can use these sub-fields in association with the parent structured data type in boxed expressions, such as nested column headers in decision tables or nested table parameters in context or function expressions.
-
For this example, under the structured Loan_Qualification data type, add a Qualification field with
"Qualified"
and"Not Qualified"
enumeration constraints, and a Reason field with no constraints. Add also a simple Back_End_Ratio and a Front_End_Ratio data type, both with"Sufficient"
and"Insufficient"
enumeration constraints.Click Save for each data type that you create.
-
Return to the decision table and, for each column, click the column header cell, set the data type to the new corresponding custom data type, and define the rule values as needed for the column with the constraints that you specified, if applicable.
Figure 132. Decision table for loan prequalification
For boxed expression types other than decision tables, you follow these guidelines similarly to navigate the boxed expression tables and define custom data types as needed.
For example, the following boxed function expression uses custom tCandidate
and tProfile
structured data types to associate data for online dating compatibility:
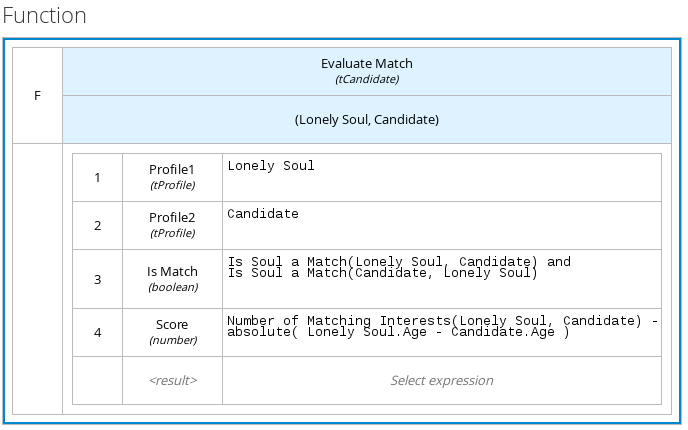
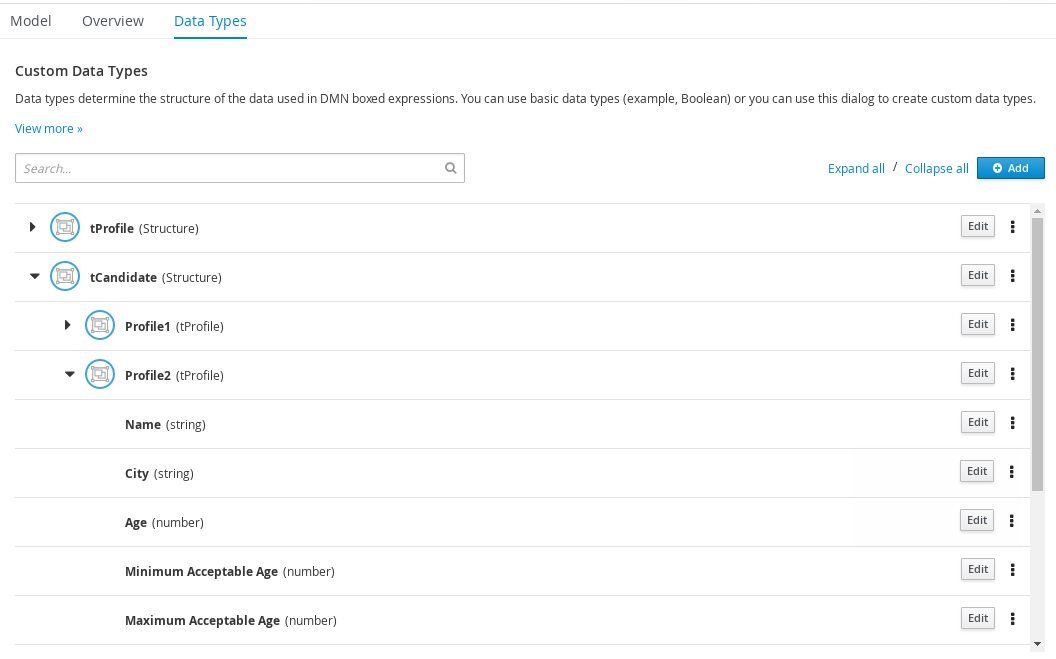
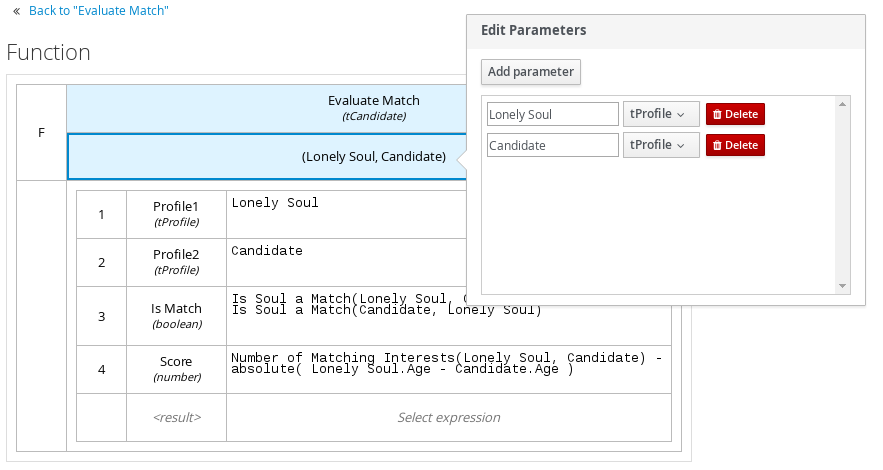
8.3.3. DMN designer navigation and properties in Business Central
The DMN designer provides the following additional features to help you navigate through the components and properties of decision requirements diagrams (DRDs).
- DMN file and diagram views
-
In the upper-left corner of the DMN designer, select the Project Explorer view to navigate between all DMN and other files or select the Decision Navigator view to navigate between the nodes and boxed expressions of a selected DRD:
Figure 136. Project Explorer viewFigure 137. Decision Navigator viewIn the upper-right corner of the DMN designer, select the Explore diagram icon to view an elevated preview of the selected DRD and to navigate between the nodes of the selected DRD:
Figure 138. Explore diagram view - DRD properties and design
-
In the upper-right corner of the DMN designer, select the Diagram properties icon to modify the identifying information, data types, and appearance of a selected DRD, DRD node, or boxed expression cell:
Figure 139. DRD node propertiesTo view the properties of the entire DRD, click the DRD canvas background instead of a specific node.
8.4. DMN model execution
You can create or import DMN files in your Drools project using Business Central or package the DMN files as part of your project knowledge JAR (KJAR) file without Business Central. After you implement your DMN files in your Drools project, you can execute the DMN decision service by deploying the KIE container that contains it to KIE Server for remote access or by manipulating the KIE container directly as a dependency of the calling application. Other options for creating and deploying DMN knowledge packages are also available, and most are similar for all types of knowledge assets, such as DRL files or process definitions.
For information about including external DMN assets with your project packaging and deployment method, see Build, Deploy, Utilize and Run.
8.4.1. Embedding a DMN call directly in a Java application
A KIE container is local when the knowledge assets are either embedded directly into the calling program or are physically pulled in using Maven dependencies for the KJAR. You typically embed knowledge assets directly into a project if there is a tight relationship between the version of the code and the version of the DMN definition. Any changes to the decision take effect after you have intentionally updated and redeployed the application. A benefit of this approach is that proper operation does not rely on any external dependencies to the run time, which can be a limitation of locked-down environments.
Using Maven dependencies enables further flexibility because the specific version of the decision can dynamically change, (for example, by using a system property), and it can be periodically scanned for updates and automatically updated. This introduces an external dependency on the deploy time of the service, but executes the decision locally, reducing reliance on an external service being available during run time.
-
A KIE container is deployed in KIE Server in the form of a KJAR that includes the DMN model, ideally compiled as an executable model for more efficient execution:
mvn clean install -DgenerateDMNModel=yes
For more information about project packaging and deployment and executable models, see Build, Deploy, Utilize and Run.
-
In your client application, add the following dependencies to the relevant classpath of your Java project:
<!-- Required for the DMN runtime API --> <dependency> <groupId>org.kie</groupId> <artifactId>kie-dmn-core</artifactId> <version>${drools.version}</version> </dependency> <!-- Required if not using classpath KIE container --> <dependency> <groupId>org.kie</groupId> <artifactId>kie-ci</artifactId> <version>${drools.version}</version> </dependency>
The
<version>
is the Maven artifact version for Drools currently used in your project (for example, 7.23.0.Final-redhat-00002). -
Create a KIE container from
classpath
orReleaseId
:KieServices kieServices = KieServices.Factory.get(); ReleaseId releaseId = kieServices.newReleaseId( "org.acme", "my-kjar", "1.0.0" ); KieContainer kieContainer = kieServices.newKieContainer( releaseId );
Alternative option:
KieServices kieServices = KieServices.Factory.get(); KieContainer kieContainer = kieServices.getKieClasspathContainer();
-
Obtain
DMNRuntime
from the KIE container and a reference to the DMN model to be evaluated, by using the modelnamespace
andmodelName
:DMNRuntime dmnRuntime = KieRuntimeFactory.of(kieContainer.getKieBase()).get(DMNRuntime.class); String namespace = "http://www.redhat.com/_c7328033-c355-43cd-b616-0aceef80e52a"; String modelName = "dmn-movieticket-ageclassification"; DMNModel dmnModel = dmnRuntime.getModel(namespace, modelName);
-
Execute the decision services for the desired model:
DMNContext dmnContext = dmnRuntime.newContext(); (1) for (Integer age : Arrays.asList(1,12,13,64,65,66)) { dmnContext.set("Age", age); (2) DMNResult dmnResult = dmnRuntime.evaluateAll(dmnModel, dmnContext); (3) for (DMNDecisionResult dr : dmnResult.getDecisionResults()) { (4) log.info("Age: " + age + ", " + "Decision: '" + dr.getDecisionName() + "', " + "Result: " + dr.getResult()); } }
1 Instantiate a new DMN Context to be the input for the model evaluation. Note that this example is looping through the Age Classification decision multiple times. 2 Assign input variables for the input DMN context. 3 Evaluate all DMN decisions defined in the DMN model. 4 Each evaluation may result in one or more results, creating the loop. This example prints the following output:
Age 1 Decision 'AgeClassification' : Child Age 12 Decision 'AgeClassification' : Child Age 13 Decision 'AgeClassification' : Adult Age 64 Decision 'AgeClassification' : Adult Age 65 Decision 'AgeClassification' : Senior Age 66 Decision 'AgeClassification' : Senior
If the DMN model was not previously compiled as an executable model for more efficient execution, you can enable the following property when you execute your DMN models:
-Dorg.kie.dmn.compiler.execmodel=true
8.4.2. Executing a DMN service using the KIE Server Java client API
The KIE Server Java client API provides a lightweight approach to invoking a remote DMN service either through the REST or JMS interfaces of KIE Server. This approach reduces the number of runtime dependencies necessary to interact with a KIE base. Decoupling the calling code from the decision definition also increases flexibility by enabling them to iterate independently at the appropriate pace.
For more information about the KIE Server Java client API, see KIE Server Java client API for KIE containers and business assets.
-
KIE Server is installed and configured, including a known user name and credentials for a user with the
kie-server
role. For installation options, see Installation and Setup (Core and IDE). -
A KIE container is deployed in KIE Server in the form of a KJAR that includes the DMN model, ideally compiled as an executable model for more efficient execution:
mvn clean install -DgenerateDMNModel=yes
For more information about project packaging and deployment and executable models, see Build, Deploy, Utilize and Run.
-
You have the container ID of the KIE container containing the DMN model. If more than one model is present, you must also know the model namespace and model name of the relevant model.
-
In your client application, add the following dependency to the relevant classpath of your Java project:
<!-- Required for the KIE Server Java client API --> <dependency> <groupId>org.kie.server</groupId> <artifactId>kie-server-client</artifactId> <version>${drools.version}</version> </dependency>
The
<version>
is the Maven artifact version for Drools currently used in your project (for example, 7.23.0.Final-redhat-00002). -
Instantiate a
KieServicesClient
instance with the appropriate connection information.Example:
KieServicesConfiguration conf = KieServicesFactory.newRestConfiguration(URL, USER, PASSWORD); (1) conf.setMarshallingFormat(MarshallingFormat.JSON); (2) KieServicesClient kieServicesClient = KieServicesFactory.newKieServicesClient(conf);
1 The connection information: -
Example URL:
http://localhost:8080/kie-server/services/rest/server
-
The credentials should reference a user with the
kie-server
role.
2 The Marshalling format is an instance of org.kie.server.api.marshalling.MarshallingFormat
. It controls whether the messages will be JSON or XML. Options for Marshalling format are JSON, JAXB, or XSTREAM. -
-
Obtain a
DMNServicesClient
from the KIE server Java client connected to the related KIE Server by invoking the methodgetServicesClient()
on the KIE server Java client instance:DMNServicesClient dmnClient = kieServicesClient.getServicesClient(DMNServicesClient.class );
The
dmnClient
can now execute decision services on KIE Server. -
Execute the decision services for the desired model.
Example:
for (Integer age : Arrays.asList(1,12,13,64,65,66)) { DMNContext dmnContext = dmnClient.newContext(); (1) dmnContext.set("Age", age); (2) ServiceResponse<DMNResult> serverResp = (3) dmnClient.evaluateAll($kieContainerId, $modelNamespace, $modelName, dmnContext); DMNResult dmnResult = serverResp.getResult(); (4) for (DMNDecisionResult dr : dmnResult.getDecisionResults()) { log.info("Age: " + age + ", " + "Decision: '" + dr.getDecisionName() + "', " + "Result: " + dr.getResult()); } }
1 Instantiate a new DMN Context to be the input for the model evaluation. Note that this example is looping through the Age Classification decision multiple times. 2 Assign input variables for the input DMN Context. 3 Evaluate all the DMN Decisions defined in the DMN model: -
$kieContainerId
is the ID of the container where the KJAR containing the DMN model is deployed -
$modelNamespace
is the namespace for the model. -
$modelName
is the name for the model.
4 The DMN Result object is available from the server response. At this point, the
dmnResult
contains all the decision results from the evaluated DMN model.You can also execute only a specific DMN decision in the model by using alternative methods of the
DMNServicesClient
.If the KIE container only contains one DMN model, you can omit $modelNamespace
and$modelName
because the KIE Server API selects it by default. -
8.4.3. Executing a DMN service using the KIE Server REST API
Directly interacting with the REST endpoints of KIE Server provides the most separation between the calling code and the decision logic definition. The calling code is completely free of direct dependencies, and you can implement it in an entirely different development platform such as node.js
or .net
. The examples in this section demonstrate Nix-style curl commands but provide relevant information to adapt to any REST client.
For more information about the KIE Server REST API, see KIE Server REST API for KIE containers and business assets.
-
KIE Server is installed and configured, including a known user name and credentials for a user with the
kie-server
role. For installation options, see Installation and Setup (Core and IDE). -
A KIE container is deployed in KIE Server in the form of a KJAR that includes the DMN model, ideally compiled as an executable model for more efficient execution:
mvn clean install -DgenerateDMNModel=yes
For more information about project packaging and deployment and executable models, see Build, Deploy, Utilize and Run.
-
You have the container ID of the KIE container containing the DMN model. If more than one model is present, you must also know the model namespace and model name of the relevant model.
-
Determine the base URL for accessing the KIE Server REST API endpoints. This requires knowing the following values (with the default local deployment values as an example):
-
Host (
localhost
) -
Port (
8080
) -
Root context (
kie-server
) -
Base REST path (
services/rest/
)
Example base URL in local deployment:
http://localhost:8080/kie-server/services/rest/
-
-
Determine user authentication requirements.
When users are defined directly in the KIE Server configuration, HTTP Basic authentication is used and requires the user name and password. Successful requests require that the user have the
kie-server
role.The following example demonstrates how to add credentials to a curl request:
curl -u username:password <request>
If KIE Server is configured with Red Hat Single Sign-On, the request must include a bearer token:
curl -H "Authorization: bearer $TOKEN" <request>
-
Specify the format of the request and response. The REST API endpoints work with both JSON and XML formats and are set using request headers:
JSONcurl -H "accept: application/json" -H "content-type: application/json"
XMLcurl -H "accept: application/xml" -H "content-type: application/xml"
-
(Optional) Query the container for a list of deployed decision models:
[GET]
server/containers/{containerId}/dmn
Example curl request:
curl -u krisv:krisv -H "accept: application/xml" -X GET "http://localhost:8080/kie-server/services/rest/server/containers/MovieDMNContainer/dmn"
Sample XML output:
<?xml version="1.0" encoding="UTF-8" standalone="yes"?> <response type="SUCCESS" msg="OK models successfully retrieved from container 'MovieDMNContainer'"> <dmn-model-info-list> <model> <model-namespace>http://www.redhat.com/_c7328033-c355-43cd-b616-0aceef80e52a</model-namespace> <model-name>dmn-movieticket-ageclassification</model-name> <model-id>_99</model-id> <decisions> <dmn-decision-info> <decision-id>_3</decision-id> <decision-name>AgeClassification</decision-name> </dmn-decision-info> </decisions> </model> </dmn-model-info-list> </response>
Sample JSON output:
{ "type" : "SUCCESS", "msg" : "OK models successfully retrieved from container 'MovieDMNContainer'", "result" : { "dmn-model-info-list" : { "models" : [ { "model-namespace" : "http://www.redhat.com/_c7328033-c355-43cd-b616-0aceef80e52a", "model-name" : "dmn-movieticket-ageclassification", "model-id" : "_99", "decisions" : [ { "decision-id" : "_3", "decision-name" : "AgeClassification" } ] } ] } } }
-
Execute the model:
[POST]
server/containers/{containerId}/dmn
Example curl request:
curl -u krisv:krisv -H "accept: application/json" -H "content-type: application/json" -X POST "http://localhost:8080/kie-server/services/rest/server/containers/MovieDMNContainer/dmn" -d "{ \"model-namespace\" : \"http://www.redhat.com/_c7328033-c355-43cd-b616-0aceef80e52a\", \"model-name\" : \"dmn-movieticket-ageclassification\", \"decision-name\" : [ ], \"decision-id\" : [ ], \"dmn-context\" : {\"Age\" : 66}}"
Example JSON request:
{ "model-namespace" : "http://www.redhat.com/_c7328033-c355-43cd-b616-0aceef80e52a", "model-name" : "dmn-movieticket-ageclassification", "decision-name" : [ ], "decision-id" : [ ], "dmn-context" : {"Age" : 66} }
Example XML request (JAXB format):
<?xml version="1.0" encoding="UTF-8"?> <dmn-evaluation-context> <model-namespace>http://www.redhat.com/_c7328033-c355-43cd-b616-0aceef80e52a</model-namespace> <model-name>dmn-movieticket-ageclassification</model-name> <dmn-context xsi:type="jaxbListWrapper" xmlns:xsi="http://www.w3.org/2001/XMLSchema-instance"> <type>MAP</type> <element xsi:type="jaxbStringObjectPair" key="Age"> <value xsi:type="xs:int" xmlns:xs="http://www.w3.org/2001/XMLSchema">66</value> </element> </dmn-context> </dmn-evaluation-context>
Regardless of the request format, the request requires the following elements:
-
Model namespace
-
Model name
-
Context object containing input values
Example JSON response:
{ "type" : "SUCCESS", "msg" : "OK from container 'MovieDMNContainer'", "result" : { "dmn-evaluation-result" : { "messages" : [ ], "model-namespace" : "http://www.redhat.com/_c7328033-c355-43cd-b616-0aceef80e52a", "model-name" : "dmn-movieticket-ageclassification", "decision-name" : [ ], "dmn-context" : { "Age" : 66, "AgeClassification" : "Senior" }, "decision-results" : { "_3" : { "messages" : [ ], "decision-id" : "_3", "decision-name" : "AgeClassification", "result" : "Senior", "status" : "SUCCEEDED" } } } } }
Example XML (JAXB format) response:
<?xml version="1.0" encoding="UTF-8" standalone="yes"?> <response type="SUCCESS" msg="OK from container 'MovieDMNContainer'"> <dmn-evaluation-result> <model-namespace>http://www.redhat.com/_c7328033-c355-43cd-b616-0aceef80e52a</model-namespace> <model-name>dmn-movieticket-ageclassification</model-name> <dmn-context xsi:type="jaxbListWrapper" xmlns:xsi="http://www.w3.org/2001/XMLSchema-instance"> <type>MAP</type> <element xsi:type="jaxbStringObjectPair" key="Age"> <value xsi:type="xs:int" xmlns:xs="http://www.w3.org/2001/XMLSchema">66</value> </element> <element xsi:type="jaxbStringObjectPair" key="AgeClassification"> <value xsi:type="xs:string" xmlns:xs="http://www.w3.org/2001/XMLSchema">Senior</value> </element> </dmn-context> <messages/> <decisionResults> <entry> <key>_3</key> <value> <decision-id>_3</decision-id> <decision-name>AgeClassification</decision-name> <result xsi:type="xs:string" xmlns:xs="http://www.w3.org/2001/XMLSchema" xmlns:xsi="http://www.w3.org/2001/XMLSchema-instance">Senior</result> <messages/> <status>SUCCEEDED</status> </value> </entry> </decisionResults> </dmn-evaluation-result> </response>
-
9. Predictive Model Markup Language (PMML)
9.1. Predictive Model Markup Language (PMML)
Predictive Model Markup Language (PMML) is an XML-based standard established by the Data Mining Group (DMG) for defining statistical and data-mining models. PMML models can be shared between PMML-compliant platforms and across organizations so that business analysts and developers are unified in designing, analyzing, and implementing PMML-based assets and services.
For more information about the background and applications of PMML, see the DMG PMML specification.
9.1.1. PMML conformance levels
The PMML specification defines producer and consumer conformance levels in a software implementation to ensure that PMML models are created and integrated reliably. For the formal definitions of each conformance level, see the DMG PMML conformance page.
The following are summaries of the PMML conformance levels:
- Producer conformance
-
A tool or application is producer conforming if it generates valid PMML documents for at least one type of model. Satisfying PMML producer conformance requirements ensures that a model definition document is syntactically correct and defines a model instance that is consistent with semantic criteria that are defined in model specifications.
- Consumer conformance
-
An application is consumer conforming if it accepts valid PMML documents for at least one type of model. Satisfying consumer conformance requirements ensures that a PMML model created according to producer conformance can be integrated and used as defined. For example, if an application is consumer conforming for Regression model types, then valid PMML documents defining models of this type produced by different conforming producers would be interchangeable in the application.
Drools includes consumer conformance support for the following PMML 4.2.1 model types:
-
Mining models (with sub-types
modelChain
,selectAll
, andselectFirst
)
For a list of all PMML model types, including those not supported in Drools, see the DMG PMML specification.
9.2. PMML model examples
PMML defines an XML schema that enables PMML models to be used between different PMML-compliant platforms. The PMML specification enables multiple software platforms to work with the same file for authoring, testing, and production execution, assuming producer and consumer conformance are met.
The following are examples of PMML Regression, Scorecard, Tree, and Mining models. These examples illustrate the supported types of models that you can integrate with your decision services in Drools.
For more PMML examples, see the DMG PMML Sample Files page.
<PMML version="4.2" xsi:schemaLocation="http://www.dmg.org/PMML-4_2 http://www.dmg.org/v4-2-1/pmml-4-2.xsd" xmlns:xsi="http://www.w3.org/2001/XMLSchema-instance" xmlns="http://www.dmg.org/PMML-4_2">
<Header copyright="JBoss"/>
<DataDictionary numberOfFields="5">
<DataField dataType="double" name="fld1" optype="continuous"/>
<DataField dataType="double" name="fld2" optype="continuous"/>
<DataField dataType="string" name="fld3" optype="categorical">
<Value value="x"/>
<Value value="y"/>
</DataField>
<DataField dataType="double" name="fld4" optype="continuous"/>
<DataField dataType="double" name="fld5" optype="continuous"/>
</DataDictionary>
<RegressionModel algorithmName="linearRegression" functionName="regression" modelName="LinReg" normalizationMethod="logit" targetFieldName="fld4">
<MiningSchema>
<MiningField name="fld1"/>
<MiningField name="fld2"/>
<MiningField name="fld3"/>
<MiningField name="fld4" usageType="predicted"/>
<MiningField name="fld5" usageType="target"/>
</MiningSchema>
<RegressionTable intercept="0.5">
<NumericPredictor coefficient="5" exponent="2" name="fld1"/>
<NumericPredictor coefficient="2" exponent="1" name="fld2"/>
<CategoricalPredictor coefficient="-3" name="fld3" value="x"/>
<CategoricalPredictor coefficient="3" name="fld3" value="y"/>
<PredictorTerm coefficient="0.4">
<FieldRef field="fld1"/>
<FieldRef field="fld2"/>
</PredictorTerm>
</RegressionTable>
</RegressionModel>
</PMML>
<PMML version="4.2" xsi:schemaLocation="http://www.dmg.org/PMML-4_2 http://www.dmg.org/v4-2-1/pmml-4-2.xsd" xmlns:xsi="http://www.w3.org/2001/XMLSchema-instance" xmlns="http://www.dmg.org/PMML-4_2">
<Header copyright="JBoss"/>
<DataDictionary numberOfFields="4">
<DataField name="param1" optype="continuous" dataType="double"/>
<DataField name="param2" optype="continuous" dataType="double"/>
<DataField name="overallScore" optype="continuous" dataType="double" />
<DataField name="finalscore" optype="continuous" dataType="double" />
</DataDictionary>
<Scorecard modelName="ScorecardCompoundPredicate" useReasonCodes="true" isScorable="true" functionName="regression" baselineScore="15" initialScore="0.8" reasonCodeAlgorithm="pointsAbove">
<MiningSchema>
<MiningField name="param1" usageType="active" invalidValueTreatment="asMissing">
</MiningField>
<MiningField name="param2" usageType="active" invalidValueTreatment="asMissing">
</MiningField>
<MiningField name="overallScore" usageType="target"/>
<MiningField name="finalscore" usageType="predicted"/>
</MiningSchema>
<Characteristics>
<Characteristic name="ch1" baselineScore="50" reasonCode="reasonCh1">
<Attribute partialScore="20">
<SimplePredicate field="param1" operator="lessThan" value="20"/>
</Attribute>
<Attribute partialScore="100">
<CompoundPredicate booleanOperator="and">
<SimplePredicate field="param1" operator="greaterOrEqual" value="20"/>
<SimplePredicate field="param2" operator="lessOrEqual" value="25"/>
</CompoundPredicate>
</Attribute>
<Attribute partialScore="200">
<CompoundPredicate booleanOperator="and">
<SimplePredicate field="param1" operator="greaterOrEqual" value="20"/>
<SimplePredicate field="param2" operator="greaterThan" value="25"/>
</CompoundPredicate>
</Attribute>
</Characteristic>
<Characteristic name="ch2" reasonCode="reasonCh2">
<Attribute partialScore="10">
<CompoundPredicate booleanOperator="or">
<SimplePredicate field="param2" operator="lessOrEqual" value="-5"/>
<SimplePredicate field="param2" operator="greaterOrEqual" value="50"/>
</CompoundPredicate>
</Attribute>
<Attribute partialScore="20">
<CompoundPredicate booleanOperator="and">
<SimplePredicate field="param2" operator="greaterThan" value="-5"/>
<SimplePredicate field="param2" operator="lessThan" value="50"/>
</CompoundPredicate>
</Attribute>
</Characteristic>
</Characteristics>
</Scorecard>
</PMML>
<PMML version="4.2" xsi:schemaLocation="http://www.dmg.org/PMML-4_2 http://www.dmg.org/v4-2-1/pmml-4-2.xsd" xmlns:xsi="http://www.w3.org/2001/XMLSchema-instance" xmlns="http://www.dmg.org/PMML-4_2">
<Header copyright="JBOSS"/>
<DataDictionary numberOfFields="5">
<DataField dataType="double" name="fld1" optype="continuous"/>
<DataField dataType="double" name="fld2" optype="continuous"/>
<DataField dataType="string" name="fld3" optype="categorical">
<Value value="true"/>
<Value value="false"/>
</DataField>
<DataField dataType="string" name="fld4" optype="categorical">
<Value value="optA"/>
<Value value="optB"/>
<Value value="optC"/>
</DataField>
<DataField dataType="string" name="fld5" optype="categorical">
<Value value="tgtX"/>
<Value value="tgtY"/>
<Value value="tgtZ"/>
</DataField>
</DataDictionary>
<TreeModel functionName="classification" modelName="TreeTest">
<MiningSchema>
<MiningField name="fld1"/>
<MiningField name="fld2"/>
<MiningField name="fld3"/>
<MiningField name="fld4"/>
<MiningField name="fld5" usageType="predicted"/>
</MiningSchema>
<Node score="tgtX">
<True/>
<Node score="tgtX">
<SimplePredicate field="fld4" operator="equal" value="optA"/>
<Node score="tgtX">
<CompoundPredicate booleanOperator="surrogate">
<SimplePredicate field="fld1" operator="lessThan" value="30.0"/>
<SimplePredicate field="fld2" operator="greaterThan" value="20.0"/>
</CompoundPredicate>
<Node score="tgtX">
<SimplePredicate field="fld2" operator="lessThan" value="40.0"/>
</Node>
<Node score="tgtZ">
<SimplePredicate field="fld2" operator="greaterOrEqual" value="10.0"/>
</Node>
</Node>
<Node score="tgtZ">
<CompoundPredicate booleanOperator="or">
<SimplePredicate field="fld1" operator="greaterOrEqual" value="60.0"/>
<SimplePredicate field="fld1" operator="lessOrEqual" value="70.0"/>
</CompoundPredicate>
<Node score="tgtZ">
<SimpleSetPredicate booleanOperator="isNotIn" field="fld4">
<Array type="string">optA optB</Array>
</SimpleSetPredicate>
</Node>
</Node>
</Node>
<Node score="tgtY">
<CompoundPredicate booleanOperator="or">
<SimplePredicate field="fld4" operator="equal" value="optA"/>
<SimplePredicate field="fld4" operator="equal" value="optC"/>
</CompoundPredicate>
<Node score="tgtY">
<CompoundPredicate booleanOperator="and">
<SimplePredicate field="fld1" operator="greaterThan" value="10.0"/>
<SimplePredicate field="fld1" operator="lessThan" value="50.0"/>
<SimplePredicate field="fld4" operator="equal" value="optA"/>
<SimplePredicate field="fld2" operator="lessThan" value="100.0"/>
<SimplePredicate field="fld3" operator="equal" value="false"/>
</CompoundPredicate>
</Node>
<Node score="tgtZ">
<CompoundPredicate booleanOperator="and">
<SimplePredicate field="fld4" operator="equal" value="optC"/>
<SimplePredicate field="fld2" operator="lessThan" value="30.0"/>
</CompoundPredicate>
</Node>
</Node>
</Node>
</TreeModel>
</PMML>
<PMML version="4.2" xsi:schemaLocation="http://www.dmg.org/PMML-4_2 http://www.dmg.org/v4-2-1/pmml-4-2.xsd" xmlns:xsi="http://www.w3.org/2001/XMLSchema-instance" xmlns="http://www.dmg.org/PMML-4_2">
<Header>
<Application name="Drools-PMML" version="7.0.0-SNAPSHOT" />
</Header>
<DataDictionary numberOfFields="7">
<DataField name="age" optype="continuous" dataType="double" />
<DataField name="occupation" optype="categorical" dataType="string">
<Value value="SKYDIVER" />
<Value value="ASTRONAUT" />
<Value value="PROGRAMMER" />
<Value value="TEACHER" />
<Value value="INSTRUCTOR" />
</DataField>
<DataField name="residenceState" optype="categorical" dataType="string">
<Value value="AP" />
<Value value="KN" />
<Value value="TN" />
</DataField>
<DataField name="validLicense" optype="categorical" dataType="boolean" />
<DataField name="overallScore" optype="continuous" dataType="double" />
<DataField name="grade" optype="categorical" dataType="string">
<Value value="A" />
<Value value="B" />
<Value value="C" />
<Value value="D" />
<Value value="F" />
</DataField>
<DataField name="qualificationLevel" optype="categorical" dataType="string">
<Value value="Unqualified" />
<Value value="Barely" />
<Value value="Well" />
<Value value="Over" />
</DataField>
</DataDictionary>
<MiningModel modelName="SampleModelChainMine" functionName="classification">
<MiningSchema>
<MiningField name="age" />
<MiningField name="occupation" />
<MiningField name="residenceState" />
<MiningField name="validLicense" />
<MiningField name="overallScore" />
<MiningField name="qualificationLevel" usageType="target"/>
</MiningSchema>
<Segmentation multipleModelMethod="modelChain">
<Segment id="1">
<True />
<Scorecard modelName="Sample Score 1" useReasonCodes="true" isScorable="true" functionName="regression" baselineScore="0.0" initialScore="0.345">
<MiningSchema>
<MiningField name="age" usageType="active" invalidValueTreatment="asMissing" />
<MiningField name="occupation" usageType="active" invalidValueTreatment="asMissing" />
<MiningField name="residenceState" usageType="active" invalidValueTreatment="asMissing" />
<MiningField name="validLicense" usageType="active" invalidValueTreatment="asMissing" />
<MiningField name="overallScore" usageType="predicted" />
</MiningSchema>
<Output>
<OutputField name="calculatedScore" displayName="Final Score" dataType="double" feature="predictedValue" targetField="overallScore" />
</Output>
<Characteristics>
<Characteristic name="AgeScore" baselineScore="0.0" reasonCode="ABZ">
<Extension name="cellRef" value="$B$8" />
<Attribute partialScore="10.0">
<Extension name="cellRef" value="$C$10" />
<SimplePredicate field="age" operator="lessOrEqual" value="5" />
</Attribute>
<Attribute partialScore="30.0" reasonCode="CX1">
<Extension name="cellRef" value="$C$11" />
<CompoundPredicate booleanOperator="and">
<SimplePredicate field="age" operator="greaterOrEqual" value="5" />
<SimplePredicate field="age" operator="lessThan" value="12" />
</CompoundPredicate>
</Attribute>
<Attribute partialScore="40.0" reasonCode="CX2">
<Extension name="cellRef" value="$C$12" />
<CompoundPredicate booleanOperator="and">
<SimplePredicate field="age" operator="greaterOrEqual" value="13" />
<SimplePredicate field="age" operator="lessThan" value="44" />
</CompoundPredicate>
</Attribute>
<Attribute partialScore="25.0">
<Extension name="cellRef" value="$C$13" />
<SimplePredicate field="age" operator="greaterOrEqual" value="45" />
</Attribute>
</Characteristic>
<Characteristic name="OccupationScore" baselineScore="0.0">
<Extension name="cellRef" value="$B$16" />
<Attribute partialScore="-10.0" reasonCode="CX2">
<Extension name="description" value="skydiving is a risky occupation" />
<Extension name="cellRef" value="$C$18" />
<SimpleSetPredicate field="occupation" booleanOperator="isIn">
<Array n="2" type="string">SKYDIVER ASTRONAUT</Array>
</SimpleSetPredicate>
</Attribute>
<Attribute partialScore="10.0">
<Extension name="cellRef" value="$C$19" />
<SimpleSetPredicate field="occupation" booleanOperator="isIn">
<Array n="2" type="string">TEACHER INSTRUCTOR</Array>
</SimpleSetPredicate>
</Attribute>
<Attribute partialScore="5.0">
<Extension name="cellRef" value="$C$20" />
<SimplePredicate field="occupation" operator="equal" value="PROGRAMMER" />
</Attribute>
</Characteristic>
<Characteristic name="ResidenceStateScore" baselineScore="0.0" reasonCode="RES">
<Extension name="cellRef" value="$B$22" />
<Attribute partialScore="-10.0">
<Extension name="cellRef" value="$C$24" />
<SimplePredicate field="residenceState" operator="equal" value="AP" />
</Attribute>
<Attribute partialScore="10.0">
<Extension name="cellRef" value="$C$25" />
<SimplePredicate field="residenceState" operator="equal" value="KN" />
</Attribute>
<Attribute partialScore="5.0">
<Extension name="cellRef" value="$C$26" />
<SimplePredicate field="residenceState" operator="equal" value="TN" />
</Attribute>
</Characteristic>
<Characteristic name="ValidLicenseScore" baselineScore="0.0">
<Extension name="cellRef" value="$B$28" />
<Attribute partialScore="1.0" reasonCode="LX00">
<Extension name="cellRef" value="$C$30" />
<SimplePredicate field="validLicense" operator="equal" value="true" />
</Attribute>
<Attribute partialScore="-1.0" reasonCode="LX00">
<Extension name="cellRef" value="$C$31" />
<SimplePredicate field="validLicense" operator="equal" value="false" />
</Attribute>
</Characteristic>
</Characteristics>
</Scorecard>
</Segment>
<Segment id="2">
<True />
<TreeModel modelName="SampleTree" functionName="classification" missingValueStrategy="lastPrediction" noTrueChildStrategy="returnLastPrediction">
<MiningSchema>
<MiningField name="age" usageType="active" />
<MiningField name="validLicense" usageType="active" />
<MiningField name="calculatedScore" usageType="active" />
<MiningField name="qualificationLevel" usageType="predicted" />
</MiningSchema>
<Output>
<OutputField name="qualification" displayName="Qualification Level" dataType="string" feature="predictedValue" targetField="qualificationLevel" />
</Output>
<Node score="Well" id="1">
<True/>
<Node score="Barely" id="2">
<CompoundPredicate booleanOperator="and">
<SimplePredicate field="age" operator="greaterOrEqual" value="16" />
<SimplePredicate field="validLicense" operator="equal" value="true" />
</CompoundPredicate>
<Node score="Barely" id="3">
<SimplePredicate field="calculatedScore" operator="lessOrEqual" value="50.0" />
</Node>
<Node score="Well" id="4">
<CompoundPredicate booleanOperator="and">
<SimplePredicate field="calculatedScore" operator="greaterThan" value="50.0" />
<SimplePredicate field="calculatedScore" operator="lessOrEqual" value="60.0" />
</CompoundPredicate>
</Node>
<Node score="Over" id="5">
<SimplePredicate field="calculatedScore" operator="greaterThan" value="60.0" />
</Node>
</Node>
<Node score="Unqualified" id="6">
<CompoundPredicate booleanOperator="surrogate">
<SimplePredicate field="age" operator="lessThan" value="16" />
<SimplePredicate field="calculatedScore" operator="lessOrEqual" value="40.0" />
<True />
</CompoundPredicate>
</Node>
</Node>
</TreeModel>
</Segment>
</Segmentation>
</MiningModel>
</PMML>
9.3. PMML support in Drools
Drools includes consumer conformance support for the following PMML 4.2.1 model types:
-
Mining models (with sub-types
modelChain
,selectAll
, andselectFirst
)
For a list of all PMML model types, including those not supported in Drools, see the DMG PMML specification.
Drools does not include a built-in PMML model editor, but you can use an XML or PMML-specific authoring tool to create PMML models and then integrate the PMML models in your decision services in Drools. You can import PMML files into your project in Business Central (Menu → Design → Projects → Import Asset) or package the PMML files as part of your project knowledge JAR (KJAR) file without Business Central.
When you add a PMML file to a project in Drools, multiple assets are generated. Each type of PMML model generates a different set of assets, but all PMML model types generate at least the following set of assets:
-
A DRL file that contains all of the rules associated with your PMML model
-
At least two Java classes:
-
A data class that is used as the default object type for the model type
-
A
RuleUnit
class that is used to manage data sources and rule execution
-
If a PMML file has MiningModel
as the root model, multiple instances of each of these files are generated.
For more information about including assets such as PMML files with your project packaging and deployment method, see Build, Deploy, Utilize and Run.
9.3.1. PMML naming conventions in Drools
The following are naming conventions for generated PMML packages, classes, and rules:
-
If no package name is given in a PMML model file, then the default package name
org.kie.pmml.pmml_4_2
is prefixed to the model name for the generated rules in the format"org.kie.pmml.pmml_4_2"+modelName
. -
The package name for the generated
RuleUnit
Java class is the same as the package name for the generated rules. -
The name of the generated
RuleUnit
Java class is the model name withRuleUnit
added to it in the formatmodelName+"RuleUnit"
. -
Each PMML model has at least one data class that is generated. The package name for these classes is
org.kie.pmml.pmml_4_2.model
. -
The names of generated data classes are determined by the model type, prefixed with the model name:
-
Regression models: One data class named
modelName+"RegressionData"
-
Scorecard models: One data class named
modelName+"ScoreCardData"
-
Tree models: Two data classes, the first named
modelName+"TreeNode"
and the second namedmodelName+"TreeToken"
-
Mining models: One data class named
modelName+"MiningModelData"
-
The mining model also generates all of the rules and classes that are within each of its segments. |
9.3.2. PMML extensions in Drools
The PMML specification supports Extension
elements that extend the content of a PMML model. You can use extensions at almost every level of a PMML model definition, and as the first and last child in the main element of a model for maximum flexibility. For more information about PMML extensions, see the DMG PMML Extension Mechanism.
To optimize PMML integration, Drools supports the following additional PMML extensions:
-
modelPackage
: Designates a package name for the generated rules and Java classes. Include this extension in theHeader
section of the PMML model file. -
adapter
: Designates the type of construct (bean
ortrait
) that is used to contain input and output data for rules. Insert this extension in theMiningSchema
orOutput
section (or both) of the PMML model file. -
externalClass
: Used in conjunction with theadapter
extension in defining aMiningField
orOutputField
. This extension contains a class with an attribute name that matches the name of theMiningField
orOutputField
element.
9.4. PMML model execution
You can import PMML files into your Drools project using Business Central (Menu → Design → Projects → Import Asset) or package the PMML files as part of your project knowledge JAR (KJAR) file without Business Central. After you implement your PMML files in your Drools project, you can execute the PMML-based decision service by embedding PMML calls directly in your Java application or by sending an ApplyPmmlModelCommand
command to a configured KIE Server.
For more information about including PMML assets with your project packaging and deployment method, see Build, Deploy, Utilize and Run.
9.4.1. Embedding a PMML call directly in a Java application
A KIE container is local when the knowledge assets are either embedded directly into the calling program or are physically pulled in using Maven dependencies for the KJAR. You typically embed knowledge assets directly into a project if there is a tight relationship between the version of the code and the version of the PMML definition. Any changes to the decision take effect after you have intentionally updated and redeployed the application. A benefit of this approach is that proper operation does not rely on any external dependencies to the run time, which can be a limitation of locked-down environments.
Using Maven dependencies enables further flexibility because the specific version of the decision can dynamically change (for example, by using a system property), and it can be periodically scanned for updates and automatically updated. This introduces an external dependency on the deploy time of the service, but executes the decision locally, reducing reliance on an external service being available during run time.
-
A KJAR containing the PMML model to execute has been created. For more information about project packaging, see Build, Deploy, Utilize and Run.
-
In your client application, add the following dependencies to the relevant classpath of your Java project:
<!-- Required for the PMML compiler --> <dependency> <groupId>org.drools</groupId> <artifactId>kie-pmml</artifactId> <version>${drools.version}</version> </dependency> <!-- Required for the KIE public API --> <dependency> <groupId>org.kie</groupId> <artifactId>kie-api</artifactId> <version>${drools.version}</version> </dependencies> <!-- Required if not using classpath KIE container --> <dependency> <groupId>org.kie</groupId> <artifactId>kie-ci</artifactId> <version>${drools.version}</version> </dependency>
The
<version>
is the Maven artifact version for Drools currently used in your project (for example, 7.23.0.Final-redhat-00002). -
Create a KIE container from
classpath
orReleaseId
:KieServices kieServices = KieServices.Factory.get(); ReleaseId releaseId = kieServices.newReleaseId( "org.acme", "my-kjar", "1.0.0" ); KieContainer kieContainer = kieServices.newKieContainer( releaseId );
Alternative option:
KieServices kieServices = KieServices.Factory.get(); KieContainer kieContainer = kieServices.getKieClasspathContainer();
-
Create an instance of the
PMMLRequestData
class, which applies your PMML model to a set of data:public class PMMLRequestData { private String correlationId; (1) private String modelName; (2) private String source; (3) private List<ParameterInfo<?>> requestParams; (4) ... }
1 Identifies data that is associated with a particular request or result 2 The name of the model that should be applied to the request data 3 Used by internally generated PMMLRequestData
objects to identify the segment that generated the request4 The default mechanism for sending input data points -
Create an instance of the
PMML4Result
class, which holds the output information that is the result of applying the PMML-based rules to the input data:public class PMML4Result { private String correlationId; private String segmentationId; (1) private String segmentId; (2) private int segmentIndex; (3) private String resultCode; (4) private Map<String, Object> resultVariables; (5) ... }
1 Used when the model type is MiningModel
. ThesegmentationId
is used to differentiate between multiple segmentations.2 Used in conjunction with the segmentationId
to identify which segment generated the results.3 Used to maintain the order of segments. 4 Used to determine whether the model was successfully applied, where OK
indicates success.5 Contains the name of a resultant variable and its associated value. In addition to the normal getter methods, the
PMML4Result
class also supports the following methods for directly retrieving the values for result variables:public <T> Optional<T> getResultValue(String objName, String objField, Class<T> clazz, Object...params) public Object getResultValue(String objName, String objField, Object...params)
-
Create an instance of the
ParameterInfo
class, which serves as a wrapper for basic data type objects used as part of thePMMLRequestData
class:public class ParameterInfo<T> { (1) private String correlationId; private String name; (2) private String capitalizedName; private Class<T> type; (3) private T value; (4) ... }
1 The parameterized class to handle many different types 2 The name of the variable that is expected as input for the model 3 The class that is the actual type of the variable 4 The actual value of the variable -
Execute the PMML model based on the required PMML class instances that you have created:
public void executeModel(KieBase kbase, Map<String,Object> variables, String modelName, String correlationId, String modelPkgName) { RuleUnitExecutor executor = RuleUnitExecutor.create().bind(kbase); PMMLRequestData request = new PMMLRequestData(correlationId, modelName); PMML4Result resultHolder = new PMML4Result(correlationId); variables.entrySet().forEach( es -> { request.addRequestParam(es.getKey(), es.getValue()); }); DataSource<PMMLRequestData> requestData = executor.newDataSource("request"); DataSource<PMML4Result> resultData = executor.newDataSource("results"); DataSource<PMMLData> internalData = executor.newDataSource("pmmlData"); requestData.insert(request); resultData.insert(resultHolder); List<String> possiblePackageNames = calculatePossiblePackageNames(modelName, modelPkgName); Class<? extends RuleUnit> ruleUnitClass = getStartingRuleUnit("RuleUnitIndicator", (InternalKnowledgeBase)kbase, possiblePackageNames); if (ruleUnitClass != null) { executor.run(ruleUnitClass); if ( "OK".equals(resultHolder.getResultCode()) ) { // extract result variables here } } } protected Class<? extends RuleUnit> getStartingRuleUnit(String startingRule, InternalKnowledgeBase ikb, List<String> possiblePackages) { RuleUnitRegistry unitRegistry = ikb.getRuleUnitRegistry(); Map<String,InternalKnowledgePackage> pkgs = ikb.getPackagesMap(); RuleImpl ruleImpl = null; for (String pkgName: possiblePackages) { if (pkgs.containsKey(pkgName)) { InternalKnowledgePackage pkg = pkgs.get(pkgName); ruleImpl = pkg.getRule(startingRule); if (ruleImpl != null) { RuleUnitDescr descr = unitRegistry.getRuleUnitFor(ruleImpl).orElse(null); if (descr != null) { return descr.getRuleUnitClass(); } } } } return null; } protected List<String> calculatePossiblePackageNames(String modelId, String...knownPackageNames) { List<String> packageNames = new ArrayList<>(); String javaModelId = modelId.replaceAll("\\s",""); if (knownPackageNames != null && knownPackageNames.length > 0) { for (String knownPkgName: knownPackageNames) { packageNames.add(knownPkgName + "." + javaModelId); } } String basePkgName = PMML4UnitImpl.DEFAULT_ROOT_PACKAGE+"."+javaModelId; packageNames.add(basePkgName); return packageNames; }
Rules are executed by the
RuleUnitExecutor
class. TheRuleUnitExecutor
class creates KIE sessions and adds the requiredDataSource
objects to those sessions, and then executes the rules based on theRuleUnit
that is passed as a parameter to therun()
method. ThecalculatePossiblePackageNames
and thegetStartingRuleUnit
methods determine the fully qualified name of theRuleUnit
class that is passed to therun()
method.
To facilitate your PMML model execution, you can also use a PMML4ExecutionHelper
class supported in Drools. For more information about the PMML helper class, see PMML execution helper class.
9.4.1.1. PMML execution helper class
Drools provides a PMML4ExecutionHelper
class that helps create the PMMLRequestData
class required for PMML model execution and that helps execute rules using the RuleUnitExecutor
class.
The following are examples of a PMML model execution without and with the PMML4ExecutionHelper
class, as a comparison:
PMML4ExecutionHelper
public void executeModel(KieBase kbase,
Map<String,Object> variables,
String modelName,
String correlationId,
String modelPkgName) {
RuleUnitExecutor executor = RuleUnitExecutor.create().bind(kbase);
PMMLRequestData request = new PMMLRequestData(correlationId, modelName);
PMML4Result resultHolder = new PMML4Result(correlationId);
variables.entrySet().forEach( es -> {
request.addRequestParam(es.getKey(), es.getValue());
});
DataSource<PMMLRequestData> requestData = executor.newDataSource("request");
DataSource<PMML4Result> resultData = executor.newDataSource("results");
DataSource<PMMLData> internalData = executor.newDataSource("pmmlData");
requestData.insert(request);
resultData.insert(resultHolder);
List<String> possiblePackageNames = calculatePossiblePackageNames(modelName,
modelPkgName);
Class<? extends RuleUnit> ruleUnitClass = getStartingRuleUnit("RuleUnitIndicator",
(InternalKnowledgeBase)kbase,
possiblePackageNames);
if (ruleUnitClass != null) {
executor.run(ruleUnitClass);
if ( "OK".equals(resultHolder.getResultCode()) ) {
// extract result variables here
}
}
}
protected Class<? extends RuleUnit> getStartingRuleUnit(String startingRule, InternalKnowledgeBase ikb, List<String> possiblePackages) {
RuleUnitRegistry unitRegistry = ikb.getRuleUnitRegistry();
Map<String,InternalKnowledgePackage> pkgs = ikb.getPackagesMap();
RuleImpl ruleImpl = null;
for (String pkgName: possiblePackages) {
if (pkgs.containsKey(pkgName)) {
InternalKnowledgePackage pkg = pkgs.get(pkgName);
ruleImpl = pkg.getRule(startingRule);
if (ruleImpl != null) {
RuleUnitDescr descr = unitRegistry.getRuleUnitFor(ruleImpl).orElse(null);
if (descr != null) {
return descr.getRuleUnitClass();
}
}
}
}
return null;
}
protected List<String> calculatePossiblePackageNames(String modelId, String...knownPackageNames) {
List<String> packageNames = new ArrayList<>();
String javaModelId = modelId.replaceAll("\\s","");
if (knownPackageNames != null && knownPackageNames.length > 0) {
for (String knownPkgName: knownPackageNames) {
packageNames.add(knownPkgName + "." + javaModelId);
}
}
String basePkgName = PMML4UnitImpl.DEFAULT_ROOT_PACKAGE+"."+javaModelId;
packageNames.add(basePkgName);
return packageNames;
}
PMML4ExecutionHelper
public void executeModel(KieBase kbase,
Map<String,Object> variables,
String modelName,
String modelPkgName,
String correlationId) {
PMML4ExecutionHelper helper = PMML4ExecutionHelperFactory.getExecutionHelper(modelName, kbase);
helper.addPossiblePackageName(modelPkgName);
PMMLRequestData request = new PMMLRequestData(correlationId, modelName);
variables.entrySet().forEach(entry -> {
request.addRequestParam(entry.getKey(), entry.getValue);
});
PMML4Result resultHolder = helper.submitRequest(request);
if ("OK".equals(resultHolder.getResultCode)) {
// extract result variables here
}
}
When you use the PMML4ExecutionHelper
, you do not need to specify the possible package names nor the RuleUnit
class as you would in a typical PMML model execution.
To construct a PMML4ExecutionHelper
class, you use the PMML4ExecutionHelperFactory
class to determine how instances of PMML4ExecutionHelper
are retrieved.
The following are the available PMML4ExecutionHelperFactory
class methods for constructing a PMML4ExecutionHelper
class:
- PMML4ExecutionHelperFactory methods for PMML assets in a KIE base
-
Use these methods when PMML assets have already been compiled and are being used from an existing KIE base:
public static PMML4ExecutionHelper getExecutionHelper(String modelName, KieBase kbase) public static PMML4ExecutionHelper getExecutionHelper(String modelName, KieBase kbase, boolean includeMiningDataSources)
- PMML4ExecutionHelperFactory methods for PMML assets on the project classpath
-
Use these methods when PMML assets are on the project classpath. The
classPath
argument is the project classpath location of the PMML file:public static PMML4ExecutionHelper getExecutionHelper(String modelName, String classPath, KieBaseConfiguration kieBaseConf) public static PMML4ExecutionHelper getExecutionHelper(String modelName,String classPath, KieBaseConfiguration kieBaseConf, boolean includeMiningDataSources)
- PMML4ExecutionHelperFactory methods for PMML assets in a byte array
-
Use these methods when PMML assets are in the form of a byte array:
public static PMML4ExecutionHelper getExecutionHelper(String modelName, byte[] content, KieBaseConfiguration kieBaseConf) public static PMML4ExecutionHelper getExecutionHelper(String modelName, byte[] content, KieBaseConfiguration kieBaseConf, boolean includeMiningDataSources)
- PMML4ExecutionHelperFactory methods for PMML assets in a
Resource
-
Use these methods when PMML assets are in the form of an
org.kie.api.io.Resource
object:public static PMML4ExecutionHelper getExecutionHelper(String modelName, Resource resource, KieBaseConfiguration kieBaseConf) public static PMML4ExecutionHelper getExecutionHelper(String modelName, Resource resource, KieBaseConfiguration kieBaseConf, boolean includeMiningDataSources)
The classpath, byte array, and resource PMML4ExecutionHelperFactory methods create a KIE container for the generated rules and Java classes. The container is used as the source of the KIE base that the RuleUnitExecutor uses. The container is not persisted. The PMML4ExecutionHelperFactory method for PMML assets that are already in a KIE base does not create a KIE container in this way.
|
9.4.2. Executing a PMML model using KIE Server
You can execute PMML models that have been deployed to KIE Server by sending the ApplyPmmlModelCommand
command to the configured KIE Server. When you use this command, a PMMLRequestData
object is sent to the KIE Server and a PMML4Result
result object is received as a reply. You can send PMML requests to KIE Server through the KIE Server REST API from a configured Java class or directly from a REST client.
-
KIE Server is installed and configured, including a known user name and credentials for a user with the
kie-server
role. For installation options, see Installation and Setup (Core and IDE). -
A KIE container is deployed in KIE Server in the form of a KJAR that includes the PMML model. For more information about project packaging, see Build, Deploy, Utilize and Run.
-
You have the container ID of the KIE container containing the PMML model.
-
In your client application, add the following dependencies to the relevant classpath of your Java project:
<!-- Required for the PMML compiler --> <dependency> <groupId>org.drools</groupId> <artifactId>kie-pmml</artifactId> <version>${drools.version}</version> </dependency> <!-- Required for the KIE public API --> <dependency> <groupId>org.kie</groupId> <artifactId>kie-api</artifactId> <version>${drools.version}</version> </dependencies> <!-- Required for the KIE Server Java client API --> <dependency> <groupId>org.kie.server</groupId> <artifactId>kie-server-client</artifactId> <version>${drools.version}</version> </dependency> <!-- Required if not using classpath KIE container --> <dependency> <groupId>org.kie</groupId> <artifactId>kie-ci</artifactId> <version>${drools.version}</version> </dependency>
The
<version>
is the Maven artifact version for Drools currently used in your project (for example, 7.23.0.Final-redhat-00002). -
Create a KIE container from
classpath
orReleaseId
:KieServices kieServices = KieServices.Factory.get(); ReleaseId releaseId = kieServices.newReleaseId( "org.acme", "my-kjar", "1.0.0" ); KieContainer kieContainer = kieServices.newKieContainer( releaseId );
Alternative option:
KieServices kieServices = KieServices.Factory.get(); KieContainer kieContainer = kieServices.getKieClasspathContainer();
-
Create a class for sending requests to KIE Server and receiving responses:
public class ApplyScorecardModel { private static final ReleaseId releaseId = new ReleaseId("org.acme","my-kjar","1.0.0"); private static final String containerId = "SampleModelContainer"; private static KieCommands commandFactory; private static ClassLoader kjarClassLoader; (1) private RuleServicesClient serviceClient; (2) // Attributes specific to your class instance private String rankedFirstCode; private Double score; // Initialization of non-final static attributes static { commandFactory = KieServices.Factory.get().getCommands(); // Specifications for kjarClassLoader, if used KieMavenRepository kmp = KieMavenRepository.getMavenRepository(); File artifactFile = kmp.resolveArtifact(releaseId).getFile(); if (artifactFile != null) { URL urls[] = new URL[1]; try { urls[0] = artifactFile.toURI().toURL(); classLoader = new KieURLClassLoader(urls,PMML4Result.class.getClassLoader()); } catch (MalformedURLException e) { logger.error("Error getting classLoader for "+containerId); logger.error(e.getMessage()); } } else { logger.warn("Did not find the artifact file for "+releaseId.toString()); } } public ApplyScorecardModel(KieServicesConfiguration kieConfig) { KieServicesClient clientFactory = KieServicesFactory.newKieServicesClient(kieConfig); serviceClient = clientFactory.getServicesClient(RuleServicesClient.class); } ... // Getters and setters ... // Method for executing the PMML model on KIE Server public void applyModel(String occupation, int age) { PMMLRequestData input = new PMMLRequestData("1234","SampleModelName"); (3) input.addRequestParam(new ParameterInfo("1234","occupation",String.class,occupation)); input.addRequestParam(new ParameterInfo("1234","age",Integer.class,age)); CommandFactoryServiceImpl cf = (CommandFactoryServiceImpl)commandFactory; ApplyPmmlModelCommand command = (ApplyPmmlModelCommand) cf.newApplyPmmlModel(request); (4) ServiceResponse<ExecutionResults> results = ruleClient.executeCommandsWithResults(CONTAINER_ID, command); (5) if (results != null) { (6) PMML4Result resultHolder = (PMML4Result)results.getResult().getValue("results"); if (resultHolder != null && "OK".equals(resultHolder.getResultCode())) { this.score = resultHolder.getResultValue("ScoreCard","score",Double.class).get(); Map<String,Object> rankingMap = (Map<String,Object>)resultHolder.getResultValue("ScoreCard","ranking"); if (rankingMap != null && !rankingMap.isEmpty()) { this.rankedFirstCode = rankingMap.keySet().iterator().next(); } } } } }
1 Defines the class loader if you did not include the KJAR in your client project dependencies 2 Identifies the service client as defined in the configuration settings, including KIE Server REST API access credentials 3 Initializes a PMMLRequestData
object4 Creates an instance of the ApplyPmmlModelCommand
5 Sends the command using the service client 6 Retrieves the results of the executed PMML model -
Execute the class instance to send the PMML invocation request to KIE Server.
Alternatively, you can use JMS and REST interfaces to send the
ApplyPmmlModelCommand
command to KIE Server. For REST requests, you use theApplyPmmlModelCommand
command as aPOST
request tohttp://SERVER:PORT/kie-server/services/rest/server/containers/instances/{containerId}
in JSON, JAXB, or XStream request format.XStream request format for PMML model execution in KIE Server is currently not supported with Java 11. Example POST endpointhttp://localhost:8080/kie-server/services/rest/server/containers/instances/SampleModelContainer
Example JSON request body{ "commands": [ { "apply-pmml-model-command": { "outIdentifier": null, "packageName": null, "hasMining": false, "requestData": { "correlationId": "123", "modelName": "SimpleScorecard", "source": null, "requestParams": [ { "correlationId": "123", "name": "param1", "type": "java.lang.Double", "value": "10.0" }, { "correlationId": "123", "name": "param2", "type": "java.lang.Double", "value": "15.0" } ] } } } ] }
Example curl request with endpoint and bodycurl -X POST "http://localhost:8080/kie-server/services/rest/server/containers/instances/SampleModelContainer" -H "accept: application/json" -H "content-type: application/json" -d "{ \"commands\": [ { \"apply-pmml-model-command\": { \"outIdentifier\": null, \"packageName\": null, \"hasMining\": false, \"requestData\": { \"correlationId\": \"123\", \"modelName\": \"SimpleScorecard\", \"source\": null, \"requestParams\": [ { \"correlationId\": \"123\", \"name\": \"param1\", \"type\": \"java.lang.Double\", \"value\": \"10.0\" }, { \"correlationId\": \"123\", \"name\": \"param2\", \"type\": \"java.lang.Double\", \"value\": \"15.0\" } ] } } } ]}"
Example JSON response{ "results" : [ { "value" : {"org.kie.api.pmml.DoubleFieldOutput":{ "value" : 40.8, "correlationId" : "123", "segmentationId" : null, "segmentId" : null, "name" : "OverallScore", "displayValue" : "OverallScore", "weight" : 1.0 }}, "key" : "OverallScore" }, { "value" : {"org.kie.api.pmml.PMML4Result":{ "resultVariables" : { "OverallScore" : { "value" : 40.8, "correlationId" : "123", "segmentationId" : null, "segmentId" : null, "name" : "OverallScore", "displayValue" : "OverallScore", "weight" : 1.0 }, "ScoreCard" : { "modelName" : "SimpleScorecard", "score" : 40.8, "holder" : { "modelName" : "SimpleScorecard", "correlationId" : "123", "voverallScore" : null, "moverallScore" : true, "vparam1" : 10.0, "mparam1" : false, "vparam2" : 15.0, "mparam2" : false }, "enableRC" : true, "pointsBelow" : true, "ranking" : { "reasonCh1" : 5.0, "reasonCh2" : -6.0 } } }, "correlationId" : "123", "segmentationId" : null, "segmentId" : null, "segmentIndex" : 0, "resultCode" : "OK", "resultObjectName" : null }}, "key" : "results" } ], "facts" : [ ] }
10. Experimental Features
10.1. Declarative Agenda
Declarative Agenda is experimental, and all aspects are highly likely to change in the future. @Eager and @Direct are temporary annotations to control the behaviour of rules, which will also change as Declarative Agenda evolves. Annotations instead of attributes where chosen, to reflect their experimental nature. |
The declarative agenda allows to use rules to control which other rules can fire and when. While this will add a lot more overhead than the simple use of salience, the advantage is it is declarative and thus more readable and maintainable and should allow more use cases to be achieved in a simpler fashion.
This feature is off by default and must be explicitly enabled, that is because it is considered highly experimental for the moment and will be subject to change, but can be activated on a given KieBase by adding the declarativeAgenda='enabled' attribute in the corresponding kbase tag of the kmodule.xml file as in the following example.
<kmodule xmlns:xsi="http://www.w3.org/2001/XMLSchema-instance"
xmlns="http://www.drools.org/xsd/kmodule">
<kbase name="DeclarativeKBase" declarativeAgenda="enabled">
<ksession name="KSession">
</kbase>
</kmodule>
The basic idea is:
-
All rule’s Matches are inserted into WorkingMemory as facts. So you can now do pattern matching against a Match. The rule’s metadata and declarations are available as fields on the Match object.
-
You can use the kcontext.blockMatch( Match match ) for the current rule to block the selected match. Only when that rule becomes false will the match be eligible for firing. If it is already eligible for firing and is later blocked, it will be removed from the agenda until it is unblocked.
-
A match may have multiple blockers and a count is kept. All blockers must became false for the counter to reach zero to enable the Match to be eligible for firing.
-
kcontext.unblockAllMatches( Match match ) is an over-ride rule that will remove all blockers regardless
-
An activation may also be cancelled, so it never fires with cancelMatch
-
An unblocked Match is added to the Agenda and obeys normal salience, agenda groups, ruleflow groups etc.
-
The @Direct annotations allows a rule to fire as soon as it’s matched, this is to be used for rules that block/unblock matches, it is not desirable for these rules to have side effects that impact else where.
void blockMatch(Match match);
void unblockAllMatches(Match match);
void cancelMatch(Match match);
Here is a basic example that will block all matches from rules that have metadata @department('sales'). They will stay blocked until the blockerAllSalesRules rule becomes false, i.e. "go2" is retracted.
rule rule1 @Eager @department('sales') when
$s : String( this == 'go1' )
then
list.add( kcontext.rule.name + ':' + $s );
end
rule rule2 @Eager @department('sales') when
$s : String( this == 'go1' )
then
list.add( kcontext.rule.name + ':' + $s );
end
rule blockerAllSalesRules @Direct @Eager when
$s : String( this == 'go2' )
$i : Match( department == 'sales' )
then
list.add( $i.rule.name + ':' + $s );
kcontext.blockMatch( $i );
end
Further than annotate the blocking rule with @Direct, it is also necessary to annotate all the rules that could be potentially blocked by it with @Eager. This is because, since the Match has to be evaluated by the pattern matching of the blocking rule, the potentially blocked ones cannot be evaluated lazily, otherwise won’t be any Match to be evaluated. |
This example shows how you can use active property to count the number of active or inactive (already fired) matches.
rule rule1 @Eager @department('sales') when
$s : String( this == 'go1' )
then
list.add( kcontext.rule.name + ':' + $s );
end
rule rule2 @Eager @department('sales') when
$s : String( this == 'go1' )
then
list.add( kcontext.rule.name + ':' + $s );
end
rule rule3 @Eager @department('sales') when
$s : String( this == 'go1' )
then
list.add( kcontext.rule.name + ':' + $s );
end
rule countActivateInActive @Direct @Eager when
$s : String( this == 'go2' )
$active : Number( this == 1 ) from accumulate( $a : Match( department == 'sales', active == true ), count( $a ) )
$inActive : Number( this == 2 ) from accumulate( $a : Match( department == 'sales', active == false ), count( $a ) )
then
kcontext.halt( );
end
10.2. Browsing graphs of objects with OOPath
When the field of a fact is a collection it is possible to bind and reason over all the items in that collection on by one using the from
keyword.
Nevertheless, when it is required to browse a graph of object the extensive use of the from
conditional element may result in a verbose and cubersome syntax like in the following example:
rule "Find all grades for Big Data exam" when
$student: Student( $plan: plan )
$exam: Exam( course == "Big Data" ) from $plan.exams
$grade: Grade() from $exam.grades
then /* RHS */ end
In this example it has been assumed to use a domain model consisting of a Student
who has a Plan
of study: a Plan
can have zero or more Exam
s and an Exam
zero or more Grade
s.
Note that only the root object of the graph (the Student
in this case) needs to be in the working memory in order to make this works.
By borrowing ideas from XPath, this syntax can be made more succinct, as XPath has a compact notation for navigating through related elements while handling collections and filtering constraints.
This XPath-inspired notation has been called OOPath
since it is explictly intended to browse graph of objects.
Using this notation the former example can be rewritten as it follows:
rule "Find all grades for Big Data exam" when
Student( $grade: /plan/exams[course == "Big Data"]/grades )
then /* RHS */ end
Formally, the core grammar of an OOPath
expression can be defined in EBNF notation in this way.
OOPExpr = [ID ( ":" | ":=" )] ( "/" | "?/" ) OOPSegment { ( "/" | "?/" | "." ) OOPSegment } ;
OOPSegment = ID ["#" ID] ["[" ( Number | Constraints ) "]"]
In practice an OOPath
expression has the following features.
-
It has to start with
/
or with a?/
in case of a completely non-reactive OOPath (see below). -
It can dereference a single property of an object with the
.
operator -
It can dereference a multiple property of an object using the
/
operator. If a collection is returned, it will iterate over the values in the collection -
While traversing referenced objects it can filter away those not satisfying one or more constraints, written as predicate expressions between square brackets like in:
Student( $grade: /plan/exams[ course == "Big Data" ]/grades )
-
During oopath traversal it is also possible to downcast the traversed object to a subclass of the class declared in the generic collection. In this way subsequent constraints can also safely access to properties declared only in that subclass like in:
Student( $grade: /plan/exams#AdvancedExam[ course == "Big Data", level > 3 ]/grades )
Objects that are not instances of the class specificed in this inline cast will be automatically filtered away.
-
A constraint can also have a beckreference to an object of the graph traversed before the currently iterated one. For example the following OOPath:
Student( $grade: /plan/exams/grades[ result > ../averageResult ] )
will match only the grades having a result above the average for the passed exam.
-
A constraint can also recursively be another OOPath as it follows:
Student( $exam: /plan/exams[ /grades[ result > 20 ] ] )
-
Items can also be accessed by their index by putting it between square brackets like in:
Student( $grade: /plan/exams[0]/grades )
To adhere to Java convention OOPath indexes are 0-based, compared to XPath 1-based
10.2.1. Reactive and Non-Reactive OOPath
At the moment Drools is not able to react to updates involving a deeply nested object traversed during the evaluation of an OOPath
expression.
To make these objects reactive to changes it is then necessary to make them extend the class org.drools.core.phreak.ReactiveObject
.
It is planned to overcome this limitation by implementing a mechanism that automatically instruments the classes belonging to a specific domain model.
Having extendend that class, the domain objects can notify the Drools engine when one of its field has been updated by invoking the inherited method notifyModification
as in the following example:
public void setCourse(String course) {
this.course = course;
notifyModification(this);
}
In this way when using an OOPath like the following:
Student( $grade: /plan/exams[ course == "Big Data" ]/grades )
if an exam is moved to a different course, the rule is re-triggered and the list of grades matching the rule recomputed.
It is also possible to have reactivity only in one subpart of the OOPath as in:
Student( $grade: /plan/exams[ course == "Big Data" ]?/grades )
Here, using the ?/
separator instead of the /
one, the Drools engine will react to a change made to an exam, or if an exam is added to the plan, but not if a new grade is added to an existing exam.
Of course if a OOPath chunk is not reactive, all remaining part of the OOPath from there till the end of the expression will be non-reactive as well.
For instance the following OOPath
Student( $grade: ?/plan/exams[ course == "Big Data" ]/grades )
will be completely non-reactive.
For this reason it is not allowed to use the ?/
separator more than once in the same OOPath so an expression like:
Student( $grade: /plan?/exams[ course == "Big Data" ]?/grades )
will cause a compile time error.
10.3. Traits
WARNING : this feature is still experimental and subject to changes
The same fact may have multiple dynamic types which do not fit naturally in a class hierarchy. Traits allow to model this very common scenario. A trait is an interface that can be applied (and eventually removed) to an individual object at runtime. To create a trait rather than a traditional bean, one has to declare them explicitly as in the following example:
declare trait GoldenCustomer
// fields will map to getters/setters
code : String
balance : long
discount : int
maxExpense : long
end
At runtime, this declaration results in an interface, which can be used to write patterns, but can not be instantiated directly. In order to apply a trait to an object, we provide the new don keyword, which can be used as simply as this:
when
$c : Customer()
then
GoldenCustomer gc = don( $c, GoldenCustomer.class );
end
when a core object dons a trait, a proxy class is created on the fly (one such class will be generated lazily for each core/trait class combination). The proxy instance, which wraps the core object and implements the trait interface, is inserted automatically and will possibly activate other rules. An immediate advantage of declaring and using interfaces, getting the implementation proxy for free from the Drools engine, is that multiple inheritance hierarchies can be exploited when writing rules. The core classes, however, need not implement any of those interfaces statically, also facilitating the use of legacy classes as cores. In fact, any object can don a trait, provided that they are declared as @Traitable. Notice that this annotation used to be optional, but now is mandatory.
import org.drools.core.factmodel.traits.Traitable;
declare Customer
@Traitable
code : String
balance : long
end
The only connection between core classes and trait interfaces is at the proxy level: a trait is not specifically tied to a core class. This means that the same trait can be applied to totally different objects. For this reason, the trait does not transparently expose the fields of its core object. So, when writing a rule using a trait interface, only the fields of the interface will be available, as usual. However, any field in the interface that corresponds to a core object field, will be mapped by the proxy class:
when
$o: OrderItem( $p : price, $code : custCode )
$c: GoldenCustomer( code == $code, $a : balance, $d: discount )
then
$c.setBalance( $a - $p*$d );
end
In this case, the code and balance would be read from the underlying Customer object. Likewise, the setAccount will modify the underlying object, preserving a strongly typed access to the data structures. A hard field must have the same name and type both in the core class and all donned interfaces. The name is used to establish the mapping: if two fields have the same name, then they must also have the same declared type. The annotation @org.drools.core.factmodel.traits.Alias allows to relax this restriction. If an @Alias is provided, its value string will be used to resolve mappings instead of the original field name. @Alias can be applied both to traits and core beans.
import org.drools.core.factmodel.traits.*;
declare trait GoldenCustomer
balance : long @Alias( "org.acme.foo.accountBalance" )
end
declare Person
@Traitable
name : String
savings : long @Alias( "org.acme.foo.accountBalance" )
end
when
GoldenCustomer( balance > 1000 ) // will react to new Person( 2000 )
then
end
More work is being done on relaxing this constraint (see the experimental section on "logical" traits later). Now, one might wonder what happens when a core class does NOT provide the implementation for a field defined in an interface. We call hard fields those trait fields which are also core fields and thus readily available, while we define soft those fields which are NOT provided by the core class. Hidden fields, instead, are fields in the core class not exposed by the interface.
So, while hard field management is intuitive, there remains the problem of soft and hidden fields. Hidden fields are normally only accessible using the core class directly. However, the "fields" Map can be used on a trait interface to access a hidden field. If the field can’t be resolved, null will be returned. Notice that this feature is likely to change in the future.
when
$sc : GoldenCustomer( fields[ "age" ] > 18 ) // age is declared by the underlying core class, but not by GoldenCustomer
then
Soft fields, instead, are stored in a Map-like data structure that is specific to each core object and referenced by the proxy(es), so that they are effectively shared even when an object dons multiple traits.
when
$sc : GoldenCustomer( $c : code, // hard getter
$maxExpense : maxExpense > 1000 // soft getter
)
then
$sc.setDiscount( ... ); // soft setter
end
A core object also holds a reference to all its proxies, so that it is possible to track which type(s) have been added to an object, using a sort of dynamic "instanceof" operator, which we called isA. The operator can accept a String, a class literal or a list of class literals. In the latter case, the constraint is satisfied only if all the traits have been donned.
$sc : GoldenCustomer( $maxExpense : maxExpense > 1000,
this isA "SeniorCustomer", this isA [ NationalCustomer.class, OnlineCustomer.class ]
)
Eventually, the business logic may require that a trait is removed from a wrapped object. To this end, we provide two options. The first is a "logical don", which will result in a logical insertion of the proxy resulting from the traiting operation. The TMS will ensure that the trait is removed when its logical support is removed in the first place.
then
don( $x, // core object
Customer.class, // trait class
true // optional flag for logical insertion
)
The second is the use of the "shed" keyword, which causes the removal of any type that is a subtype (or equivalent) of the one passed as an argument. Notice that, as of version 5.5, shed would only allow to remove a single specific trait.
then
Thing t = shed( $x, GoldenCustomer.class ) // also removes any trait that
This operation returns another proxy implementing the org.drools.core.factmodel.traits.Thing interface, where the getFields() and getCore() methods are defined. Internally, in fact, all declared traits are generated to extend this interface (in addition to any others specified). This allows to preserve the wrapper with the soft fields which would otherwise be lost.
A trait and its proxies are also correlated in another way. Starting from version 5.6, whenever a core object is "modified", its proxies are "modified" automatically as well, to allow trait-based patterns to react to potential changes in hard fields. Likewise, whenever a trait proxy (mached by a trait pattern) is modified, the modification is propagated to the core class and the other traits. Morover, whenever a don operation is performed, the core object is also modified automatically, to reevaluate any "isA" operation which may be triggered.
Potentially, this may result in a high number of modifications, impacting performance (and correctness) heavily. So two solutions are currently implemented. First, whenever a core object is modified, only the most specific traits (in the sense of inheritance between trait interfaces) are updated and an internal blocking mechanism is in place to ensure that each potentially matching pattern is evaluated once and only once. So, in the following situation:
declare trait GoldenCustomer end
declare trait NationalGoldenustomer extends GoldenCustomer end
declare trait SeniorGoldenCustomer extends GoldenCustomer end
a modification of an object that is both a GoldenCustomer, a NationalGoldenCustomer and a SeniorGoldenCustomer wold cause only the latter two proxies to be actually modified. The first would match any pattern for GoldenCustomer and NationalGoldenCustomer; the latter would instead be prevented from rematching GoldenCustomer, but would be allowed to match SeniorGoldenCustomer patterns. It is not necessary, instead, to modify the GoldenCustomer proxy since it is already covered by at least one other more specific trait.
The second method, up to the usr, is to mark traits as @PropertyReactive. Property reactivity is trait-enabled and takes into account the trait field mappings, so to block unnecessary propagations.
10.3.1. Cascading traits
WARNING : This feature is extremely experimental and subject to changes
Normally, a hard field must be exposed with its original type by all traits donned by an object, to prevent situations such as
declare Person
@Traitable
name : String
id : String
end
declare trait Customer
id : String
end
declare trait Patient
id : long // Person can't don Patient, or an exception will be thrown
end
Should a Person don both Customer and Patient, the type of the hard field id would be ambiguous. However, consider the following example, where GoldenCustomers refer their best friends so that they become Customers as well:
declare Person
@Traitable( logical=true )
bestFriend : Person
end
declare trait Customer end
declare trait GoldenCustomer extends Customer
refers : Customer @Alias( "bestFriend" )
end
Aside from the @Alias, a Person-as-GoldenCustomer’s best friend might be compatible with the requirements of the trait GoldenCustomer, provided that they are some kind of Customer themselves. Marking a Person as "logically traitable" - i.e. adding the annotation @Traitable( logical = true ) - will instruct the Drools engine to try and preserve the logical consistency rather than throwing an exception due to a hard field with different type declarations (Person vs Customer). The following operations would then work:
Person p1 = new Person();
Person p2 = new Person();
p1.setBestFriend( p2 );
...
Customer c2 = don( p2, Customer.class );
...
GoldenCustomer gc1 = don( p1, GoldenCustomer.class );
...
p1.getBestFriend(); // returns p2
gc1.getRefers(); // returns c2, a Customer proxy wrapping p2
Notice that, by the time p1 becomes GoldenCustomer, p2 must have already become a Customer themselves, otherwise a runtime exception will be thrown since the very definition of GoldenCustomer would have been violated.
In some cases, however, one might want to infer, rather than verify, that p2 is a Customer by virtue that p1 is a GoldenCustomer. This modality can be enabled by marking Customer as "logical", using the annotation @org.drools.core.factmodel.traits.Trait( logical = true ). In this case, should p2 not be a Customer by the time that p1 becomes a GoldenCustomer, it will be automatically don the trait Customer to preserve the logical integrity of the system.
Notice that the annotation on the core class enables the dynamic type management for its fields, whereas the annotation on the traits determines whether they will be enforced as integrity constraints or cascaded dynamically.
import org.drools.factmodel.traits.*;
declare trait Customer
@Trait( logical = true )
end
Drools Integration
Integration Documentation
11. Drools commands
11.1. Runtime commands in Drools
Drools supports runtime commands that you can send to KIE Server for asset-related operations, such as executing all rules or inserting or retracting objects in a KIE session. The full list of supported runtime commands is located in the org.drools.core.command.runtime
package in your Drools instance.
In the KIE Server REST API, you use the global org.drools.core.command.runtime
commands or the rule-specific org.drools.core.command.runtime.rule
commands as the request body for POST
requests to http://SERVER:PORT/kie-server/services/rest/server/containers/instances/{containerId}
. For more information about using the KIE Server REST API, see KIE Server REST API for KIE containers and business assets.
In the KIE Server Java client API, you can embed these commands in your Java application along with the relevant Java client. For example, for rule-related commands, you use the RuleServicesClient
Java client with the embedded commands. For more information about using the KIE Server Java client API, see KIE Server Java client API for KIE containers and business assets.
11.1.1. Sample runtime commands in Drools
The following are sample runtime commands that you can use with the KIE Server REST API or Java client API for asset-related operations in KIE Server:
-
BatchExecutionCommand
-
InsertObjectCommand
-
RetractCommand
-
ModifyCommand
-
GetObjectCommand
-
GetObjectsCommand
-
InsertElementsCommand
-
FireAllRulesCommand
-
QueryCommand
-
SetGlobalCommand
-
GetGlobalCommand
For the full list of supported runtime commands, see the org.drools.core.command.runtime
package in your Drools instance.
Each command in this section includes a REST request body example (JSON) for the KIE Server REST API and an embedded Java command example for the KIE Server Java client API. The Java examples use an object org.drools.compiler.test.Person
with the fields name
(String) and age
(Integer).
- BatchExecutionCommand
-
Contains multiple commands to be executed together.
Table 16. Command attributes Name Description Requirement commands
List of commands to be executed.
Required
lookup
Sets the KIE session ID on which the commands will be executed. For stateless KIE sessions, this attribute is required. For stateful KIE sessions, this attribute is optional and if not specified, the default KIE session is used.
Required for stateless KIE session, optional for stateful KIE session
Example JSON request body{ "lookup": "ksession1", "commands": [ { "insert": { "object": { "org.drools.compiler.test.Person": { "name": "john", "age": 25 } } } }, { "fire-all-rules": { "max": 10, "out-identifier": "firedActivations" } } ] }
Example Java commandBatchExecutionCommand command = new BatchExecutionCommand(); command.setLookup("ksession1"); InsertObjectCommand insertObjectCommand = new InsertObjectCommand(new Person("john", 25)); FireAllRulesCommand fireAllRulesCommand = new FireAllRulesCommand(); command.getCommands().add(insertObjectCommand); command.getCommands().add(fireAllRulesCommand); ksession.execute(command);
Example server response (JSON){ "response": [ { "type": "SUCCESS", "msg": "Container command-script-container successfully called.", "result": { "execution-results": { "results": [ { "value": 0, "key": "firedActivations" } ], "facts": [] } } } ] }
- InsertObjectCommand
-
Inserts an object into the KIE session.
Table 17. Command attributes Name Description Requirement object
The object to be inserted
Required
out-identifier
ID of the
FactHandle
created from the object insertion and added to the execution resultsOptional
return-object
Boolean to determine whether the object must be returned in the execution results (default:
true
)Optional
entry-point
Entry point for the insertion
Optional
Example JSON request body{ "commands": [ { "insert": { "entry-point": "my stream", "object": { "org.drools.compiler.test.Person": { "age": 25, "name": "john" } }, "out-identifier": "john", "return-object": false } } ] }
Example Java commandCommand insertObjectCommand = CommandFactory.newInsert(new Person("john", 25), "john", false, null); ksession.execute(insertObjectCommand);
Example server response (JSON){ "response": [ { "type": "SUCCESS", "msg": "Container command-script-container successfully called.", "result": { "execution-results": { "results": [], "facts": [ { "value": { "org.drools.core.common.DefaultFactHandle": { "external-form": "0:4:436792766:-2127720265:4:DEFAULT:NON_TRAIT:java.util.LinkedHashMap" } }, "key": "john" } ] } } } ] }
- RetractCommand
-
Retracts an object from the KIE session.
Table 18. Command attributes Name Description Requirement fact-handle
The
FactHandle
associated with the object to be retractedRequired
Example JSON request body{ "commands": [ { "retract": { "fact-handle": "0:4:436792766:-2127720265:4:DEFAULT:NON_TRAIT:java.util.LinkedHashMap" } } ] }
Example Java command: UseFactHandleFromString
RetractCommand retractCommand = new RetractCommand(); retractCommand.setFactHandleFromString("123:234:345:456:567");
Example Java command: UseFactHandle
from inserted objectRetractCommand retractCommand = new RetractCommand(factHandle);
Example server response (JSON){ "response": [ { "type": "SUCCESS", "msg": "Container employee-rostering successfully called.", "result": { "execution-results": { "results": [], "facts": [] } } } ] }
- ModifyCommand
-
Modifies a previously inserted object in the KIE session.
Table 19. Command attributes Name Description Requirement fact-handle
The
FactHandle
associated with the object to be modifiedRequired
setters
List of setters for object modifications
Required
Example JSON request body{ "commands": [ { "modify": { "fact-handle": "0:4:436792766:-2127720265:4:DEFAULT:NON_TRAIT:java.util.LinkedHashMap", "setters": { "accessor": "age", "value": 25 } } } ] }
Example Java commandModifyCommand modifyCommand = new ModifyCommand(factHandle); List<Setter> setters = new ArrayList<Setter>(); setters.add(new SetterImpl("age", "25")); modifyCommand.setSetters(setters);
Example server response (JSON){ "response": [ { "type": "SUCCESS", "msg": "Container employee-rostering successfully called.", "result": { "execution-results": { "results": [], "facts": [] } } } ] }
- GetObjectCommand
-
Retrieves an object from a KIE session.
Table 20. Command attributes Name Description Requirement fact-handle
The
FactHandle
associated with the object to be retrievedRequired
out-identifier
ID of the
FactHandle
created from the object insertion and added to the execution resultsOptional
Example JSON request body{ "commands": [ { "get-object": { "fact-handle": "0:4:436792766:-2127720265:4:DEFAULT:NON_TRAIT:java.util.LinkedHashMap", "out-identifier": "john" } } ] }
Example Java commandGetObjectCommand getObjectCommand = new GetObjectCommand(); getObjectCommand.setFactHandleFromString("123:234:345:456:567"); getObjectCommand.setOutIdentifier("john");
Example server response (JSON){ "response": [ { "type": "SUCCESS", "msg": "Container command-script-container successfully called.", "result": { "execution-results": { "results": [ { "value": null, "key": "john" } ], "facts": [] } } } ] }
- GetObjectsCommand
-
Retrieves all objects from the KIE session as a collection.
Table 21. Command attributes Name Description Requirement object-filter
Filter for the objects returned from the KIE session
Optional
out-identifier
Identifier to be used in the execution results
Optional
Example JSON request body{ "commands": [ { "get-objects": { "out-identifier": "objects" } } ] }
Example Java commandGetObjectsCommand getObjectsCommand = new GetObjectsCommand(); getObjectsCommand.setOutIdentifier("objects");
Example server response (JSON){ "response": [ { "type": "SUCCESS", "msg": "Container command-script-container successfully called.", "result": { "execution-results": { "results": [ { "value": [ { "org.apache.xerces.dom.ElementNSImpl": "<?xml version=\"1.0\" encoding=\"UTF-16\"?>\n<object xmlns:xsi=\"http://www.w3.org/2001/XMLSchema-instance\" xsi:type=\"person\"><age>25</age><name>john</name>\n <\/object>" }, { "org.drools.compiler.test.Person": { "name": "john", "age": 25 } } ], "key": "objects" } ], "facts": [] } } } ] }
- InsertElementsCommand
-
Inserts a list of objects into the KIE session.
Table 22. Command attributes Name Description Requirement objects
The list of objects to be inserted into the KIE session
Required
out-identifier
ID of the
FactHandle
created from the object insertion and added to the execution resultsOptional
return-object
Boolean to determine whether the object must be returned in the execution results. Default value:
true
.Optional
entry-point
Entry point for the insertion
Optional
Example JSON request body{ "commands": [ { "insert-elements": { "objects": [ { "containedObject": { "@class": "org.drools.compiler.test.Person", "age": 25, "name": "john" } }, { "containedObject": { "@class": "Person", "age": 35, "name": "sarah" } } ] } } ] }
Example Java commandList<Object> objects = new ArrayList<Object>(); objects.add(new Person("john", 25)); objects.add(new Person("sarah", 35)); Command insertElementsCommand = CommandFactory.newInsertElements(objects);
Example server response (JSON){ "response": [ { "type": "SUCCESS", "msg": "Container command-script-container successfully called.", "result": { "execution-results": { "results": [], "facts": [ { "value": { "org.drools.core.common.DefaultFactHandle": { "external-form": "0:4:436792766:-2127720265:4:DEFAULT:NON_TRAIT:java.util.LinkedHashMap" } }, "key": "john" }, { "value": { "org.drools.core.common.DefaultFactHandle": { "external-form": "0:4:436792766:-2127720266:4:DEFAULT:NON_TRAIT:java.util.LinkedHashMap" } }, "key": "sarah" } ] } } } ] }
- FireAllRulesCommand
-
Executes all rules in the KIE session.
Table 23. Command attributes Name Description Requirement max
Maximum number of rules to be executed. The default is
-1
and does not put any restriction on execution.Optional
out-identifier
ID to be used for retrieving the number of fired rules in execution results.
Optional
agenda-filter
Agenda Filter to be used for rule execution.
Optional
Example JSON request body{ "commands" : [ { "fire-all-rules": { "max": 10, "out-identifier": "firedActivations" } } ] }
Example Java commandFireAllRulesCommand fireAllRulesCommand = new FireAllRulesCommand(); fireAllRulesCommand.setMax(10); fireAllRulesCommand.setOutIdentifier("firedActivations");
Example server response (JSON){ "response": [ { "type": "SUCCESS", "msg": "Container command-script-container successfully called.", "result": { "execution-results": { "results": [ { "value": 0, "key": "firedActivations" } ], "facts": [] } } } ] }
- QueryCommand
-
Executes a query defined in the KIE base.
Table 24. Command attributes Name Description Requirement name
Query name.
Required
out-identifier
ID of the query results. The query results are added in the execution results with this identifier.
Optional
arguments
List of objects to be passed as a query parameter.
Optional
Example JSON request body{ "commands": [ { "query": { "name": "persons", "arguments": [], "out-identifier": "persons" } } ] }
Example Java commandQueryCommand queryCommand = new QueryCommand(); queryCommand.setName("persons"); queryCommand.setOutIdentifier("persons");
Example server response (JSON){ "type": "SUCCESS", "msg": "Container stateful-session successfully called.", "result": { "execution-results": { "results": [ { "value": { "org.drools.core.runtime.rule.impl.FlatQueryResults": { "idFactHandleMaps": { "type": "LIST", "componentType": null, "element": [ { "type": "MAP", "componentType": null, "element": [ { "value": { "org.drools.core.common.DisconnectedFactHandle": { "id": 1, "identityHashCode": 1809949690, "objectHashCode": 1809949690, "recency": 1, "object": { "org.kie.server.testing.Person": { "fullname": "John Doe", "age": 47 } }, "entryPointId": "DEFAULT", "traitType": "NON_TRAIT", "external-form": "0:1:1809949690:1809949690:1:DEFAULT:NON_TRAIT:org.kie.server.testing.Person" } }, "key": "$person" } ] } ] }, "idResultMaps": { "type": "LIST", "componentType": null, "element": [ { "type": "MAP", "componentType": null, "element": [ { "value": { "org.kie.server.testing.Person": { "fullname": "John Doe", "age": 47 } }, "key": "$person" } ] } ] }, "identifiers": { "type": "SET", "componentType": null, "element": [ "$person" ] } } }, "key": "persons" } ], "facts": [] } } }
- SetGlobalCommand
-
Sets an object to a global state.
Table 25. Command attributes Name Description Requirement identifier
ID of the global variable defined in the KIE base
Required
object
Object to be set into the global variable
Optional
out
Boolean to exclude the global variable you set from the execution results
Optional
out-identifier
ID of the global execution result
Optional
Example JSON request body{ "commands": [ { "set-global": { "identifier": "helper", "object": { "org.kie.server.testing.Person": { "fullname": "kyle", "age": 30 } }, "out-identifier": "output" } } ] }
Example Java commandSetGlobalCommand setGlobalCommand = new SetGlobalCommand(); setGlobalCommand.setIdentifier("helper"); setGlobalCommand.setObject(new Person("kyle", 30)); setGlobalCommand.setOut(true); setGlobalCommand.setOutIdentifier("output");
Example server response (JSON){ "type": "SUCCESS", "msg": "Container stateful-session successfully called.", "result": { "execution-results": { "results": [ { "value": { "org.kie.server.testing.Person": { "fullname": "kyle", "age": 30 } }, "key": "output" } ], "facts": [] } } }
- GetGlobalCommand
-
Retrieves a previously defined global object.
Table 26. Command attributes Name Description Requirement identifier
ID of the global variable defined in the KIE base
Required
out-identifier
ID to be used in the execution results
Optional
Example JSON request body{ "commands": [ { "get-global": { "identifier": "helper", "out-identifier": "helperOutput" } } ] }
Example Java commandGetGlobalCommand getGlobalCommand = new GetGlobalCommand(); getGlobalCommand.setIdentifier("helper"); getGlobalCommand.setOutIdentifier("helperOutput");
Example server response (JSON){ "response": [ { "type": "SUCCESS", "msg": "Container command-script-container successfully called.", "result": { "execution-results": { "results": [ { "value": null, "key": "helperOutput" } ], "facts": [] } } } ] }
12. CDI
12.1. Introduction
CDI, Contexts and Dependency Injection, is Java specification that provides declarative controls and strucutres to an application. KIE can use it to automatically instantiate and bind things, without the need to use the programmatic API.
12.2. Annotations
@KContainer, @KBase and @KSession all support an optional 'name' attribute. CDI typically does "getOrCreate" when it injects, all injections receive the same instance for the same set of annotations. the 'name' annotation forces a unique instance for each name, although all instance for that name will be identity equals.
12.2.1. @KReleaseId
Used to bind an instance to a specific version of a KieModule. If kie-ci is on the classpath this will resolve dependencies automatically, downloading from remote repositories.
12.2.2. @KContainer
@KContainer is optional as it can be detected and added by the use of @Inject and variable type inferrence.
@Inject
private KieContainer kContainer;
@Inject
@KReleaseId(groupId = "jar1", artifactId = "art1", version = "1.1")
private KieContainer kContainer;
@Inject
@KContainer(name = "kc1")
@KReleaseId(groupId = "jar1", artifactId = "art1", version = "1.1")
private KieContainer kContainer;
12.2.3. @KBase
@KBase is optional as it can be detected and added by the use of @Inject and variable type inference.
The default argument, if given, maps to the value attribute and specifies the name of the KieBase from the kmodule.xml file.
@Inject
private KieBase kbase;
@Inject
@KReleaseId( groupId = "jar1", artifactId = "art1", version = "1.0")
private KieBase kbase;
@Inject
@KBase("kbase1")
@KReleaseId( groupId = "jar1", artifactId = "art1", version = "1.0")
private KieBase kbase1v10;
@Inject
@KBase("kbase1")
@KReleaseId( groupId = "jar1", artifactId = "art1", version = "1.1")
private KieBase kbase1v10;
@Inject
@KSession(value="kbase1", name="kb1")
@KReleaseId( groupId = "jar1", artifactId = "art1", version = "1.0")
private KieBase kbase1kb1;
@Inject
@KSession(value="kbase1", name="kb2")
@KReleaseId( groupId = "jar1", artifactId = "art1", version = "1.0")
private KieBase kbase1kb2;
12.2.4. @KSession for KieSession
@KSession is optional as it can be detected and added by the use of @Inject and variable type inference.
The default argument, if given, maps to the value attribute and specifies the name of the KieSession from the kmodule.xml file
@Inject
private KieSession ksession;
@Inject
@KReleaseId( groupId = "jar1", artifactId = "art1", version = "1.0")
private KieSession ksession;
@Inject
@KSession("ksession1")
@KReleaseId( groupId = "jar1", artifactId = "art1", version = "1.0")
private KieSession ksessionv10;
@Inject
@KSession("ksession1")
@KReleaseId( groupId = "jar1", artifactId = "art1", version = "1.1")
private KieSession ksessionv11;
@Inject
@KSession(value="ksession1", name="ks1")
@KReleaseId( groupId = "jar1", artifactId = "art1", version = "1.0")
private KieSession ksession1ks1
@Inject
@KSession(value="ksession1", name="ks2")
@KReleaseId( groupId = "jar1", artifactId = "art1", version = "1.0")
private KieSession ksession1ks2
12.2.5. @KSession for StatelessKieSession
@KSession is optional as it can be detected and added by the use of @Inject and variable type inference.
The default argument, if given, maps to the value attribute and specifies the name of the KieSession from the kmodule.xml file.
@Inject
private StatelessKieSession ksession;
@Inject
@KReleaseId( groupId = "jar1", artifactId = "art1", version = "1.0")
private StatelessKieSession ksession;
@Inject
@KSession("ksession1")
@KReleaseId( groupId = "jar1", rtifactId = "art1", version = "1.0")
private StatelessKieSession ksessionv10;
@Inject
@KSession("ksession1")
@KReleaseId( groupId = "jar1", rtifactId = "art1", version = "1.1")
private StatelessKieSession ksessionv11;
@Inject
@KSession(value="ksession1", name="ks1")
@KReleaseId( groupId = "jar1", artifactId = "art1", version = "1.0")
private StatelessKieSession ksession1ks1
@Inject
@KSession(value="ksession1", name="ks2")
@KReleaseId( groupId = "jar1", artifactId = "art1", version = "1.0")
private StatelessKieSession ksession1ks2
12.3. API Example Comparison
CDI can inject instances into fields, or even pass them as arguments. In this example field injection is used.
@Inject
@KSession("ksession1")
KieSession kSession;
public void go(PrintStream out) {
kSession.setGlobal("out", out);
kSession.insert(new Message("Dave", "Hello, HAL. Do you read me, HAL?"));
kSession.fireAllRules();
}
This is less code and more declarative than the API approach.
public void go(PrintStream out) {
KieServices ks = KieServices.Factory.get();
KieContainer kContainer = ks.getKieClasspathContainer();
KieSession kSession = kContainer.newKieSession("ksession1");
kSession.setGlobal("out", out);
kSession.insert(new Message("Dave", "Hello, HAL. Do you read me, HAL?"));
kSession.fireAllRules();
}
13. Integration with Spring
13.1. Important Changes for Drools 6.0
Drools Spring integration has undergone a complete makeover inline with the changes for Drools 6.0. The following are some of the major changes
-
The recommended prefix for the Drools Spring has changed from 'drools:' to 'kie:'
-
New Top Level Tags in 6.0
-
kie:kmodule
-
kie:import (from version 6.2)
-
kie:releaseId (from version 6.2)
-
-
The following tags are no longer valid as top level tags.
-
kie:kbase - A child of the kie:kmodule tag.
-
kie:ksession - A child of the kie:kbase tag.
-
-
Removed Tags from previous versions Drools 5.x
-
drools:resources
-
drools:resource
-
drools:grid
-
drools:grid-node
-
13.2. Integration with Drools Expert
In this section we will explain the kie namespace.
13.2.1. KieModule
The <kie:kmodule> defines a collection of KieBase and associated KieSession’s. The kmodule tag has one MANDATORY parameter 'id'.
Attribute | Description | Required |
---|---|---|
id |
Bean’s id is the name to be referenced from other beans. Standard Spring ID semantics apply. |
Yes |
A kmodule tag can contain only the following tags as children.
-
kie:kbase
Refer to the documentation of kmodule.xml in the Drools Expert documentation for detailed explanation of the need for kmodule.
13.2.2. KieBase
13.2.2.1. <kie:kbase>'s parameters as attributes:
Attribute | Description | Required |
---|---|---|
name |
Name of the KieBase |
Yes |
packages |
Comma separated list of resource packages to be included in this kbase |
No |
includes |
kbase names to be included. All resources from the corresponding kbase are included in this kbase. |
No |
default |
Boolean (TRUE/FALSE). Default kbase, if not provided, it is assumed to be FALSE |
No |
scope |
prototype | singleton. If not provided assumed to be singleton (default) |
No |
eventProcessingMode |
Event Processing Mode. Valid options are STREAM, CLOUD |
No |
equalsBehavior |
Valid options are IDENTITY, EQUALITY |
No |
declarativeAgenda |
Valid options are enabled, disabled, true, false |
No |
13.2.2.3. <kie:kbase>'s definition example
A kmodule can contain multiple (1..n) kbase elements.
<kie:kmodule id="sample_module">
<kie:kbase name="kbase1" packages="org.drools.spring.sample">
...
</kie:kbase>
</kie:kmodule>
<bean id="kiePostProcessor"
class="org.kie.spring.KModuleBeanFactoryPostProcessor"/>
13.2.2.4. Spring Bean Scope (for KieBase and KieSession)
When defining a KieBase or a KieSession, you have the option of declaring a scope for that bean. For example, To force Spring to produce a new bean instance each time one is needed, you should declare the bean’s scope attribute to be 'prototype'. Similar way if you want Spring to return the same bean instance each time one is needed, you should declare the bean’s scope attribute to be 'singleton'.
13.2.3. IMPORTANT NOTE
For proper initialization of the kmodule objects (kbase/ksession), it is mandatory for a bean of type org.kie.spring.KModuleBeanFactoryPostProcessor
or org.kie.spring.annotations.KModuleAnnotationPostProcessor
be defined.
<bean id="kiePostProcessor"
class="org.kie.spring.KModuleBeanFactoryPostProcessor"/>
<bean id="kiePostProcessor"
class="org.kie.spring.annotations.KModuleAnnotationPostProcessor"/>
Without the org.kie.spring.KModuleBeanFactoryPostProcessor or org.kie.spring.annotations.KModuleAnnotationPostProcessor bean definition, the kie-spring integration will fail to work. |
13.2.4. KieSessions
<kie:ksession> element defines KieSessions. The same tag is used to define both Stateful (org.kie.api.runtime.KieSession) and Stateless (org.kie.api.runtime.StatelessKieSession) sessions.
13.2.4.1. <kie:ksession>'s parameters as attributes:
Attribute | Description | Required |
---|---|---|
name |
ksession’s name. |
Yes |
type |
is the session stateful or stateless?. If this attribute is empty or missing, the session is assumed to be of type Stateful. |
No |
default |
Is this the default session? |
no |
scope |
prototype | singleton. If not provided assumed to be singleton (default) |
no |
clockType |
REALTIME or PSEUDO |
no |
listeners-ref |
Specifies the reference to the event listeners group (see 'Defining a Group of listeners' section below). |
no |
<kie:kmodule id="sample-kmodule">
<kie:kbase name="drl_kiesample3" packages="drl_kiesample3">
<kie:ksession name="ksession1" type="stateless"/>
<kie:ksession name="ksession2"/>
</kie:kbase>
</kie:kmodule>
<bean id="kiePostProcessor"
class="org.kie.spring.KModuleBeanFactoryPostProcessor"/>
13.2.4.2. Spring Bean Scope (for KieBase and KieSession)
When defining a KieBase or a KieSession, you have the option of declaring a scope for that bean. For example, To force Spring to produce a new bean instance each time one is needed, you should declare the bean’s scope attribute to be 'prototype'. Similar way if you want Spring to return the same bean instance each time one is needed, you should declare the bean’s scope attribute to be 'singleton'.
13.2.5. Kie:ReleaseId
13.2.5.1. <kie:releaseId>'s parameters as attributes:
Attribute | Description | Required |
---|---|---|
id |
Bean’s id is the name to be referenced from other beans. Standard Spring ID semantics apply. |
Yes |
groupId |
groupId from Maven GAV |
Yes |
artifactId |
artifactId from Maven GAV |
Yes |
version |
version from Maven GAV |
Yes |
<kie:releaseId id="beanId" groupId="org.kie.spring"
artifactId="named-artifactId" version="1.0.0-SNAPSHOT"/>
13.2.6. Kie:Import
Starting with version 6.2, kie-spring allows for importing of kie objects from kjars found on the classpath. Two modes of importing the kie objects are currently supported.
Attribute | Description | Required |
---|---|---|
releaseId |
Reference to a Bean ID. Standard Spring ID semantics apply. |
No |
enableScanner |
Enable Scanner. This attribute is used only if 'releaseId' is specified. |
No |
scannerInterval |
Scanning Interval in milli seconds. This attribute is used only if 'releaseId' is specified. |
No |
13.2.6.1. Global Import
The import tag will force the automatic scan of all the jars on the classpath, initialize the Kie Objects (Kbase/KSessions) and import these objects into the spring context.
<kie:import />
13.2.6.2. Specific Import - ReleaseId
Using the releaseId-ref attribute on the import tag will initialize the specific Kie Objects (Kbase/KSessions) and import these objects into the spring context.
<kie:import releaseId-ref="namedKieSession"/>
<kie:releaseId id="namedKieSession" groupId="org.drools"
artifactId="named-kiesession" version="7.23.0.Final"/>
Kie Scanning feature can be enabled for KieBase’s imported with a specific releaseId. This feature is currently not available for global imports.
<kie:import releaseId-ref="namedKieSession"
enableScanner="true" scannerInterval="1000"/>
<kie:releaseId id="namedKieSession" groupId="org.drools"
artifactId="named-kiesession" version="7.23.0.Final"/>
If the scanner is defined and enabled, an implicit KieScanner object is created and inserted into the spring context. It can be retrived from the spring context.
// the implicit name would be releaseId#scanner
KieScanner releaseIdScanner = context.getBean("namedKieSession#scanner", KieScanner.class);
releaseIdScanner.scanNow();
kie-ci must be available on the classpath for the releaseId importing feature to work. |
13.2.7. Annotations
@KContainer, @KBase and @KSession all support an optional 'name' attribute. Spring typically does "get" when it injects, all injections receive the same instance for the same set of annotations. the 'name' annotation forces a unique instance for each name, although all instance for that name will be identity equals.
13.2.7.1. @KReleaseId
Used to bind an instance to a specific version of a KieModule. If kie-ci is on the classpath this will resolve dependencies automatically, downloading from remote repositories.
13.2.7.2. @KContainer
@KContainer
private KieContainer kContainer;
@KContainer
@KReleaseId(groupId = "jar1", artifactId = "art1", version = "1.1")
private KieContainer kContainer;
@KContainer(name = "kc1")
@KReleaseId(groupId = "jar1", artifactId = "art1", version = "1.1")
private KieContainer kContainer;
13.2.7.3. @KBase
The default argument, if given, maps to the value attribute and specifies the name of the KieBase from the spring xml file.
@KBase
private KieBase kbase;
@KBase
@KReleaseId( groupId = "jar1", artifactId = "art1", version = "1.0")
private KieBase kbase;
@KBase("kbase1")
@KReleaseId( groupId = "jar1", artifactId = "art1", version = "1.0")
private KieBase kbase1v10;
@KBase("kbase1")
@KReleaseId( groupId = "jar1", artifactId = "art1", version = "1.1")
private KieBase kbase1v11;
@KSession("ksession1")
@KReleaseId( groupId = "jar1", artifactId = "art1", version = "1.0")
private KieSession ksession11kb2;
@KSession("ksession1")
@KReleaseId( groupId = "jar1", artifactId = "art1", version = "1.1")
private KieSession ksession11kb2;
13.2.7.4. @KSession for KieSession
The default argument, if given, maps to the value attribute and specifies the name of the KieSession from the kmodule.xml or spring xml file
@KSession
private KieSession ksession;
@KSession
@KReleaseId( groupId = "jar1", artifactId = "art1", version = "1.0")
private KieSession ksession;
@KSession("ksession1")
@KReleaseId( groupId = "jar1", artifactId = "art1", version = "1.0")
private KieSession ksessionv10;
@KSession("ksession1")
@KReleaseId( groupId = "jar1", artifactId = "art1", version = "1.1")
private KieSession ksessionv11;
@KSession("ksession1")
@KReleaseId( groupId = "jar1", artifactId = "art1", version = "1.0")
private KieSession ksession1ks1
@KSession("ksession1")
@KReleaseId( groupId = "jar1", artifactId = "art1", version = "1.0")
private KieSession ksession1ks2
13.2.7.5. @KSession for StatelessKieSession
The default argument, if given, maps to the value attribute and specifies the name of the KieSession from the kmodule.xml or spring xml file.
@KSession
private StatelessKieSession ksession;
@KSession
@KReleaseId( groupId = "jar1", artifactId = "art1", version = "1.0")
private StatelessKieSession ksession;
@KSession("ksession1")
@KReleaseId( groupId = "jar1", rtifactId = "art1", version = "1.0")
private StatelessKieSession ksessionv10;
@KSession("ksession1")
@KReleaseId( groupId = "jar1", rtifactId = "art1", version = "1.1")
private StatelessKieSession ksessionv11;
@KSession(value="ksession1", name="ks1")
@KReleaseId( groupId = "jar1", artifactId = "art1", version = "1.0")
private StatelessKieSession ksession1ks1
@KSession(value="ksession1", name="ks2")
@KReleaseId( groupId = "jar1", artifactId = "art1", version = "1.0")
private StatelessKieSession ksession1ks2
13.2.7.6. IMPORTANT NOTE
When annotations are used, For proper initialization of the kmodule objects (kbase/ksession), it is mandatory for either a bean of type org.kie.spring.annotations.KModuleAnnotationPostProcessor
be defined
<bean id="kiePostProcessor"
class="org.kie.spring.annotations.KModuleAnnotationPostProcessor"/>
<context:component-scan base-package="org.kie.spring.annotations"/>
The post processor is different when annotations are used. |
13.2.8. Event Listeners
Drools supports adding 3 types of listeners to KieSessions - AgendaListener, WorkingMemoryListener, ProcessEventListener
The kie-spring module allows you to configure these listeners to KieSessions using XML tags. These tags have identical names as the actual listener interfaces i.e., <kie:agendaEventListener….>, <kie:ruleRuntimeEventListener….> and <kie:processEventListener….>.
kie-spring provides features to define the listeners as standalone (individual) listeners and also to define them as a group.
13.2.8.2. Attributes:
Attribute | Required | Description |
---|---|---|
ref |
No |
A reference to another declared bean. |
<bean id="mock-agenda-listener" class="mocks.MockAgendaEventListener"/>
<bean id="mock-rr-listener" class="mocks.MockRuleRuntimeEventListener"/>
<bean id="mock-process-listener" class="mocks.MockProcessEventListener"/>
<kie:kmodule id="listeners_kmodule">
<kie:kbase name="drl_kiesample" packages="drl_kiesample">
<kie:ksession name="ksession2">
<kie:agendaEventListener ref="mock-agenda-listener"/>
<kie:processEventListener ref="mock-process-listener"/>
<kie:ruleRuntimeEventListener ref="mock-rr-listener"/>
</kie:ksession>
</kie:kbase>
</kie:kmodule>
<bean id="kiePostProcessor"
class="org.kie.spring.KModuleBeanFactoryPostProcessor"/>
13.2.8.3. Nested Elements:
-
bean
-
class = String
-
name = String (optional)
-
<kie:kmodule id="listeners_module">
<kie:kbase name="drl_kiesample" packages="drl_kiesample">
<kie:ksession name="ksession1">
<kie:agendaEventListener>
<bean class="mocks.MockAgendaEventListener"/>
</kie:agendaEventListener>
</kie:ksession>
</kie:kbase>
</kie:kmodule>
<bean id="kiePostProcessor"
class="org.kie.spring.KModuleBeanFactoryPostProcessor"/>
13.2.8.4. Empty Tag : Declaration with no 'ref' and without a nestedbean
When a listener is defined without a reference to a implementing bean and does not contain a nested bean, <drools:ruleRuntimeEventListener/> the underlying implementation adds the Debug version of the listener defined in the API.
The debug listeners print the corresponding Event toString message to _System.err. _
<bean id="mock-agenda-listener" class="mocks.MockAgendaEventListener"/>
<bean id="mock-rr-listener" class="mocks.MockRuleRuntimeEventListener"/>
<bean id="mock-process-listener" class="mocks.MockProcessEventListener"/>
<kie:kmodule id="listeners_module">
<kie:kbase name="drl_kiesample" packages="drl_kiesample">
<kie:ksession name="ksession2">
<kie:agendaEventListener />
<kie:processEventListener />
<kie:ruleRuntimeEventListener />
</kie:ksession>
</kie:kbase>
</kie:kmodule>
<bean id="kiePostProcessor"
class="org.kie.spring.KModuleBeanFactoryPostProcessor"/>
13.2.8.5. Mix and Match of different declaration styles
The drools-spring module allows you to mix and match the different declarative styles within the same KieSession. The below sample provides more clarity.
<bean id="mock-agenda-listener" class="mocks.MockAgendaEventListener"/>
<bean id="mock-rr-listener" class="mocks.MockRuleRuntimeEventListener"/>
<bean id="mock-process-listener" class="mocks.MockProcessEventListener"/>
<kie:kmodule id="listeners_module">
<kie:kbase name="drl_kiesample" packages="drl_kiesample">
<kie:ksession name="ksession1">
<kie:agendaEventListener>
<bean class="org.kie.spring.mocks.MockAgendaEventListener"/>
</kie:agendaEventListener>
</kie:ksession>
<kie:ksession name="ksession2">
<kie:agendaEventListener ref="mock-agenda-listener"/>
<kie:processEventListener ref="mock-process-listener"/>
<kie:ruleRuntimeEventListener ref="mock-rr-listener"/>
</kie:ksession>
</kie:kbase>
</kie:kmodule>
<bean id="kiePostProcessor"
class="org.kie.spring.KModuleBeanFactoryPostProcessor"/>
13.2.8.6. Defining multiple listeners of the same type
It is also valid to define multiple beans of the same event listener types for a KieSession.
<bean id="mock-agenda-listener" class="mocks.MockAgendaEventListener"/>
<kie:kmodule id="listeners_module">
<kie:kbase name="drl_kiesample" packages="drl_kiesample">
<kie:ksession name="ksession1">
<kie:agendaEventListener ref="mock-agenda-listener"/>
<kie:agendaEventListener>
<bean class="org.kie.spring.mocks.MockAgendaEventListener"/>
</kie:agendaEventListener>
</kie:ksession>
</kie:kbase>
</kie:kmodule>
<bean id="kiePostProcessor"
class="org.kie.spring.KModuleBeanFactoryPostProcessor"/>
13.2.8.7. Defining a Group of listeners:
drools-spring allows for grouping of listeners. This is particularly useful when you define a set of listeners and want to attach them to multiple sessions. The grouping feature is also very useful, when we define a set of listeners for 'testing' and then want to switch them for 'production' use.
13.2.8.8. Attributes:
Attribute | Required | Description |
---|---|---|
ID |
yes |
Unique identifier |
13.2.8.9. Nested Elements:
-
kie:agendaEventListener…
-
kie:ruleRuntimeEventListener…
-
kie:processEventListener…
The above mentioned child elements can be declared in any order. Only one declaration of each type is allowed in a group. |
13.2.8.10. Example:
<bean id="mock-agenda-listener" class="mocks.MockAgendaEventListener"/>
<bean id="mock-rr-listener" class="mocks.MockRuleRuntimeEventListener"/>
<bean id="mock-process-listener" class="mocks.MockProcessEventListener"/>
<kie:kmodule id="listeners_module">
<kie:kbase name="drl_kiesample" packages="drl_kiesample">
<kie:ksession name="statelessWithGroupedListeners" type="stateless"
listeners-ref="debugListeners"/>
</kie:kbase>
</kie:kmodule>
<kie:eventListeners id="debugListeners">
<kie:agendaEventListener ref="mock-agenda-listener"/>
<kie:processEventListener ref="mock-process-listener"/>
<kie:ruleRuntimeEventListener ref="mock-rr-listener"/>
</kie:eventListeners>
<bean id="kiePostProcessor"
class="org.kie.spring.KModuleBeanFactoryPostProcessor"/>
13.2.9. Loggers
Drools supports adding 2 types of loggers to KieSessions - ConsoleLogger, FileLogger.
The kie-spring module allows you to configure these loggers to KieSessions using XML tags. These tags have identical names as the actual logger interfaces i.e., <kie:consoleLogger….> and <kie:fileLogger….>.
13.2.9.1. Defining a console logger:
A console logger can be attached to a KieSession by using the <kie:consoleLogger/> tag. This tag has no attributes and must be present directly under a <kie:ksession….> element.
<kie:kmodule id="loggers_module">
<kie:kbase name="drl_kiesample" packages="drl_kiesample">
<kie:ksession name="ConsoleLogger-statefulSession" type="stateful">
<kie:consoleLogger/>
</kie:ksession>
</kie:kbase>
</kie:kmodule>
<bean id="kiePostProcessor"
class="org.kie.spring.KModuleBeanFactoryPostProcessor"/>
13.2.9.2. Defining a file logger:
A file logger can be attached to a KieSession by using the <kie:fileLogger/> tag. This tag has the following attributes and must be present directly under a <kie:ksession….> element.
Attribute | Required | Description |
---|---|---|
ID |
yes |
Unique identifier |
file |
yes |
Path to the actual file on the disk |
threaded |
no |
Defaults to false. Valid values are 'true’or 'false' |
interval |
no |
Integer. Specifies the interval for flushing the contents from memory to the disk. |
<kie:kmodule id="loggers_module">
<kie:kbase name="drl_kiesample" packages="drl_kiesample">
<kie:ksession name="ConsoleLogger-statefulSession" type="stateful">
<kie:fileLogger id="fl_logger" file="#{ systemProperties['java.io.tmpdir'] }/log1"/>
<kie:fileLogger id="tfl_logger" file="#{ systemProperties['java.io.tmpdir'] }/log2"
threaded="true" interval="5"/>
</kie:ksession>
</kie:kbase>
</kie:kmodule>
<bean id="kiePostProcessor"
class="org.kie.spring.KModuleBeanFactoryPostProcessor"/>
13.2.9.3. Closing a FileLogger
To prevent leaks, it is advised to close the _<kie:fileLogger …> _ programmatically.
LoggerAdaptor adaptor = (LoggerAdaptor) context.getBean("fl_logger");
adaptor.close();
13.2.10. Defining Batch Commands
A <kie:batch> element can be used to define a set of batch commands for a given ksession.This tag has no attributes and must be present directly under a <kie:ksession….> element. The commands supported are
-
insert-object
-
ref = String (optional)
-
Anonymous bean
-
-
set-global
-
identifier = String (required)
-
reg = String (optional)
-
Anonymous bean
-
-
fire-all-rules
-
max : n
-
-
fire-until-halt
-
start-process
-
parameter
-
identifier = String (required)
-
ref = String (optional)
-
Anonymous bean
-
-
-
signal-event
-
ref = String (optional)
-
event-type = String (required)
-
process-instance-id =n (optional)
-
<kie:kmodule id="batch_commands_module">
<kie:kbase name="drl_kiesample" packages="drl_kiesample">
<kie:ksession name="ksessionForCommands" type="stateful">
<kie:batch>
<kie:insert-object ref="person2"/>
<kie:set-global identifier="persons" ref="personsList"/>
<kie:fire-all-rules max="10"/>
</kie:batch>
</kie:ksession>
</kie:kbase>
</kie:kmodule>
<bean id="kiePostProcessor"
class="org.kie.spring.KModuleBeanFactoryPostProcessor"/>
13.2.11. Persistence
-
jpa-persistence
-
transaction-manager
-
ref = String
-
-
entity-manager-factory
-
ref = String
-
-
<kie:kstore id="kstore" /> <!-- provides KnowledgeStoreService implementation -->
<bean id="myEmf"
class="org.springframework.orm.jpa.LocalContainerEntityManagerFactoryBean">
<property name="dataSource" ref="ds" />
<property name="persistenceUnitName"
value="org.drools.persistence.jpa.local" />
</bean>
<bean id="txManager" class="org.springframework.orm.jpa.JpaTransactionManager">
<property name="entityManagerFactory" ref="myEmf" />
</bean>
<kie:kmodule id="persistence_module">
<kie:kbase name="drl_kiesample" packages="drl_kiesample">
<kie:ksession name="jpaSingleSessionCommandService">
<kie:configuration>
<kie:jpa-persistence>
<kie:transaction-manager ref="txManager"/>
<kie:entity-manager-factory ref="myEmf"/>
</kie:jpa-persistence>
</kie:configuration>
</kie:ksession>
</kie:kbase>
</kie:kmodule>
<bean id="kiePostProcessor"
class="org.kie.spring.KModuleBeanFactoryPostProcessor"/>
13.2.12. Leveraging Other Spring Features
This section provides details on leveraging other standard spring features when integrating with Drools Expert.
13.2.12.1. Using Spring Expressions (Spel)
<kie:kmodule id="batch_commands_module">
<kie:kbase name="drl_kiesample" packages="#{packageRepository.packages}">
<kie:ksession name="ksessionForCommands" type="stateful"/>
</kie:kbase>
</kie:kmodule>
<bean id="kiePostProcessor"
class="org.kie.spring.KModuleBeanFactoryPostProcessor"/>
<bean id="packageRepository" class="sample.package.class.PackageRepo">
<property name="packages" value="drl_kiesample3">
</bean>
<kie:kmodule id="loggers_module">
<kie:kbase name="drl_kiesample" packages="drl_kiesample">
<kie:ksession name="ConsoleLogger-statefulSession" type="stateful">
<kie:fileLogger id="fl" file="#{ systemProperties['java.io.tmpdir'] }/log1"/>
<kie:fileLogger id="tfl" file="#{ systemProperties['java.io.tmpdir'] }/log2"
threaded="true" interval="5"/>
</kie:ksession>
</kie:kbase>
</kie:kmodule>
<bean id="kiePostProcessor"
class="org.kie.spring.KModuleBeanFactoryPostProcessor"/>
13.2.12.2. Using Spring Profiles
Spring 3.1 introduces a new profile attribute to the beans element of the spring-beans schema. This attribute acts as a switch when enabling and disabling profiles in different environments. One potential use of this attribute can be to have the same kbase defined with debug loggers in 'dev' environment and without loggers in 'prod' environment.
The below code snippet illustrates the concept of 'profiles'.
<beans xmlns="http://www.springframework.org/schema/beans"
xmlns:xsi="http://www.w3.org/2001/XMLSchema-instance"
xmlns:kie="http://drools.org/schema/kie-spring"
xsi:schemaLocation="http://www.springframework.org/schema/beans
http://www.springframework.org/schema/beans/spring-beans-4.0.xsd
http://drools.org/schema/kie-spring http://drools.org/schema/kie-spring.xsd">
<beans profile="development">
<kie:kmodule id="test-kmodule">
<kie:kbase name="drl_kiesample" packages="drl_kiesample">
<kie:ksession name="ksession1" type="stateless">
<kie:consoleLogger />
</kie:ksession>
</kie:kbase>
</kie:kmodule>
...
</beans>
<beans profile="production">
<kie:kmodule id="test-kmodule">
<kie:kbase name="drl_kiesample" packages="drl_kiesample">
<kie:ksession name="ksession1" type="stateless"/>
</kie:kbase>
</kie:kmodule>
...
</beans>
</beans>
As shown above, the Spring XML contains the definition of the profiles. While loading the ApplicationContext you have to tell Spring which profile you’re loading.
There are several ways of selecting your profile and the most useful is by using the "spring.profiles.active" system property.
System.setProperty("spring.profiles.active", "development");
ApplicationContext ctx = new ClassPathXmlApplicationContext("beans.xml");
Obviously, it is not a good practice to hard code things as shown above and the recommended practice is to keep the system properties definitions independent of the application.
-Dspring.profiles.active="development"
The profiles can also be loaded and enabled programmtically
...
GenericXmlApplicationContext ctx = new GenericXmlApplicationContext("beans.xml");
ConfigurableEnvironment env = ctx.getEnvironment();
env.setActiveProfiles("development");
ctx.refresh();
...
13.3. Integration with jBPM Human Task
This chapter describes the infrastructure used when configuring a human task server with Spring as well as a little bit about the infrastructure used when doing this.
13.3.1. How to configure Spring with jBPM Human task
The jBPM human task server can be configured to use Spring persistence. The following is a dependency graph for a configuration that uses local transactions and Spring’s thread-safe EntityManager proxy:
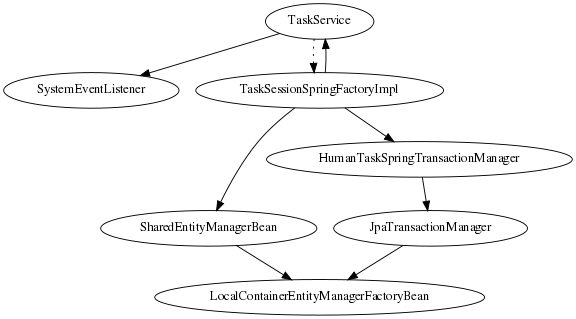
A TaskService
instance is dependent on two other bean types: a drools SystemEventListener
bean as well as a TaskSessionSpringFactoryImpl
bean.
The TaskSessionSpringFactoryImpl
bean is howerver not injected into the TaskService
bean because this would cause a circular dependency.
To solve this problem, when the TaskService
bean is injected into the TaskSessionSpringFactoryImpl
bean, the setter method used secretly injects the TaskSessionSpringFactoryImpl
instance back into the TaskService
bean and initializes the TaskService
bean as well.
The TaskSessionSpringFactoryImpl
bean is responsible for creating all the internal instances in human task that deal with transactions and persistence context management.
Besides a TaskService
instance, this bean also requires a transaction manager and a persistence context to be injected.
Specifically, it requires an instance of a HumanTaskSpringTransactionManager
bean (as a transaction manager) and an instance of a SharedEntityManagerBean
bean (as a persistence context instance).
We also use some of the standard Spring beans in order to configure persistence: there’s a bean to hold the EntityManagerFactory
instance as well as the SharedEntityManagerBean
instance.
The SharedEntityManagerBean
provides a shared, thread-safe proxy for the actual EntityManager
.
The HumanTaskSpringTransactionManager
bean serves as a wrapper around the Spring transaction manager, in this case the JpaTransactionManager
.
An instance of a JpaTransactionManager
bean is also instantiated because of this.
<?xml version="1.0" encoding="UTF-8"?>
<beans xmlns="http://www.springframework.org/schema/beans"
xmlns:xsi="http://www.w3.org/2001/XMLSchema-instance"
xmlns:jbpm="http://drools.org/schema/drools-spring"
xsi:schemaLocation="http://www.springframework.org/schema/beans http://www.springframework.org/schema/beans/spring-beans-3.0.xsd
http://drools.org/schema/drools-spring org/drools/container/spring/drools-spring-1.2.0.xsd">
<!-- persistence & transactions-->
<bean id="htEmf" class="org.springframework.orm.jpa.LocalContainerEntityManagerFactoryBean">
<property name="persistenceUnitName" value="org.jbpm.task" />
</bean>
<bean id="htEm" class="org.springframework.orm.jpa.support.SharedEntityManagerBean">
<property name="entityManagerFactory" ref="htEmf"/>
</bean>
<bean id="jpaTxMgr" class="org.springframework.orm.jpa.JpaTransactionManager">
<property name="entityManagerFactory" ref="htEmf" />
<!-- this must be true if using the SharedEntityManagerBean, and false otherwise -->
<property name="nestedTransactionAllowed" value="true"/>
</bean>
<bean id="htTxMgr" class="org.drools.container.spring.beans.persistence.HumanTaskSpringTransactionManager">
<constructor-arg ref="jpaTxMgr" />
</bean>
<!-- human-task beans -->
<bean id="systemEventListener" class="org.drools.SystemEventListenerFactory" factory-method="getSystemEventListener" />
<bean id="taskService" class="org.jbpm.task.service.TaskService" >
<property name="systemEventListener" ref="systemEventListener" />
</bean>
<bean id="springTaskSessionFactory" class="org.jbpm.task.service.persistence.TaskSessionSpringFactoryImpl"
init-method="initialize" depends-on="taskService" >
<!-- if using the SharedEntityManagerBean, make sure to enable nested transactions -->
<property name="entityManager" ref="htEm" />
<property name="transactionManager" ref="htTxMgr" />
<property name="useJTA" value="false" />
<property name="taskService" ref="taskService" />
</bean>
</beans>
When using the SharedEntityManagerBean
instance, it’s important to configure the Spring transaction manager to use nested transactions.
This is because the SharedEntityManagerBean
is a transactional persistence context and will close the persistence context after every operation.
However, the human task server needs to be able to access (persisted) entities after operations.
Nested transactions allow us to still have access to entities that otherwise would have been detached and are no longer accessible, especially when using an ORM framework that uses lazy-initialization of entities.
Also, while the TaskSessionSpringFactoryImpl
bean takes an “useJTA”
parameter, at the moment, JTA transactions with Spring have not yet been fully tested.
14. Integration with Aries Blueprint
14.1. Integration with Drools Expert
In this section we will explain the kie namespace.
14.1.1. KieModule
The <kie:kmodule> defines a collection of KieBase and associated KieSession’s. The kmodule tag has one MANDATORY parameter 'id'.
Attribute | Description | Required |
---|---|---|
id |
Bean’s id is the name to be referenced from other beans. Standard Blueprint ID semantics applies. |
Yes |
A kmodule tag can contain only the following tags as children.
-
kie:kbase
Refer to the documentation of kmodule.xml in the Drools Expert documentation for detailed explanation of the need for kmodule.
14.1.2. KieBase
14.1.2.1. <kie:kbase>'s parameters as attributes:
Attribute | Description | Required |
---|---|---|
name |
Name of the KieBase |
Yes |
packages |
Comma separated list of resource packages to be included in this kbase |
No |
includes |
kbase names to be included. All resources from the corresponding kbase are included in this kbase. |
No |
default |
Boolean (TRUE/FALSE). Default kbase, if not provided, it is assumed to be FALSE |
No |
scope |
prototype | singleton. If not provided assumed to be singleton (default) |
No |
eventProcessingMode |
Event Processing Mode. Valid options are STREAM, CLOUD |
No |
equalsBehavior |
Valid options are IDENTITY, EQUALITY |
No |
14.1.2.3. <kie:kbase>'s definition example
A kmodule can contain multiple (1..n) kbase elements.
<kie:kmodule id="sample_module">
<kie:kbase name="kbase1" packages="org.drools.blueprint.sample">
...
</kie:kbase>
</kie:kmodule>
14.1.2.4. Blueprint Bean Scope (for KieBase and KieSession)
When defining a KieBase or a KieSession, you have the option of declaring a scope for that bean. For example, To force Blueprint to produce a new bean instance each time one is needed, you should declare the bean’s scope attribute to be 'prototype'. Similar way if you want Blueprint to return the same bean instance each time one is needed, you should declare the bean’s scope attribute to be 'singleton'.
14.1.3. KieSessions
<kie:ksession> element defines KieSessions. The same tag is used to define both Stateful (org.kie.api.runtime.KieSession) and Stateless (org.kie.api.runtime.StatelessKieSession) sessions.
14.1.3.1. <kie:ksession>'s parameters as attributes:
Attribute | Description | Required |
---|---|---|
name |
ksession’s name. |
Yes |
type |
is the session stateful or stateless?. If this attribute is empty or missing, the session is assumed to be of type Stateful. |
No |
default |
Is this the default session? |
No |
scope |
prototype | singleton. If not provided assumed to be singleton (default) |
No |
clockType |
REALTIME or PSEUDO |
No |
listeners-ref |
Specifies the reference to the event listeners group (see 'Defining a Group of listeners' section below). |
No |
<kie:kmodule id="sample-kmodule">
<kie:kbase name="drl_kiesample3" packages="drl_kiesample3">
<kie:ksession name="ksession1" type="stateless"/>
<kie:ksession name="ksession2"/>
</kie:kbase>
</kie:kmodule>
14.1.4. Kie:ReleaseId
14.1.4.1. <kie:releaseId>'s parameters as attributes:
Attribute | Description | Required |
---|---|---|
id |
Bean’s id is the name to be referenced from other beans. Standard Blueprint ID semantics applies. |
Yes |
groupId |
groupId from Maven GAV |
Yes |
artifactId |
artifactId from Maven GAV |
Yes |
version |
version from Maven GAV |
Yes |
<kie:releaseId id="beanId" groupId="org.kie.blueprint"
artifactId="named-artifactId" version="1.0.0-SNAPSHOT"/>
14.1.5. Kie:Import
Starting with version 6.5, kie-aries-blueprint allows for importing of kie objects from kjars found on the classpath. Two modes of importing the kie objects are currently supported.
Attribute | Description | Required |
---|---|---|
releaseId |
Reference to a Bean ID. Standard Blueprint ID semantics applies. |
No |
enableScanner |
Enable Scanner. This attribute is used only if 'releaseId' is specified. |
No |
scannerInterval |
Scanning Interval in milli seconds. This attribute is used only if 'releaseId' is specified. |
No |
14.1.5.1. Global Import
The import tag will force the automatic scan of all the jars on the classpath, initialize the Kie Objects (Kbase/KSessions) and import these objects into the blueprint context.
<kie:import />
14.1.5.2. Specific Import - ReleaseId
Using the releaseId-ref attribute on the import tag will initialize the specific Kie Objects (Kbase/KSessions) and import these objects into the blueprint context.
<kie:import releaseId-ref="namedKieSession"/>
<kie:releaseId id="namedKieSession" groupId="org.drools"
artifactId="named-kiesession" version="7.23.0.Final"/>
Kie Scanning feature can be enabled for KieBase’s imported with a specific releaseId. This feature is currently not available for global imports.
<kie:import releaseId-ref="namedKieSession"
enableScanner="true" scannerInterval="1000"/>
<kie:releaseId id="namedKieSession" groupId="org.drools"
artifactId="named-kiesession" version="7.23.0.Final"/>
If the scanner is defined and enabled, an implicit KieScanner object is created and inserted into the blueprint container. It can be programmatically retrived from the blueprint container using the -scanner suffix.
// the implicit name would be releaseId-scanner
KieScanner releaseIdScanner = (KieScanner)container.getComponentInstance("namedKieSession-scanner");
releaseIdScanner.scanNow();
kie-ci must be available on the classpath for the releaseId importing feature to work. |
14.1.6. Event Listeners
Drools supports adding 3 types of listeners to KieSessions - AgendaListener, WorkingMemoryListener, ProcessEventListener
The kie-aries-blueprint module allows you to configure these listeners to KieSessions using XML tags. These tags have identical names as the actual listener interfaces i.e., <kie:agendaEventListener….>, <kie:ruleRuntimeEventListener….> and <kie:processEventListener….>.
kie-aries-blueprint provides features to define the listeners as standalone (individual) listeners and also to define them as a group.
14.1.6.2. Attributes:
Attribute | Required | Description |
---|---|---|
ref |
No |
A reference to another declared bean. |
<bean id="mock-agenda-listener" class="mocks.MockAgendaEventListener"/>
<bean id="mock-rr-listener" class="mocks.MockRuleRuntimeEventListener"/>
<bean id="mock-process-listener" class="mocks.MockProcessEventListener"/>
<kie:kmodule id="listeners_kmodule">
<kie:kbase name="drl_kiesample" packages="drl_kiesample">
<kie:ksession name="ksession2">
<kie:agendaEventListener ref="mock-agenda-listener"/>
<kie:processEventListener ref="mock-process-listener"/>
<kie:ruleRuntimeEventListener ref="mock-rr-listener"/>
</kie:ksession>
</kie:kbase>
</kie:kmodule>
14.1.6.3. Defining multiple listeners of the same type
It is also valid to define multiple beans of the same event listener types for a KieSession.
<bean id="mock-agenda-listener1" class="mocks.MockAgendaEventListener"/>
<bean id="mock-agenda-listener2" class="mocks.MockAgendaEventListener"/>
<kie:kmodule id="listeners_module">
<kie:kbase name="drl_kiesample" packages="drl_kiesample">
<kie:ksession name="ksession1">
<kie:agendaEventListener ref="mock-agenda-listener1"/>
<kie:agendaEventListener ref="mock-agenda-listener2"/>
</kie:ksession>
</kie:kbase>
</kie:kmodule>
14.1.6.4. Defining a Group of listeners:
kie-aries-blueprinty allows for grouping of listeners. This is particularly useful when you define a set of listeners and want to attach them to multiple sessions. The grouping feature is also very useful, when we define a set of listeners for 'testing' and then want to switch them for 'production' use.
14.1.6.5. Attributes:
Attribute | Required | Description |
---|---|---|
ID |
yes |
Unique identifier |
14.1.6.6. Nested Elements:
-
kie:agendaEventListener…
-
kie:ruleRuntimeEventListener…
-
kie:processEventListener…
The above mentioned child elements can be declared in any order. Only one declaration of each type is allowed in a group. |
14.1.6.7. Example:
<bean id="mock-agenda-listener" class="mocks.MockAgendaEventListener"/>
<bean id="mock-rr-listener" class="mocks.MockRuleRuntimeEventListener"/>
<bean id="mock-process-listener" class="mocks.MockProcessEventListener"/>
<kie:kmodule id="listeners_module">
<kie:kbase name="drl_kiesample" packages="drl_kiesample">
<kie:ksession name="statelessWithGroupedListeners" type="stateless"
listeners-ref="debugListeners"/>
</kie:kbase>
</kie:kmodule>
<kie:eventListeners id="debugListeners">
<kie:agendaEventListener ref="mock-agenda-listener"/>
<kie:processEventListener ref="mock-process-listener"/>
<kie:ruleRuntimeEventListener ref="mock-rr-listener"/>
</kie:eventListeners>
14.1.7. Loggers
Drools supports adding 2 types of loggers to KieSessions - ConsoleLogger, FileLogger.
The kie-aries-blueprint module allows you to configure these loggers to KieSessions using XML tags. These tags have identical names as the actual logger interfaces i.e., <kie:consoleLogger….> and <kie:fileLogger….>.
14.1.7.1. Defining a console logger:
A console logger can be attached to a KieSession by using the <kie:consoleLogger/> tag. This tag has no attributes and must be present directly under a <kie:ksession….> element.
<kie:kmodule id="loggers_module">
<kie:kbase name="drl_kiesample" packages="drl_kiesample">
<kie:ksession name="ConsoleLogger-statefulSession" type="stateful">
<kie:consoleLogger/>
</kie:ksession>
</kie:kbase>
</kie:kmodule>
14.1.7.2. Defining a file logger:
A file logger can be attached to a KieSession by using the <kie:fileLogger/> tag. This tag has the following attributes and must be present directly under a <kie:ksession….> element.
Attribute | Required | Description |
---|---|---|
ID |
yes |
Unique identifier |
file |
yes |
Path to the actual file on the disk |
threaded |
no |
Defaults to false. Valid values are 'true’or 'false' |
interval |
no |
Integer. Specifies the interval for flushing the contents from memory to the disk. |
<kie:kmodule id="loggers_module">
<kie:kbase name="drl_kiesample" packages="drl_kiesample">
<kie:ksession name="ConsoleLogger-statefulSession" type="stateful">
<kie:fileLogger id="fl_logger" file="#{ systemProperties['java.io.tmpdir'] }/log1"/>
<kie:fileLogger id="tfl_logger" file="#{ systemProperties['java.io.tmpdir'] }/log2"
threaded="true" interval="5"/>
</kie:ksession>
</kie:kbase>
</kie:kmodule>
14.1.7.3. Closing a FileLogger
To prevent leaks, it is advised to close the <kie:fileLogger …> programmatically.
LoggerAdaptor adaptor = (LoggerAdaptor) container.getComponentInstance("fl_logger");
adaptor.close();
14.1.8. Defining Batch Commands
A <kie:batch> element can be used to define a set of batch commands for a given ksession.This tag has no attributes and must be present directly under a <kie:ksession….> element. The commands supported are
-
insert-object
-
ref = String (optional)
-
Anonymous bean
-
-
set-global
-
identifier = String (required)
-
reg = String (optional)
-
Anonymous bean
-
-
fire-all-rules
-
max : n
-
-
fire-until-halt
-
start-process
-
parameter
-
identifier = String (required)
-
ref = String (optional)
-
Anonymous bean
-
-
-
signal-event
-
ref = String (optional)
-
event-type = String (required)
-
process-instance-id =n (optional)
-
<kie:kmodule id="batch_commands_module">
<kie:kbase name="drl_kiesample" packages="drl_kiesample">
<kie:ksession name="ksessionForCommands" type="stateful">
<kie:batch>
<kie:insert-object ref="person2"/>
<kie:set-global identifier="persons" ref="personsList"/>
<kie:fire-all-rules max="10"/>
</kie:batch>
</kie:ksession>
</kie:kbase>
</kie:kmodule>
15. Android Integration
15.1. Integration with Drools Expert
Drools Android integration comes in two flavors, with or without the drools-compiler dependency. Without the drools-compiler dependency the KIE bases are pre-serialized at buildtime using the kie-maven-plugin. They can be then deserialized using the API, or directly injected using Roboguice. When using the drools-compiler dependency there are two options: (1) standard KieContainer API or (2) CDI-like injection with Roboguice.
15.1.1. Pre-serialized Rules
It is possible to use Drools without the drools-compiler dependency, which results in a smaller apk, by pre-serializing the compiled KIE bases during build-time and then de-serializing them at runtime.
15.1.1.1. Maven Configuration
The KieBase must be serialized during build time using kie-maven-plugin.
<dependencyManagement>
<dependencies>
<dependency>
<groupId>org.drools</groupId>
<artifactId>drools-bom</artifactId>
<version>7.23.0.Final</version>
<type>pom</type>
<scope>import</scope>
</dependencies>
</dependencyManagement>
<dependencies>
<dependency>
<groupId>org.drools</groupId>
<artifactId>drools-android</artifactId>
<exclusions>
<exclusion>
<groupId>org.slf4j</groupId>
<artifactId>slf4j-api</artifactId>
</exclusions>
</dependency>
<dependency>
<groupId>org.drools</groupId>
<artifactId>drools-core</artifactId>
</dependency>
</dependencies>
<build>
<plugins>
<plugin>
<groupId>org.kie</groupId>
<artifactId>kie-maven-plugin</artifactId>
<version>7.23.0.Final</version>
<configuration>
<kiebases>
<kiebase>HelloKB</kiebase>
</kiebases>
<resDirectory>${basedir}/src/main/res/raw</resDirectory>
</configuration>
<executions>
<execution>
<id>touch</id>
<goals>
<goal>touch</goal>
</goals>
<phase>initialize</phase>
</execution>
<execution>
<id>compile-kbase</id>
<goals>
<goal>build</goal>
</goals>
<phase>compile</phase>
</execution>
<execution>
<id>serialize</id>
<goals>
<goal>serialize</goal>
</goals>
<phase>compile</phase>
</execution>
</executions>
</plugin>
</plugins>
</build>
15.1.1.2. Loading the KieBase
The KieBase must be de-serialized before creating the sessions.
private class LoadKieBaseTask extends AsyncTask<InputStream, Void, KieBase> {
@Override
protected KieBase doInBackground(InputStream... params) {
try {
logger.debug("Loading KIE base");
final KnowledgeBase kbase = KnowledgeBaseFactory.newKnowledgeBase();
kbase.addKnowledgePackages((List<KnowledgePackage>) DroolsStreamUtils.streamIn(params[0]));
return kbase;
}catch(Exception e) {
logger.error("Drools exception", e);
return null;
}
}
}
15.1.2. KieContainer API with drools-compiler dependency
With the drools-compiler dependency standard KieContainer API can be used. This comes at a cost of a larger apk. To avoid the 65K limit, multidex (or proguard) can be used.
15.1.2.1. Maven Configuration with drools-compiler and multidex
The kie-maven-plugin must be configured to build the kiebase. Multidex must be used to allow for the increased dependencies. There are also some settings for merging various Drools XML files within the apk.
<dependencyManagement>
<dependencies>
<dependency>
<groupId>org.drools</groupId>
<artifactId>drools-bom</artifactId>
<version>7.23.0.Final</version>
<type>pom</type>
<scope>import</scope>
</dependencies>
</dependencyManagement>
<dependencies>
<dependency>
<groupId>org.drools</groupId>
<artifactId>drools-android</artifactId>
<exclusions>
<exclusion>
<groupId>org.slf4j</groupId>
<artifactId>slf4j-api</artifactId>
</exclusion>
</exclusions>
</dependency>
<dependency>
<groupId>org.drools</groupId>
<artifactId>drools-compiler</artifactId>
<exclusions>
<exclusion>
<groupId>org.slf4j</groupId>
<artifactId>slf4j-api</artifactId>
</exclusion>
<exclusion>
<groupId>xmlpull</groupId>
<artifactId>xmlpull</artifactId>
</exclusion>
<exclusion>
<groupId>xpp3</groupId>
<artifactId>xpp3_min</artifactId>
</exclusion>
<exclusion>
<groupId>org.slf4j</groupId>
<artifactId>slf4j-api</artifactId>
</exclusion>
<exclusion>
<groupId>org.eclipse.jdt.core.compiler</groupId>
<artifactId>ecj</artifactId>
</exclusion>
</exclusions>
</dependency>
</dependencies>
<build>
<plugins>
<plugin>
<groupId>org.kie</groupId>
<artifactId>kie-maven-plugin</artifactId>
<version>7.23.0.Final</version>
<executions>
<execution>
<id>compile-kbase</id>
<goals>
<goal>build</goal>
</goals>
<phase>compile</phase>
</execution>
</executions>
</plugin>
<plugin>
<groupId>com.simpligility.maven.plugins</groupId>
<artifactId>android-maven-plugin</artifactId>
<version>4.2.1</version>
<extensions>true</extensions>
<configuration>
<sdk>
<platform>21</platform>
</sdk>
<dex>
<coreLibrary>true</coreLibrary>
<jvmArguments><jvmArgument>-Xmx2048m</jvmArgument></jvmArguments>
<multiDex>true</multiDex>
<mainDexList>maindex.txt</mainDexList>
</dex>
<extractDuplicates>true</extractDuplicates>
<apk>
<metaInf>
<includes>
<include>services/**</include>
<include>kmodule.*</include>
<include>HelloKB/**</include>
<include>drools**</include>
<include>maven/${project.groupId}/${project.artifactId}/**</include>
</includes>
</metaInf>
</apk>
</configuration>
</plugin>
</plugins>
</build>
15.2. Integration with Roboguice
15.2.1. Pre-serialized Rules with Roboguice
With Roboguice pre-serialized KIE bases can be injected using the @KBase annotation.
15.2.1.1. Annotations
@KBase supports an optional 'name' attribute. CDI typically does "getOrCreate" when it injects, all injections receive the same instance for the same set of annotations. the 'name' annotation forces a unique instance for each name, although all instances for that name will be identity equals.
15.2.1.2. @KBase
The default argument maps to the value attribute and specifies the name of the KieBase from the kmodule.xml file.
@KBase("kbase1")
private KieBase kbase;
15.2.1.3. AndroidManifest.xml configuration
The Roboguice module needs to be specified in the manifest.
<application
android:largeHeap="true"
android:allowBackup="true"
android:icon="@drawable/ic_launcher"
android:label="@string/app_name"
android:theme="@style/AppTheme">
<meta-data
android:name="roboguice.modules"
android:value="org.drools.android.roboguice.DroolsModule"/>
<activity
android:label="@string/app_name"
android:name="org.drools.examples.android.SplashActivity">
<intent-filter>
<action android:name="android.intent.action.MAIN"/>
<category android:name="android.intent.category.LAUNCHER"/>
</intent-filter>
</activity>
</application>
15.2.2. KieContainer with drools-compiler dependency and Roboguice
With Roboguice and drools-compiler almost the full CDI syntax can be used to inject KieContainers, KieBases, and KieSessions.
15.2.2.1. Annotations
@KContainer, @KBase and @KSession all support an optional 'name' attribute. CDI typically does "getOrCreate" when it injects, all injections receive the same instance for the same set of annotations. the 'name' annotation forces a unique instance for each name, although all instance for that name will be identity equals.
15.2.2.2. @KContainer
@Inject
private KieContainer kContainer;
15.2.2.3. @KBase
The default argument, if given, maps to the value attribute and specifies the name of the KieBase from the kmodule.xml file.
@Inject
private KieBase kbase;
@Inject
@KBase("kbase1")
private KieBase kbase;
15.2.2.4. @KSession for KieSession
@KSession is optional as it can be detected and added by the use of @Inject and variable type inference.
The default argument, if given, maps to the value attribute and specifies the name of the KieSession from the kmodule.xml file
@Inject
private KieSession ksession;
@Inject
@KSession("ksession1")
private KieSession ksession;
15.2.2.5. @KSession for StatelessKieSession
@KSession is optional as it can be detected and added by the use of @Inject and variable type inference.
The default argument, if given, maps to the value attribute and specifies the name of the KieSession from the kmodule.xml file.
@Inject
private StatelessKieSession ksession;
@Inject
@KSession("ksession1")
private StatelessKieSession ksession;
15.2.2.6. AndroidManifest.xml configuration
The Roboguice module needs to be specified in the manifest.
<application
android:largeHeap="true"
android:allowBackup="true"
android:icon="@drawable/ic_launcher"
android:label="@string/app_name"
android:theme="@style/AppTheme">
<meta-data
android:name="roboguice.modules"
android:value="org.drools.android.roboguice.DroolsContainerModule"/>
<activity
android:label="@string/app_name"
android:name="org.drools.examples.android.SplashActivity">
<intent-filter>
<action android:name="android.intent.action.MAIN"/>
<category android:name="android.intent.category.LAUNCHER"/>
</intent-filter>
</activity>
</application>
16. Apache Camel Integration
16.1. Camel
Camel provides a light weight bus framework for getting information into and out of Drools.
Drools introduces two elements to make easy integration.
-
Drools Policy
Augments any JAXB or XStream data loaders. For JAXB it adds drools related paths ot the contextpath, for XStream it adds custom converters and aliases for Drools classes. It also handles setting the ClassLoader to the targeted ksession.
-
Drools Endpoint
Executes the payload against the specified drools session
Drools can be configured like any normal camel component, but notice the policy that wraps the drools related segments. This will route all payloads to ksession1
<bean id="kiePolicy" class="org.kie.camel.component.KiePolicy" />
<camelContext id="camel" xmlns="http://camel.apache.org/schema/spring">
<route>
<from uri="cxfrs://bean://rsServer"/>
<policy ref="kiePolicy">
<unmarshal ref="xstream" />
<to uri="kie:ksession1" />
<marshal ref="xstream" />
</policy>
</route>
</camelContext>
It is possible to not specify the session in the drools endpoint uri, and instead "multiplex" based on an attribute or header. In this example the policy will check either the header field "DroolsLookup" for the named session to execute and if that isn’t specified it’ll check the "lookup" attribute on the incoming payload.
<bean id="kiePolicy" class="org.kie.camel.component.KiePolicy" />
<camelContext id="camel" xmlns="http://camel.apache.org/schema/spring">
<route>
<from uri="cxfrs://bean://rsServer"/>
<policy ref="kiePolicy">
<unmarshal ref="xstream" />
<to uri="kie:dynamic" />
<marshal ref="xstream" />
</policy>
</route>
</camelContext>
public class MyTest extends CamelSpringTestSupport {
@Override
protected AbstractXmlApplicationContext createApplicationContext() {
return new ClassPathXmlApplicationContext("org/drools/camel/component/CxfRsSpring.xml");
}
public void test1() throws Exception {
String cmd = "";
cmd += "<batch-execution lookup=\"ksession1\">\n";
cmd += " <insert out-identifier=\"salaboy\">\n";
cmd += " <org.drools.pipeline.camel.Person>\n";
cmd += " <name>salaboy</name>\n";
cmd += " </org.drools.pipeline.camel.Person>\n";
cmd += " </insert>\n";
cmd += " <fire-all-rules/>\n";
cmd += "</batch-execution>\n";
Object object = this.context.createProducerTemplate().requestBody("direct://client", cmd);
System.out.println( object );
}
}
The following urls show sample script examples for jaxb, xstream and json marshalling using:
17. Drools Camel Server
17.1. Introduction
The drools camel server (drools-camel-server) module is a war which you can deploy to execute KnowledgeBases remotely for any sort of client application. This is not limited to JVM application clients, but any technology that can use HTTP, through a REST interface. This version of the execution server supports stateless and stateful sessions in a native way.
17.2. Deployment
Drools Camel Server is a war file, which can be deployed in a application server (such as JBoss AS). As the service is stateless, it is possible to have have as many of these services deployed as you need to serve the client load. Deploy on JBoss AS 4.x / Tomcat 6.x works out-of-the-box, instead some external dependencies must be added and the configuration must be changed to be deployed in JBoss AS 5
17.3. Configuration
Inside the war file you will find a few XML configuration files.
-
beans.xml
-
Skeleton XML that imports knowledge-services.xml and camel-server.xml
-
-
camel-server.xml
-
Configures CXF endpoints with Camel Routes
-
Came Routes pipeline messages to various configured knowledge services
-
-
knowledge-services.xml
-
Various KIE bases and Sessions
-
-
camel-client.xml
-
Sample camel client showing how to send and receive a message
-
Used by "out of the box" test.jsp
-
17.3.1. REST/Camel Services configuration
The next step is configure the services that are going to be exposed through drools-server. You can modify this configuration in camel-server.xml file.
<?xml version="1.0" encoding="UTF-8"?>
<beans xmlns="http://www.springframework.org/schema/beans"
xmlns:xsi="http://www.w3.org/2001/XMLSchema-instance"
xmlns:cxf="http://camel.apache.org/schema/cxf"
xmlns:jaxrs="http://cxf.apache.org/jaxrs"
xsi:schemaLocation="
http://www.springframework.org/schema/beans http://www.springframework.org/schema/beans/spring-beans-2.5.xsd
http://camel.apache.org/schema/cxf http://camel.apache.org/schema/cxf/camel-cxf.xsd
http://cxf.apache.org/jaxrs http://cxf.apache.org/schemas/jaxrs.xsd
http://camel.apache.org/schema/spring http://camel.apache.org/schema/spring/camel-spring.xsd">
<import resource="classpath:META-INF/cxf/cxf.xml" />
<import resource="classpath:META-INF/cxf/cxf-extension-jaxrs-binding.xml"/>
<import resource="classpath:META-INF/cxf/cxf-servlet.xml" />
<!--
! If you are running on JBoss you will need to copy a camel-jboss.jar into the lib and set this ClassLoader configuration
! http://camel.apache.org/camel-jboss.html
! <bean id="jbossResolver" class="org.apache.camel.jboss.JBossPackageScanClassResolver"/>
-->
<!--
! Define the server end point.
! Copy and paste this element, changing id and the address, to expose services on different urls.
! Different Camel routes can handle different end point paths.
-->
<cxf:rsServer id="rsServer"
address="/rest"
serviceClass="org.kie.jax.rs.CommandExecutorImpl">
<cxf:providers>
<bean class="org.kie.jax.rs.CommandMessageBodyReader"/>
</cxf:providers>
</cxf:rsServer>
<cxf:cxfEndpoint id="soapServer"
address="/soap"
serviceName="ns:CommandExecutor"
endpointName="ns:CommandExecutorPort"
wsdlURL="soap.wsdl"
xmlns:ns="http://soap.jax.drools.org/" >
<cxf:properties>
<entry key="dataFormat" value="MESSAGE"/>
<entry key="defaultOperationName" value="execute"/>
</cxf:properties>
</cxf:cxfEndpoint>
<!-- Leave this, as it's needed to make Camel "drools" aware -->
<bean id="kiePolicy" class="org.kie.camel.component.KiePolicy" />
<camelContext id="camel" xmlns="http://camel.apache.org/schema/spring">
<!--
! Routes incoming messages from end point id="rsServer".
! Example route unmarshals the messages with xstream and executes against ksession1.
! Copy and paste this element, changing marshallers and the 'to' uri, to target different sessions, as needed.
!-->
<route>
<from uri="cxfrs://bean://rsServer"/>
<policy ref="kiePolicy">
<unmarshal ref="xstream" />
<to uri="kie:ksession1" />
<marshal ref="xstream" />
</policy>
</route>
<route>
<from uri="cxf://bean://soapServer"/>
<policy ref="kiePolicy">
<unmarshal ref="xstream" />
<to uri="kie:ksession1" />
<marshal ref="xstream" />
</policy>
</route>
</camelContext>
</beans>
17.3.1.1. RESTful service endpoint creation
In the next XML snippet code we are creating a RESTful (JAX-RS) endpoint bound to /kservice/rest address and using org.drools.jax.rs.CommandExecutorImpl as the service implementer. This class is only used to instantiate the service endpoint because all the internal implementation is managed by Camel, and you can see in the source file that the exposed execute service must be never called.
Also a JAX-RS Provider is provided to determine if the message transported can be processed in this service endpoint.
<cxf:rsServer id="rsServer"
address="/rest"
serviceClass="org.kie.jax.rs.CommandExecutorImpl">
<cxf:providers>
<bean class="org.kie.jax.rs.CommandMessageBodyReader"/>
</cxf:providers>
</cxf:rsServer>
Ideally this configuration doesn’t need to be modified, at least the Service Class and the JAX-RS Provider, but you can add more endpoints associated to different addresses to use them in other Camel Routes.
After all this initial configuration, you can start config your own Knowledge Services.
17.3.1.2. Camel Kie Policy & Context creation
KiePolicy is used to add Drools support in Camel, basically what it does is to add interceptors into the camel route to create Camel Processors on the fly and modify the internal navigation route. If you want to have SOAP support you need to create your custom Drools Policy, but it’s going to be added in the next release.
But you don’t need to know more internal details, only instantiate this bean:
<bean id="kiePolicy" class="org.kie.camel.component.KiePolicy" />
The next is create the camel route that will have the responsibility to execute the commands sent through JAX-RS. Basically we create a route definition associated with the JAX-RS definition as the data input, the camel policy to be used and inside the “execution route” or ProcessorDefinitions. As you can see, we set XStream as the marshaller/unmarshaller and the drools execution route definition
<camelContext id="camel" xmlns="http://camel.apache.org/schema/spring">
<route>
<from uri="cxfrs://bean://rsServer"/>
<policy ref="kiePolicy">
<unmarshal ref="xstream" />
<to uri="kie:ksession1" />
<marshal ref="xstream" />
</policy>
</route>
<route>
<from uri="cxf://bean://soapServer"/>
<policy ref="kiePolicy">
<unmarshal ref="xstream" />
<to uri="kie:ksession1" />
<marshal ref="xstream" />
</policy>
</route>
</camelContext>
The drools endpoint creation has the next arguments
<to uri="kie:{1}/{2}" />
-
Execution Node identifier that is registered in the CamelContext
-
KIE session identifier that was registered in the Execution Node with identifier {1}
Both parameters are configured in knowledge-services.xml file.
17.3.1.3. Knowledge Services configuration
The next step is create the KIE sessions that you are going to use.
<beans xmlns="http://www.springframework.org/schema/beans"
xmlns:xsi="http://www.w3.org/2001/XMLSchema-instance"
xmlns:kie="http://drools.org/schema/kie-spring"
xsi:schemaLocation="http://www.springframework.org/schema/beans http://www.springframework.org/schema/beans/spring-beans-3.0.xsd
http://drools.org/schema/kie-spring http://drools.org/schema/kie-spring.xsd">
<kie:kmodule id="drools-camel-server">
<kie:kbase name="kbase1" packages="org.drools.server">
<kie:ksession name="ksession1" type="stateless"/>
</kie:kbase>
</kie:kmodule>
<bean id="kiePostProcessor"
class="org.kie.spring.KModuleBeanFactoryPostProcessor"/>
</beans>
The execution-node is a context or registered kbases and ksessions, here kbase1 and ksession1 are planed in the node1 context. The kbase itself consists of two knowledge definitions, a DRL and an XSD. The Spring documentation contains a lot more information on configuring these knowledge services.
17.3.1.4. Test
With drools-server war unzipped you should be able to see a test.jsp and run it. This example just executes a simple "echo" type application. It sends a message to the rule server that pre-appends the word "echo" to the front and sends it back. By default the message is "Hello World", different messages can be passed using the url parameter msg - test.jsp?msg="My Custom Message".
Under the hood the jsp invokes the Test.java class, this then calls out to Camel which is where the meet happens. The camel-client.xml defines the client with just a few lines of XML:
<!-- Leave this, as it's needed to make Camel "drools" aware -->
<bean id="kiePolicy" class="org.kie.camel.component.KiePolicy" />
<camelContext id="camel" xmlns="http://camel.apache.org/schema/spring">
<route>
<from uri="direct://kservice/rest"/>
<policy ref="kiePolicy">
<to uri="cxfrs://http://localhost:8080/drools-server/kservice/rest"/>
</policy>
</route>
<route>
<from uri="direct://kservice/soap"/>
<policy ref="kiePolicy">
<to uri="cxfrs://http://localhost:8080/drools-server/kservice/soap"/>
</policy>
</route>
</camelContext>
"direct://kservice" is just a named hook, allowing Java to grab a reference and push data into it.
In this example the data is already in XML, so we don’t need to add any DataFormat
s to do the marshalling.
The KiePolicy adds some smarts to the route and you’ll see it used on the server side too.
If JAXB or XStream were used, it would inject custom paths and converters, it can also set the ClassLoader too on the server side, on the client side it automatically unwraps the Response object.
The rule itself can be found here: test.drl. Notice the type Message is declared part of the DRL and is thus not present on the Classpath.
declare Message
text : String
end
rule "echo" dialect "mvel"
when
$m : Message();
then
$m.text = "echo:" + $m.text;
end
Business Central
Business Central in Drools is built with the UberFire framework and uses the Guvnor plugin. Drools provides an additional rich set of plugins for rule-authoring metaphors.
18. Business Central (General)
18.1. Installation
18.1.1. War installation
Use the war
from the Business Central distribution zip that corresponds to your application server.
The differences between these war
files are mainly superficial.
For example, some JARs might be excluded if the application server already supplies them.
-
eap7
: tailored for Red Hat JBoss Enterprise Application Platform 7 -
wildfly14
: tailored for Wildfly 14
18.1.2. Business Central data
Business Central stores its data, by default in the directory $WORKING_DIRECTORY/.niogit
, for example wildfly-14.0.1.Final/bin/.niogit
, but it can be overridden with the system property-Dorg.uberfire.nio.git.dir
.
In production, make sure to back up the Business Central data directory. |
18.1.3. System properties
Here’s a list of all system properties:
-
org.kie.workbench.profile
: Selects the Business Central profile. Possible values areFULL
orPLANNER_AND_RULES
. A prefixFULL_
will set the profile and hide the profile preferences from the admin preferences. Default:FULL
. -
kie.maven.offline.force
: Forces Maven to behave as offline. If true, disable online dependency resolution. Default: false.Use this property for Business Central only. If you share a runtime environment with any other component, isolate the configuration and apply it only to Business Central.
-
org.appformer.m2repo.url
: Location of the default Maven repository Business Central uses when looking for dependencies. Usually this points to the Maven repository inside the Workbench for examplehttp://localhost:8080/business-central/maven2
. Please set this before starting up the Workbench. Default: File path to the inner m2 repository. -
org.uberfire.nio.git.dir
: Location of the directory.niogit
. Default: working directory -
org.uberfire.nio.git.dirname
: Name of the git directory. Default:.niogit
-
org.uberfire.nio.git.proxy.ssh.over.http
: Defines that SSH should use an HTTP Proxy. Default:false
-
http.proxyHost
: Defines the host name of the HTTP Proxy. Default:null
-
http.proxyPort
: Defines the host port (integer value) of the HTTP Proxy. Default:null
-
org.uberfire.nio.git.proxy.ssh.over.https
: Defines that SSH should use an HTTPS Proxy. Default:false
-
https.proxyHost
: Defines the host name of the HTTPS Proxy. Default:null
-
https.proxyPort
: Defines the host port (integer value) of the HTTPS Proxy. Default:null
-
org.uberfire.nio.git.http.enabled
: Enables or disables the HTTP daemon. Default:true
-
org.uberfire.nio.git.http.host
: If the HTTP daemon is enabled, it uses this property as the host identifier. This is an informative property that is used to display how to access the Git repository over HTTP. The HTTP still relies on the servlet container. Default:localhost
-
org.uberfire.nio.git.http.hostname
: If the HTTP daemon is enabled, it uses this property as the host name identifier. This is an informative property that is used to display how to access the Git repository over HTTP. The HTTP still relies on the servlet container. Default:localhost
-
org.uberfire.nio.git.http.port
: If the HTTP daemon is enabled, it uses this property as the port number. This is an informative property that is used to display how to access the Git repository over HTTP. The HTTP still relies on the servlet container. Default:8080
-
org.uberfire.nio.git.https.enabled
: Enables or disables the HTTPS daemon. Default:false
-
org.uberfire.nio.git.https.host
: If the HTTPS daemon is enabled, it uses this property as the host identifier. This is an informative property that is used to display how to access the Git repository over HTTPS. The HTTPS still relies on the servlet container. Default:localhost
-
org.uberfire.nio.git.https.hostname
: If the HTTPS daemon is enabled, it uses this property as the host name identifier. This is an informative property that is used to display how to access the Git repository over HTTPS. The HTTPS still relies on the servlet container. Default:localhost
-
org.uberfire.nio.git.https.port
: If the HTTPS daemon is enabled, it uses this property as the port number. This is an informative property that is used to display how to access the Git repository over HTTPS. The HTTPS still relies on the servlet container. Default:8080
-
org.uberfire.nio.git.daemon.enabled
: Enables/disables git daemon. Default:true
-
org.uberfire.nio.git.daemon.host
: If git daemon enabled, uses this property as local host identifier. Default:localhost
-
org.uberfire.nio.git.daemon.hostname
: If the git daemon is enabled, uses this property as the local host name identifier. Default:localhost
-
org.uberfire.nio.git.daemon.port
: If git daemon enabled, uses this property as port number. Default:9418
-
org.uberfire.nio.git.http.sslVerify
: Enables or disables SSL certificate checking for Git repositories. Default:true
If the default or assigned port is already in use, a new port is automatically selected. Ensure that the ports are available and check the log for more information.
-
org.uberfire.nio.git.ssh.enabled
: Enables/disables ssh daemon. Default:true
-
org.uberfire.nio.git.ssh.host
: If ssh daemon enabled, uses this property as local host identifier. Default:localhost
-
org.uberfire.nio.git.ssh.hostname
: If the SSH daemon is enabled, uses this property as local host name identifier. Default:localhost
-
org.uberfire.nio.git.ssh.port
: If ssh daemon enabled, uses this property as port number. Default:8001
-
org.uberfire.nio.git.ssh.ciphers
: A comma-separated string of ciphers. The available ciphers areaes128-ctr
,aes192-ctr
,aes256-ctr
,arcfour128
,arcfour256
,aes192-cbc
,aes256-cbc
. If the property is not used, all available ciphers are loaded. -
org.uberfire.nio.git.ssh.macs
: A comma-separated string of message authentication codes (MACs). The available MACs arehmac-md5
,hmac-md5-96
,hmac-sha1
,hmac-sha1-96
,hmac-sha2-256
,hmac-sha2-512
. If the property is not used, all available MACs are loaded.If the default or assigned port is already in use, a new port is automatically selected. Ensure that the ports are available and check the log for more information.
-
org.uberfire.nio.git.ssh.cert.dir
: Location of the directory.security
where local certificates will be stored. Default: working directory -
org.uberfire.nio.git.ssh.passphrase
: Passphrase to access your Operating Systems public keystore when cloninggit
repositories withscp
style URLs; e.g.git@github.com:user/repository.git
. -
org.uberfire.nio.git.ssh.algorithm
: Algorithm used by SSH. Default:DSA
If you plan to use RSA or any algorithm other than DSA, make sure you setup properly your Application Server to use Bouncy Castle JCE library.
-
appformer.ssh.keystore
: Defines the custom SSH keystore to be used with Business Central by specifying a class name. If the property is not available the default SSH keystore is used. -
appformer.ssh.keys.storage.folder
: When using the default SSH keystore, this parameter defines the storage folder for the user’s SSH public keys. If the property is not available the keys are stored in the Workbench.security
folder. -
org.uberfire.metadata.index.dir
: Place where Lucene.index
folder will be stored. Default: working directory -
org.uberfire.ldap.regex.role_mapper
: Regex pattern used to map LDAP principal names to application role name. Note that the variablerole
must be part of the pattern as it is substited by the application role name when matching a principal value to role name. Default: Not used. -
org.uberfire.sys.repo.monitor.disabled
: Disable configuration monitor (do not disable unless you know what you’re doing). Default:false
-
org.uberfire.secure.key
: Secret password used by password encryption. Default:org.uberfire.admin
-
org.uberfire.secure.alg
: Crypto algorithm used by password encryption. Default:PBEWithMD5AndDES
-
org.uberfire.domain
: security-domain name used by uberfire. Default:ApplicationRealm
-
appformer.experimental.features
: enables the Experimental Features Framework -
org.guvnor.m2repo.dir
: Place where Maven repository folder will be stored. Default: working-directory/repositories/kie -
org.guvnor.project.gav.check.disabled
: Disable GAV checks. Default:false
-
org.kie.demo
: Enables external clone of a demo application from GitHub. -
org.kie.build.disable-project-explorer
: Disable automatic build of selected Project in Project Explorer. Default:false
-
org.kie.verification.disable-dtable-realtime-verification
: Disables the realtime validation and verification of decision tables. Default:false
-
org.kie.workbench.controller
: URL for connecting with a Drools controller, for example:ws://localhost:8080/kie-server-controller/websocket/controller
. -
org.uberfire.gzip.enable
: Enables or disables Gzip compression on GzipFilter. Default:true
Only Web Socket protocol is supported for connecting with a headless Drools controller. When specifying this property, Business Central will automatically disable all the features related to running the embedded Drools controller.
-
org.kie.workbench.controller.user
: User name for connecting with a Drools controller. Default:kieserver
-
org.kie.workbench.controller.pwd
: Password for connecting with a Drools controller. Default:kieserver1!
-
org.kie.workbench.controller.token
: Token string for connecting with a Drools controller.
Please refer to Using token based authentication for more details about how to use token based authentication.
-
kie.keystore.keyStoreURL
: URL to a keystore which should be used for connecting with a headless Drools controller. -
kie.keystore.keyStorePwd
: Password to a keystore. -
kie.keystore.key.ctrl.alias
: Alias of the key where password is stored. -
kie.keystore.key.ctrl.pwd
: Password of an alias with stored password.
Please refer to Securing password using key store for more details about how to use a key store for securing your passwords.
-
org.jbpm.wb.forms.renderer.ext
: Switch form rendering between Business Central and Kie Server rendered forms. By default, form rendering is done by Business Central. Default:false
. -
org.jbpm.wb.forms.renderer.name
: Allows to switch between Business Central or Kie Server rendered forms. Kie server includes two renderers,bootstrap
andpatternfly
in addition to the default renderer,workbench
. Default:workbench
.
To change one of these system properties in a WildFly or JBoss EAP cluster:
-
Edit the file
$JBOSS_HOME/domain/configuration/host.xml
. -
Locate the XML elements
server
that belong to themain-server-group
and add a system property, for example:<system-properties> <property name="org.uberfire.nio.git.dir" value="..." boot-time="false"/> ... </system-properties>
18.1.4. Trouble shooting
18.1.4.1. Loading.. does not disappear and Business Central fails to show
There have been reports that Firewalls in between the server and the browser can interfere with Server Sent Events (SSE) used by Business Central.
The issue results in the "Loading…" spinner remaining visible and Business Central failing to materialize.
The workaround is to disable the Business Central’s use of Server Sent Events by adding file /WEB-INF/classes/ErraiService.properties
to the exploded WAR containing the value errai.bus.enable_sse_support=false
.
Re-package the WAR and re-deploy.
Some Users have also reported disabling Server Sent Events does not resolve the issue. The solution found to work is to configure the JVM to use a different Entropy Gathering Device on Linux for SecureRandom
. This can be configured by setting System Property java.security.egd
to file:/dev/./urandom
. See this Stack Overflow post for details.
Please note however this affects the JVM’s random number generation and may present other challenges where strong cryptography is required. Configure with caution.
18.1.4.2. Not able to clone Business Central Git repository using ssh protocol.
Git clients using ssh to interact with the Git server that is bundled with Business Central are authenticated and authorized to perform git commands by the security API that is part of the Uberfire backend server. When using an LDAP security realm, some git clients were not being authorized as expected. This was due to the fact that for non-web clients such as Git via ssh, the principal (i.e., user or group) name assigned to a user by the application server’s user registry is the more complex DN associated to that principal by LDAP. The logic of the Uberfire backend server looked for on exact match of roles allowed with the principal name returned and therefore failed.
It is now possible to control the role-principal matching via the system property
org.uberfire.ldap.regex.role_mapper
which takes as its value a Regex pattern to be applied when matching LDAP principal to role names. The pattern must contain the literal word variable 'role'. During authorization the variable is replaced by each of the allow application roles. If the pattern is matched the role is added to the user.
For instance, if the DN for the admin group in LDAP is
DN: cn=admin,ou=groups,dc=example,dc=com
and its intended role is admin, then setting org.uberfire.ldap.regex.role_mapper
with value
cn[\\ ]*=[\\ ]*role
will find a match on role 'admin'.
18.2. Quick Start
These steps help you get started with minimum of effort.
They should not be a substitute for reading the documentation in full.
18.2.1. Importing examples
If Business Central is empty you are shown an empty Space page. Clicking "Try Samples" button below will show the examples that are available.
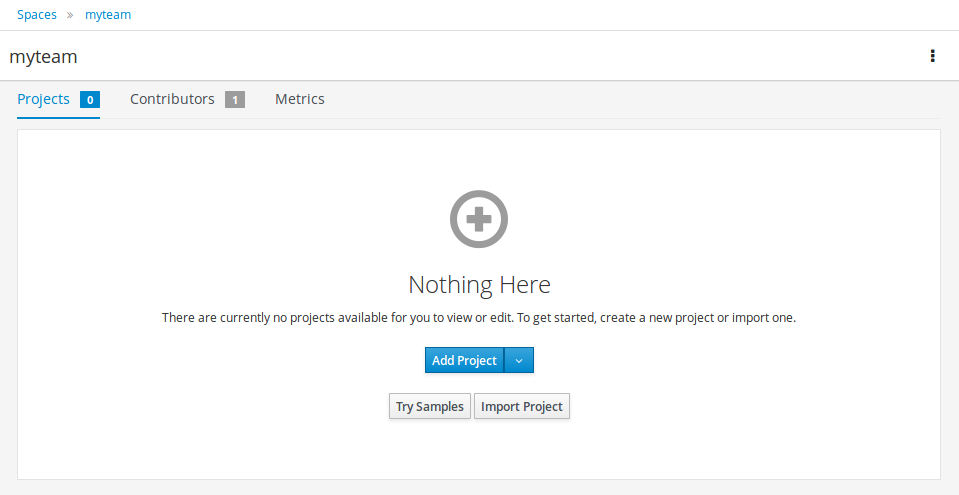
Once "Try Samples" page opens, you can select one or more examples and click "Ok".
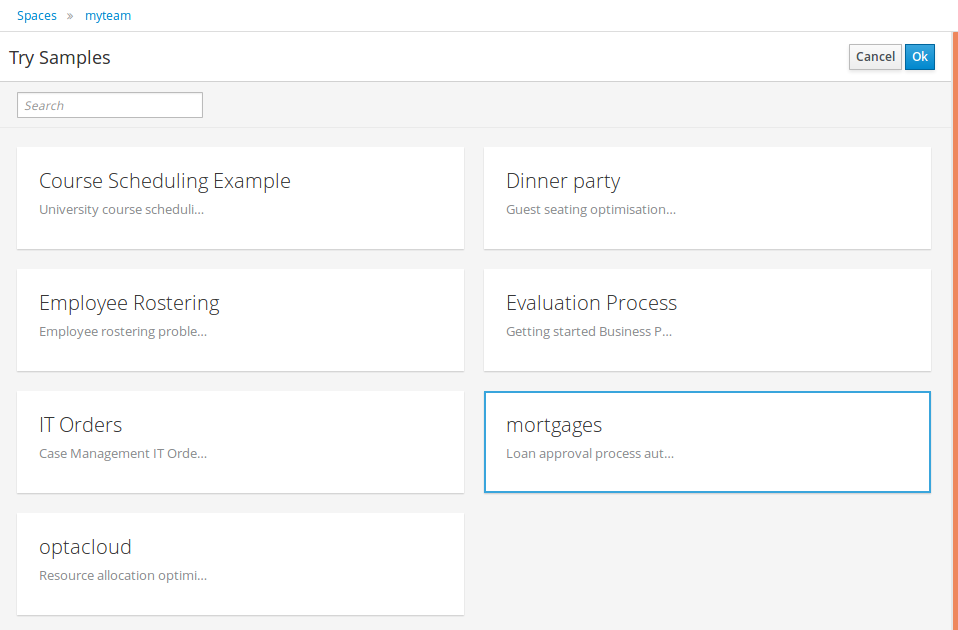
If Business Central already contains Projects the examples can be imported with the "Try Samples" button found from the menu.
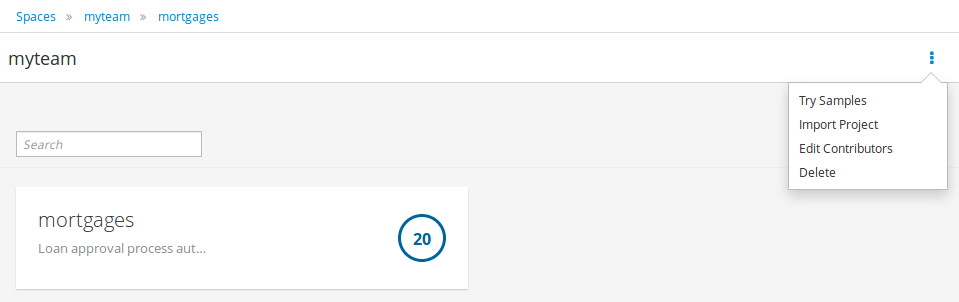
18.2.2. Add Project
Alternatively, to importing an example, a new empty project can be created from the Space page with "Add Project".
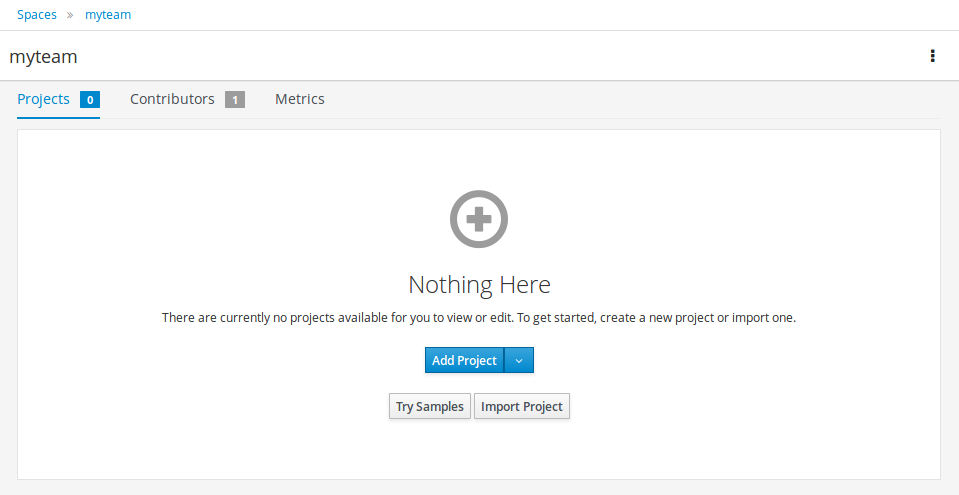
Give the Project a name and optional description.
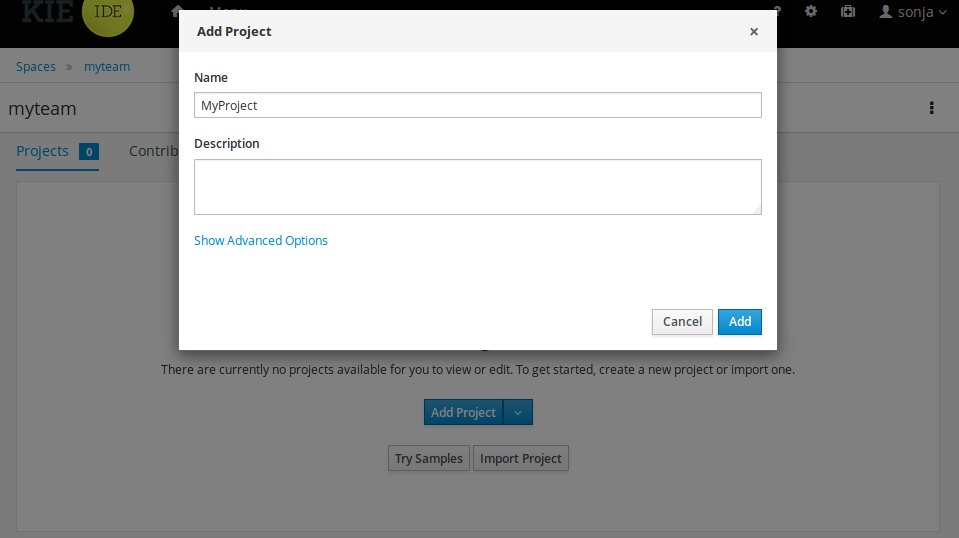
18.2.3. Define Data Model
After a Project has been created you need to define Types to be used by your rules.
Select "Data Object" from the "Add Asset" menu.
You can also use types contained in existing JARs. Please consult the full documentation for details. |
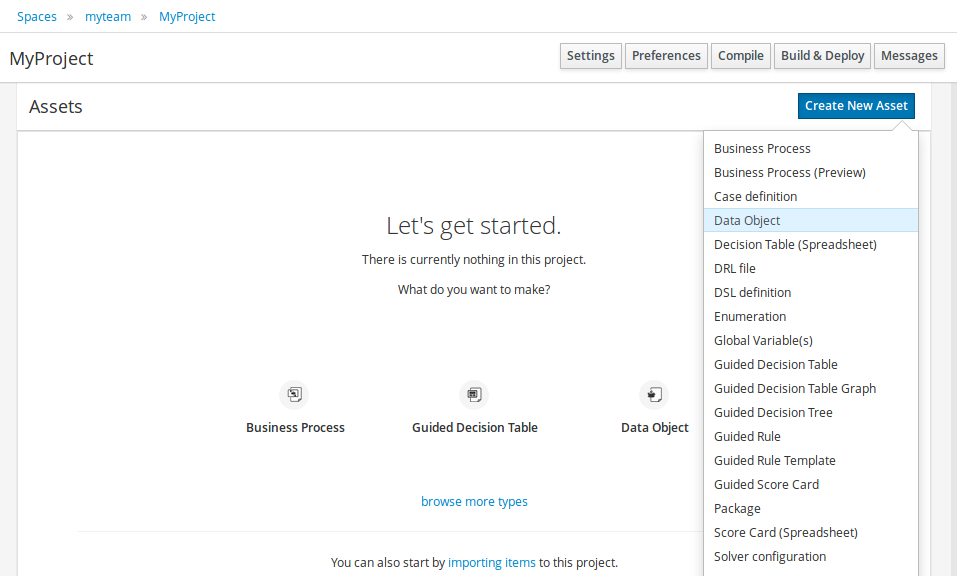
Set the name and select a package for the new type.
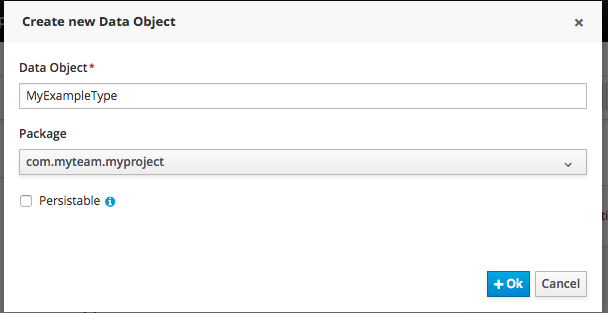
Click "+ add field" button and set a field name and type and click on "Create" to create a field for the type.
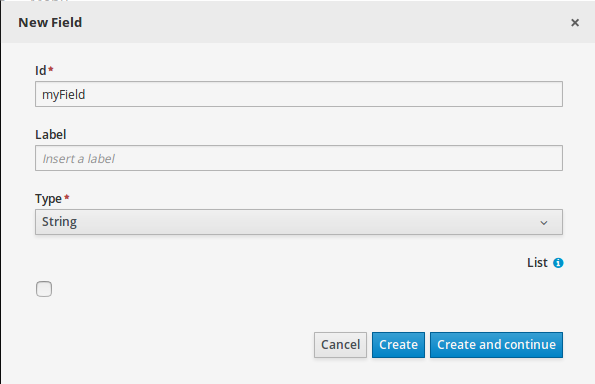
Click "Save" to update the model.
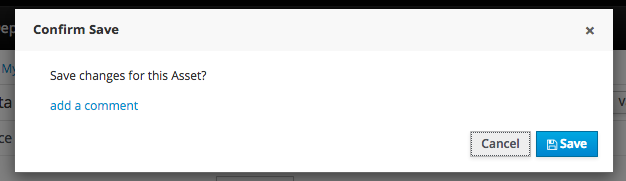
18.2.4. Define Rule
Select "DRL file" (for example) from the "Add Asset" menu.
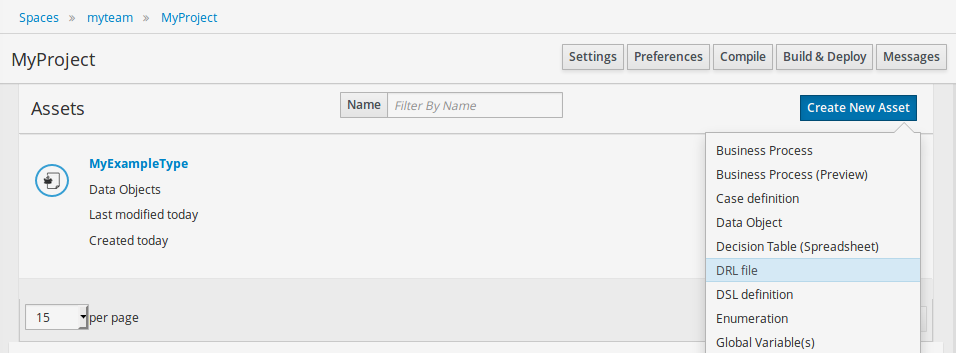
Enter a file name for the new rule.
Make sure you select the same package as the rule had. It is possible to have rules and data models in different packages, but let’s keep things simple for demo purposes. |
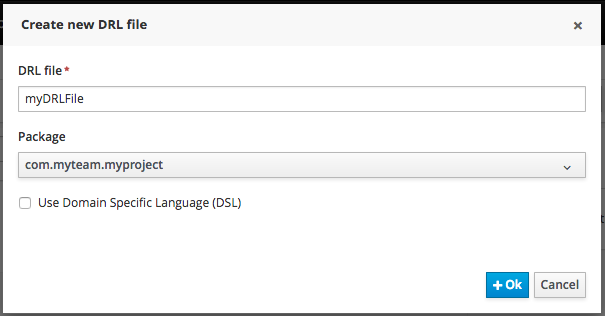
Enter a definition for the rule.
The definition process differs from asset type to asset type.
The full documentation has details about the different editors.
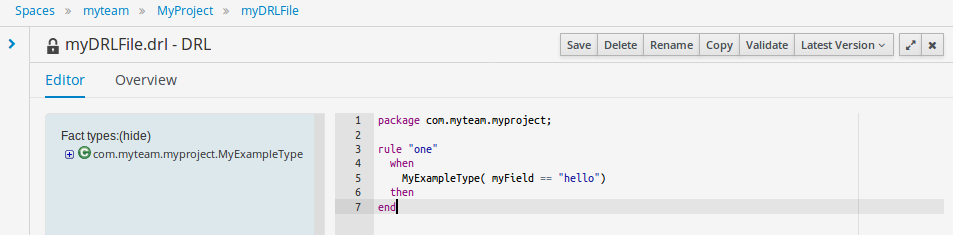
Once the rule has been defined it will need to be saved in the same way we saved the model.
18.2.5. Build and Deploy
Once rules have been defined within a project; the project can be built and deployed to the Business Central’s Maven Artifact Repository.
To build a project select the "Build & Deploy" from the Project Authoring.
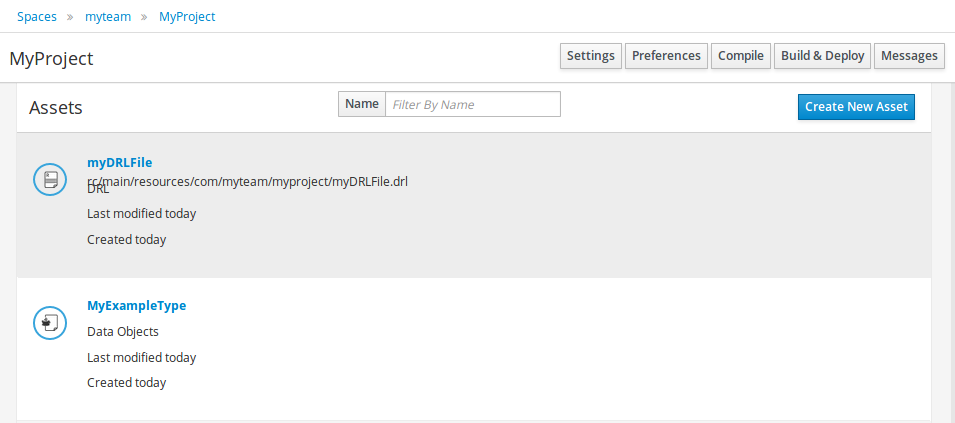
Click "Build & Deploy" to build the project and deploy it to the Business Central’s Maven Artifact Repository.
When you select Build & Deploy Business Central will deploy to any repositories defined in the Dependency Management section of the pom in your Business Central project. You can edit the pom.xml file associated with your Business Central project under the Repository View of the project explorer. Details on dependency management in maven can be found here : http://maven.apache.org/guides/introduction/introduction-to-dependency-mechanism.html
If there are errors during the build process they will be reported in the "Messages" panel.
Now the project has been built and deployed; it can be referenced from your own projects as any other Maven Artifact.
The full documentation contains details about integrating projects with your own applications.
18.3. Configuration
18.3.1. Basic user management
Business Central authenticates its users against the application server’s authentication and authorization (JAAS).
On JBoss EAP and WildFly, add a user with the script $JBOSS_HOME/bin/add-user.sh
(or .bat
):
$ ./add-user.sh
// Type: Application User
// Realm: empty (defaults to ApplicationRealm)
// Role: admin
There is no need to restart the application server.
18.3.2. Roles
Business Central uses the following roles:
-
admin
-
analyst
-
developer
-
manager
-
user
18.3.2.1. Admin
Administrates the BPMS system.
-
Manages users
-
Manages VFS Repositories
-
Has full access to make any changes necessary
18.3.2.2. Developer
Developer can do almost everything admin can do, except clone repositories.
-
Manages rules, models, process flows, forms and dashboards
-
Manages the asset repository
-
Can create, build and deploy projects
-
Can use the JBDS connection to view processes
18.3.2.3. Analyst
Analyst is a weaker version of developer and does not have access to the asset repository or the ability to deploy projects.
18.3.2.4. Business user
Daily user of the system to take actions on business tasks that are required for the processes to continue forward. Works primarily with the task lists.
-
Does process management
-
Handles tasks and dashboards
18.3.2.5. Manager/Viewer-only User
Viewer of the system that is interested in statistics around the business processes and their performance, business indicators, and other reporting of the system and people who interact with the system.
-
Only has access to dashboards
18.4. Introduction
18.4.1. Log in and log out
Create a user with the role admin
and log in with those credentials.
After successfully logging in, the account username is displayed at the top right. Click on it to review the roles of the current account.
18.4.2. Home screen
After logging in, the home screen shows. The actual content of the home screen depends on the Business Central variant (Drools, jBPM, …).
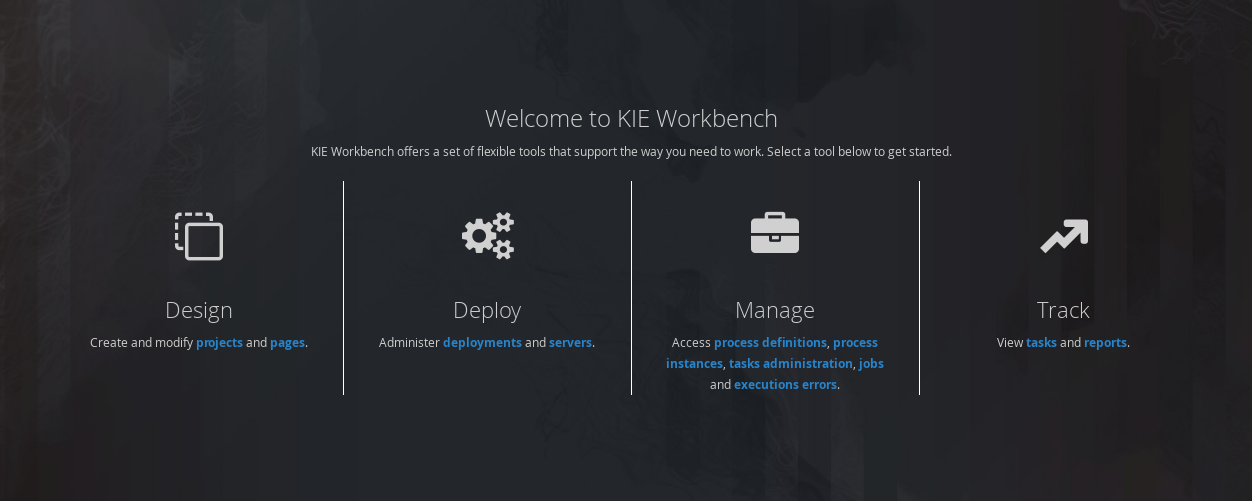
18.4.3. Business Central overview
Business Central is structured with Spaces and Projects:
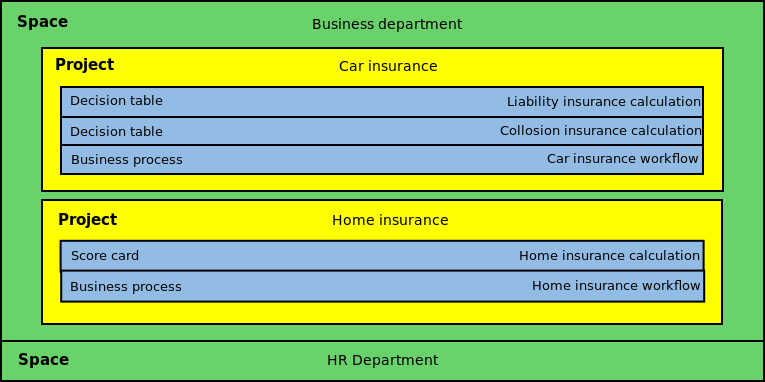
18.4.3.1. Space
Spaces are useful to model departments and divisions.
A Space can hold multiple Projects.
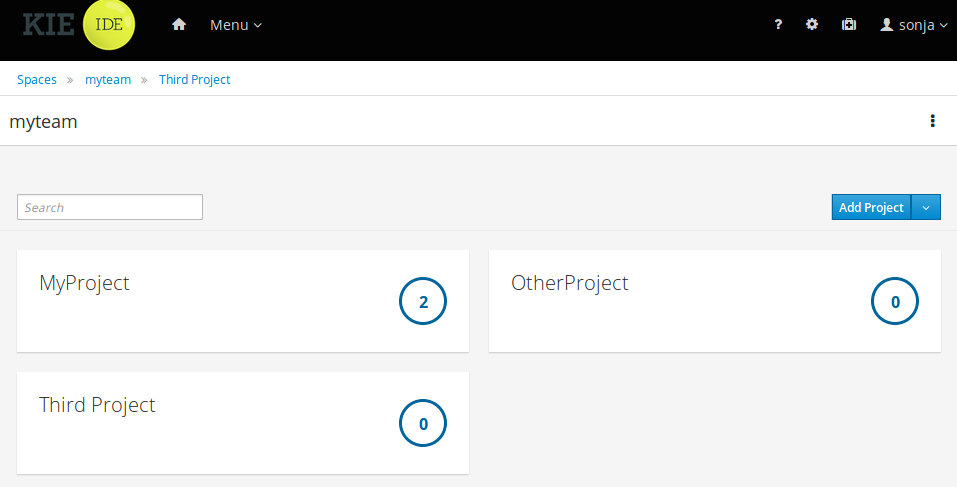
18.4.3.2. Project
Projects are the place where assets are stored and each project belongs to a single Space.
Projects are in fact a Virtual File System based storage, that by default uses GIT as backend. Such setup allows Business Central to work with multiple backends and, in the same time, take full advantage of backend specifics features like in GIT case versioning, branching and even external access.
A new Project can be created from scratch or cloned from an existing repository.
One of the biggest advantage of using GIT as backend is the ability to clone a repository from external and use your preferred tools to edit and build your assets.
Never clone your repositories directly from .niogit directory. |
18.4.4. Business Central user interface concepts
Business Central consists of different logical entities:
-
Part
A Part is a screen or editor with which the user can interact to perform operations.
Example Parts are "Project Explorer", "Project Editor", "Guided Rule Editor" etc.
-
Page
A perspective is a logical grouping of related Panels and Parts. A perspective is usually named as page, since it is a term far more familiar to end users whereas a perspective is more developer oriented. Notice however, Business Central supports both developer created pages and those created by end users from the page builder (aka Content Management) tooling but, generally speaking, page is used to refer both.
The user can switch between pages by clicking on one of the top-level menu items; such as "Home", "Authoring", "Deploy" etc.
18.5. Changing the layout
18.5.1. Resizing
Move the mouse pointer over the panel splitter (a grey horizontal or vertical line in between panels).
The cursor will by changing indicate it is positioned correctly over the splitter. Press and hold the left mouse button and drag the splitter to the required position; then release the left mouse button.
18.6. Authoring (General)
18.6.1. Artifact Repository
Projects often need external artifacts in their classpath in order to build, for example a domain model JARs. The artifact repository holds those artifacts.
The Artifact Repository is a full blown Maven repository. It follows the semantics of a Maven remote repository: all snapshots are timestamped. But it is often stored on the local hard drive.
By default the artifact repository is stored under $WORKING_DIRECTORY/repositories/kie
, but it can be overridden with the system property-Dorg.guvnor.m2repo.dir
.
There is only 1 Maven repository per installation.
The Artifact Repository screen shows a list of the artifacts in the Maven repository:
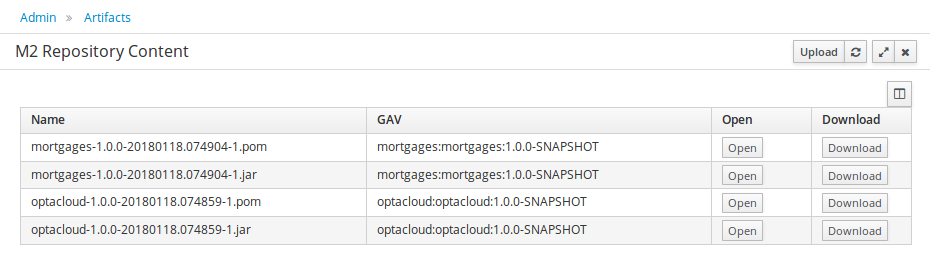
To add a new artifact to that Maven repository, either:
-
Use the upload button and select a JAR. If the JAR contains a POM file under
META-INF/maven
(which every JAR build by Maven has), no further information is needed. Otherwise, a groupId, artifactId and version need be given too.
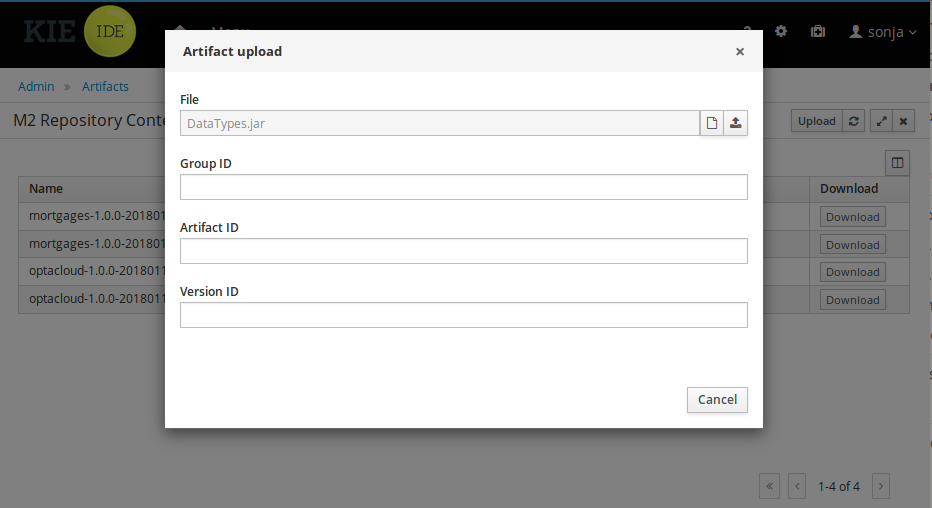
-
Using Maven,
mvn deploy
to that Maven repository. Refresh the list to make it show up.
This remote Maven repository is relatively simple. It does not support proxying, mirroring, … like Nexus or Archiva. |
18.6.2. Asset Editor
The Asset Editor is the principle component of the Business Central user interface. It consists of two main views Editor and Overview.
-
The views
Figure 151. The Asset Editor - Editor tab-
A : The editing area - exactly what form the editor takes depends on the Asset type. An asset can only be edited by one user at a time to avoid conflicts. When a user begins to edit an asset, a lock will automatically be acquired. This is indicated by a lock symbol appearing on the asset title bar as well as in the project explorer view (see Project Explorer for details). If a user starts editing an already locked asset a pop-up notification will appear to inform the user that the asset can’t currently be edited, as it is being worked on by another user. Changes will be prevented until the editing user saves or closes the asset, or logs out of Business Central. Session timeouts will also cause locks to be released. Every user further has the option to force a lock release, if required (see the Metadata section below).
-
B : This menu bar contains various actions for the Asset; such as Save, Rename, Copy etc. Note that saving, renaming and deleting are deactivated if the asset is locked by a different user.
-
C : Different views for asset content or asset information.
-
Editor shows the main editor for the asset
-
Overview contains the metadata and conversation views for this editor. Explained in more detail below.
-
Source shows the asset in plain DRL. Note: This tab is only visible if the asset content can be generated into DRL.
-
Data Objects contains the model available for authoring. By default only Data Objects that reside within the same package as the asset are available for authoring. Data Objects outside of this package can be imported to become available for authoring the asset.
-
Figure 152. The Asset Editor - Data Objects tab -
-
Overview
-
A : General information about the asset and the asset’s description.
"Type:" The format name of the type of Asset.
"Description:" Description for the asset.
"Used in projects:" Names the projects where this rule is used.
"Last Modified:" Who made the last change and when.
"Created on:" Who created the asset and when.
-
B : Version history for the asset. Selecting a version loads the selected version into this editor.
-
C : Meta data (from the "Dublin Core" standard)
-
D : Comments regarding the development of the Asset can be recorded here.
-
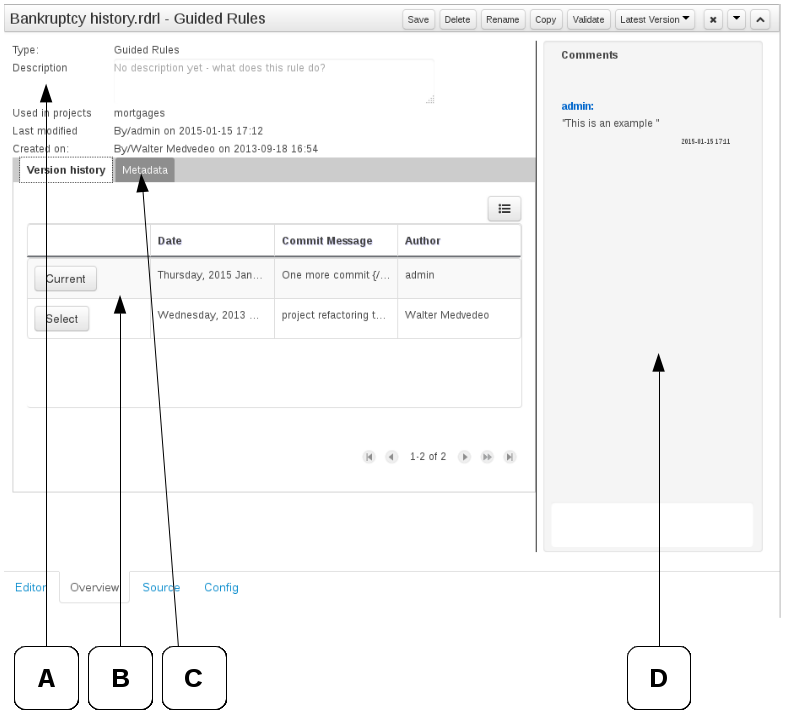
-
Metadata
-
A : Meta data:-
"Tags:" A tagging system for grouping the assets.
"Note:" A comment made when the Asset was last updated (i.e. why a change was made)
"URI:" URI to the asset inside the Git repository.
"Subject/Type/External link/Source" : Other miscellaneous meta data for the Asset.
"Lock status" : Shows the lock status of the asset and, if locked, allows to force unlocking the asset.
-
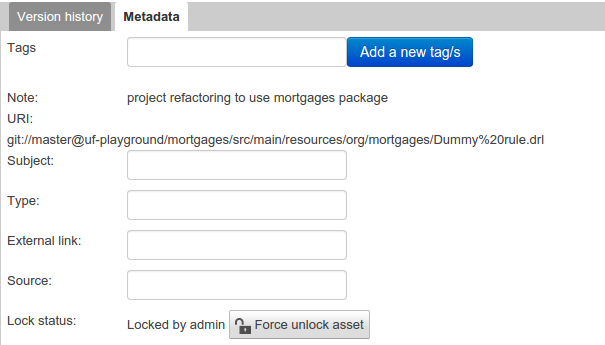
-
Locking
Business Central supports pessimistic locking of assets. When one User starts editing an asset it is locked to change by other Users. The lock is held until a period of inactivity lapses, the Editor is closed or the application stopped and restarted. Locks can also be forcibly removed on the MetaData section of the Overview tab.
A "padlock" icon is shown in the Editor’s title bar and beside the asset in the Project Explorer when an asset is locked.
Figure 155. The Asset Editor - Locked assets cannot be edited by other users
18.6.3. Tags Editor
Tags allow assets to be labelled with any number of tags that you define. These tags can be used to filter assets on the Project Explorer enabling "Tag filtering".
18.6.3.1. Creating Tags
To create tags you simply have to write them on the Tags input and press the "Add new Tag/s" button. The Tag Editor allows creating tags one by one or writing more than one separated with a white space.
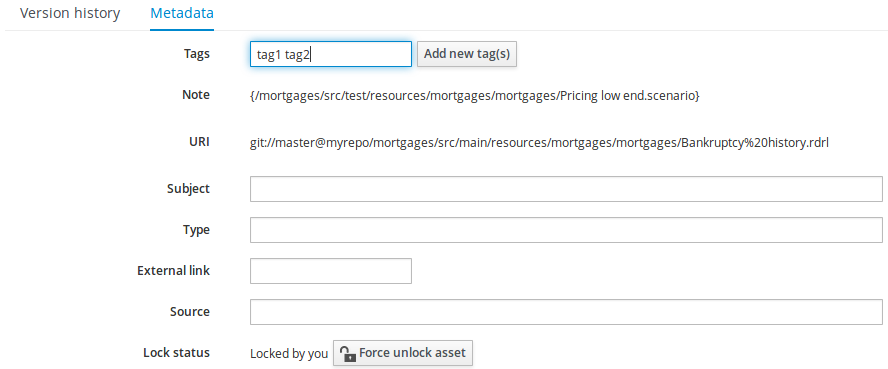
Once you created new Tags they will appear over the Editor allowing you to remove them by pressing on them if you want.
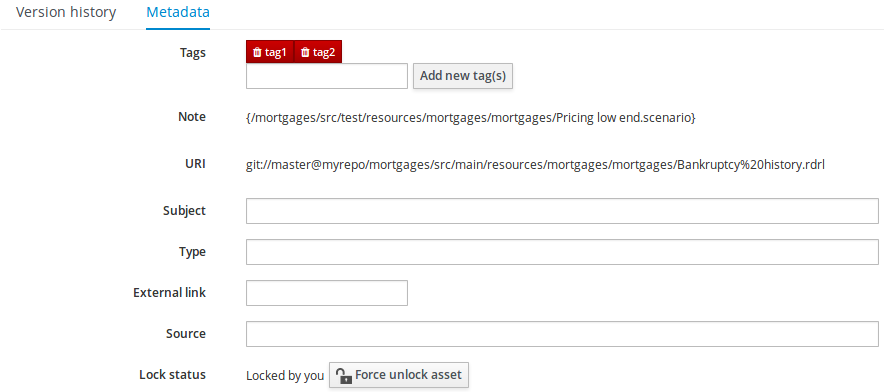
18.6.4. Project Explorer
The Project Explorer provides the ability to browse files inside the current Project. The Project Explorer can be accessed from the left side when an Asset Editor is open.
18.6.4.1. Initial view
If a file is currently being edited by another user, a lock symbol will be displayed in front of the file name. The symbol is blue in case the lock is owned by the currently authenticated user, otherwise black. Moving the mouse pointer over the lock symbol will display a tooltip providing the name of the user who is currently editing the file (and therefore owning the lock). To learn more about locking see Asset Editor for details.
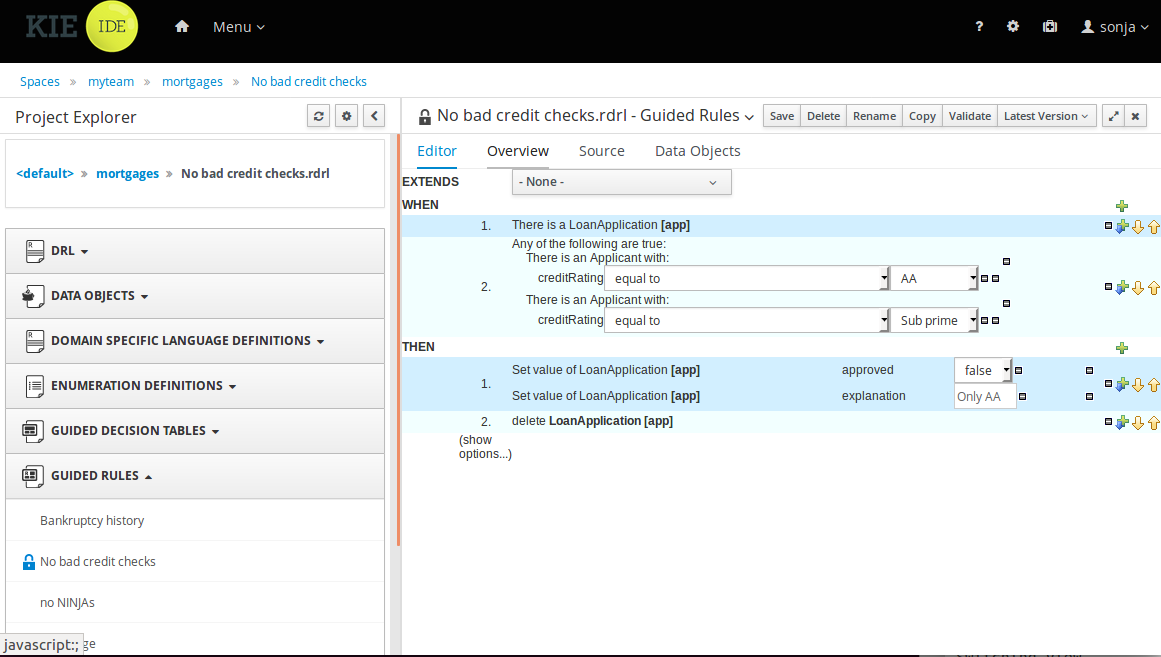
18.6.4.2. Different views
Project Explorer supports multiple views.
-
Project View
A simplified view of the underlying project structure. Certain system files are hidden from view.
-
Repository View
A complete view of the underlying project structure including all files; either user-defined or system generated.
Views can be selected by clicking on the icon within the Project Explorer, as shown below.
Both Project and Repository Views can be further refined by selecting either "Show as Folders" or "Show as Links".
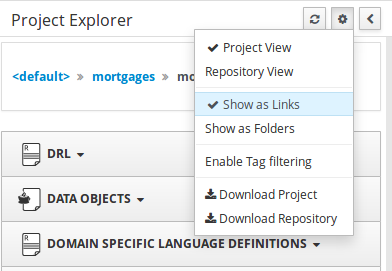
18.6.4.3. Download Project or Repository
Download Download and Download Repository make it possible to download the project or repository as a zip file.
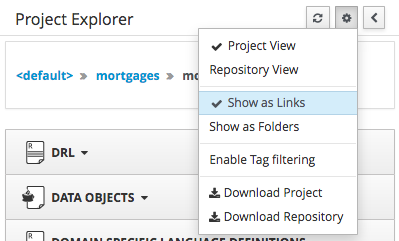
18.6.4.4. Filtering by Tag
To make easy view the elements on packages that contain a lot of assets, is possible to enabling the Tag filter, whichs allows you to filter the assets by their tags.
To see how to add tags to an asset look at: Tags Editor
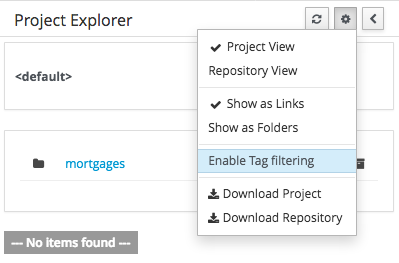
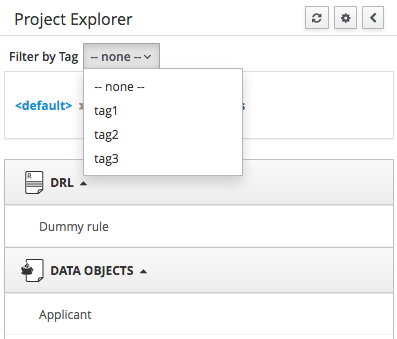
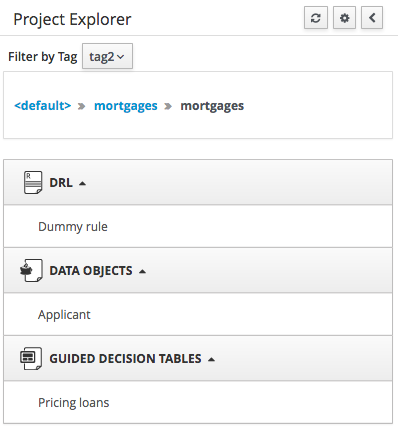
18.6.4.5. Copy, Rename, Delete and Download Actions
Copy, rename and delete actions are available on Links mode, for packages in of Project View and for files and directories in Repository View. Download action is available for directories. Download option downloads the selected the selected directory as a zip file.
-
A : Copy
-
B : Rename
-
C : Delete
-
D : Download
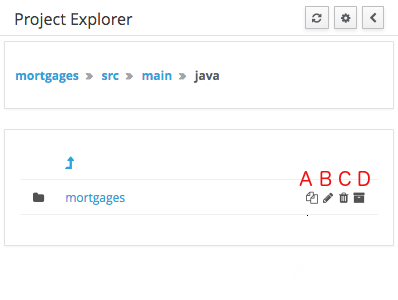
Business Central roadmap includes a refactoring and an impact analyses tools, but currenctly doesn’t have it. Until both tools are provided make sure that your changes (copy/rename/delete) on packages, files or directories doesn’t have a major impact on your project. In cases that your change had an unexcepcted impact, Business Central enables you to restore your repository using the Repository editor. |
Files locked by other users as well as directories that contain such files cannot be renamed or deleted until the corresponding locks are released. If that is the case the rename and delete symbols will be deactivated. To learn more about locking see Asset Editor for details. ![]() |
18.6.5. Project Editor
The Project Editor screen can be accessed from Project Explorer. Project Editor shows the settings for the currently active project.
Unlike most of the Business Central editors, project editor edits more than one file. Showing everything that is needed for configuring the KIE project in one place.
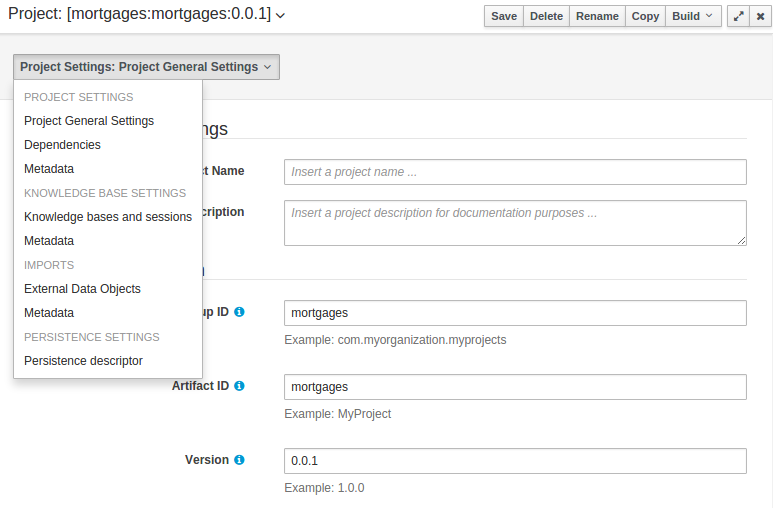
18.6.5.1. Build & Deploy
Build & Depoy builds the current project and deploys the KJAR into the Business Central internal Maven repository.
18.6.5.2. Project Settings
Project Settings edits the pom.xml file used by Maven.
Project General Settings
General settings provide tools for project name and GAV-data (Group, Artifact, Version). GAV values are used as identifiers to differentiate projects and versions of the same project.
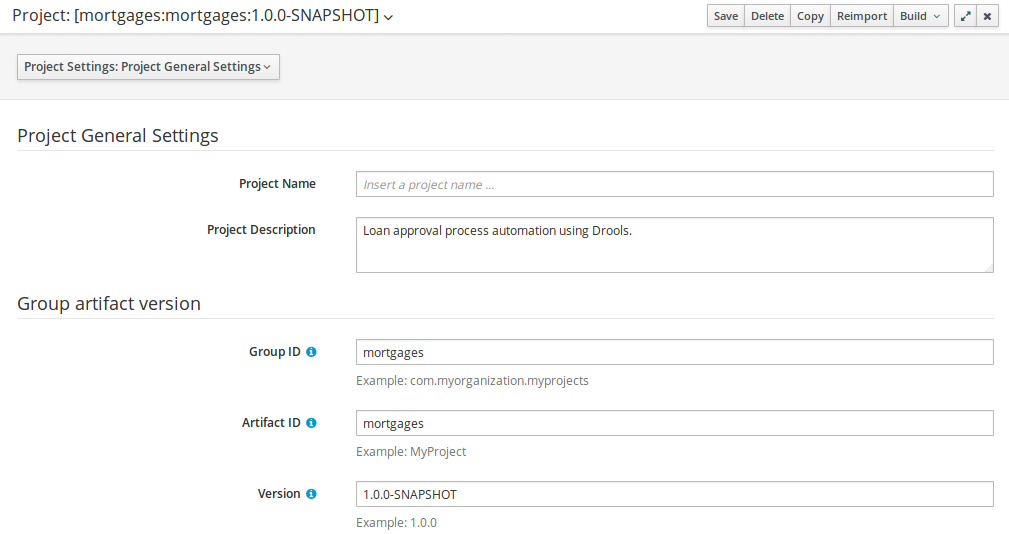
Dependencies
The project may have any number of either internal or external dependencies. Dependency is a project that has been built and deployed to a Maven repository. Internal dependencies are projects built and deployed in the same Business Central as the project. External dependencies are retrieved from repositories outside of the current Business Central. Each dependency uses the GAV-values to specify the project name and version that is used by the project.
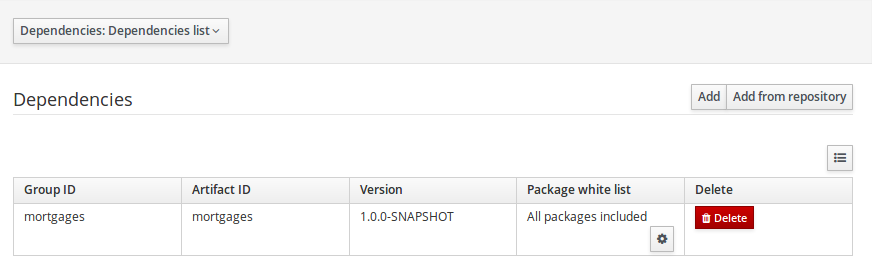
Classes and declared types in white listed packages show up as Data Objects that can be imported in assets. The full list is stored in package-name-white-list file that is stored in each project root.
Package white list has three modes:
-
All packages included: Every package defined in this jar is white listed.
-
Packages not included: None of the packages listed in this jar are white listed.
-
Some packages included: Only part of the packages in the jar are white listed.
Metadata
Metadata for the pom.xml file.
18.6.5.3. KIE base Settings
KIE base Settings edits the kmodule.xml file used by Drools.
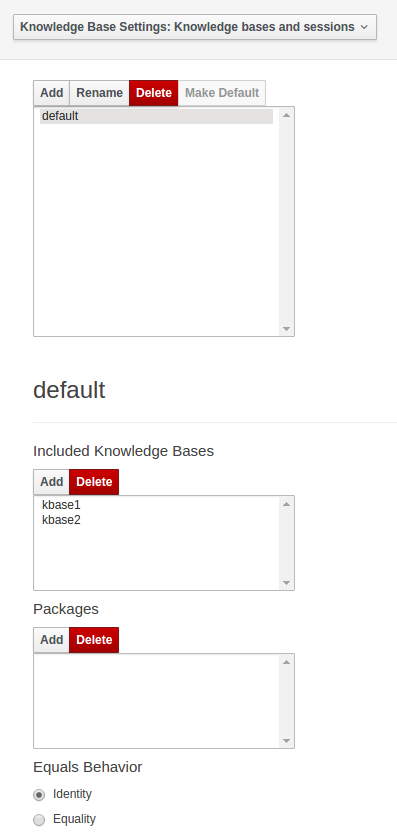
For more information about the KIE base properties, check the Drools Expert documentation for kmodule.xml. |
KIE bases and sessions
KIE bases and sessions lists the KIE bases and the KIE sessions specified for the project.
Lists all the KIE bases by name. Only one KIE base can be set as default.
KIE base can include other KIE bases. The models, rules and any other content in the included KIE base will be visible and usable by the currently selected KIE base.
Rules and models are stored in packages. The packages property specifies what packages are included into this KIE base.
Equals behavior is explained in the Drools Expert part of the documentation.
Event processing mode is explained in the Drools Fusion part of the documentation.
The table lists all the KIE sessions in the selected KIE base. There can be only one default of each type. The types are stateless and stateful. Clicking the pen-icon opens a popup that shows more properties for the KIE session.
Metadata
Metadata for the kmodule.xml
18.6.5.4. Imports
Settings edits the project.imports
file used by the Business Central editors.
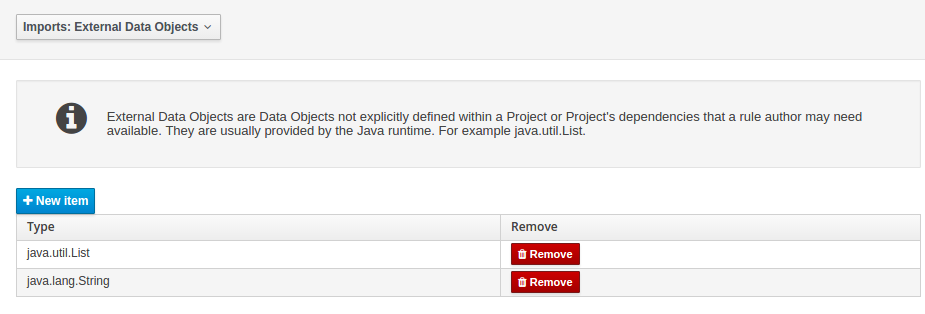
External Data Objects
Data Objects provided by the Java Runtime environment may need to be registered to be available to rule authoring where such Data Objects are not implicitly available as part of an existing Data Object defined within the Business Central or a Project dependency.
For example an Author may want to define a rule that checks for java.util.ArrayList
in Working Memory.
If a domain Data Object has a field of type java.util.ArrayList
there is no need create a registraton.
Metadata
Metadata for the project.imports file.
18.6.5.5. Duplicate GAV detection
When performing any of the following operations a check is now made against all Maven Repositories, resolved for the Project, for whether the Project’s GroupId, ArtifactId and Version pre-exist.
If a clash is found the operation is prevented; although this can be overridden by Users with the admin
role.
The feature can be disabled by setting the System Property |
Resolved repositories are those discovered in:-
-
The Project’s
POM
section (or any parent<repositories>
POM
). -
The Project’s
POM
section.<distributionManagement>
-
Maven’s global
settings.xml
configuration file.
Affected operations:-
-
Creation of new Managed Repositories.
-
Saving a Project defintion with the Project Editor.
-
Adding new Modules to a Managed Multi-Module Repository.
-
Saving the
pom.xml
file. -
Build & installing a Project with the Project Editor.
-
Build & deploying a Project with the Project Editor.
-
Asset Management operations building, installing or deloying Projects.
-
REST
operations creating, installing or deploying Projects.
Users with the Admin
role can override the list of Repositories checked using the "Repositories" settings in the Project Editor.
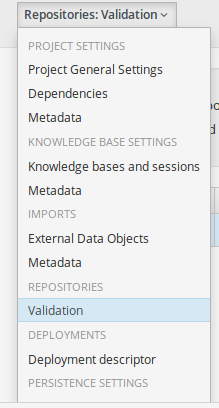
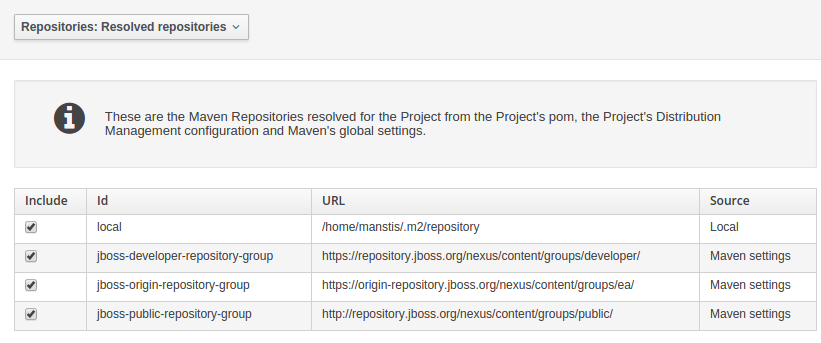
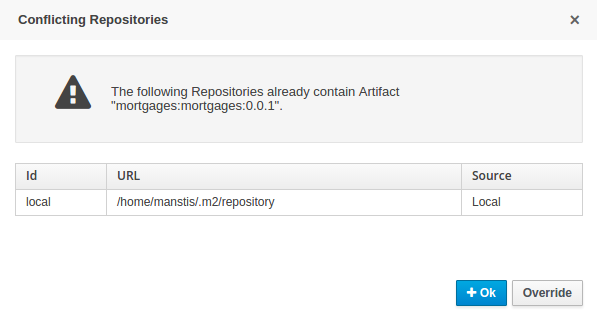
18.6.6. Validation
Business Central provides a common and consistent service for users to understand whether files authored within the environment are valid.
18.6.6.1. Problem Panel
The Problems Panel shows real-time validation results of assets within a Project.
When a Project is selected from the Project Explorer the Problems Panel will refresh with validation results of the chosen Project.
When files are created, saved or deleted the Problems Panel content will update to show either new validation errors, or remove existing if a file was deleted.
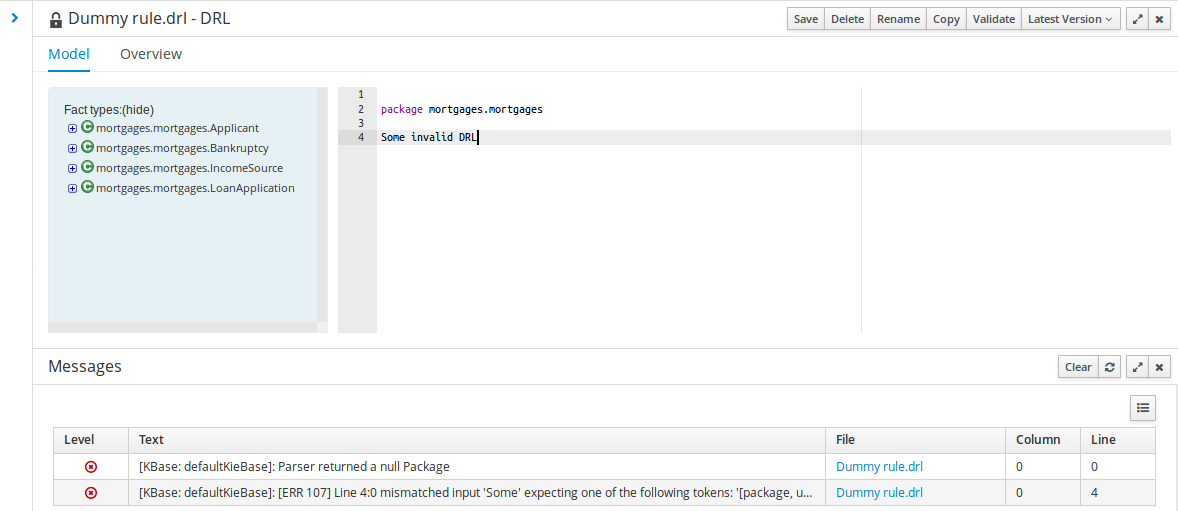
18.6.6.2. On demand validation
It is not always desirable to save a file in order to determine whether it is in a valid state.
All of the file editors provide the ability to validate the content before it is saved.
Clicking on the 'Validate' button shows validation errors, if any.
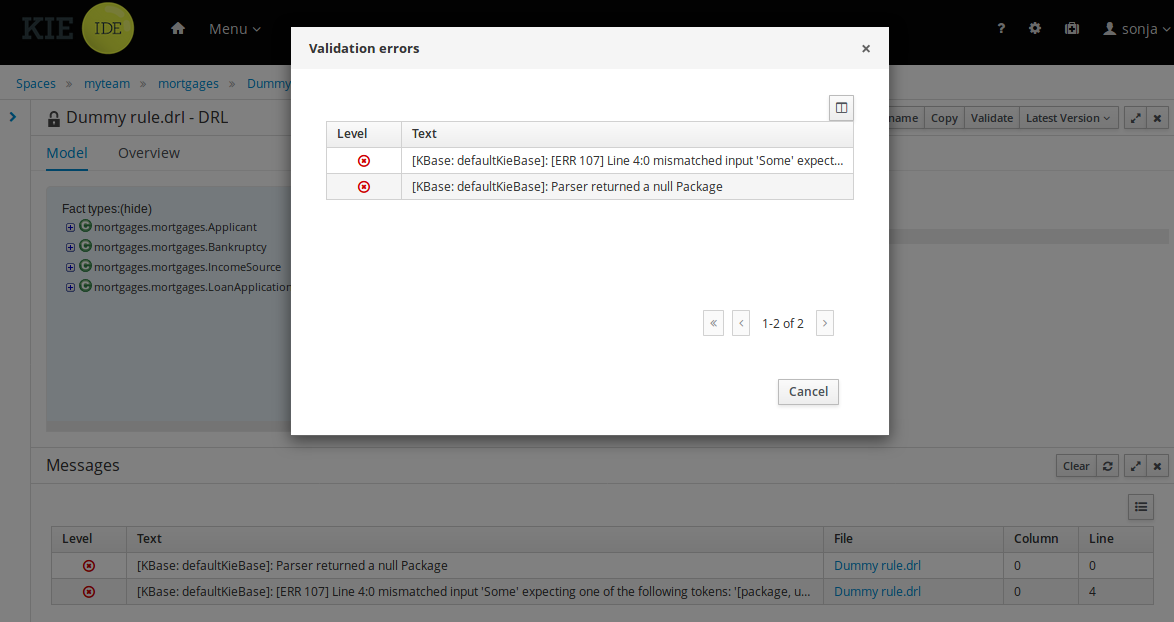
18.6.7. Data Modeller
18.6.7.1. First steps to create a data model
By default, a data model is always constrained to the context of a project. For the purpose of this tutorial, we will assume that a correctly configured project already exists and the authoring page is open.
To start the creation of a data model inside a project, take the following steps:
-
From the home panel, select the Desing page and select the given project.
Figure 176. Go to authoring page and select a project -
Open the Data Modeller tool by clicking on a Data Object file, or using the "Add Asset → Data Object" menu option. Set Data Object name to "PurchaseOrder" and click Ok.
Figure 177. Click on a Data Object
This will start up the Data Modeller tool, which has the following general aspect:
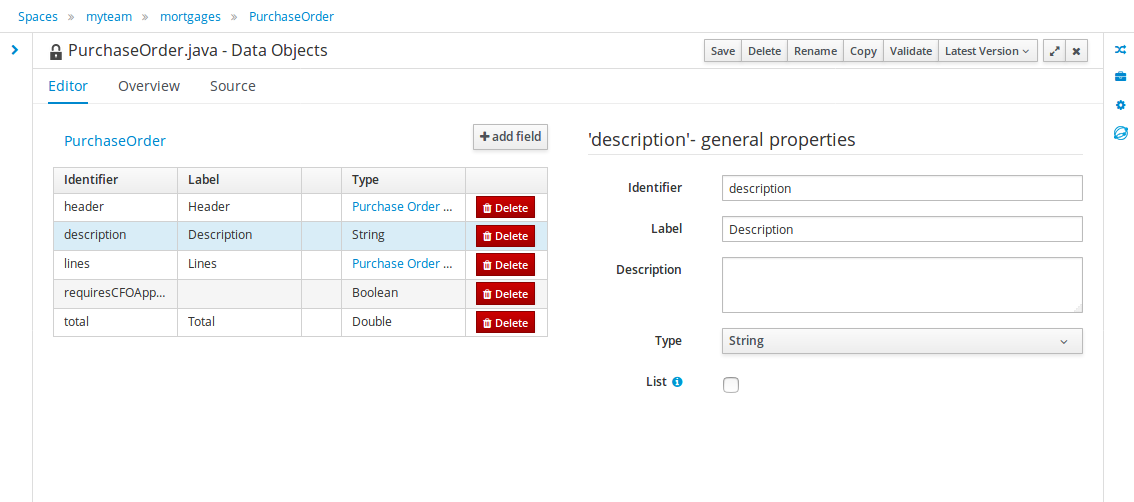
The "Editor" tab is divided into the following sections:
-
The new field section is dedicated to the creation of new fields, and is opened when the "add field" button is pressed.
Figure 179. New field creation -
The Data Object’s "field browser" section displays a list with the data object fields.
Figure 180. The Data Object’s field browser -
The "Data Object / Field general properties" section. This is the rightmost section of the Data Modeller editor and visualizes the "Data Object" or "Field" general properties, depending on user selection.
Data Object general properties can be selected by clicking on the Data Object Selector.
Figure 181. Data Object selectorFigure 182. Data Object general propertiesField general properties can be selected by clicking on a field.
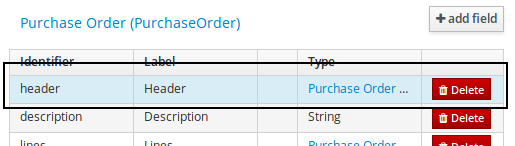
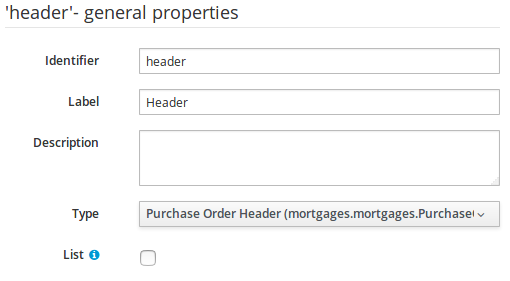
-
On the right side of Business Central a new "Tool Bar" is provided that enables the selection of different context sensitive tool windows that will let the user do domain specific configurations. Currently four tool windows are provided for the following domains "Drools & jBPM", "OptaPlanner", "Persistence" and "Advanced" configurations.
Figure 185. Data modeller Tool BarFigure 186. Drools & jBPM tool windowFigure 187. OptaPlanner tool windowTo see and use the OptaPlanner tool window, the user needs to have the role
plannermgmt
.Figure 188. Persistence tool windowFigure 189. Advanced tool window
The "Source" tab shows an editor that allows the visualization and modification of the generated java code.
-
Round trip between the "Editor" and "Source" tabs is possible, and also source code preservation is provided. It means that not matter where the Java code was generated (e.g. Eclipse, Data modeller), the data modeller will only update the necessary code blocks to maintain the model updated.
Figure 190. Source editor
The "Overview" tab shows the standard metadata and version information as the other workbench editors.
18.6.7.2. Data Objects
A data model consists of data objects which are a logical representation of some real-world data. Such data objects have a fixed set of modeller (or application-owned) properties, such as its internal identifier, a label, description, package etc. Besides those, a data object also has a variable set of user-defined fields, which are an abstraction of a real-world property of the type of data that this logical data object represents.
Creating a data object can be achieved using the Business Central "New Item - Data Object" menu option.
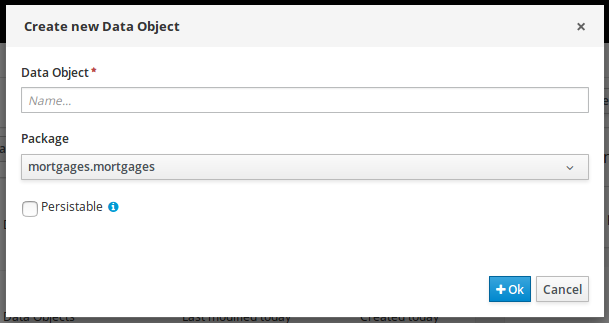
Both resource name and location are mandatory parameters. When the "Ok" button is pressed a new Java file will be created and a new editor instance will be opened for the file edition. The optional "Persistable" attribute will add by default configurations on the data object in order to make it a JPA entity. Use this option if your jBPM project needs to store data object’s information in a data base.
18.6.7.3. Properties & relationships
Once the data object has been created, it now has to be completed by adding user-defined properties to its definition. This can be achieved by pressing the "add field" button. The "New Field" dialog will be opened and the new field can be created by pressing the "Create" button. The "Create and continue" button will also add the new field to the Data Object, but won’t close the dialog. In this way multiple fields can be created avoiding the popup opening multiple times. The following fields can (or must) be filled out:
-
The field’s internal identifier (mandatory). The value of this field must be unique per data object, i.e. if the proposed identifier already exists within current data object, an error message will be displayed.
-
A label (optional): as with the data object definition, the user can define a user-friendly label for the data object field which is about to be created. This has no further implications on how fields from objects of this data object will be treated. If a label is defined, then this is how the field will be displayed throughout the data modeller tool.
-
A field type (mandatory): each data object field needs to be assigned with a type.
This type can be either of the following:
-
A 'primitive java object' type: these include most of the object equivalents of the standard Java primitive types, such as Boolean, Short, Float, etc, as well as String, Date, BigDecimal and BigInteger.
Figure 192. Primitive object field types -
A 'data object' type: any user defined data object automatically becomes a candidate to be defined as a field type of another data object, thus enabling the creation of relationships between them. A data object field can be created either in 'single' or in 'multiple' form, the latter implying that the field will be defined as a collection of this type, which will be indicated by selecting "List" checkbox.
-

-
A 'primitive java' type: these include java primitive types byte, short, int, long, float, double, char and boolean.
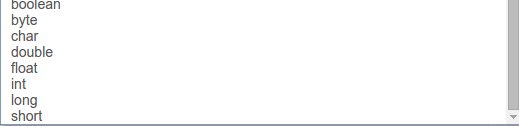
When finished introducing the initial information for a new field, clicking the 'Create' button will add the newly created field to the end of the data object’s fields table below:
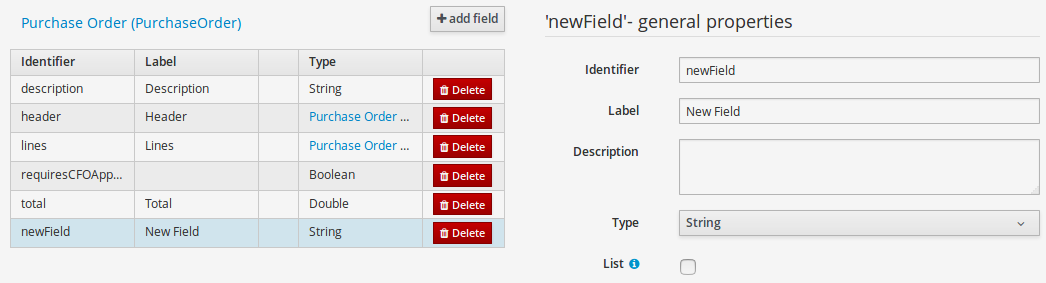
The new field will also automatically be selected in the data object’s field list, and its properties will be shown in the Field general properties editor. Additionally the field properties will be loaded in the different tool windows, in this way the field will be ready for edition in whatever selected tool window.
At any time, any field (without restrictions) can be deleted from a data object definition by clicking on the corresponding 'x' icon in the data object’s fields table.
18.6.7.4. Additional options
As stated before, both Data Objects as well as Fields require some of their initial properties to be set upon creation. Additionally there are three domains of properties that can be configured for a given Data Object. A domain is basically a set of properties related to a given business area. Current available domains are, "Drools & jJBPM", "Persistence" and the "Advanced" domain. To work on a given domain the user should select the corresponding "Tool window" (see below) on the right side toolbar. Every tool window usually provides two editors, the "Data Object" level editor and the "Field" level editor, that will be shown depending on the last selected item, the Data Object or the Field.
Drools & jBPM domain
The Drools & jBPM domain editors manages the set of Data Object or Field properties related to drools applications.
The Drools & jBPM object editor manages the object level drools properties
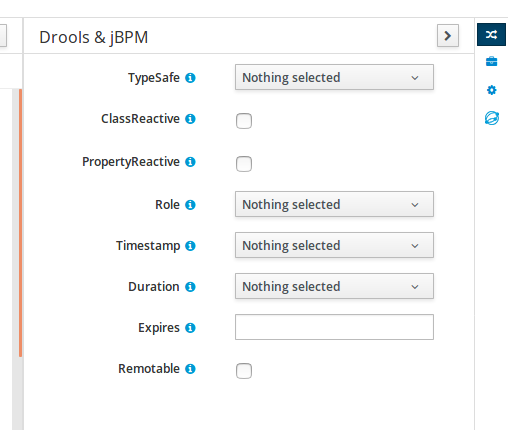
-
TypeSafe: this property allows to enable/disable the type safe behaviour for current type. By default all type declarations are compiled with type safety enabled. (See Drools for more information on this matter).
-
ClassReactive: this property allows to mark this type to be treated as "Class Reactive" by the Drools engine. (See Drools for more information on this matter).
-
PropertyReactive: this property allows to mark this type to be treated as "Property Reactive" by the Drools engine. (See Drools for more information on this matter).
-
Role: this property allows to configure how the Drools engine should handle instances of this type: either as regular facts or as events. By default all types are handled as a regular fact, so for the time being the only value that can be set is "Event" to declare that this type should be handled as an event. (See Drools Fusion for more information on this matter).
-
Timestamp: this property allows to configure the "timestamp" for an event, by selecting one of his attributes. If set the Drools engine will use the timestamp from the given attribute instead of reading it from the Session Clock. If not, the Drools engine will automatically assign a timestamp to the event. (See Drools Fusion for more information on this matter).
-
Duration: this property allows to configure the "duration" for an event, by selecting one of his attributes. If set the Drools engine will use the duration from the given attribute instead of using the default event duration = 0. (See Drools Fusion for more information on this matter).
-
Expires: this property allows to configure the "time offset" for an event expiration. If set, this value must be a temporal interval in the form: [d][#h][#m][#s][[ms]] Where [ ] means an optional parameter and # means a numeric value. e.g.: 1d2h, means one day and two hours. (See Drools Fusion for more information on this matter).
-
Remotable: If checked this property makes the Data Object available to be used with jBPM remote services as REST, JMS and WS. (See jBPM for more information on this matter).
The Drools & jBPM object editor manages the field level drools properties
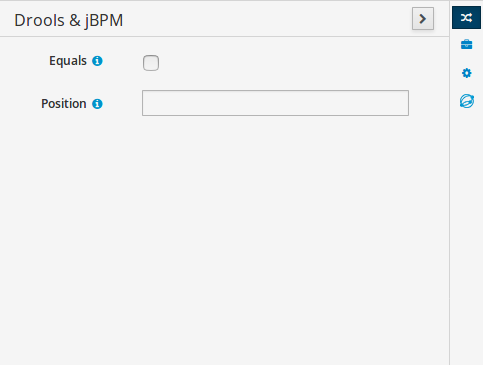
-
Equals: checking this property for a Data Object field implies that it will be taken into account, at the code generation level, for the creation of both the equals() and hashCode() methods in the generated Java class. We will explain this in more detail in the following section.
-
Position: this field requires a zero or positive integer. When set, this field will be interpreted by the Drools engine as a positional argument (see the section below and also the Drools documentation for more information on this subject).
Persistence domain
The Persistence domain editors manages the set of Data Object or Field properties related to persistence.
Persistence domain object editor manages the object level persistence properties
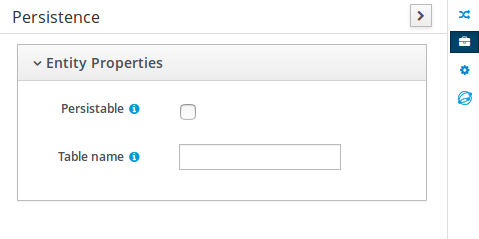
-
Persistable: this property allows to configure current Data Object as persistable.
-
Table name: this property allows to set a user defined database table name for current Data Object.
The persistence domain field editor manages the field level persistence properties and is divided in three sections.
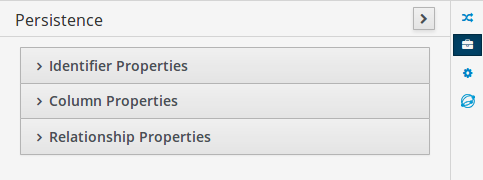
A persistable Data Object should have one and only one field defined as the Data Object identifier. The identifier is typically a unique number that distinguishes a given Data Object instance from all other instances of the same class.
-
Is Identifier: marks current field as the Data Object identifier. A persistable Data Object should have one and only one field marked as identifier, and it should be a base java type, like String, Integer, Long, etc. A field that references a Data Object, or is a multiple field can not be marked as identifier. And also composite identifiers are not supported in this version. When a persistable Data Object is created an identifier field is created by default with the properly initializations, it’s strongly recommended to use this identifier.
-
Generation Strategy: the generation strategy establishes how the identifier values will be automatically generated when the Data Object instances are created and stored in a database. (e.g. by the forms associated to jBPM processes human tasks.) When the by default Identifier field is created, the generation strategy will be also automatically set and it’s strongly recommended to use this configuration.
-
Sequence Generator: the generator represents the seed for the values that will be used by the Generation Strategy. When the by default Identifier field is created the Sequence Generator will be also automatically generated and properly configured to be used by the Generation Strategy.
The column properties section enables the customization of some properties of the database column that will store the field value.
-
Column name: optional value that sets the database column name for the given field.
-
Unique: When checked the unique property establishes that current field value should be a unique key when stored in the database. (if not set the default value is false)
-
Nullable: When checked establishes that current field value can be null when stored in a database. (if not set the default value is true)
-
Insertable: When checked establishes that column will be included in SQL INSERT statements generated by the persistence provider. (if not set the default value is true)
-
Updatable: When checked establishes that the column will be included SQL UPDATE statements generated by the persistence provider. (if not set the default value is true)
When the field’s type is a Data Object type, or a list of a Data Object type a relationship type should be set in order to let the persistence provider to manage the relation. Fortunately this relation type is automatically set when such kind of fields are added to an already marked as persistable Data Object. The relationship type is set by the following popup.
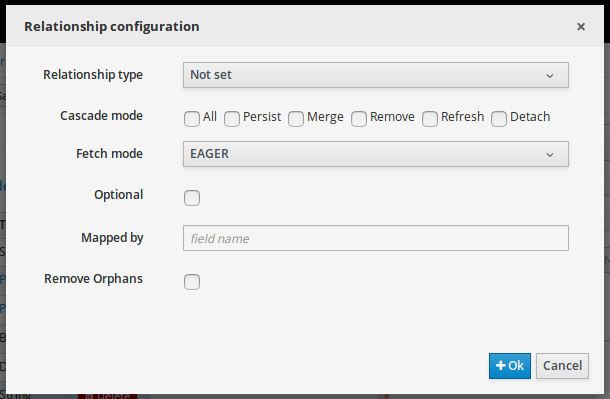
-
Relationship type: sets the type of relation from one of the following options:
One to one: typically used for 1:1 relations where "A is related to one instance of B", and B exists only when A exists. e.g. PurchaseOrder → PurchaseOrderHeader (a PurchaseOrderHeader exists only if the PurchaseOrder exists)
One to many: typically used for 1:N relations where "A is related to N instances of B", and the related instances of B exists only when A exists. e.g. PurchaseOrder → PurchaseOrderLine (a PurchaseOrderLine exists only if the PurchaseOrder exists)
Many to one: typically used for 1:1 relations where "A is related to one instance of B", and B can exist even without A. e.g. PurchaseOrder → Client (a Client can exist in the database even without an associated PurchaseOrder)
Many to many: typically used for N:N relations where "A can be related to N instances of B, and B can be related to M instances of A at the same time", and both B an A instances can exits in the database independently of the related instances. e.g. Course → Student. (Course can be related to N Students, and a given Student can attend to M courses)
When a field of type "Data Object" is added to a given persistable Data Object, the "Many to One" relationship type is generated by default.
And when a field of type "list of Data Object" is added to a given persistable Data Object , the "One to Many" relationship is generated by default.
-
Cascade mode: Defines the set of cascadable operations that are propagated to the associated entity. The value cascade=ALL is equivalent to cascade={PERSIST, MERGE, REMOVE, REFRESH}. e.g. when A → B, and cascade "PERSIST or ALL" is set, if A is saved, then B will be also saved.
The by default cascade mode created by the data modeller is "ALL" and it’s strongly recommended to use this mode when Data Objects are being used by jBPM processes and forms.
-
Fetch mode: Defines how related data will be fetched from database at reading time.
EAGER: related data will be read at the same time. e.g. If A → B, when A is read from database B will be read at the same time.
LAZY: reading of related data will be delayed usually to the moment they are required. e.g. If PurchaseOrder → PurchaseOrderLine the lines reading will be postponed until a method "getLines()" is invoked on a PurchaseOrder instance.
The default fetch mode created by the data modeller is "EAGER" and it’s strongly recommended to use this mode when Data Objects are being used by jBPM processes and forms.
-
Optional: establishes if the right side member of a relationship can be null.
-
Mapped by: used for reverse relations.
Advanced domain
The advanced domain enables the configuration of whatever parameter set by the other domains as well as the adding of arbitrary parameters. As it will be shown in the code generation section every "Data Object / Field" parameter is represented by a java annotation. The advanced mode enables the configuration of this annotations.
The advanced domain editor has the same shape for both Data Object and Field.
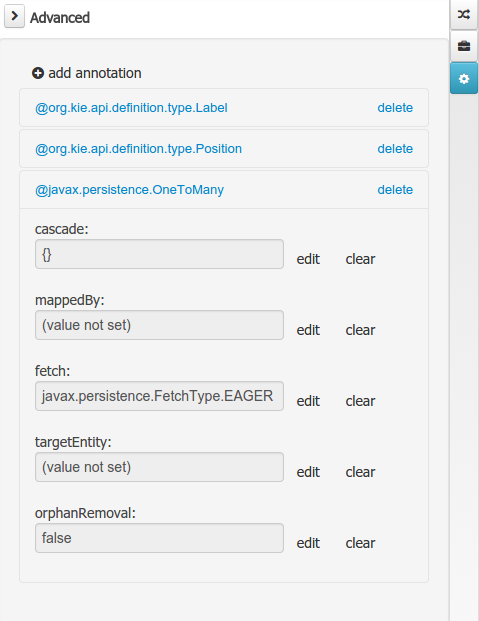
The following operations are available
-
delete: enables the deletion of a given Data Object or Field annotation.
-
clear: clears a given annotation parameter value.
-
edit: enables the edition of a given annotation parameter value.
-
add annotation: The add annotation button will start a wizard that will let the addition of whatever java annotation available in the project dependencies.
Add annotation wizard step #1: the first step of the wizard requires the entering of a fully qualified class name of an annotation, and by pressing the "search" button the annotation definition will be loaded into the wizard. Additionally when the annotation definition is loaded, different wizard steps will be created in order to enable the completion of the different annotation parameters. Required parameters will be marked with "*".
Figure 202. Annotation definition loaded into the wizard.Whenever it’s possible the wizard will provide a suitable editor for the given parameters.
Figure 203. Automatically generated enum values editor for an Enumeration annotation parameter.A generic parameter editor will be provided when it’s not possible to calculate a customized editor
Figure 204. Generic annotation parameter editorWhen all required parameters has been entered and validated, the finish button will be enabled and the wizard can be completed by adding the annotation to the given Data Object or Field.
18.6.7.5. Generate data model code.
The data model in itself is merely a visual tool that allows the user to define high-level data structures, for them to interact with the Drools engine on the one hand, and the jBPM platform on the other. In order for this to become possible, these high-level visual structures have to be transformed into low-level artifacts that can effectively be consumed by these platforms. These artifacts are Java POJOs (Plain Old Java Objects), and they are generated every time the data model is saved, by pressing the "Save" button in the top Data Modeller Menu. Additionally when the user round trip between the "Editor" and "Source" tab, the code is auto generated to maintain the consistency with the Editor view and vice versa.

The resulting code is generated according to the following transformation rules:
-
The data object’s identifier property will become the Java class’s name. It therefore needs to be a valid Java identifier.
-
The data object’s package property becomes the Java class’s package declaration.
-
The data object’s superclass property (if present) becomes the Java class’s extension declaration.
-
The data object’s label and description properties will translate into the Java annotations "@org.kie.api.definition.type.Label" and "@org.kie.api.definition.type.Description", respectively. These annotations are merely a way of preserving the associated information, and as yet are not processed any further.
-
The data object’s role property (if present) will be translated into the "@org.kie.api.definition.type.Role" Java annotation, that IS interpreted by the application platform, in the sense that it marks this Java class as a Drools Event Fact-Type.
-
The data object’s type safe property (if present) will be translated into the "@org.kie.api.definition.type.TypeSafe Java annotation. (see Drools)
-
The data object’s class reactive property (if present) will be translated into the "@org.kie.api.definition.type.ClassReactive Java annotation. (see Drools)
-
The data object’s property reactive property (if present) will be translated into the "@org.kie.api.definition.type.PropertyReactive Java annotation. (see Drools)
-
The data object’s timestamp property (if present) will be translated into the "@org.kie.api.definition.type.Timestamp Java annotation. (see Drools)
-
The data object’s duration property (if present) will be translated into the "@org.kie.api.definition.type.Duration Java annotation. (see Drools)
-
The data object’s expires property (if present) will be translated into the "@org.kie.api.definition.type.Expires Java annotation. (see Drools)
-
The data object’s remotable property (if present) will be translated into the "@org.kie.api.remote.Remotable Java annotation. (see jBPM)
A standard Java default (or no parameter) constructor is generated, as well as a full parameter constructor, i.e. a constructor that accepts as parameters a value for each of the data object’s user-defined fields.
The data object’s user-defined fields are translated into Java class fields, each one of them with its own getter and setter method, according to the following transformation rules:
-
The data object field’s identifier will become the Java field identifier. It therefore needs to be a valid Java identifier.
-
The data object field’s type is directly translated into the Java class’s field type. In case the field was declared to be multiple (i.e. 'List'), then the generated field is of the "java.util.List" type.
-
The equals property: when it is set for a specific field, then this class property will be annotated with the "@org.kie.api.definition.type.Key" annotation, which is interpreted by the Drools engine, and it will 'participate' in the generated equals() method, which overwrites the equals() method of the Object class. The latter implies that if the field is a 'primitive' type, the equals method will simply compares its value with the value of the corresponding field in another instance of the class. If the field is a sub-entity or a collection type, then the equals method will make a method-call to the equals method of the corresponding data object’s Java class, or of the java.util.List standard Java class, respectively.
If the equals property is checked for ANY of the data object’s user defined fields, then this also implies that in addition to the default generated constructors another constructor is generated, accepting as parameters all of the fields that were marked with Equals. Furthermore, generation of the equals() method also implies that also the Object class’s hashCode() method is overwritten, in such a manner that it will call the hashCode() methods of the corresponding Java class types (be it 'primitive' or user-defined types) for all the fields that were marked with Equals in the Data Model.
-
The position property: this field property is automatically set for all user-defined fields, starting from 0, and incrementing by 1 for each subsequent new field. However the user can freely changes the position among the fields. At code generation time this property is translated into the "@org.kie.api.definition.type.Position" annotation, which can be interpreted by the Drools engine. Also, the established property order determines the order of the constructor parameters in the generated Java class.
As an example, the generated Java class code for the Purchase Order data object, corresponding to its definition as shown in the following figure purchase_example.jpg is visualized in the figure at the bottom of this chapter. Note that the two of the data object’s fields, namely 'header' and 'lines' were marked with Equals, and have been assigned with the positions 2 and 1, respectively).
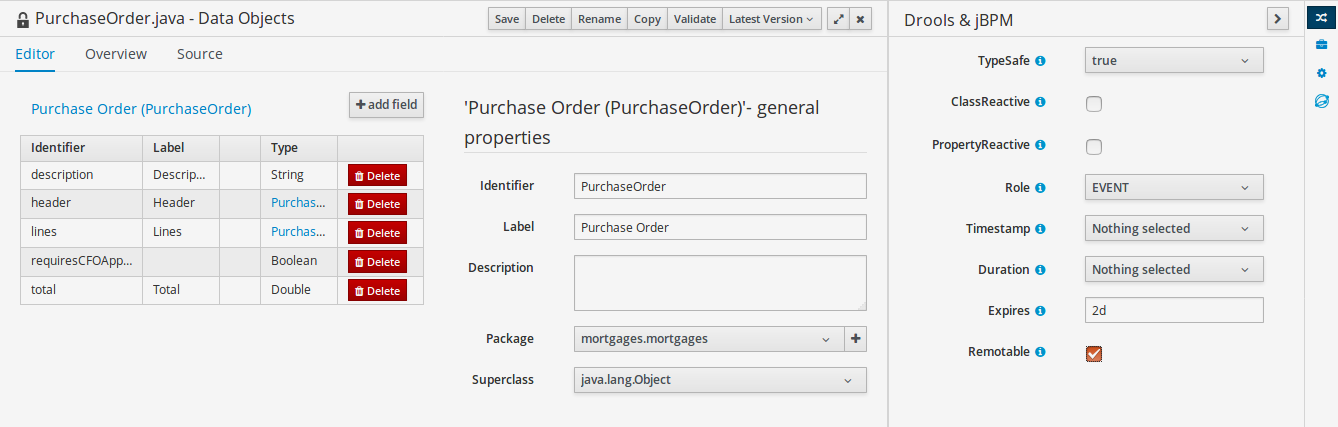
package org.jbpm.examples.purchases;
/**
* This class was automatically generated by the data modeler tool.
*/
@org.kie.api.definition.type.Label("Purchase Order")
@org.kie.api.definition.type.TypeSafe(true)
@org.kie.api.definition.type.Role(org.kie.api.definition.type.Role.Type.EVENT)
@org.kie.api.definition.type.Expires("2d")
@org.kie.api.remote.Remotable
public class PurchaseOrder implements java.io.Serializable
{
static final long serialVersionUID = 1L;
@org.kie.api.definition.type.Label("Total")
@org.kie.api.definition.type.Position(3)
private java.lang.Double total;
@org.kie.api.definition.type.Label("Description")
@org.kie.api.definition.type.Position(0)
private java.lang.String description;
@org.kie.api.definition.type.Label("Lines")
@org.kie.api.definition.type.Position(2)
@org.kie.api.definition.type.Key
private java.util.List<org.jbpm.examples.purchases.PurchaseOrderLine> lines;
@org.kie.api.definition.type.Label("Header")
@org.kie.api.definition.type.Position(1)
@org.kie.api.definition.type.Key
private org.jbpm.examples.purchases.PurchaseOrderHeader header;
@org.kie.api.definition.type.Position(4)
private java.lang.Boolean requiresCFOApproval;
public PurchaseOrder()
{
}
public java.lang.Double getTotal()
{
return this.total;
}
public void setTotal(java.lang.Double total)
{
this.total = total;
}
public java.lang.String getDescription()
{
return this.description;
}
public void setDescription(java.lang.String description)
{
this.description = description;
}
public java.util.List<org.jbpm.examples.purchases.PurchaseOrderLine> getLines()
{
return this.lines;
}
public void setLines(java.util.List<org.jbpm.examples.purchases.PurchaseOrderLine> lines)
{
this.lines = lines;
}
public org.jbpm.examples.purchases.PurchaseOrderHeader getHeader()
{
return this.header;
}
public void setHeader(org.jbpm.examples.purchases.PurchaseOrderHeader header)
{
this.header = header;
}
public java.lang.Boolean getRequiresCFOApproval()
{
return this.requiresCFOApproval;
}
public void setRequiresCFOApproval(java.lang.Boolean requiresCFOApproval)
{
this.requiresCFOApproval = requiresCFOApproval;
}
public PurchaseOrder(java.lang.Double total, java.lang.String description,
java.util.List<org.jbpm.examples.purchases.PurchaseOrderLine> lines,
org.jbpm.examples.purchases.PurchaseOrderHeader header,
java.lang.Boolean requiresCFOApproval)
{
this.total = total;
this.description = description;
this.lines = lines;
this.header = header;
this.requiresCFOApproval = requiresCFOApproval;
}
public PurchaseOrder(java.lang.String description,
org.jbpm.examples.purchases.PurchaseOrderHeader header,
java.util.List<org.jbpm.examples.purchases.PurchaseOrderLine> lines,
java.lang.Double total, java.lang.Boolean requiresCFOApproval)
{
this.description = description;
this.header = header;
this.lines = lines;
this.total = total;
this.requiresCFOApproval = requiresCFOApproval;
}
public PurchaseOrder(
java.util.List<org.jbpm.examples.purchases.PurchaseOrderLine> lines,
org.jbpm.examples.purchases.PurchaseOrderHeader header)
{
this.lines = lines;
this.header = header;
}
@Override
public boolean equals(Object o)
{
if (this == o)
return true;
if (o == null || getClass() != o.getClass())
return false;
org.jbpm.examples.purchases.PurchaseOrder that = (org.jbpm.examples.purchases.PurchaseOrder) o;
if (lines != null ? !lines.equals(that.lines) : that.lines != null)
return false;
if (header != null ? !header.equals(that.header) : that.header != null)
return false;
return true;
}
@Override
public int hashCode()
{
int result = 17;
result = 31 * result + (lines != null ? lines.hashCode() : 0);
result = 31 * result + (header != null ? header.hashCode() : 0);
return result;
}
}
18.6.7.6. Using external models
Using an external model means the ability to use a set for already defined POJOs in current project context. In order to make those POJOs available a dependency to the given JAR should be added. Once the dependency has been added the external POJOs can be referenced from current project data model.
There are two ways to add a dependency to an external JAR file:
-
Dependency to a JAR file already installed in current local M2 repository (typically associated the user home).
-
Dependency to a JAR file installed in current Business Central "Guvnor M2 repository". (internal to the application)
Dependency to a JAR file in local M2 repository
To add a dependency to a JAR file in local M2 repository follow this steps.
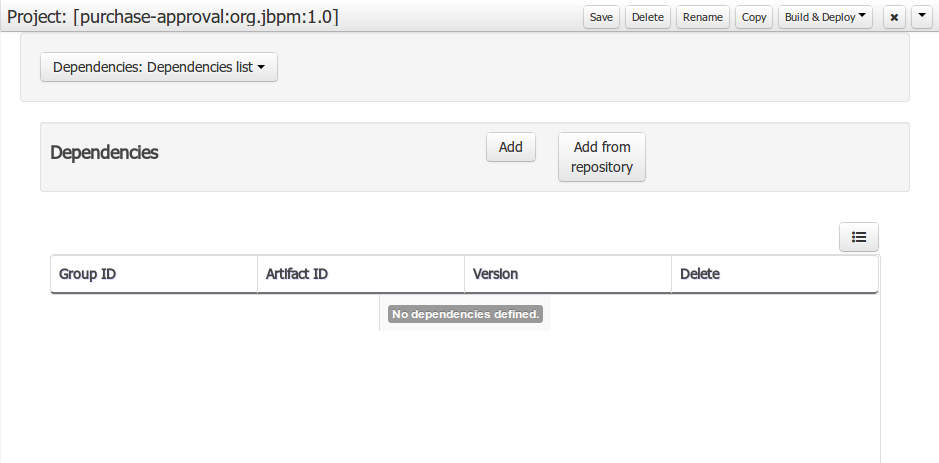
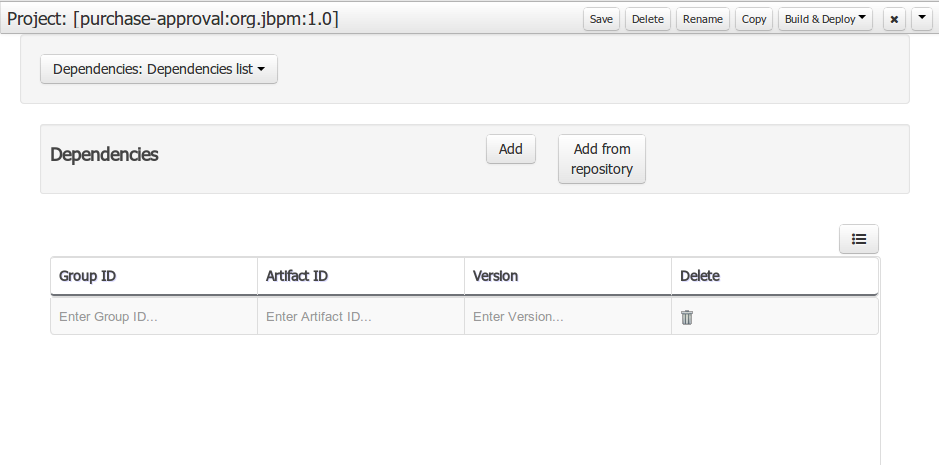
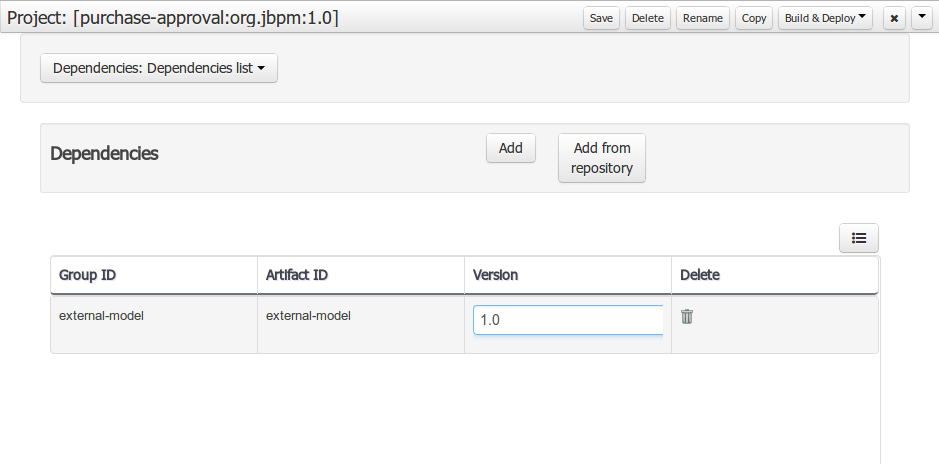
When project is saved the POJOs defined in the external file will be available.
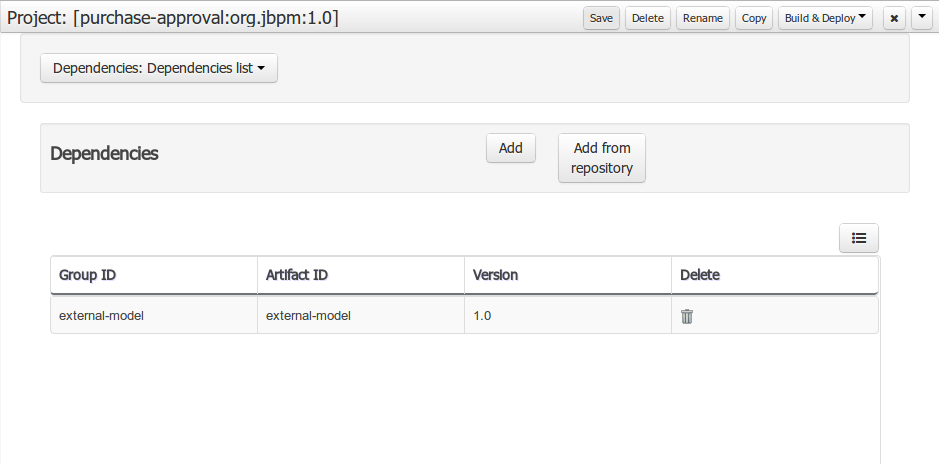
Dependency to a JAR file in current "Guvnor M2 repository".
To add a dependency to a JAR file in current "Guvnor M2 repository" follow this steps.
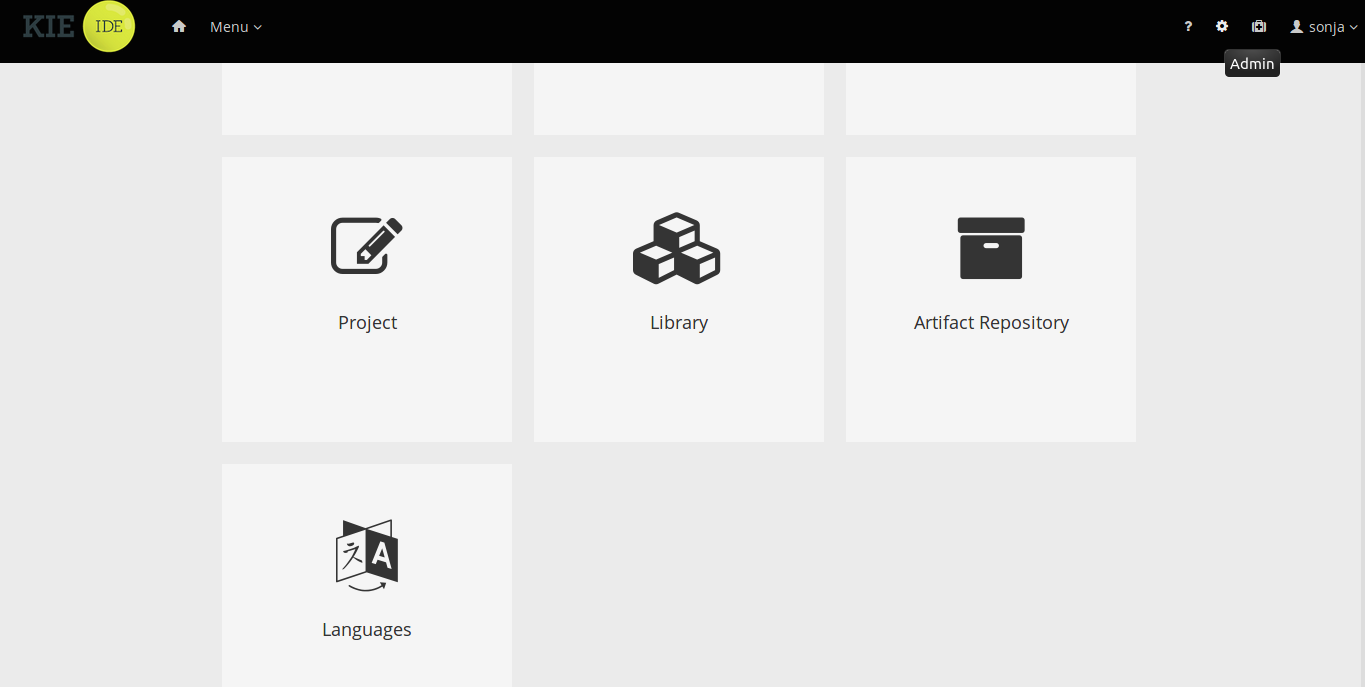
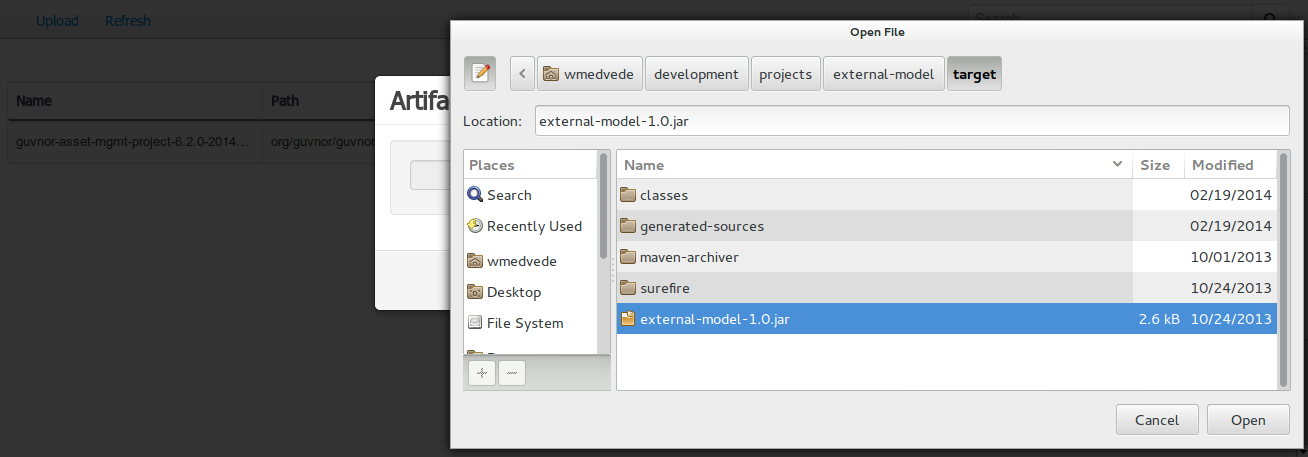
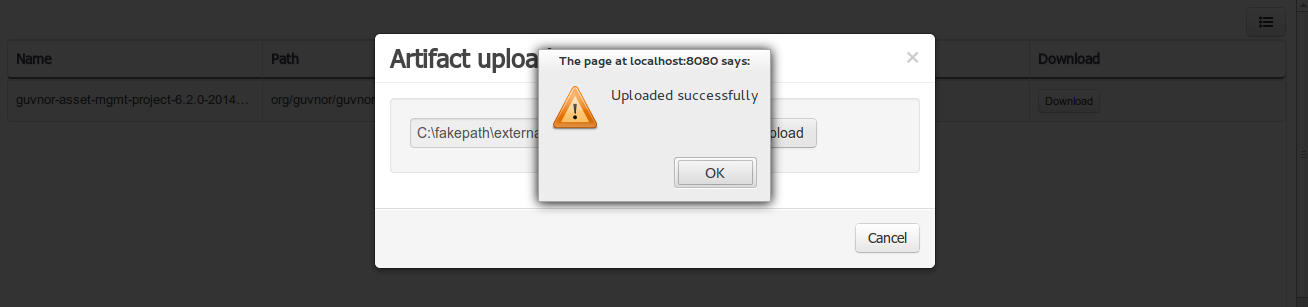
Once the file has been loaded it will be displayed in the repository files list.
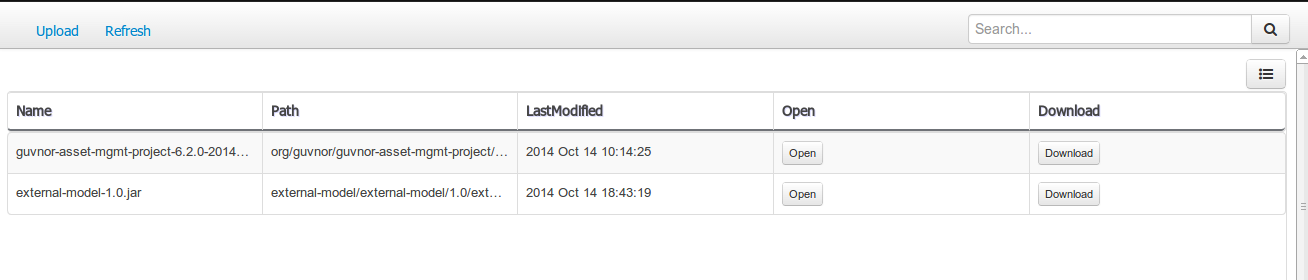
If the uploaded file is not a valid Maven JAR (don’t have a pom.xml file) the system will prompt the user in order to provide a GAV for the file to be installed.
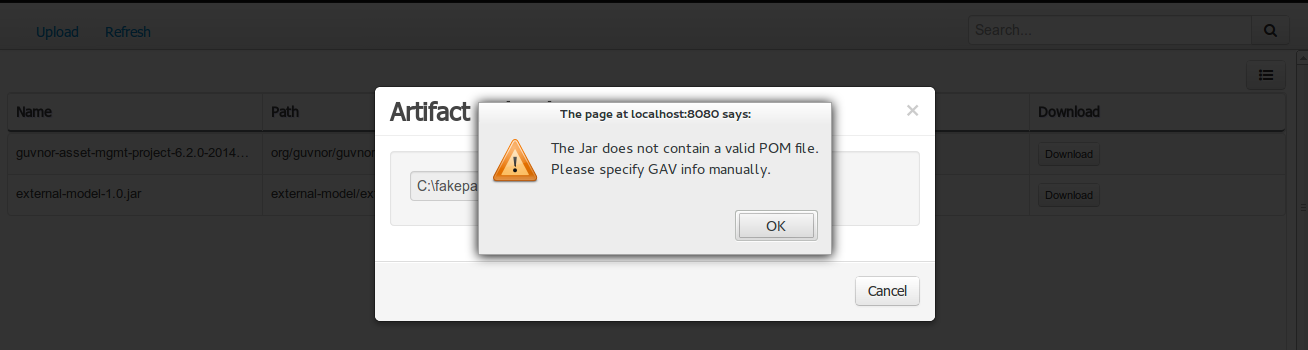
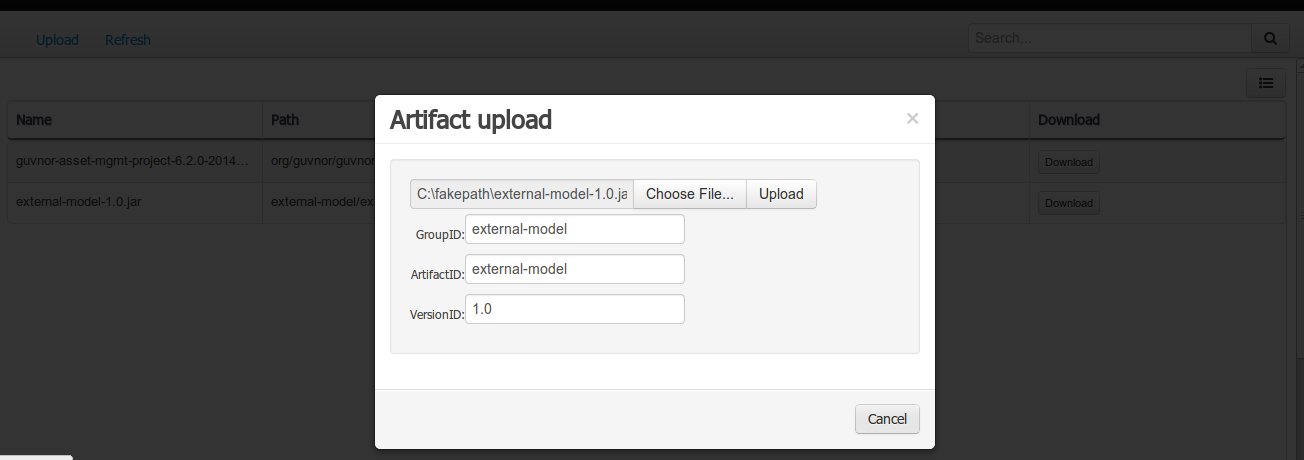
Open the project editor (see bellow) and click on the "Add from repository" button to open the JAR selector to see all the installed JAR files in current "Guvnor M2 repository". When the desired file is selected the project should be saved in order to make the new dependency available.
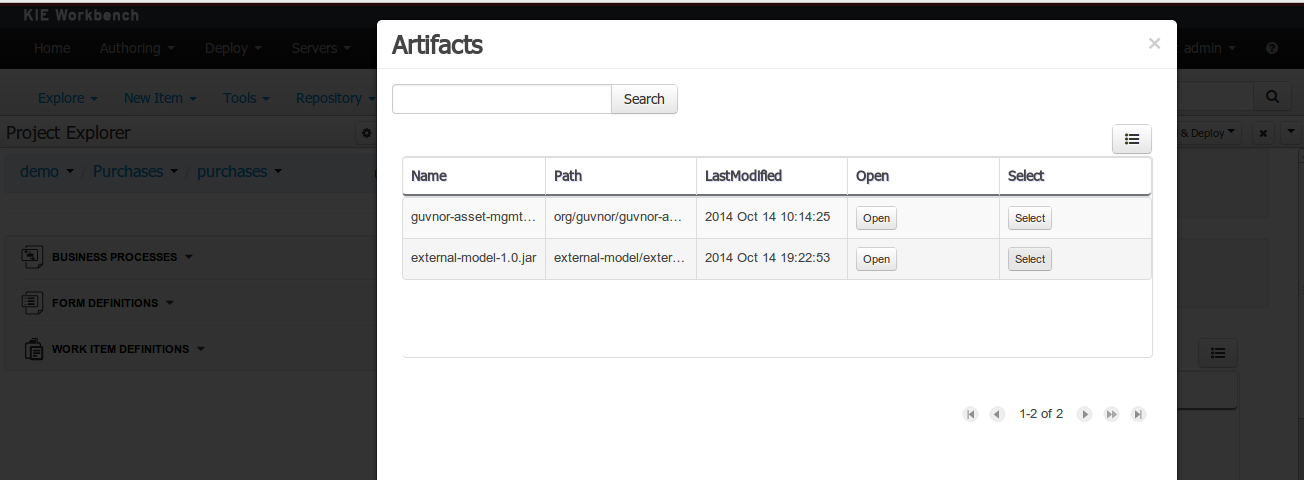
Using the external objects
When a dependency to an external JAR has been set, the external POJOs can be used in the context of current project data model in the following ways:
-
External POJOs can be extended by current model data objects.
-
External POJOs can be used as field types for current model data objects.
The following screenshot shows how external objects are prefixed with the string " -ext- " in order to be quickly identified.
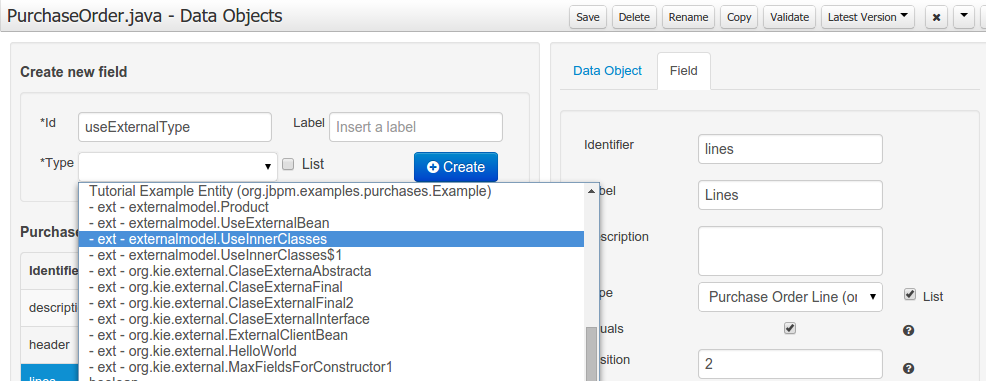
18.6.7.7. Roundtrip and concurrency
Current version implements roundtrip and code preservation between Data modeller and Java source code. No matter where the Java code was generated (e.g. Eclipse, Data modeller), the data modeller will only create/delete/update the necessary code elements to maintain the model updated, i.e, fields, getter/setters, constructors, equals method and hashCode method. Also whatever Type or Field annotation not managed by the Data Modeler will be preserved when the Java sources are updated by the Data modeller.
Aside from code preservation, like in the other Business Central editors, concurrent modification scenarios are still possible. Common scenarios are when two different users are updating the model for the same project, e.g. using the data modeller or executing a 'git push command' that modifies project sources.
From an application context’s perspective, we can basically identify two different main scenarios:
No changes have been undertaken through the application
In this scenario the application user has basically just been navigating through the data model, without making any changes to it. Meanwhile, another user modifies the data model externally.
In this case, no immediate warning is issued to the application user. However, as soon as the user tries to make any kind of change, such as add or remove data objects or properties, or change any of the existing ones, the following pop-up will be shown:
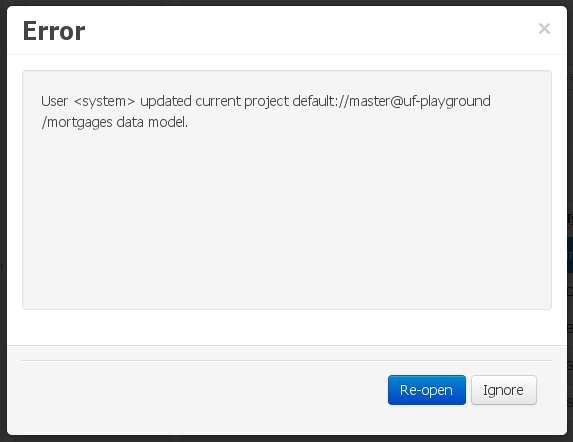
The user can choose to either:
-
Re-open the data model, thus loading any external changes, and then perform the modification he was about to undertake, or
-
Ignore any external changes, and go ahead with the modification to the model. In this case, when trying to persist these changes, another pop-up warning will be shown:
Figure 220. Force save / re-openThe "Force Save" option will effectively overwrite any external changes, while "Re-open" will discard any local changes and reload the model.
"Force Save" overwrites any external changes!
Changes have been undertaken through the application
The application user has made changes to the data model. Meanwhile, another user simultaneously modifies the data model from outside the application context.
In this alternative scenario, immediately after the external user commits his changes to the asset repository (or e.g. saves the model with the data modeller in a different session), a warning is issued to the application user:
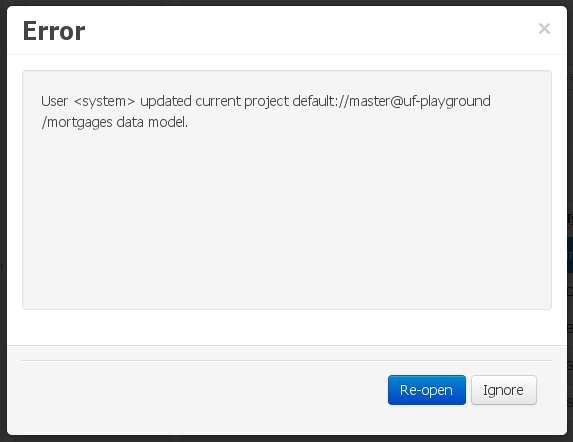
As with the previous scenario, the user can choose to either:
-
Re-open the data model, thus losing any modifications that where made through the application, or
-
Ignore any external changes, and continue working on the model.
One of the following possibilities can now occur: ** The user tries to persist the changes he made to the model by clicking the "Save" button in the data modeller top level menu. This leads to the following warning message:
+
Figure 222. Force save / re-openThe "Force Save" option will effectively overwrite any external changes, while "Re-open" will discard any local changes and reload the model.
18.6.8. Data Sets
A data set is basically a set of columns populated with some rows, a matrix of data composed of timestamps, texts and numbers. A data set can be stored in different systems: a database, an excel file, in memory or in a lot of other different systems. On the other hand, a data set definition tells Business Central modules how such data can be accessed, read and parsed.
Notice, it’s very important to make crystal clear the difference between a data set and its definition since Business Central does not take care of storing any data, it just provides an standard way to define access to those data sets regardless where the data is stored.
Let’s take for instance the data stored in a remote database. A valid data set could be, for example, an entire database table or the result of an SQL query. In both cases, the database will return a bunch of columns and rows. Now, imagine we want to get access to such data to feed some charts in a new Business Central page. First thing is to create and register a data set definition in order to indicate the following:
-
where the data set is stored,
-
how can be accessed, read and parsed and
-
what columns contains and of which type.
This chapter introduces the available Business Central tools for registering and handling data set definitions and how this definitions can be consumed in other Business Central modules like, for instance, the Page Editor.
For simplicity sake we will be using the term data set to refer to the actual data set definitions as Data set and Data set definition can be considered synonyms under the data set authoring context. |
18.6.8.1. Data Set Authoring Page
Everything related to the authoring of data sets can be found under the Data Set Authoring page which is accessible from the following top level menu entry: Extensions>Data Sets, as shown in the following screenshot.
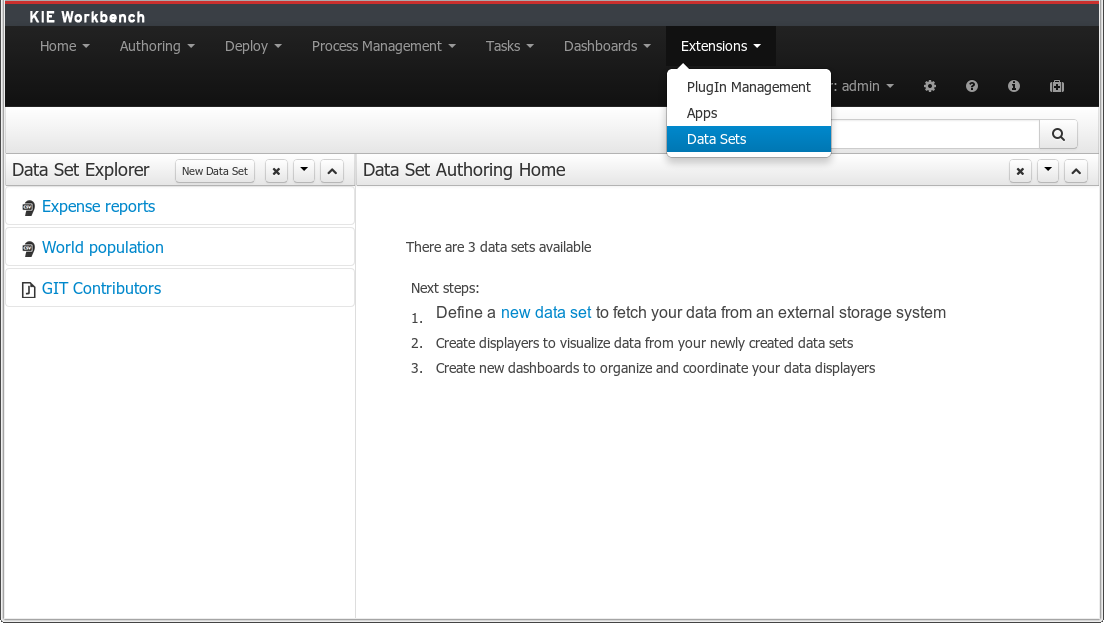
The center panel, shows a welcome screen, whilst the left panel contains the Data Set Explorer listing all the data sets available
This page is only intended to Administrator users, since defining data sets can be considered a low level task. |
18.6.8.2. Data Set Explorer
The Data Set Explorer list the data sets present in the system. Every time the user clicks on the data set it shows a brief summary alongside the following information:
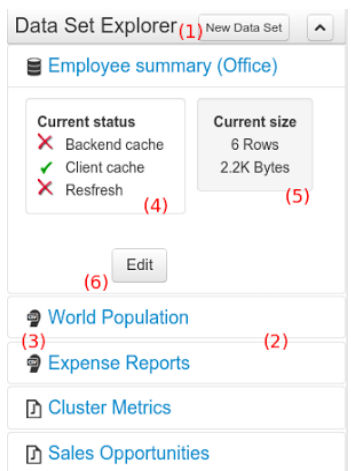
-
(1) A button for creating a new Data set
-
(2) The list of currently available Data sets
-
(3) An icon that represents the Data set’s provider type (Bean, SQL, CSV, etc)
-
(4) Details of current cache and refresh policy status
-
(5) Details of current size on backend (unit as rows) and current size on client side (unit in bytes)
-
(6) The button for editing the Data set. Once clicked the Data set editor screen is opened on the center panel
The next sections explains how to create, edit and fine tune data set definitions.
18.6.8.3. Data Set Creation
Clicking on the New Data Set button opens a new screen from which the user is able to create a new data set definition in three steps:
-
Provider type selection
Specify the kind of the remote storage system (BEAN, SQL, CSV, ElasticSearch)
-
Provider configuration
Specify the attributes for being able to look up data from the remote system. The configuration varies depending on the data provider type selected.
-
Data set columns & filter
Live data preview, column types and initial filter configuration.
Step 1: Provider type selection
Allows the user’s specify the type of data provider of the data set being created.
This screen lists all the current available data provider types and helper popovers with descriptions. Each data provider is represented with a descriptive image:
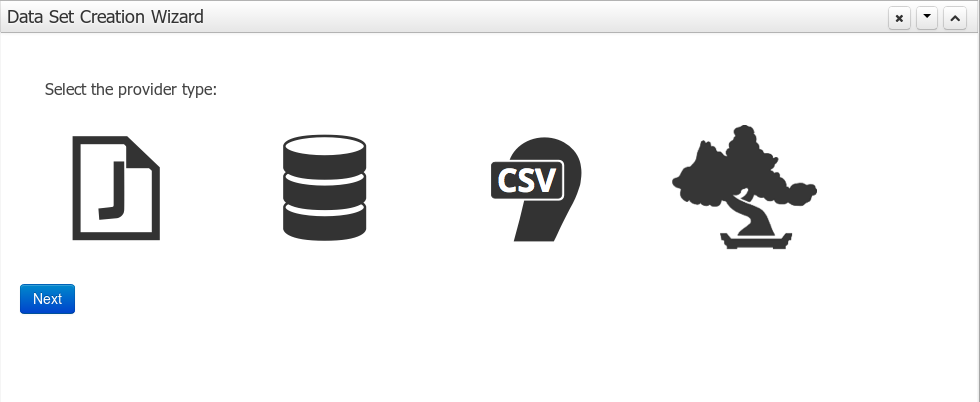
Four types are currently supported:
-
Bean (Java class) - To generate a data set directly from Java
-
SQL - For getting data from any ANSI-SQL compliant database
-
CSV - To upload the contents of a remote or local CSV file
-
Elastic Search - To query and get documents stored on Elastic Search nodes as data sets
Once a type is selected, click on Next button to continue with the next workflow step.
Step 2: Configuration
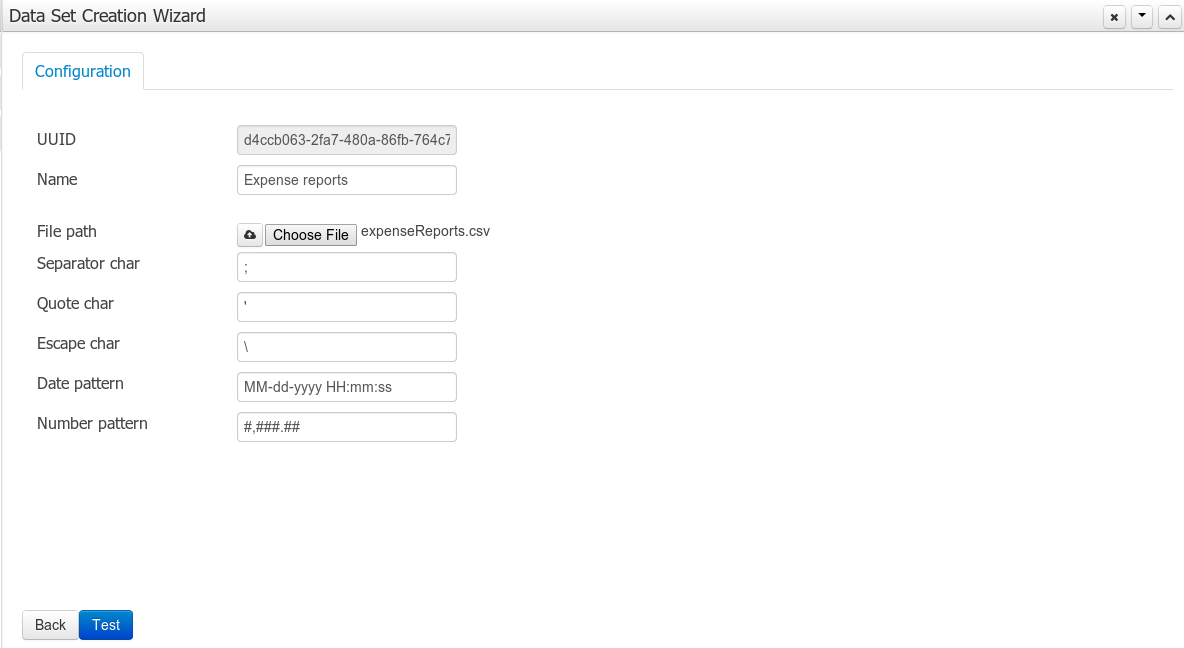
The provider type selected in the previous step will determine which configuration settings the system asks for.
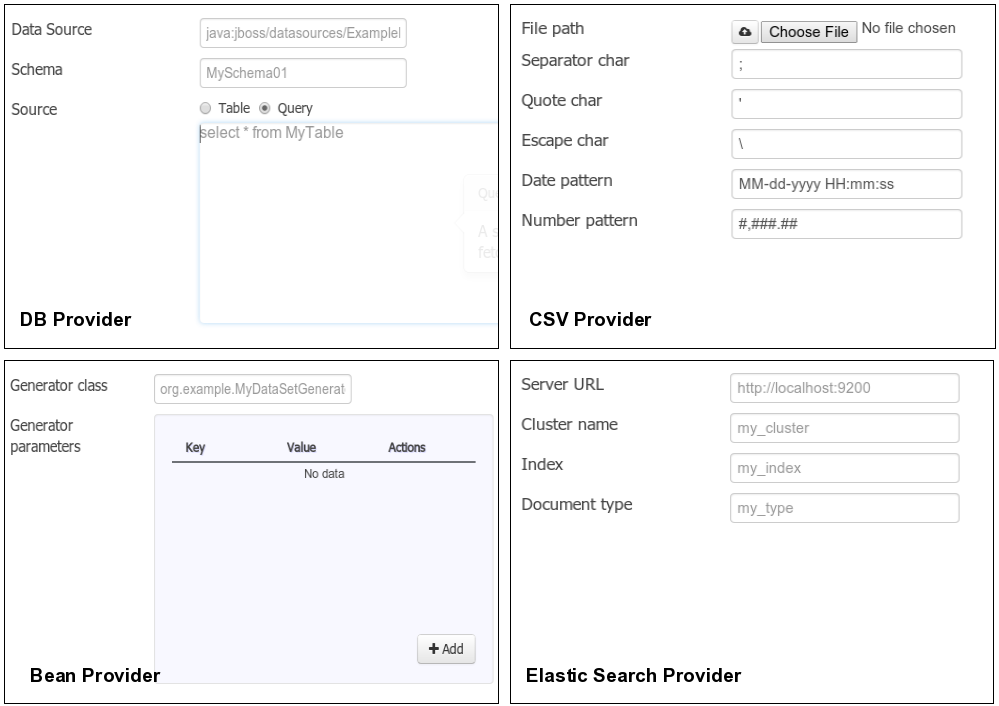
The UUID attribute is a read only field as it’s generated by the system. It’s only intended for usage in API calls or specific operations. |
Step 3: Data set columns and preview
After clicking on the Test button (see previous step), the system executes a data set lookup test call in order to check if the remote system is up and the data is available. If everything goes ok the user will see the following screen:
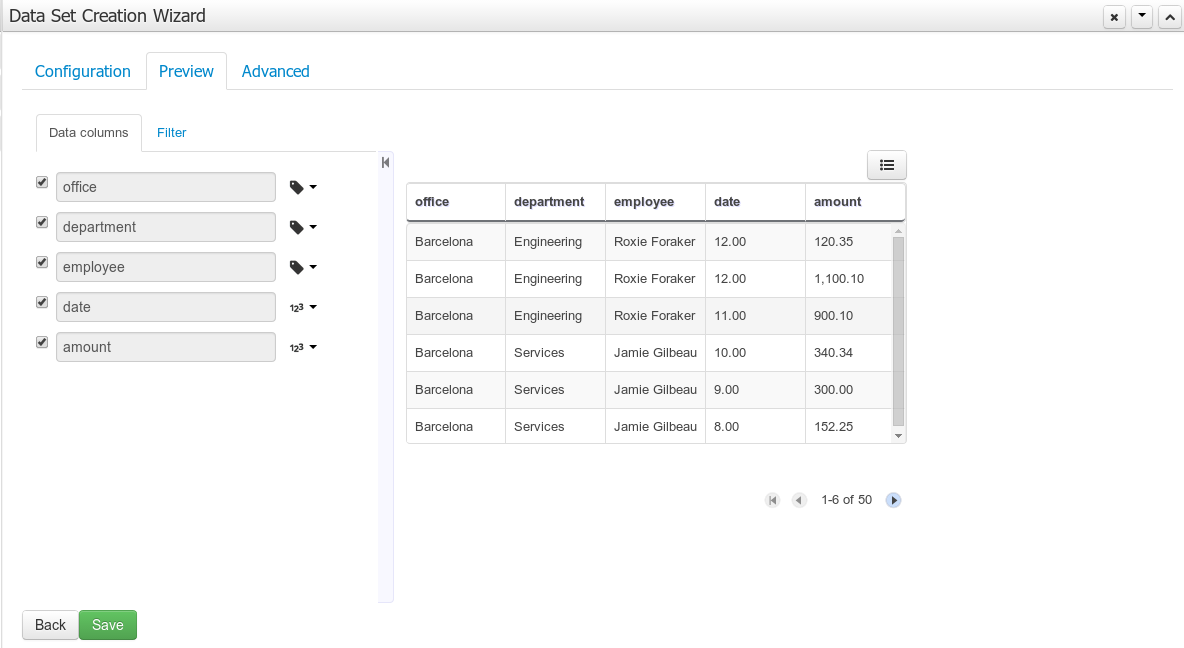
This screen shows a live data preview along with the columns the user wants to be part of the resulting data set. The user can also navigate through the data and apply some changes to the data set structure. Once finished, we can click on the Save button in order to register the new data set definition.
We can also change the configuration settings at any time just by going back to the configuration tab. We can repeat the Configuration>Test>Preview cycle as may times as needed until we consider it’s ready to be saved.
Columns
In the Columns tab area the user can select what columns are part of the resulting data set definition.
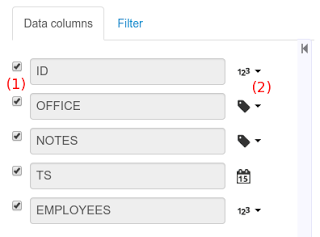
-
(1) To add or remove columns. Select only those columns you want to be part of the resulting data set
-
(2) Use the drop down image selector to change the column type
A data set may only contain columns of any of the following 4 types:
-
Label - For text values supporting group operations (similar to the SQL "group by" operator) which means you can perform data lookup calls and get one row per distinct value.
-
Text - For text values NOT supporting group operations. Typically for modeling large text columns such as abstracts, descriptions and the like.
-
Number - For numeric values. It does support aggregation functions on data lookup calls: sum, min, max, average, count, disctinct.
-
Date - For date or timestamp values. It does support time based group operations by different time intervals: minute, hour, day, month, year, …
No matter which remote system you want to retrieve data from, the resulting data set will always return a set of columns of one of the four types above. There exists, by default, a mapping between the remote system column types and the data set types. The user is able to modify the type for some columns, depending on the data provider and the column type of the remote system. The system supports the following changes to column types:
-
Label <> Text - Useful when we want to enable/disable the categorization (grouping) for the target column. For instance, imagine a database table called "document" containing a large text column called "abstract". As we do not want the system to treat such column as a "label" we might change its column type to "text". Doing so, we are optimizing the way the system handles the data set and
-
Number <> Label - Useful when we want to treat numeric columns as labels. This can be used for instance to indicate that a given numeric column is not a numeric value that can be used in aggregation functions. Despite its values are stored as numbers we want to handle the column as a "label". One example of such columns are: an item’s code, an appraisal id., …
BEAN data sets do not support changing column types as it’s up to the developer to decide which are the concrete types for each column. |
Filter
A data set definition may define a filter. The goal of the filter is to leave out rows the user does not consider necessary. The filter feature works on any data provider type and it lets the user to apply filter operations on any of the data set columns available.
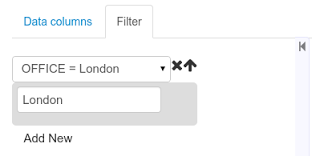
While adding or removing filter conditions and operations, the preview table on central area is updated with live data that reflects the current filter status.
There exists two strategies for filtering data sets and it’s also important to note that choosing between the two have important implications. Imagine a dashboard with some charts feeding from a expense reports data set where such data set is built on top of an SQL table. Imagine also we only want to retrieve the expense reports from the "London" office. You may define a data set containing the filter "office=London" and then having several charts feeding from such data set. This is the recommended approach. Another option is to define a data set with no initial filter and then let the individual charts to specify their own filter. It’s up to the user to decide on the best approach.
Depending on the case it might be better to define the filter at a data set level for reusing across other modules. The decision may also have impact on the performance since a filtered cached data set will have far better performance than a lot of individual non-cached data set lookup requests. (See the next section for more information about caching data sets).
Notice, for SQL data sets, the user can use both the filter feature introduced or, alternatively, just add custom filter criteria to the SQL sentence. Although, the first approach is more appropriated for non technical users since they might not have the required SQL language skills. |
18.6.8.4. Data set editor
To edit an existing data set definition go the data set explorer, expand the desired data set definition and click on the Edit button. This will cause a new editor panel to be opened and placed on the center of the screen, as shown in the next screenshot:
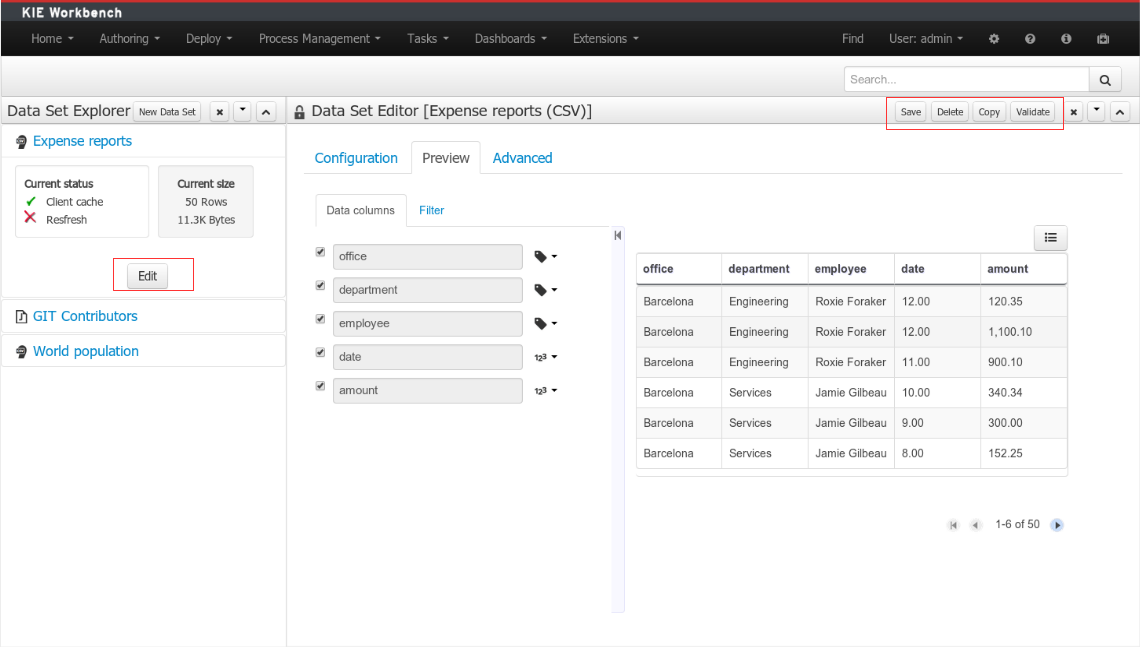

-
Save - To validate the current changes and store the data set definition.
-
Delete - To remove permanently from storage the data set definition. Any client module referencing the data set may be affected.
-
Validate - To check that all the required parameters exists and are correct, as well as to validate the data set can be retrieved with no issues.
-
Copy - To create a brand new definition as a copy of the current one.
Data set definitions are stored in the underlying GIT repository as JSON files. Any action performed is registered in the repository logs so it is possible to audit the change log later on. |
18.6.8.5. Advanced settings
In the Advanced settings tab area the user can specify caching and refresh settings. Those are very important for making the most of the system capabilities thus improving the performance and having better application responsive levels.
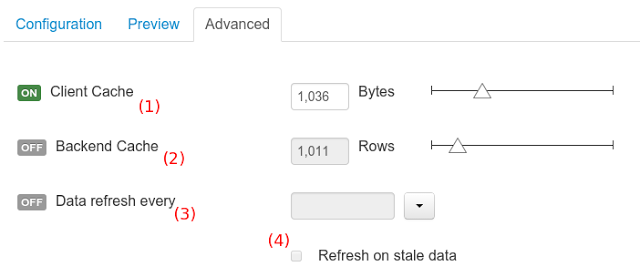
-
(1) To enable or disable the client cache and specify the maximum size (bytes).
-
(2) To enable or disable the backend cache and specify the maximum cache size (number of rows).
-
(3) To enable or disable automatic refresh for the Data set and the refresh period.
-
(4) To enable or disable the refresh on stale data setting.
Let’s dig into more details about the meaning of these settings.
18.6.8.6. Caching
The system provides caching mechanisms out-of-the-box for holding data sets and performing data operations using in-memory strategies. The use of these features brings a lot of advantages, like reducing the network traffic, remote system payload, processing times etc. On the other hand, it’s up to the user to fine tune properly the caching settings to avoid hitting performance issues.
Two cache levels are supported:
-
Client level
-
Backend level
The following diagram shows how caching is involved in any data set operation:
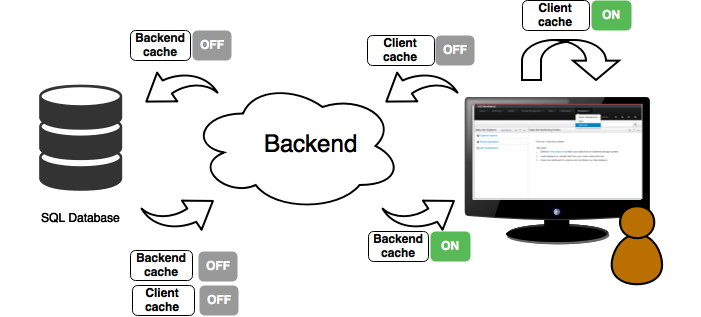
Any data look up call produces a resulting data set, so the use of the caching techniques determines where the data lookup calls are executed and where the resulting data set is located.
Client cache
If ON then the data set involved in a look up operation is pushed into the web browser so that all the components that feed from this data set do not need to perform any requests to the backend since data set operations are resolved at a client side:
-
The data set is stored in the web browser’s memory
-
The client components feed from the data set stored in the browser
-
Data set operations (grouping, aggregations, filters and sort) are processed within the web browser, by means of a Javascript data set operation engine.
If you know beforehand that your data set will remain small, you can enable the client cache. It will reduce the number of backend requests, including the requests to the storage system. On the other hand, if you consider that your data set will be quite big, disable the client cache so as to not hitting with browser issues such as slow performance or intermittent hangs.
Backend cache
Its goal is to provide a caching mechanism for data sets on backend side.
This feature allows to reduce the number of requests to the remote storage system , by holding the data set in memory and performing group, filter and sort operations using the in-memory Drools engine.
It’s useful for data sets that do not change very often and their size can be considered acceptable to be held and processed in memory. It can be also helpful on low latency connectivity issues with the remote storage. On the other hand, if your data set is going to be updated frequently, it’s better to disable the backend cache and perform the requests to the remote storage on each look up request, so the storage system is in charge of resolving the data set lookup request.
BEAN and CSV data providers relies by default on the backend cache, as in both cases the data set must be always loaded into memory in order to resolve any data lookup operation using the in-memory Drools engine. This is the reason why the backend settings are not visible in the Advanced settings tab. |
18.6.8.7. Refresh
The refresh feature allows for the invalidation of any cached data when certain conditions are meet.
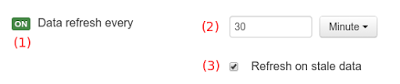
-
(1) To enable or disable the refresh feature.
-
(2) To specify the refresh interval.
-
(3) To enable or disable data set invalidation when the data is outdated.
The data set refresh policy is tightly related to data set caching, detailed in previous section. This invalidation mechanism determines the cache life-cycle.
Depending on the nature of the data there exist three main use cases:
-
Source data changes predictable - Imagine a database being updated every night. In that case, the suggested configuration is to use a "refresh interval = 1 day" and disable "refresh on stale data". That way, the system will always invalidate the cached data set every day. This is the right configuration when we know in advance that the data is going to change.
-
Source data changes unpredictable - On the other hand, if we do not know whether the database is updated every day, the suggested configuration is to use a "refresh interval = 1 day" and enable "refresh on stale data". If so the system, before invalidating any data, will check for modifications. On data modifications, the system will invalidate the current stale data set so that the cache is populated with fresh data on the next data set lookup call.
-
Real time scenarios - In real time scenarios caching makes no sense as data is going to be updated constantly. In this kind of scenarios the data sent to the client has to be constantly updated, so rather than enabling the refresh settings (remember this settings affect the caching, and caching is not enabled) it’s up to the clients consuming the data set to decide when to refresh. When the client is a dashboard then it’s just a matter of modifying the refresh settings in the Displayer Editor configuration screen and set a proper refresh period, "refresh interval = 1 second" for example.
18.6.9. Data Source Management
The data source management system provides the ability of defining data sources for accessing external databases. This data sources can be later used by other Business Central components like the Data Sets.
18.6.9.1. Database Drivers
To be able to communicate with the target database a data source will need a database driver to access it. This is why the system additionally provides the ability of defining database drivers for the data sources operation. A database driver is basically a JDBC compliant driver. We will see them in the next topics.
18.6.9.2. Data Source Authoring Page
Everything related to the authoring of data sources and drivers can be found under the Data Source Authoring page accessible from the following top level menu entry: Extensions>Data Sources, as shown in the following screenshot.
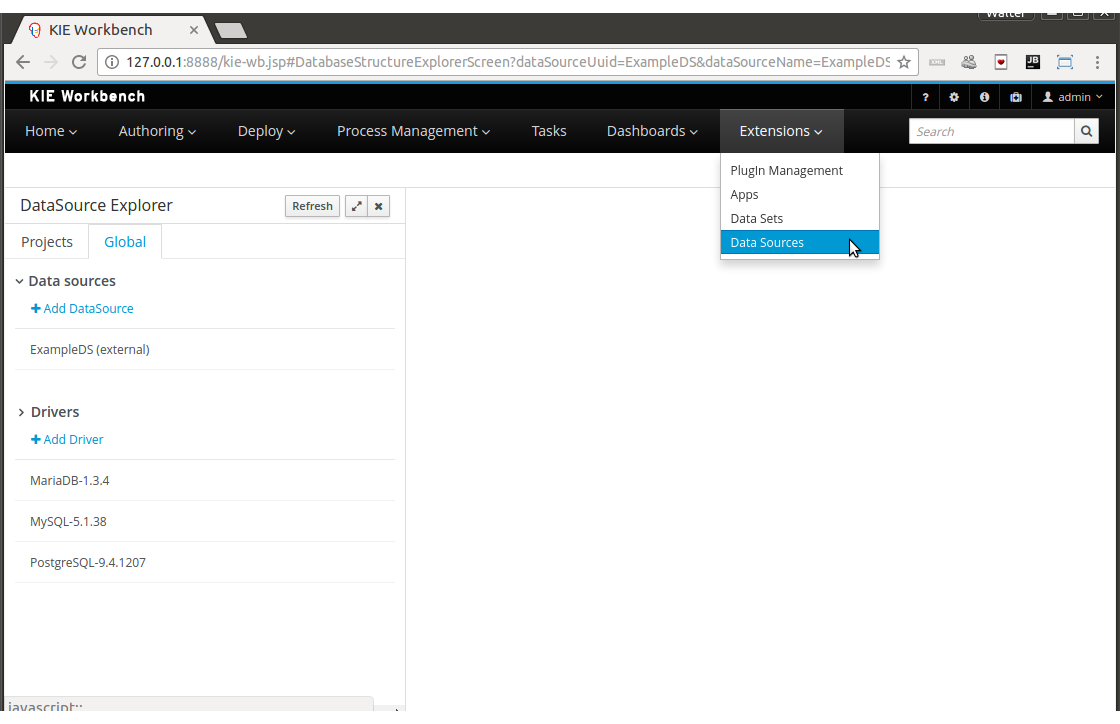
This page is only intended for Administrator users, since defining data sources can be considered a low level task. |
18.6.9.3. Data Source Explorer
The Data Source Explorer lists the data sources and drivers currently defined in the system, at the same time it provides the required actions for managing them.
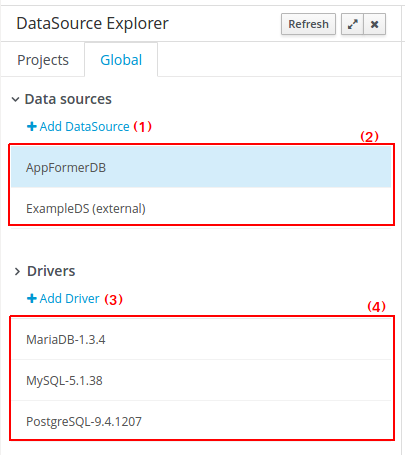
-
(1) Action link for creating a new data source
-
(2) List of currently available data sources
-
(3) Action link for creating a new driver
-
(4) List of currently available drivers
18.6.9.4. New Data Source Wizard
Clicking on the New Data Source action link opens the New Data Source Wizard:
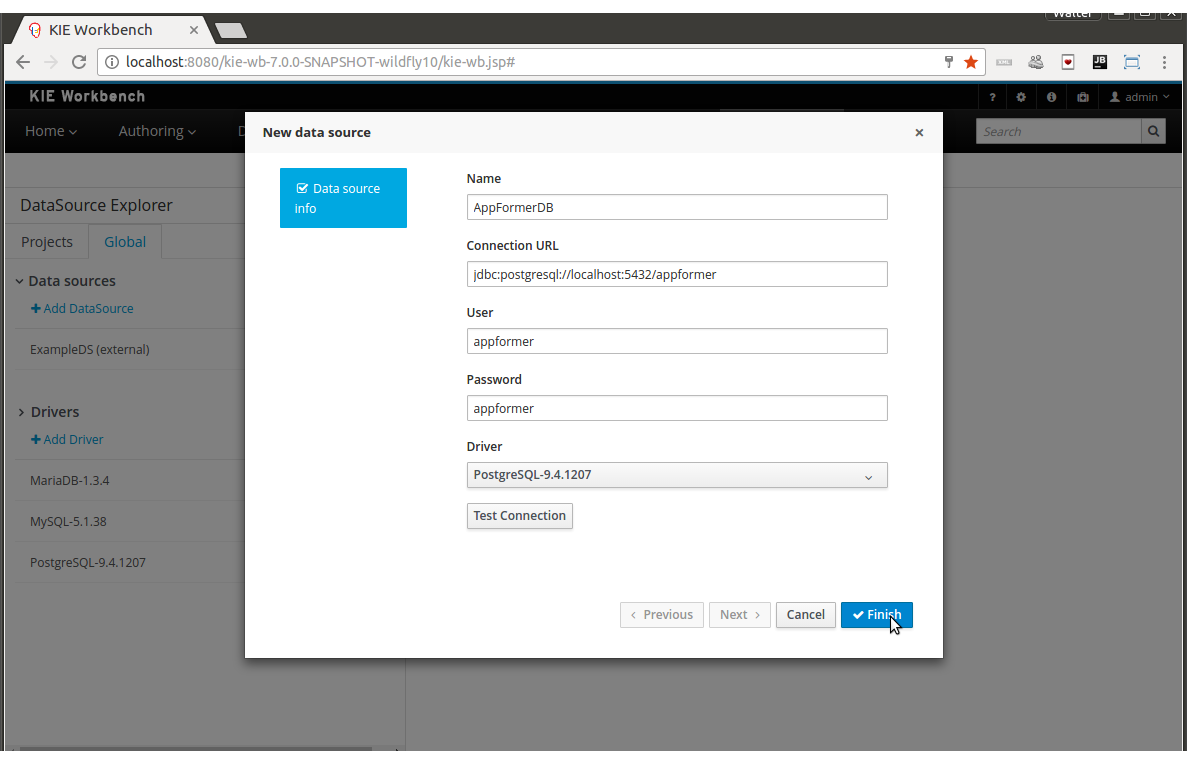
The following required parameters define a data source:
-
Name: A unique name for the data source definition.
-
Connection URL: A JDBC database connection url compliant with the selected driver type. This is an example of a connection url for a PostgreSQL database: jdbc:postgresql://localhost:5432/appformer.
-
User: A user name in the target database.
-
Password: The corresponding user password.
-
Driver: Selects the JDBC driver to be used for connecting to the target database. Note that the connection url format may vary depending on the driver, and different database vendors typically provides different drivers.
-
Test connection: Once clicked, the system will show a dialog similar to the one below showing the connection test status.
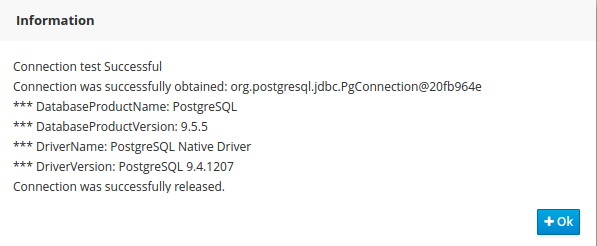
While not required, it’s recommended to use the test connection button to check the correctness of the data source parameters prior to finishing the data source creation. |
18.6.9.5. Data Source Editor
The Data Source Editor is opened by clicking on a data source item in the Data Source Explorer.
The following screenshot shows the Data Source Editor opened for the data source of the example above.
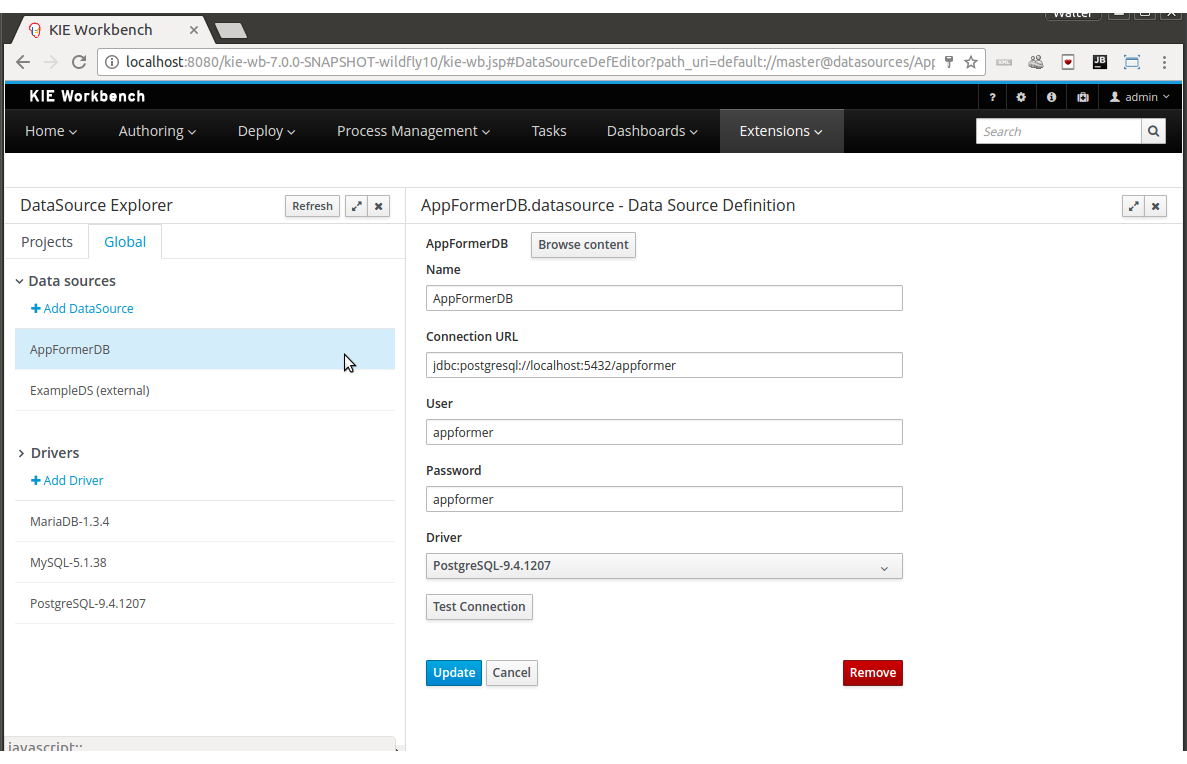
-
Main Panel: The main panel basically lets you modify the data source configuration parameters.
-
Test connection: Tests the connection.
It’s a recommended practice to test the connection prior saving a modified data source. |
18.6.9.6. Data Source Content Browser
The data source content browser is opened by clicking on the Browse Content button, and enables the navigation through the database structure pointed by the data source. The navigation is performed in three levels, Schemas level, Current schema level and Current table level.
-
Schemas level: lists all the database schemas accessible by current data source. Which schemas are listed depends on the database access rights granted to the user which was used in the connection configuration. Similarly for the following item.
-
Current schema level: shows all the database tables for the selected schema.
-
Current table level: shows the table content for the selected table.
The following screenshots show the information shown at each level, for a user that realized the following navigation steps. Selects the "public" schema → Selects the "country" table.
Schema Selection:
Clicking on the Open button opens the Current schema level for the selected schema.
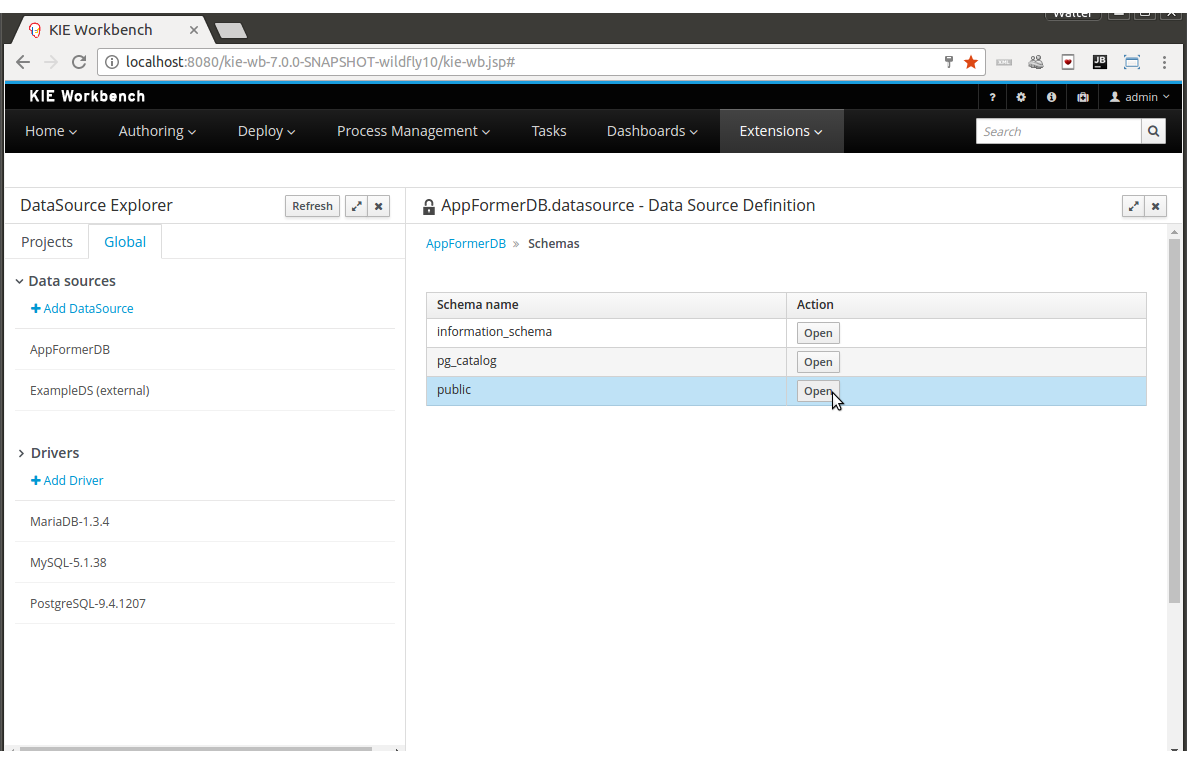
Table Selection:
Clicking on the Open button opens the Current table level for the selected table.
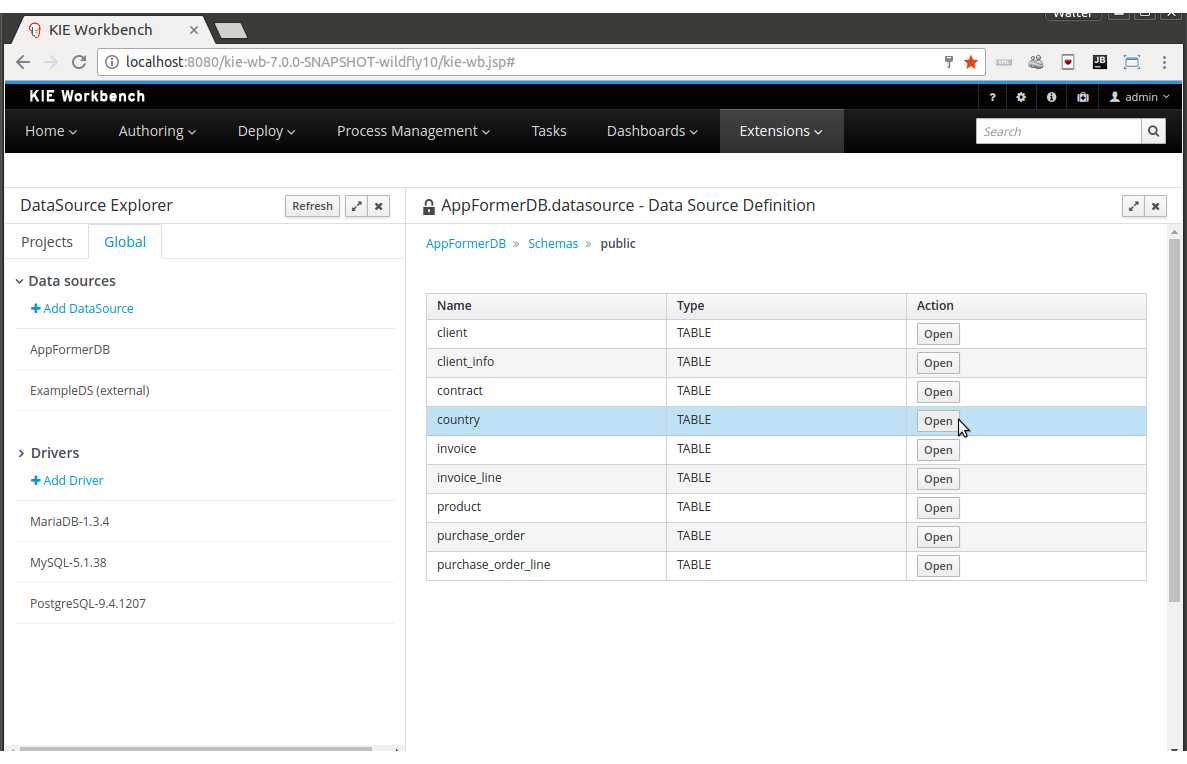
Table information:
The rows for the selected table are shown at this level.
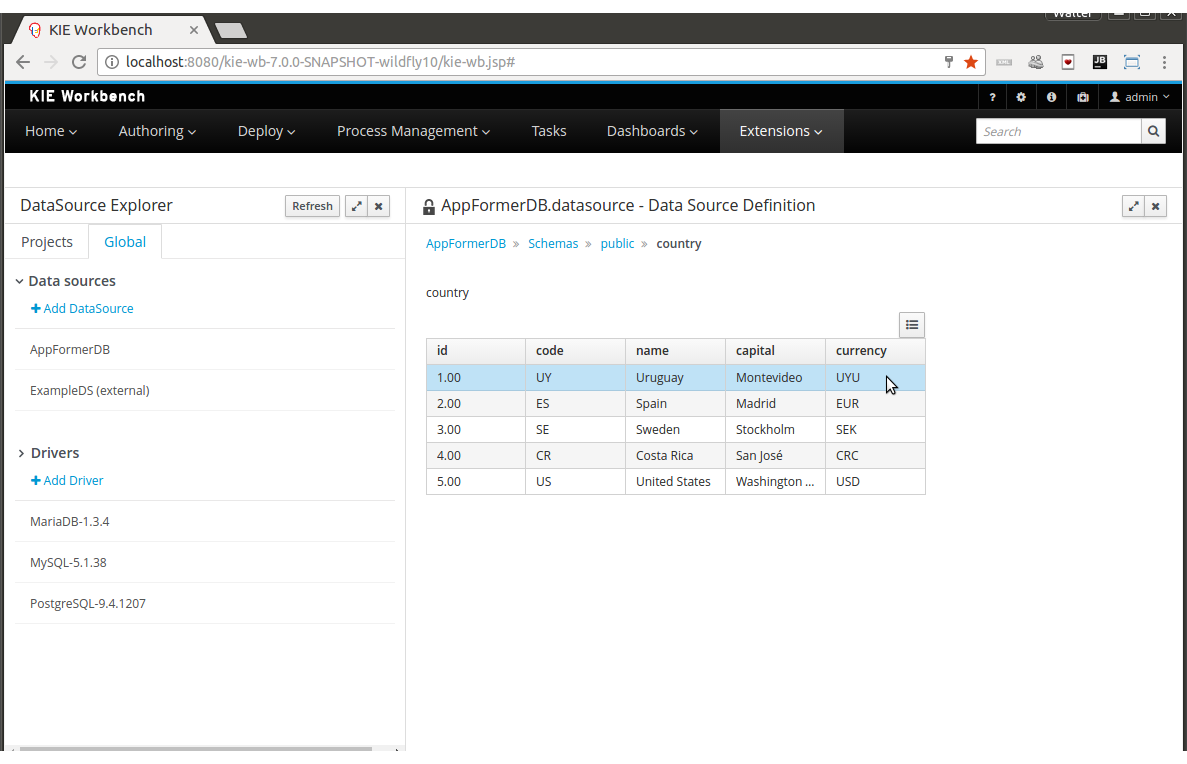
18.6.9.7. External Data Sources
External data sources are typically not defined in Business Central, instead they exist in current container and for some containers like Wildfly 11 or the JBoss EAP 7 servers they can still be listed in read only mode. In this cases only the Data Source Content Browser is enabled.
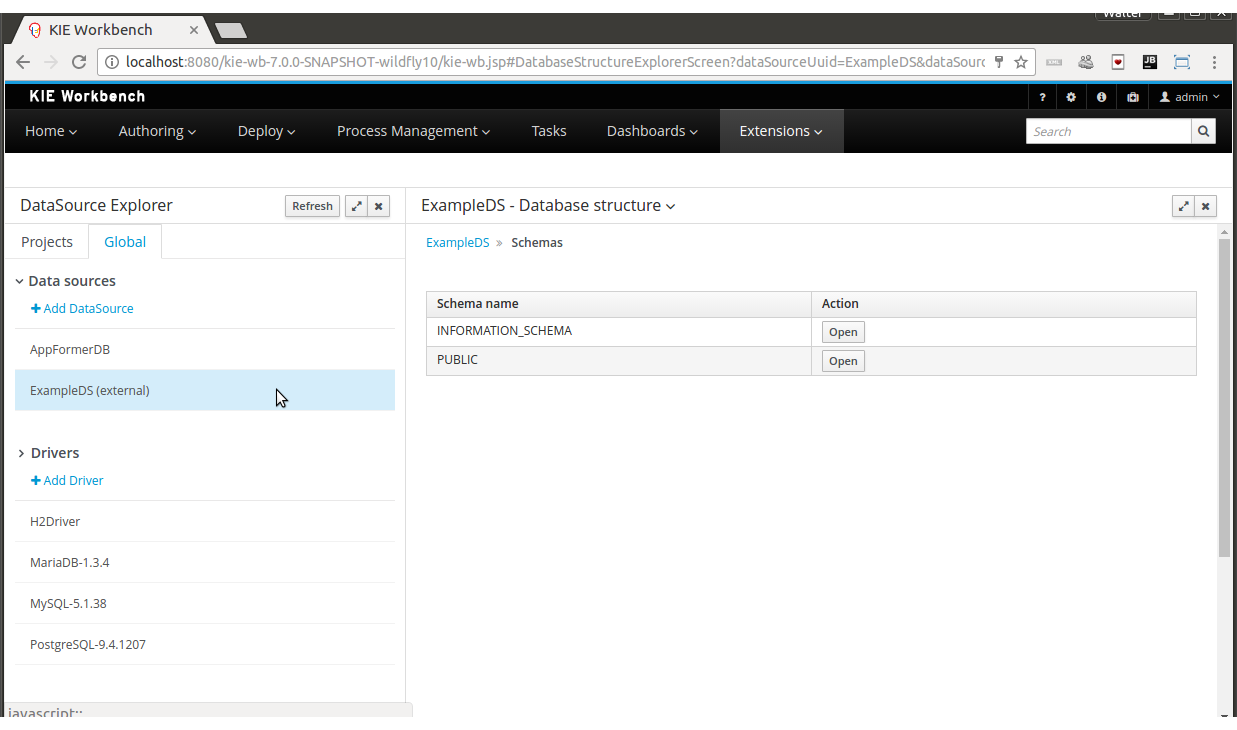
18.6.9.8. New Driver Wizard
Clicking on the New Driver action link opens the New Driver Wizard:
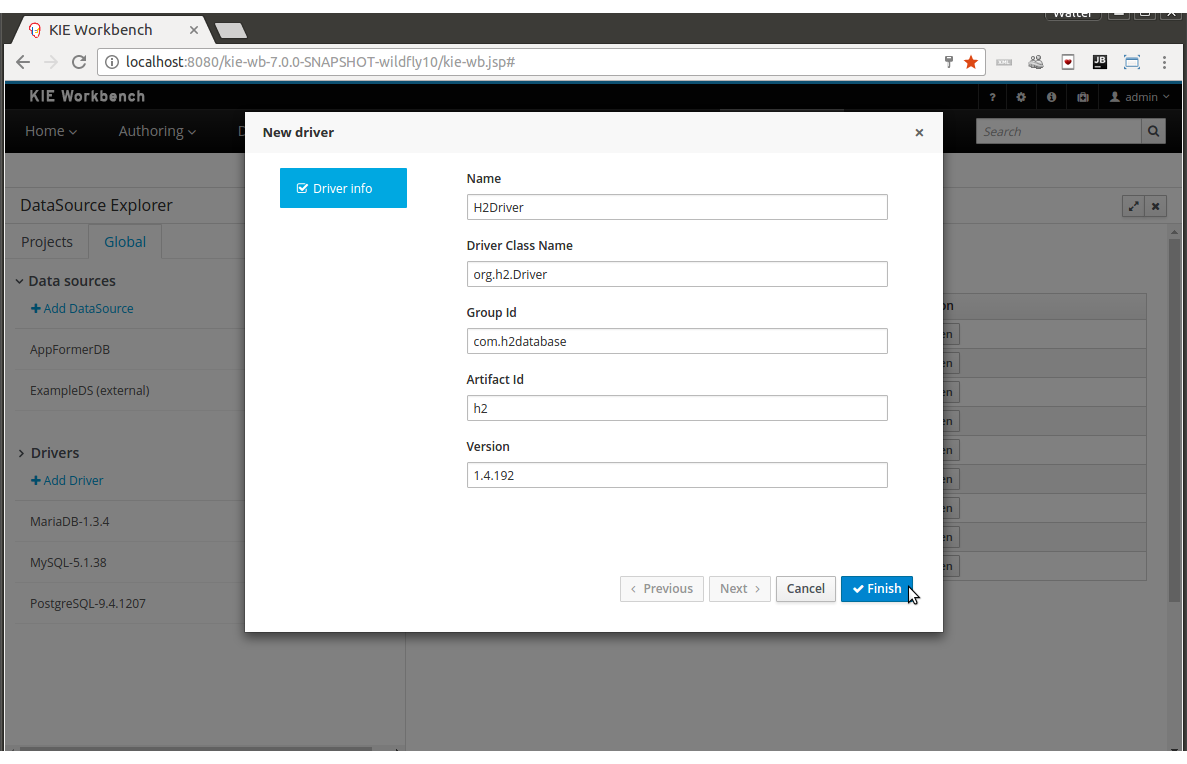
The following required parameters define a Driver:
-
Name: A unique name for the driver definition.
-
Driver Class Name: The java fully qualified name for the class that implements the JDBC driver contract.
-
Group Id: The maven group id for the artifact that contains the JDBC driver implementation.
-
Artifact Id: The maven artifact id for the artifact that contains the JDBC driver implementation.
-
Version: The maven version for the artifact that contains the JDBC driver implementation.
Some commercial database drivers (like Oracle) are not available in the maven central repository. You can use those by first uploading them via Artifact Repository page and then continue with the driver configuration as for the drivers available in the maven central repository. |
18.6.9.9. Driver Editor
The Driver Editor is opened by clicking on a driver item in the Data Source Explorer.
The following screenshot shows the Driver Editor opened for the driver of the example above.
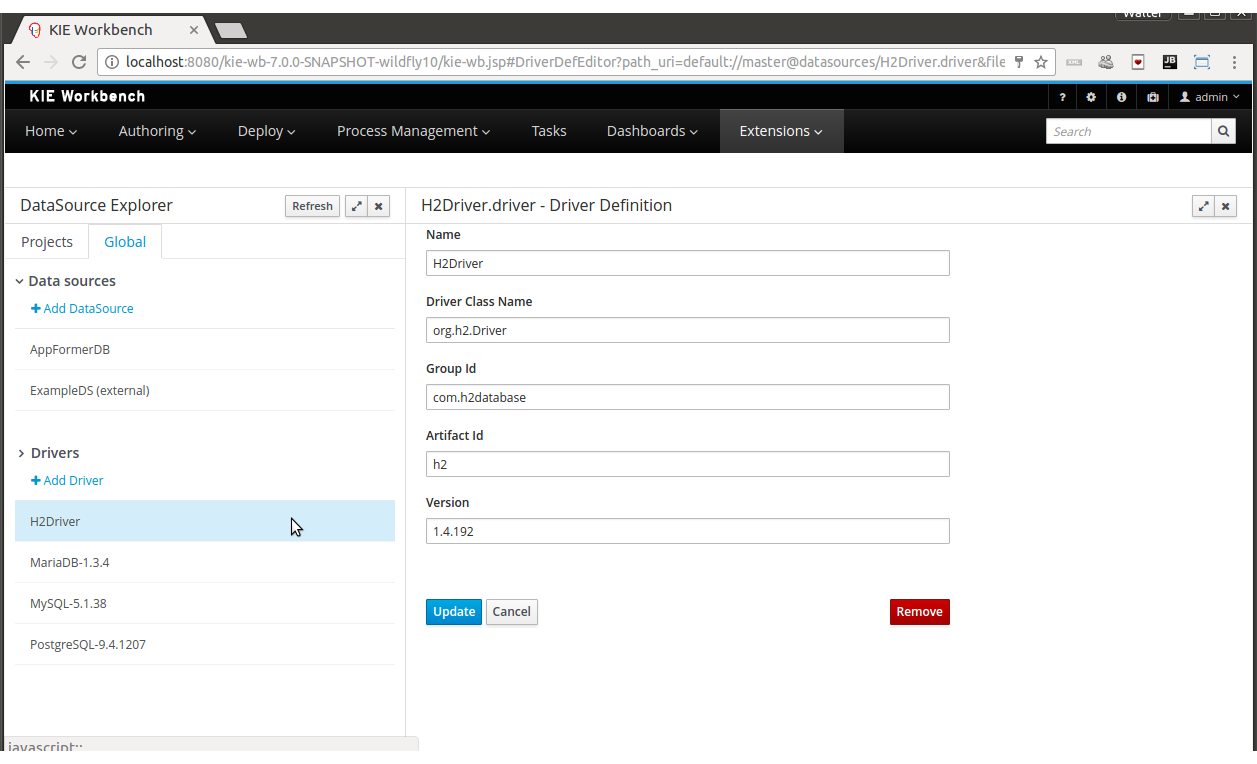
-
Main Panel: The main panel basically lets you modify the driver configuration parameters. See New Driver Wizard.
18.6.9.10. By Default Drivers
The system is shipped with a set of by default configured drivers for the most common used open source databases. And they are aligned with the latest database versions supported by the Wildfly 11 and the JBoss EAP 7 servers.
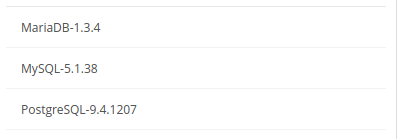
The default drivers initialization can be enabled by setting the datasource.management.disableDefaultDrivers configuration property to false. It can be set by configuring the proper value in the datasource-management.properties file, or by passing the system property -Ddatasource.management.disableDefaultDrivers=false to the JVM. For more information see Advanced Settings. |
18.6.9.11. Advanced Settings
The data source management system advanced settings can be found in the datasource-management.properties file in the WEB-INF/classes directory of the given Business Central distribution file.
The data source management system has the ability of working with two different internal implementations for the data sources and drivers. An implementation based on the Wildfly/EAP native data sources and drivers, and a container independent implementation. Wildfly/EAP Business Central distributions are configured by default for using the native Wildlfy/EAP containers implementations, and Tomcat8 distributions are configured for using the container independent implementations. This latter implementation can also be used for Wildfly/EAP containers.
The valid combinations are:
WildflyDataSourceProvider + WildflyDriverProvider
or
DBCPDataSourceProvider + DBCPDriverProvider
The datasource.management.wildfly.xxxxx properties are only suited for the WildflyXXXProviders.
18.6.9.12. Advanced Settings for Business Central Wildlfy/EAP distributions
Property name | By default value | Description |
---|---|---|
datasource.management.DataSourceProvider |
WildflyDataSourceProvider |
see Advanced Settings. |
datasource.management.DriverProvider |
WildflyDriverProvider |
see Advanced Settings. |
datasource.management.disableDefaultDrivers |
true |
Set to false to enable the default database drivers initialization. |
datasource.management.wildfly.host |
localhost |
Name or ip address used for the Wildlfy server management interface binding. |
datasource.management.wildfly.port |
9990 |
Port used for the Wildlfy server management interface binding. |
datasource.management.wildfly.admin |
Administration user for connecting to the Wildfly server running current Business Central. In general it’s not necessary to set this value but might be needed in cases when the Wildlfy management interface is bound to an address different than localhost. |
|
datasource.management.wildfly.password |
Administration user password for connecting to the Wildfly server running current Business Central. In general it’s not necessary to set this value but might be needed in cases when the Wildlfy management interface is bound to an address different than localhost. |
|
datasource.management.wildfly.realm |
ManagementRealm |
Realm for the administration user authentication. |
datasource.management.wildfly.profile |
The profile name used for starting the Wildfly domain, e.g. default, full, full-ha, etc. This value must only by set when Business Central is running in clustering mode and the hosting Wildfly servers are configured by using domains. Do not set if the Wildlfy servers are running as standalone servers. |
|
datasource.management.wildfly.serverGroup |
The server group to which current Wildfly server instance belongs, e.g. primary-server-group, etc. This value must only by set when Business Central is running in clustering mode and the hosting Wildfly servers are configured by using domains. Do not set if the Wildlfy servers are running as standalone servers. |
|
datasource.management.DefChangeHandler |
This value must only by set when Business Central is running in clustering mode. If the hosting Wildfly servers are configured by using domains the following value must be used DomainModeChangeHandler and the following value StandaloneModeChangeHandler must be used in cases when the hosting Wildlfy servers are running as standalone servers. Clustering installations that uses the DBCPXXXProviders must be configured for using the StandaloneModeChangeHandler. |
The properties above can also be set by passing system properties to the JVM using the Java standard mechanism. e.g. -Ddatasource.management.wildfly.port=1234. Values configured by using this mechanism will override the values configured in the datasource-management.properties file. |
18.6.9.13. Advanced Settings for Tomcat distributions
Property name | By default value | Description |
---|---|---|
datasource.management.DataSourceProvider |
DBCPDataSourceProvider |
This is the only option available for Tomcat 8 distributions, see Advanced Settings. |
datasource.management.DriverProvider |
DBCPDriverProvider |
This is the only option available for Tomcat 8 distributions, see Advanced Settings. |
datasource.management.disableDefaultDrivers |
true |
Set to false to enable the default database drivers initialization. |
datasource.management.DefChangeHandler |
This value must only by set when Business Central is running in clustering mode. Tomcat distributions only support the StandaloneModeChangeHandler value. |
The properties above can also be set by passing system properties to the JVM using the Java standard mechanism. e.g. -Ddatasource.management.wildfly.port=1234. Values configured by using this mechanism will override the values configured in the datasource-management.properties file. |
18.7. Security management
This section describes how administrator users can manage the application’s users, groups and permissions using an intuitive and friendly user interface in order to configure who can access the different resources and features available.
18.7.1. Basic concepts
18.7.1.1. Introduction to Business Central users, groups and roles
The Business Central security domain defines three kind of entities: user, group and role.
The security entities are being registered in the domain by consuming some realm. The realm can be either the application server’s one (Wildfly, EAP, Tomcat) or any other of the supported types, for example, using some Keycloak remote server that performs handles the target realm.
On the other hand, it’s important to notice that each realm provides, or potentially provides, its own capabilities, semantics or structure on the security domain. These kind of differences on the security domain results on inconsistencies between different environments when moving into the Business Central security domain. This way there exist some conventions which are important to understand - how security entities are being declared and how the platform behaves behind that complexity,
The way Business Central integrates the security entities from an external realm corresponds to:
-
User
A user, rather than attributes and some any other kind of metadata, which can be different across domains, represents the same kind entity in any of the supported security environments (Wildfly, EAP, Tomcat, Keybloak, etc), so the entity results in a user on Business Central as well
-
Role / Group
Both role and group are security entities, but rather than a user, the semantics, the behaviors or the structure in the domain is not usually common across environments. As an example consider that exist domains which do not support both, or domains were the semantics for group or role differs. As it results domain specific, the way the application behaves and figures out if an entity should be considered a group or a role, it’s by checking the application’s Role Registry. This way an entity will be considered an role in case it’s identifier is present in the application’s Role Registry, otherwise the entity will be considered as a group.
The Role Registry is an application’s component that provides the set of roles in the Business Central security domain. It’s being populated by consuming the entities (role-name) declared in the security-constraints section on the application’s deployment descriptor (web.xml). See source file org.uberfire.ext.security.server.RolesRegistry. |
It means that depending on the concrete environment’s configuration, some entity can be as a role, on the security environment consumed by Business Central, but it results a group in the Business Central security domain, or vice versa. It depends on the entity’s identifier by checking it it is present in the Role Registry. |
A User can be assigned to multiple roles and groups, but it is mandatory to have at least, a single role assignment for being considered valid in the Business Central security domain. It does not mean, for instance, that the user is able login, or able to consume remote services, because it depends on the concrete role/s assigned and how the roles and permissions are defined the application. |
18.7.1.2. Permissions
A permission is basically something the user can do within the application. Usually, an action related to a specific resource. For instance:
-
View a page
-
Save a project
-
View a repository
-
Delete a dashboard
A permission can be granted or denied and it can be global or resource specific. For instance:
-
Global: “Create new pages”
-
Specific: “View the home page”
As you can see, a permission is a resource + action
pair. In the concrete case of a page we have: read, update, delete and create as the available actions. That means that there are four possible permissions that could be granted for pages.
Permissions do not necessarily need to be tied to a resource. Sometimes it is also neccessary to protect access to specific features, like for instance "generate a sales report". That means, permissions can be used not only to protect access to resources but also to custom features within the application.
18.7.1.3. Authorization policy
The set of permissions assigned to every role and/or group is called the authorization (or security) policy. Every application contains a single security policy which is used every time the system checks a permission.
The authorization policy file is stored in a file called WEB-INF/classes/security-policy.properties under the application’s WAR structure.
If no policy is defined then the authorization management features are disabled and the application behaves as if all the resources & features were granted by default. |
Here is an example of a security policy file:
# Role "admin"
role.admin.permission.perspective.read=true
role.admin.permission.perspective.read.Dashboard=false
# Role "user"
role.user.permission.perspective.read=false
role.user.permission.perspective.read.Home=true
role.user.permission.perspective.read.Dashboard=true
Every entry defines a single permission which is assigned to a role/group. On application start up, the policy file is loaded and stored into memory.
18.7.1.4. Security provider
A security environment is usually provided by the use of a realm. Realms are used to restrict access to the different application’s resources. So realms contains the information about the users, groups, roles, permissions and any other related information.
In most typical scenarios the application’s security is delegated to the container’s security mechanism, which consumes a given realm at same time. It’s important to consider that there exist several realm implementations, for example Wildfly provides a realm based on the application-users.properties/application-roles.properties files, Tomcat provides a realm based on the tomcat-users.xml file, etc. So there is no single security realm to rely on, it can be different in each installation.
Due to the potential different security environments that have to be supported, the security module provides a well defined API with some default built-in security providers. A security provider is the formal name given to a concrete user and group management service implementation for a given realm.
The user & group management features available will depend on the security provider configured. If the built-in providers do not fit with the application’s security realm, it is easy to build and register your own provider.
18.7.2. Installation and setup
At the time of this writing, the application provides two pre-installed security providers:
-
Wildfly 11 / EAP 7 distribution - Both distributions use the Wildfly security provider configured for the use of the default realm files application-users.properties and application-roles.properties
-
Tomcat distribution - It uses the Tomcat security provider configured for the use of the default realm file tomcat-users.xml
Please read each provider’s documentation in order to apply the concrete settings for the target deployment environment.
On the other hand, when either using a custom security provider or using one of the availables, consider the following installation options:
-
Enable the security management feature on an existing WAR distribution
-
Setup and installation in an existing or new project
NOTE: If no security provider is installed, there will be no available user interface for managing the security realm. Once a security provider is installed and setup, the user and group management features are automatically enabled in the security management UI (see the Usage section below).
18.7.2.1. Enabling user & group management
Given an existing WAR distribution, follow these steps in order to install and enable the user & group management features:
-
Ensure the following libraries are present on WEB-INF/lib:
-
WEB-INF/lib/uberfire-security-management-api-?.jar
-
WEB-INF/lib/uberfire-security-management-backend-?.jar
-
-
Copy the security provider library to WEB-INF/lib:
-
Eg: WEB-INF/lib/uberfire-security-management-wildfly-?.jar
-
If the provider requires additional libraries, copy them as well (read each provider’s documentation for more information).
-
-
Replace the whole content of the WEB-INF/classes/security-management.properties file, or if not present, create it. The settings present on this file depend on the concrete implementation used. Please read each provider’s documentation for more information.
-
If deploying on Wildfly or EAP, check if the WEB-INF/jboss-deployment-structure.xml requires any update (read each provider’s documentation for more information).
18.7.2.2. Disabling user & group management
The user & groups management features can be disabled, and thus no services or user interface will be available, by means of either:
-
Uninstalling the security provider from the application
When no concrete security provider is installed, the user and group management features will be disabled and no services or user interface will be displayed to the user. This is the case for instance, in Weblogic and Websphere installations as there is no a security provider implementation available at the time of this writing.
-
Removing or commenting the security management configuration file
Removing or commenting all the lines in the configuration file located at WEB-INF/classes/security-management.properties is another way to disable the user and group management features.
18.7.2.3. Upgrading an existing installation
In versions prior to 7, the only way to grant access to resources like Organizational Units, Repositories or Projects was to indicate which roles were able to access a given instance. Those roles were stored in GIT as part of the instance persistent status. The CLI was the tool used to add/remove roles:
-
remove-role-repo: remove role(s) from repository
-
add-role-org-unit: add role(s) to organizational unit
-
remove-role-org-unit: remove role(s) from organizational unit
-
add-role-project: add role(s) to project
-
remove-role-project: remove role(s) from project
As of version 7, the authorization policy is based on permissions. That means is no longer required to keep a list of roles per resource instance. What is required is to define proper permission entries into the active authorization policy using the security management UI (see the Usage section below).
The commands above are no longer required so they have been removed. Basically, what those commands did is to set what roles were able to read a specific item.
In order to guarantee backward compatibility with versions prior to 7, an automatic migration tool is bundled within the application, which converts the list of roles assigned to any organizational unit, repository or project into read permission entries of the security policy.
This tool is executed when the application start ups for the first time, during the security policy deployment. So existing customers, do not have to worry about it, as they will keep their security settings.
18.7.3. Usage
The Security Management page is available under the Home section in the top menu bar.
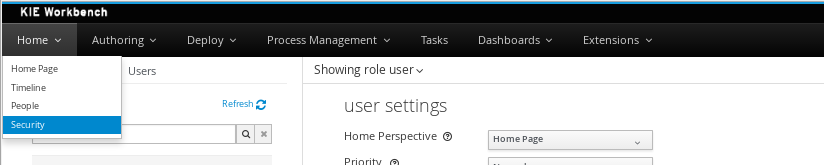
The next screenshot shows how this new page looks:
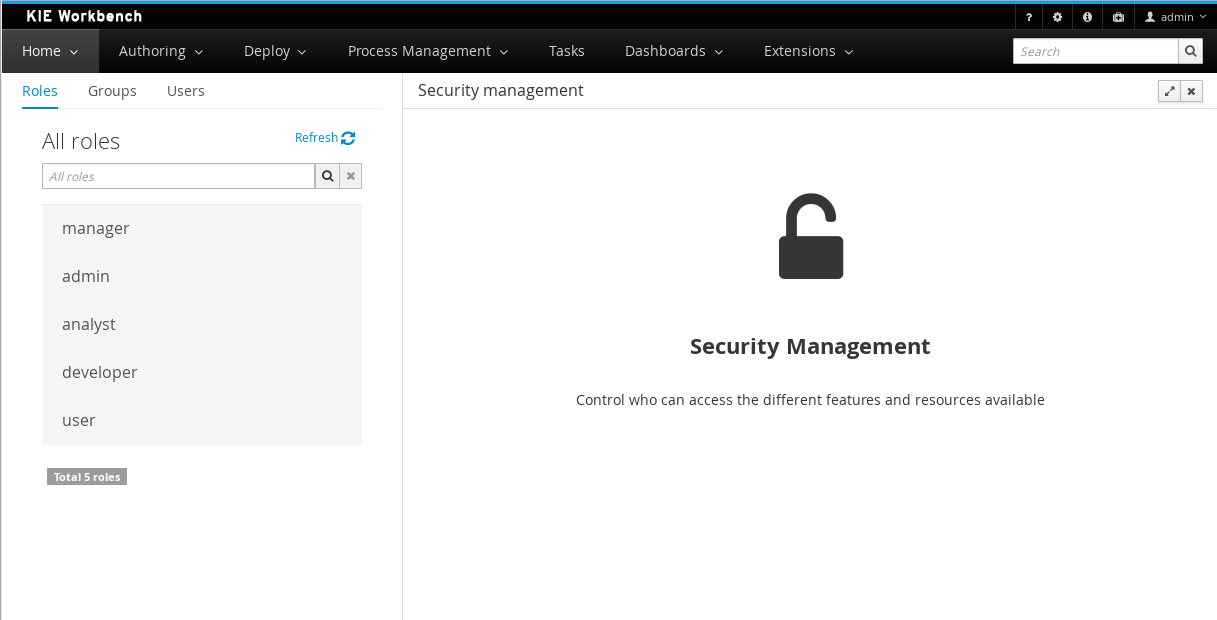
This page supports:
-
List all the roles, groups and users available
-
Create & delete users and groups
-
Edit users, assign roles or groups, and change user properties
-
Edit both roles & groups security settings, which include:
-
The home page a user will be directed to after login
-
The permissions granted or denied to the different Business Central resources and features available
-
All of the above together provides a complete users and groups management subsystem as well as a permission configuration UI for protecting access to specific resources or features.
The next sections provide a deep insight into all these features.
The user and group management related features can be entirely disabled. See the previous section Disabling user & group management. If that’s the case then both the Groups and _Users tabs will remain hidden from the user. |
18.7.3.1. User management
By selecting the Users tab in the left sidebar, the application shows all the users present by default on the application’s security realm:
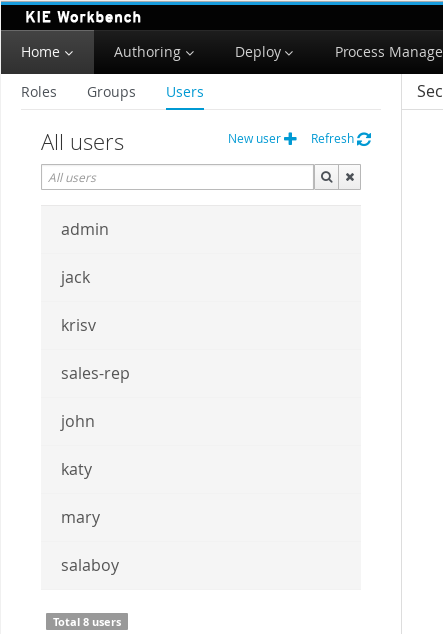
-
Searching for users
In addition to listing all the users, search is also allowed:
+ When specifying the search pattern in the search box the users listed will be reduced to only those that matches the search pattern.
+
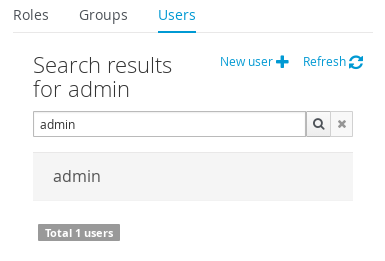
+ Search patterns depend on the concrete security provider being used by the application. Please read each provider’s documentation for more information.
-
Creating new users
By clicking on the "New user +" anchor, a form is displayed on the screen’s right.
This is a wizard like interface where the application asks for the new user name, a password as well as what roles/groups to assign.
-
Editing a user
After clicking on a user in the left sidebar, the user editor is opened on the screen’s right.
For instance, the details screen for the admin user when using the Wildfly security provider looks like the following screenshot:
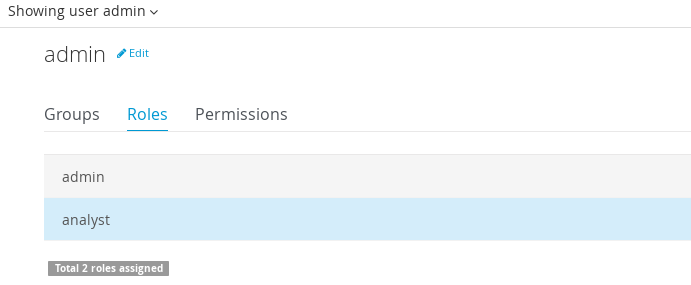
Same screen but when using the Keycloak security provider looks as:
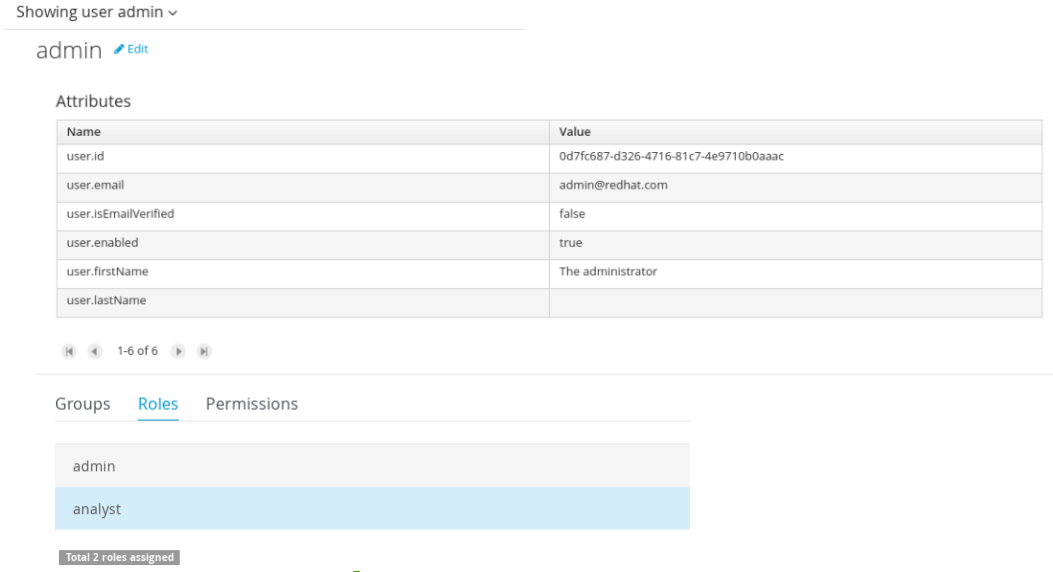
Note that when using the Keycloak provider, a new user attributes section is displayed, but it’s not present when using the Wildfly provider. This is due to the fact that the information and actions available always depend on each provider’s capabilities as explained in the Security provider capabilities section below.
Next is the type of information handled in the user’s details screen:
-
The user name
-
The user’s attributes
-
The assigned groups
-
The assigned roles
-
The permissions granted or denied
In order to update or delete an existing user, click on the Edit button present near to the username in the user editor screen:
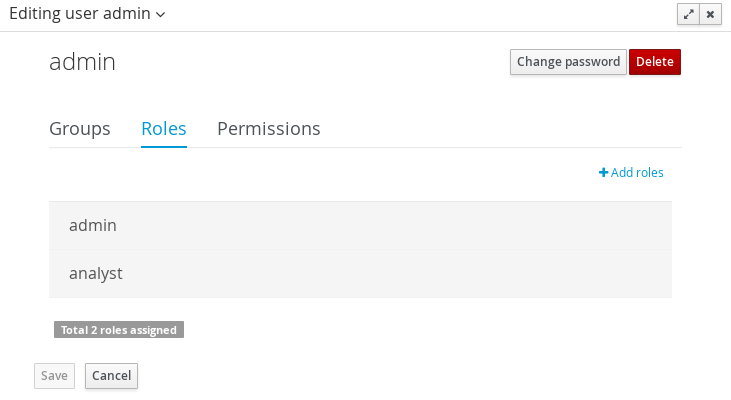
Once the editor is in edit mode, different operations can be done (provided the security provider supports them):
For instance, to modify the set of roles and groups assigned to the user or to change the user’s password as well.
-
Permissions summary
The Permissions tab shows a summary of all the permissions assigned to this particular user. This is a very helpful view as it allows administrator users to verify if a target user has the right permission levels according to the security settings of its roles and groups.
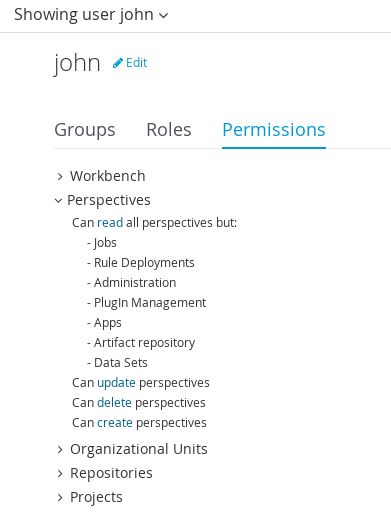
Further details about how to assign permissions to roles and groups are in the Security Settings Editor section below.
-
Updating the user’s attributes
User attributes can added or deleted using the actions available in the attributes table:
-
Updating assigned groups
From the Groups tab, a group selection popup is presented when clicking on the Add to groups button:
This popup screen allows the user to search and select or deselect the groups assigned to the user.
-
Updating assigned roles
From the Roles tab, a role selection popup is presented when clicking on Add to roles button:
This popup screen allows the user to search and select or deselect the roles assigned to the user.
-
Changing the user’s password
A change password popup screen is presented when clicking on the Change password button:
-
Deleting users
The user currently being edited can be deleted from the realm by clicking on the Delete button.
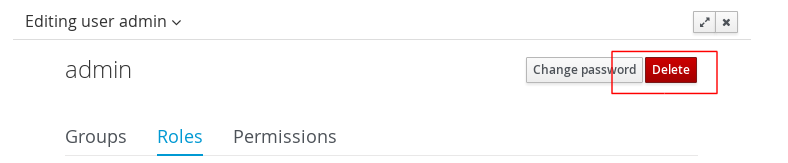
Security provider capabilities
Each security realm can provide support for different operations. For example consider the use of a Wildfly’s realm based on properties files. The contents for the applications-users.properties is like:
admin=207b6e0cc556d7084b5e2db7d822555c
salaboy=d4af256e7007fea2e581d539e05edd1b
maciej=3c8609f5e0c908a8c361ca633ed23844
kris=0bfd0f47d4817f2557c91cbab38bb92d
katy=fd37b5d0b82ce027bfad677a54fbccee
john=afda4373c6021f3f5841cd6c0a027244
jack=984ba30e11dda7b9ed86ba7b73d01481
director=6b7f87a92b62bedd0a5a94c98bd83e21
user=c5568adea472163dfc00c19c6348a665
guest=b5d048a237bfd2874b6928e1f37ee15e
kiewb=78541b7b451d8012223f29ba5141bcc2
kieserver=16c6511893651c9b4b57e0c027a96075
Notice that it’s based on key-value pairs where the key is the username, and the value is the hashed value for the user’s password. So a user is just represented by a key and its username, it does not have a name nor an address or any other meta information.
On the other hand, consider the use of a realm provided by a Keycloak server. The user information is composed by more meta-data, such as the surname, address, etc, as in the following image:
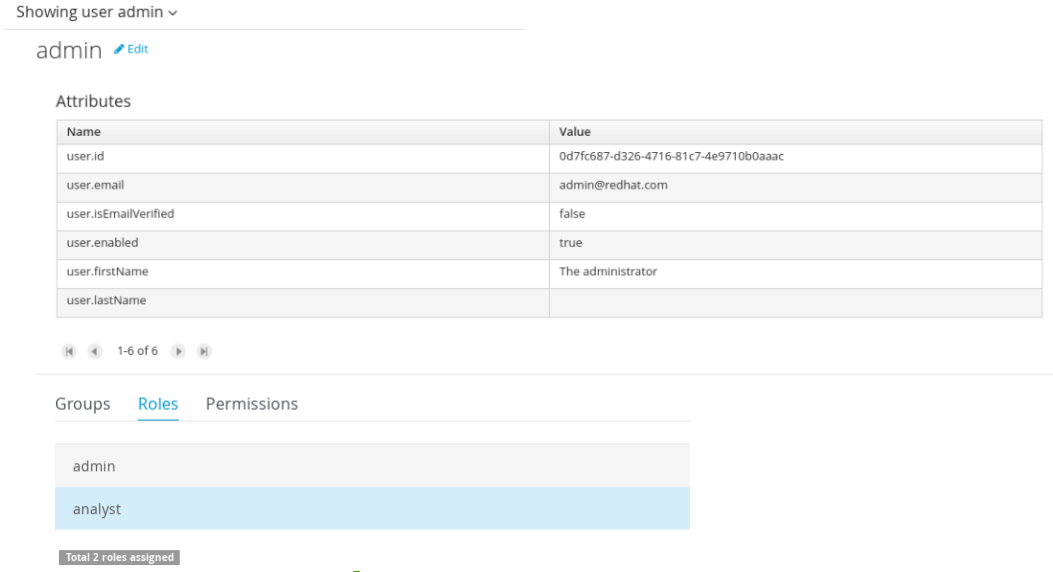
So the different services and client side components from the User and Group Management API are based on capabilities. Capabilities are used to expose or restrict the available functionality provided by the different services and client side components. Examples of capabilities are:
-
Create a user
-
Update a user
-
Delete a user
-
Update user’s attributes
-
Create a group
-
Update a group
-
Assign groups to a user
-
Assign roles to a user
Each security provider must specify a set of capabilities supported. From the previous examples, it is noted that the Wildfly security provider does not support the attributes management capability - the user is only composed by the user name. On the other hand the Keycloak provider does support this capability.
The different views and user interface components rely on the capabilities supported by each provider, so if a capability is not supported by the provider in use, the UI does not provide the views for the management of that capability. As an example, consider that a concrete provider does not support deleting users - the delete user button on the user interface will be not available.
Please take a look at the concrete service provider documentation to check all the supported capabilities for each one, the default ones can be found here.
18.7.3.2. Group management
By selecting the Groups tab in the left sidebar, the application shows all the groups present by default on the application’s security realm:
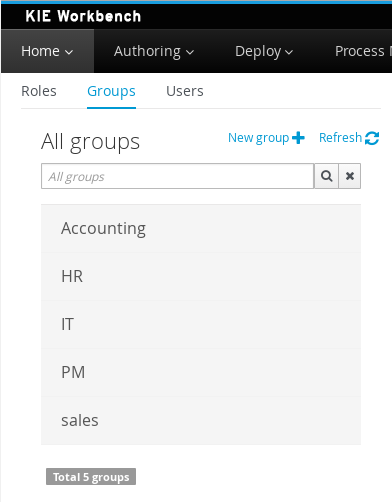
-
Searching for groups
In addition to listing all the groups, search is also allowed:
+ When specifying the search pattern in the search box the groups listed will be reduced to only those that matches the search pattern.
+
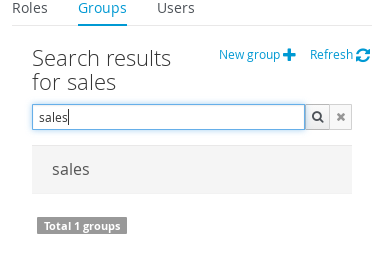
+ Search patterns depend on the concrete security provider being used by the application. Please read each provider’s documentation for more information.
-
Creating new groups
By clicking on the "New group +" anchor, a new screen will be presented on the center panel to perform a new group creation.
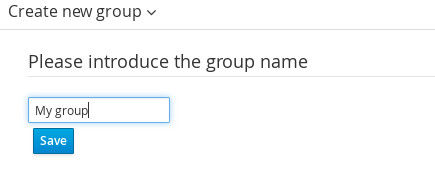
After typing a name anc clicking Save, the next step is to assign users to it:
+
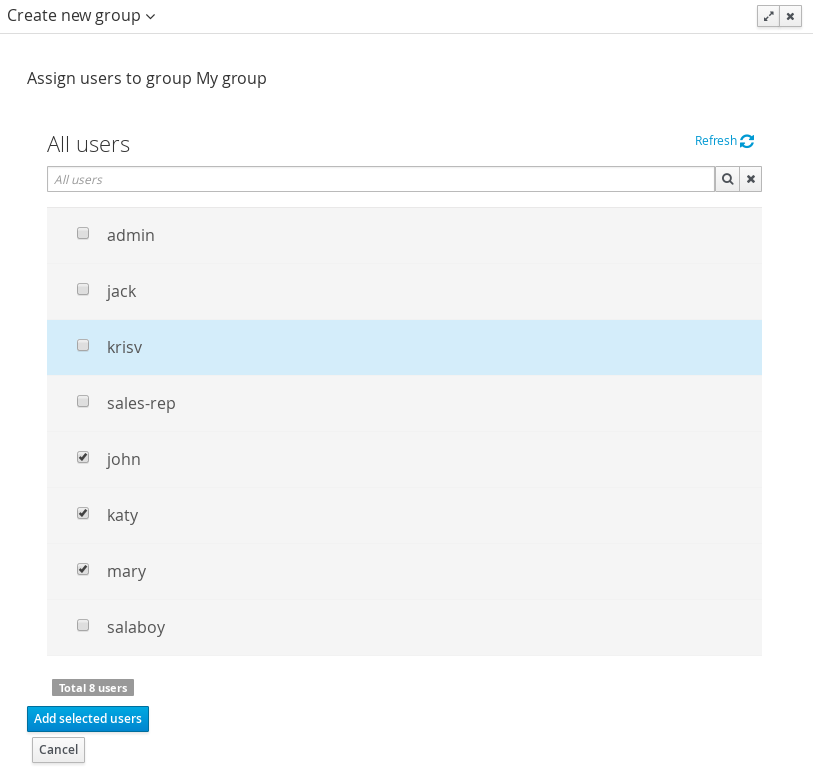
+ Clicking on the "Add selected users" button finishes the group creation.
-
Modifying a group
After clicking on a group in the left sidebar, the security settings editor for the selected group instance is opened on the screen’s right. Further details at the Security Settings Editor section.
-
Deleting groups
To delete an existing group just click on the Delete button.
18.7.3.3. Role management
By selecting the Roles tab in the left sidebar, the application shows all the application roles:
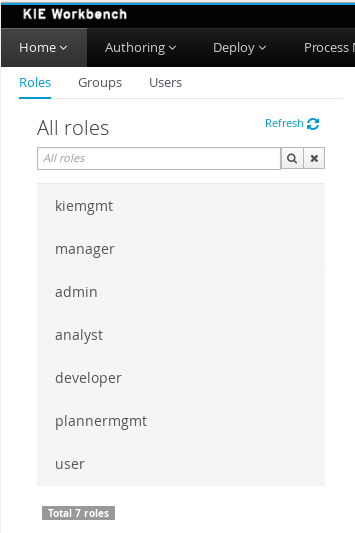
Unlike users and groups, roles can not be created nor deleted as they come from the application’s web.xml descriptor. After clicking on a role in the left sidebar, the role editor is opened on the screen’s right, which is exactly the same security settings editor used for groups. Further details at the Security Settings Editor section.
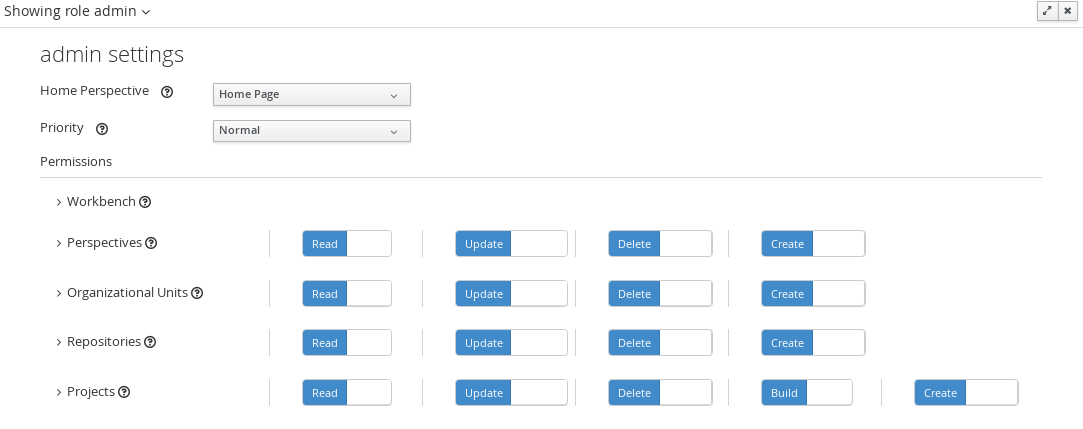
That means both role and group based permissions can be defined. The main diference between roles and group are:
-
Roles are an application defined resource. They are defined as <security-role> entries in the application’s web.xml descriptor.
-
Groups are dynamic and can be defined at runtime. The installed security provider determines where groups instances are stored.
They can be used together without any trouble. Groups are recommended though as they are a more flexible than roles.
-
Searching for roles
In addition to listing all the roles, search is also allowed:
+ When specifying the search pattern in the search box the roles listed will be reduced to only those that matches the search pattern.
+
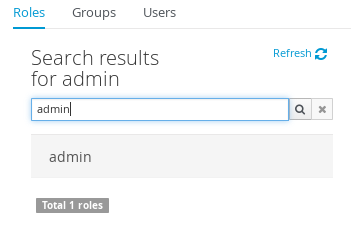
+ Search patterns depend on the concrete security provider being used by the application. Please read each provider’s documentation for more information.
18.7.4. Security Settings Editor
This editor is used to set several security settings for both roles and groups.
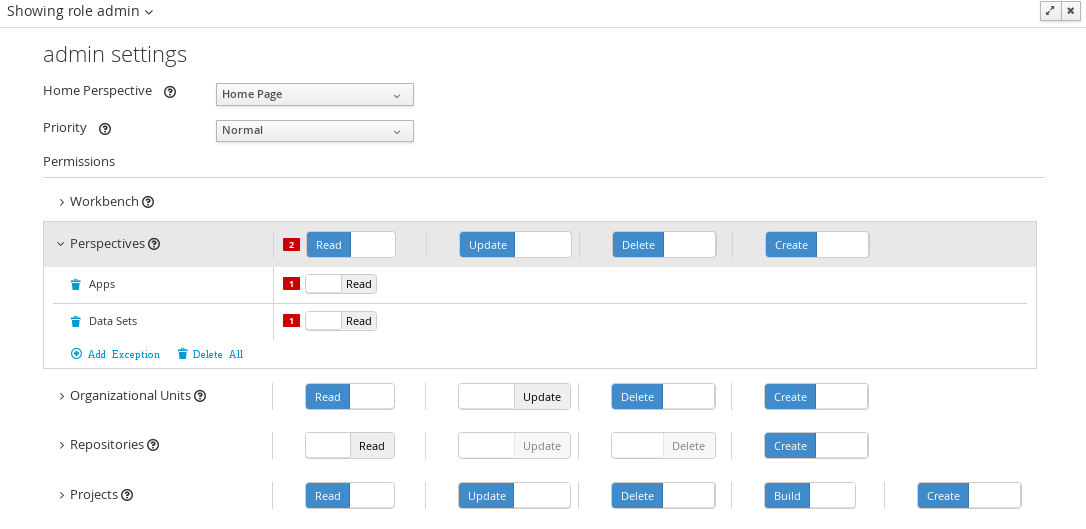
+
18.7.4.1. Home page
This is the page where the user is directed after login. This makes it possible to have different home pages for different users, since users can be assigned to different roles or groups.
18.7.4.2. Priority
It is used to determine what settings (home page, permissions, …) have precedence for those users with more that one role or group assigned.
Without this setting, it won’t be possible to determine what role/group should take precedence. For instance, an administrative role has higher priority than a non-administrative one. For users with both administrative and non-administrative roles granted, administrative privileges will always win, provided the administrative role’s priority is greater than the other.
18.7.4.3. Permissions
Currently, Business Central support the following permission categories.
-
Business Central: General Business Central permissions, not tied to any specific resource type.
-
Pages: If access to a page is denied then it will not be shown in any of application menus. Update, Delete and Create permissions change the behaviour of the page management plugin editor.
-
Organizational Units: Sets who can Create, Update or Delete organizational units from the Organizational Unit section at the Administration page. Sets also what organizational units are visible in the Project Explorer at the Project Authoring page.
-
Repositories: Sets who can Create, Update or Delete repositories from the Repositories section at the Administration page. Sets also what repositories are visible in the Project Explorer at the Project Authoring page.
-
Projects: In the Project Authoring page, sets who can Create, Update, Delete or Build projects from the Project Editor screen as well as what projects are visible in the Project Explorer.
For pages, organizational units, repositories and projects it is possible to define global permissions and add single instance exceptions afterwards. For instance, Read access can be granted to all the pages and deny access just to an individual page. This is called the grant all deny a few strategy.

The opposite, deny all grant a few strategy is also supported:

In the example above, the Update and Delete permissions are disabled as it does not makes sense to define such permissions if the user is not even able to read pages. |
18.7.5. Security Policy Storage
The security policy is stored under the Business Central VFS. Most concrete, in a GIT repo called “security”. The ACL table is stored in a file called “security-policy.properties” under the “authz” directory. Next is an example of the entries this file contains:
role.admin.home=HomePage
role.admin.priority=0
role.admin.permission.perspective.read=true
role.admin.permission.perspective.create=true
role.admin.permission.perspective.delete=true
role.admin.permission.perspective.update=true
Every time the ACL is modified from the security settings UI the changes are stored into the GIT repo.
Initially, when the application is deployed for the first time there is no security policy stored in GIT. However, the application might need to set-up a default policy with the different access profiles for each of the application roles.
In order to support default policies the system allows for declaring a security policy as part of the webapp’s content. This can be done just by placing a security-policy.properties file under the webapp’s resource classpath (the WEB-INF/classes directory inside the WAR archive is a valid one). On app start-up the following steps are executed:
-
Check if an active policy is already stored in GIT
-
If not, then check if a policy has been defined under the webapp’s classpath
-
If found, such policy is stored under GIT
The above is an auto-deploy mechanism which is used in Business Central to set-up its default security policy.
One slight variation of the deployment process is the ability to split the “security-policy.properties” file into small pieces so that it is possible, for example, to define one file per role. The split files must start by the “security-module-” prefix, for instance: “security-module-admin.properties”. The deployment mechanism will read and deploy both the "security-policy.properties" and all the optional “security-module-?.properties” found on the classpath.
Notice, despite using the split approach, the “security-policy.properties” must always be present as it is used as a marker file by the security subsystem in order to locate the other policy files. This split mechanism allows for a better organization of the whole security policy.
18.8. SSH keystore
This section provides an overview of the Business Central SSH keystore and includes a guide for platform users. It explains how to use the Business Central SSH keystore to register and use it’s SSH public keys.
18.8.1. Introduction
Business Central includes an SSH keystore service to provide proper SSH authentication for users.
It provides a configurable default SSH keystore, extensible APIs to allow custom implementations, support for multiple SSH public keys formats, and a new UI available on the Admin page to enable users to register their SSH public keys.
18.8.1.1. The default SSH keystore
The default SSH keystore included with Business Central provides a file-based storage mechanism to store users' SSH public keys.
By default, it uses Business Central .security
folder as a root path. It is possible to use a custom storage path
by setting the appformer.ssh.keys.storage.folder
property to direct to a different folder.
The SSH public keys are stored in the {securityFolderPath}/pkeys/{userName}/
folder structure.
Each SSH public key consists of a pair of files in the storage folder:
-
{keyId}.pub: a file containing the SSH public key content. The file name determines the logic key ID on the system, so do not modify the file name during runtime. For example
ssh-rsa AAAAB3NzaC1yc2EAAAADAQABAAACAQDmak4Wu23RZ6XmN94bOsqecZxuTa4RRhhQmHmTZjMB7HM57/90u/B/gB/GhsPEu1nAXL0npY56tT/MPQ8vRm2C2W9A7CzN5+z5yyL3W01YZy3kzslk77CjULjfhrcfQSL3b2sPG5jv5E5/nyC/swSytucwT/PE7aXTS9H6cHIKUdYPzIt94SHoBxWRIK7PJi9d+eLB+hmDzvbVa1ezu5a8yu2kcHi6NxxfI5iRj2rsceDTp0imC1jMoC6ZDfBvZSxL9FXTMwFdNnmTlJveBtv9nAbnAvIWlilS0VOkdj1s3GxBxeZYAcKbcsK9sJzusptk5dxGsG2Z8vInaglN6OaOQ7b7tcomzCYYwviGQ9gRX8sGsVrw39gsDIGYP2tA4bRr7ecHnlNg1b0HCchA5+QCDk4Hbz1UrnHmPA2Lg9c3WGm2qedvQdVJXuS3mlwYOqL40aXPs6890PvFJUlpiVSznF50djPnwsMxJZEf1HdTXgZD1Bh54ogZf7czyUNfkNkE69yJDbTHjpQd0cKUQnu9tVxqmBzhX31yF4VcsMeADcf2Z8wlA3n4LZnC/GwonYlq5+G93zJpFOkPhme8c2XuPuCXF795lsxyJ8SB/AlwPJAhEtm0y0s0l1l4eWqxsDxkBOgN+ivU0czrVMssHJEJb4o0FLf7iHhOW56/iMdD9w== userName
-
.{keyId}.pub.meta: a file containing the key metadata in JSON format. If a key has no metadata, a new metadata file is dynamically generated. For example:
{ "name":"Key", "creationDate":"Oct 10, 2018 10:10:50 PM", "lastTimeUsed":"Oct 11, 2018 12:11:23 PM" }
18.8.1.2. Using a custom SSH keystore
It is possible to extend and customize the platform default SSH keystore to meet more specific requirements.
Use the system property appformer.ssh.keystore
to specify the Java class name of the service to use. If the property
does not exist or it contains a wrong value, the default SSH keystore is loaded.
To create a custom implementation of the SSH keystore, your Java Class must implement the class |
18.8.2. Using the SSH keystore
This section describes how to use the SSH keystore to register your own keys and how to use them.
18.8.2.1. The SSH keystore UI
The SSH keystore provides an intuitive UI to enable users to manage their SSH public keys on the system. It is accessible from the Admin page by using the SSH Keys menu option.
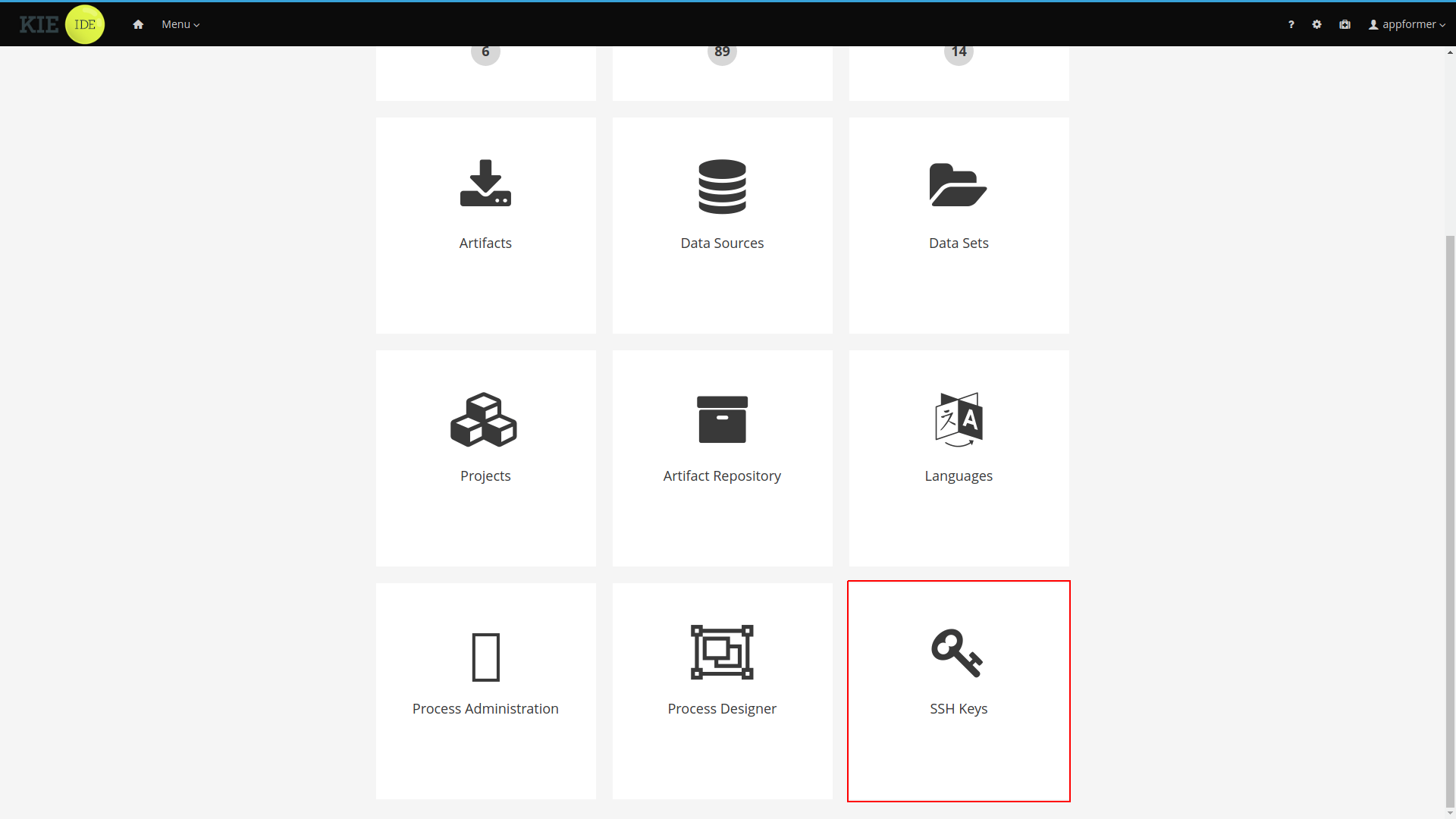
After you click the SSH Keys menu option the SSH Keys Editor will open. the editor displays a table showing the user SSH public keys and provides access to the main action buttons.
-
Add SSH Key: Used to add an SSH public key for the user.
Figure 251. Adding new SSH public key -
Delete SSH Key: Used to remove an existing SSH public key
Figure 252. Deleting a SSH public key
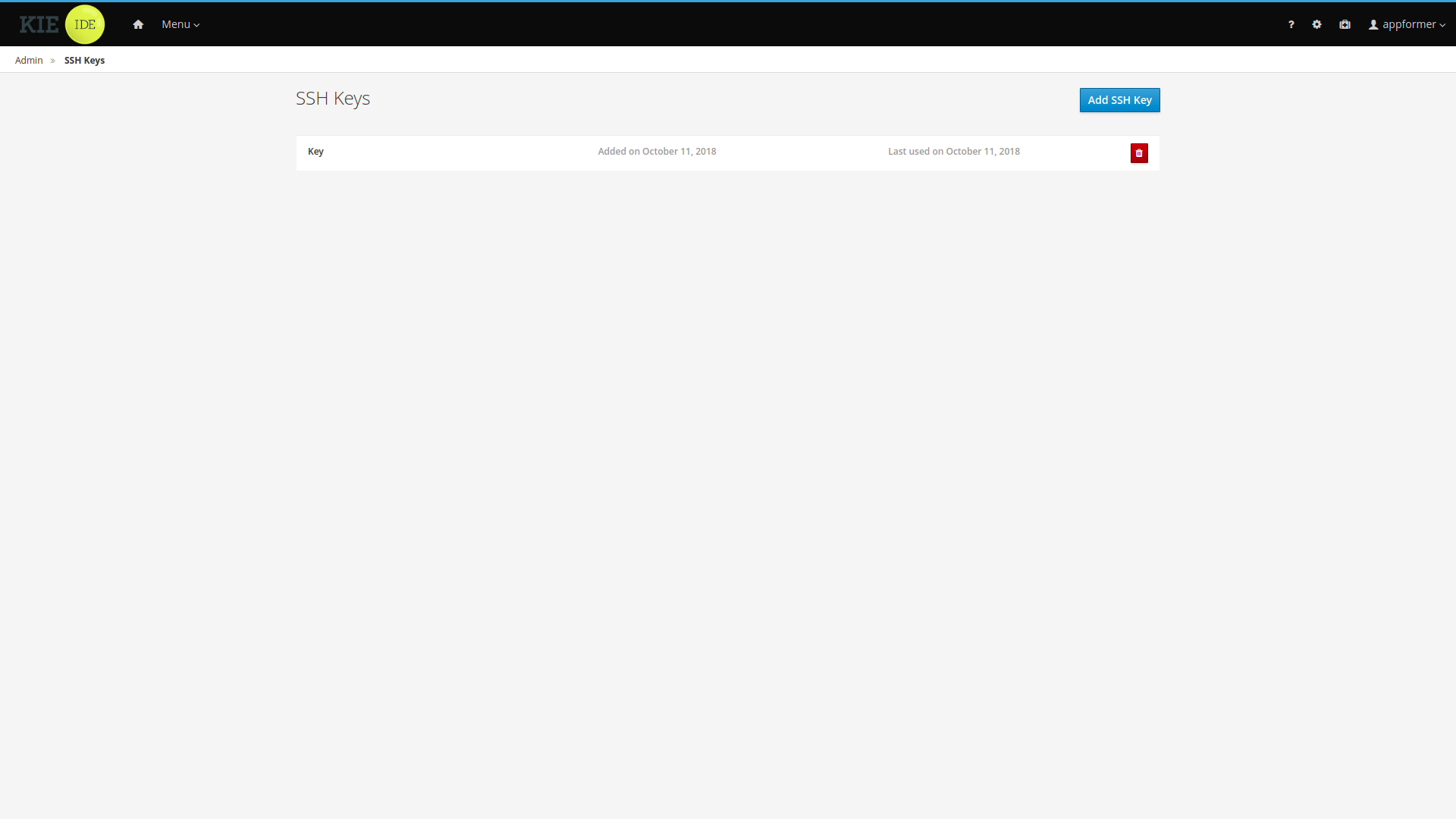
18.8.2.2. Adding SSH keys
This section explains step by step how to add an SSH public key to the SSH keystore.
Creating the SSH key on your computer
-
Open a terminal on your computer
-
Run the
ssh-keygen
command to create the key:ssh-keygen -t rsa -b 4096 -C "<your_user_login_here>"
The SSH key formats supported by the keystore are 'ssh-rsa', 'ssh-dss', 'ecdsa-sha2-nistp256', 'ecdsa-sha2-nistp384' and 'ecdsa-sha2-nistp521'.
-
When prompted, press Enter and accept the default key file location.
Enter a file in which to save the key (/home/<your_login_here>/.ssh/id_rsa): [Press enter]
-
When prompted, enter the pass phrase that you want to use.
Enter passphrase (empty for no passphrase): [Type a passphrase] Enter same passphrase again: [Type passphrase again]
-
Start the
ssh-agent
:eval "$(ssh-agent -s)" Agent pid <any-number-here>
-
Add the new SSH private key to the
ssh-agent
. If you used a different key name, replaceid_rsa
with your key namessh-add ~/.ssh/id_rsa
Registering your SSH public key with the SSH keystore
-
In Business Central, go to the gear icon next to your login to open the Admin page.
Figure 254. Accessing the Admin Page -
Open the SSH keystore UI by clicking the SSH Keys menu option.
Figure 255. SSH Keys Menu Option on Admin PageFigure 256. SSH KeysStore UI Without keys -
Copy the contents of your SSH Public key onto the clipboard. Use the
cat
command to display your key content. If you used a different key name: replaceid_rsa
with your key name, and copy it.cat ~/.ssh/id_rsa.pub
-
In the SSH keystore UI press the Add SSH Key button to open the New SSH public key form. Specify a name, copy the key content into the key field and click Add SSH Key to register the key.
Figure 257. Adding new SSH public key-
Name field cannot be empty, this field defines a meaningful name for the user to identify the key on the SSH public keys table.
-
Key must be a valid SSH Public key, so it cannot be empty and the key format must be supported by the platform.
-
18.9. Embedding Business Central in Your Application
Apart from the individual perspectives (such as the Library or Content Management), Business Central provides a number of editors used for designing and managing assets in different formats. Within Business Central, each asset type has a corresponding editor.
Business Central provides the possibility to embed the perspectives and editors in the user’s application using the standalone mode. Without actually switching to Business Central, it is possible to display perspectives and edit various assets, such as rules, processes, or decision tables, in separate applications.
To embed a part of Business Central in an application, Business Central must be deployed and running on a web server or an application server. Then, in your application, include an HTML inline frame with the proper HTTP query parameters as described in the following table.
Parameter | Values | Description |
---|---|---|
|
none |
This parameter must be included in each URL of a perspective or an editor that will be used in the standalone mode. |
|
|
Used for specifying the perspective to be displayed. |
|
|
Displays the breadcrumbs at the top of the page that can be used for navigating to the lists of spaces and projects within the Library. This parameter can be used only if |
|
|
Specifies the path to the asset to be opened in a corresponding editor. The path must be specified in the format |
URL | Description |
---|---|
http://localhost:8080/business-central/kie-wb.jsp?standalone&perspective=LibraryPerspective |
Opens the Library where it is possible to select a project to be managed. |
Opens the Library with the list of projects. The |
|
Opens the editor of the specified asset. |
|
http://localhost:8080/business-central/kie-wb.jsp?standalone&perspective=ContentManagerPerspective |
Opens the Content Management perspective, where it is possible to create and manage custom pages. |
http://localhost:8080/business-central/kie-wb.jsp?standalone&perspective=MyCustomPage |
Opens the specified custom page that has been created before using the Content Management perspective. The value of the |
18.10. Execution Server Management UI
The Execution Server Management UI allows users create and modify Server Templates and Containers, it also allows users manage Remote Servers. This screen is available via Deploy → Rule Deployments menu.
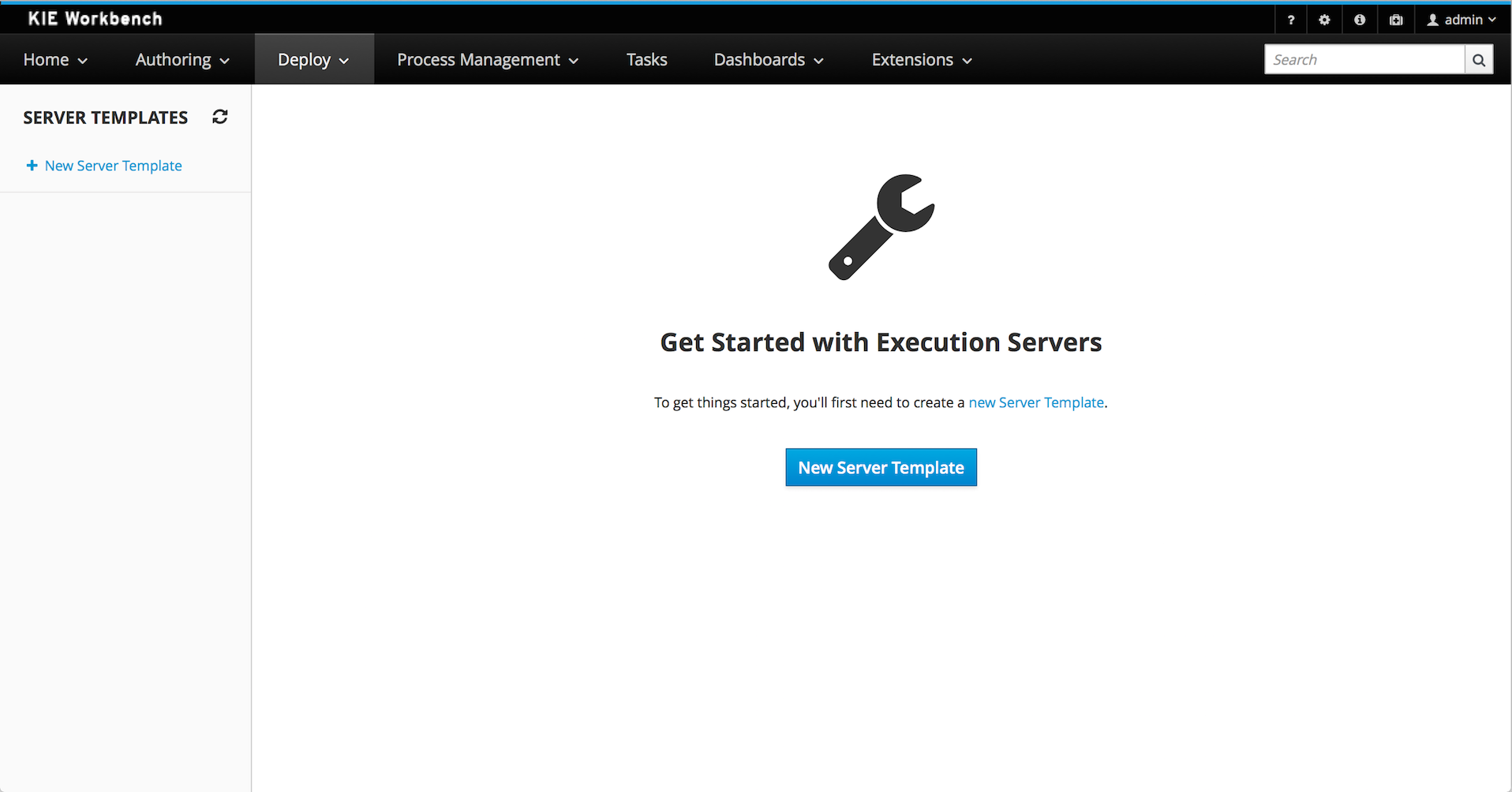
The management UI is only available for KIE Managed Servers. |
18.10.1. Server Templates
Server templates are used to define a common configuration that can be used for multiple server, thus the name: Template.
Server Templates can be created directly from the management UI or it’s automatically create when a server connects to Drools controller and there isn’t a template definition for that remote server. Server templates may have one or more capabilities, such capabilities can’t be modified, if you need modify the capabilities you’ll have to create a new template. Here is the list of current capabilities:
-
Rule (Drools)
-
Process (jBPM)
-
Planning (Optaplanner)
For Planner capability it’s mandatory to enable Rule’s capability too. |
In order to create a new Server Template you have to click at New Server Template button and follow the wizard. It’s also possible to create a container during Wizard, but for now let’s limit to just the template.
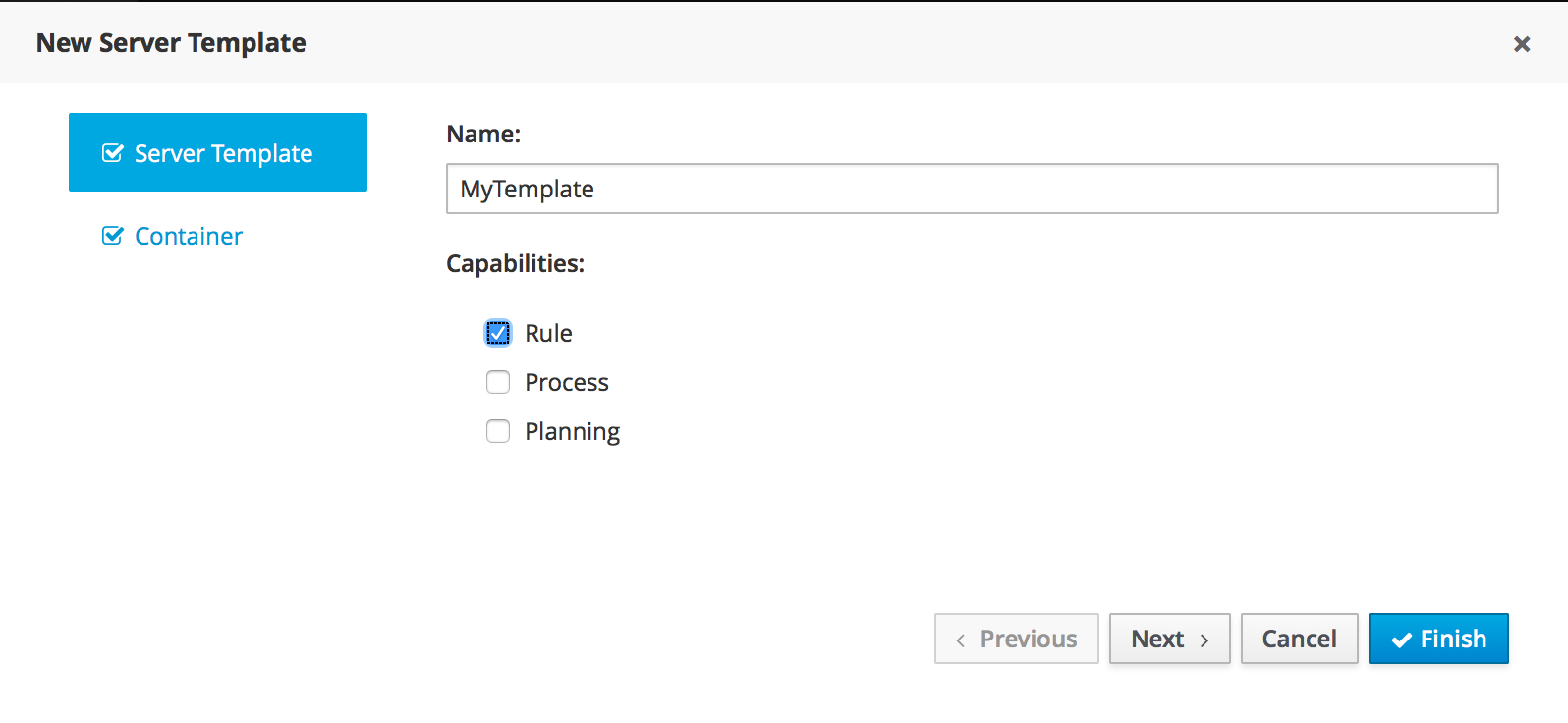
Once created you’ll get the new Template listed on the left hand side, with the new Server Template highlighted. On the right hand side you get the 2nd level navigation that lists Containers and Remote Servers that are related to selected Server Template.
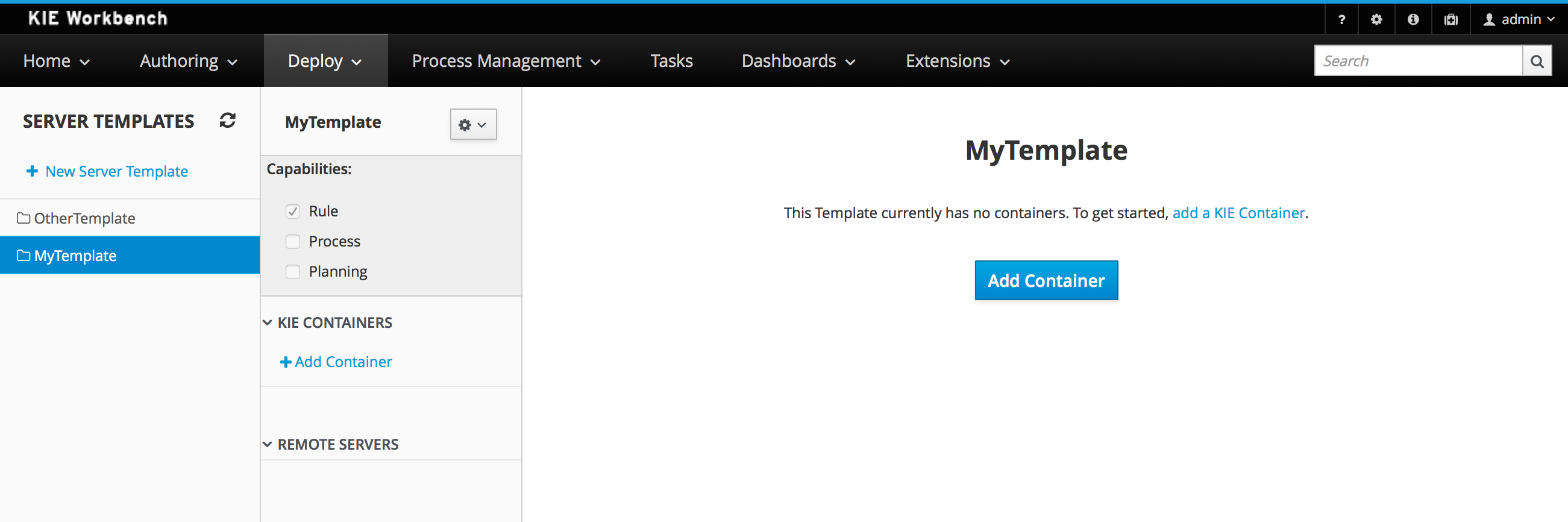
On top of the navigation is also possible to delete the current Server Template or create a copy of it.
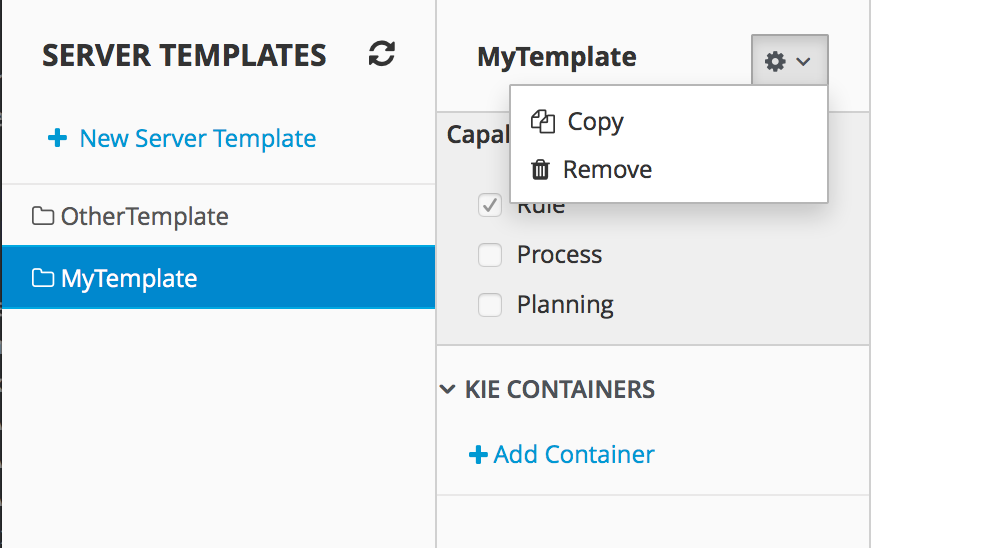
18.10.2. Container
A Container is a KIE Container configuration of the Server Template. Click the Add Container button to create a new container for the current Server Template.
The search area can help users find an specific KJARs that they are looking for.
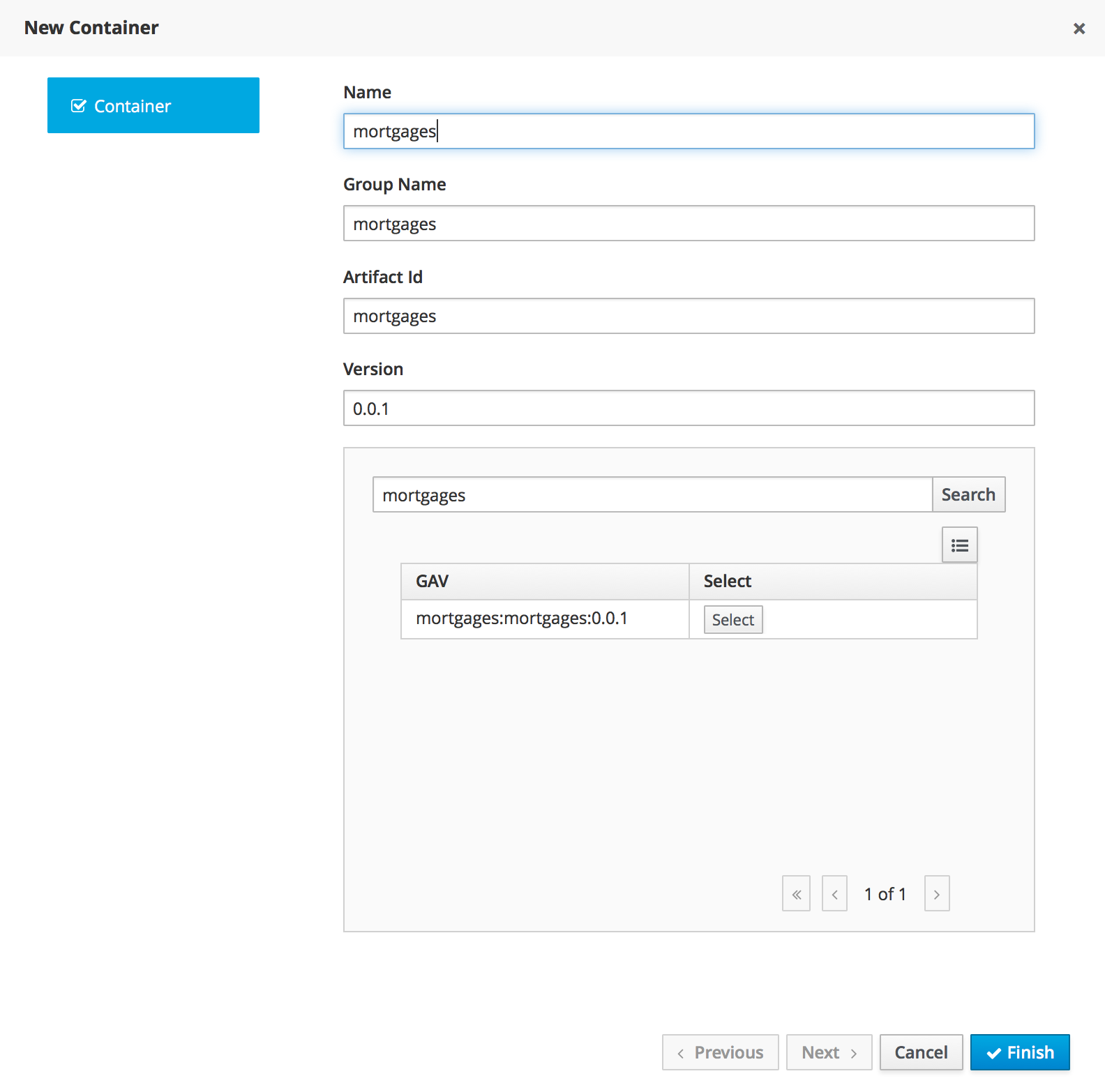
For Server Templates that have Process capabilities enabled, the Wizard has a 2nd optional step where users can configure some process related behaviors.
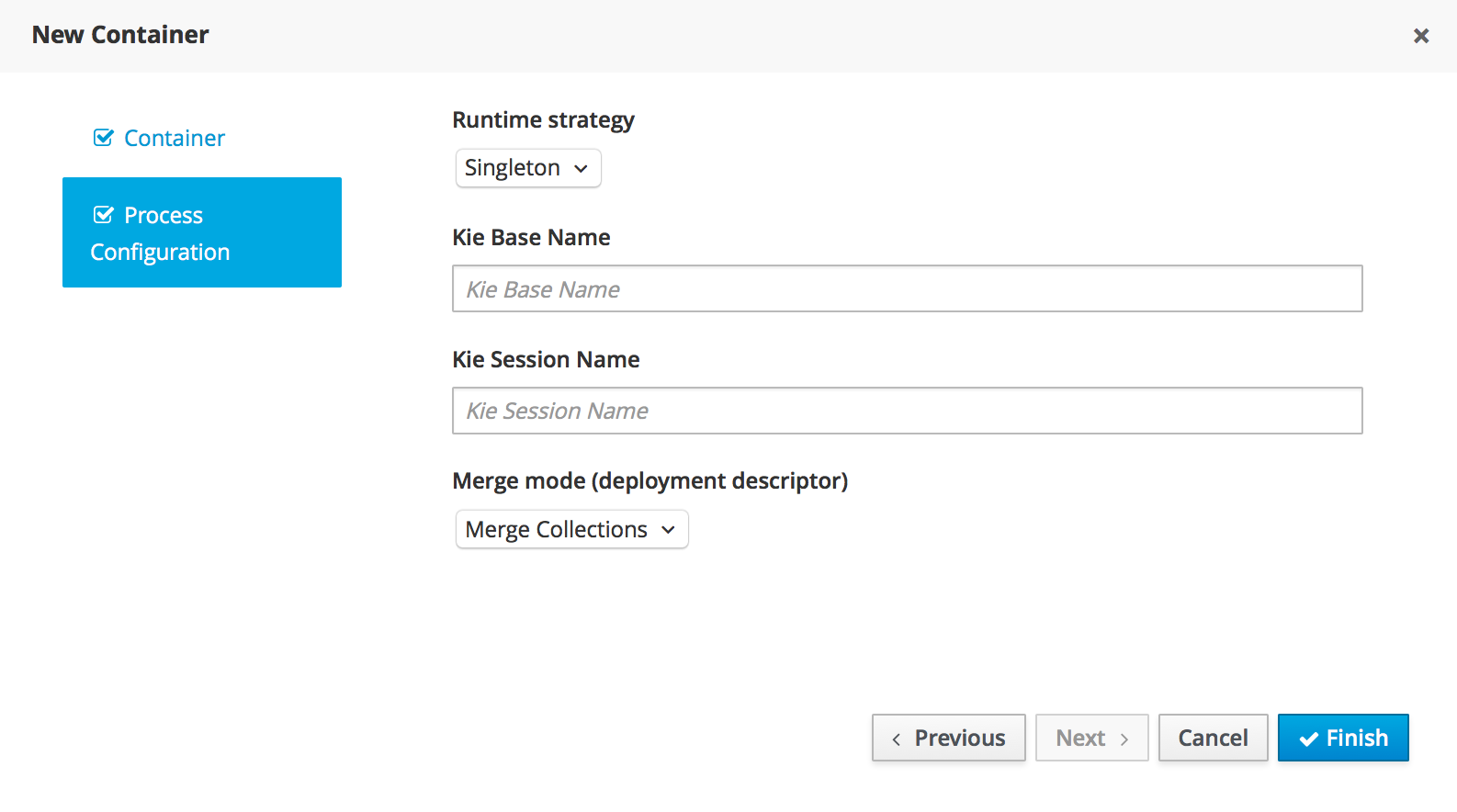
Kie Base Name determines which Kie Base of the deployed artifact will be used.
Kie Session Name determines which Kie Session of the selected Kie Base will be used.
Please notice that configurations on this tab takes effect only if the deployed project contains some business processeses. It is not enough if the server template has the extension for processes enabled. |
Once created the new Container will be displayed on the containers list just above the list of remote servers. Just after created a container is by default Stopped which is the only state that allows users to remove it.
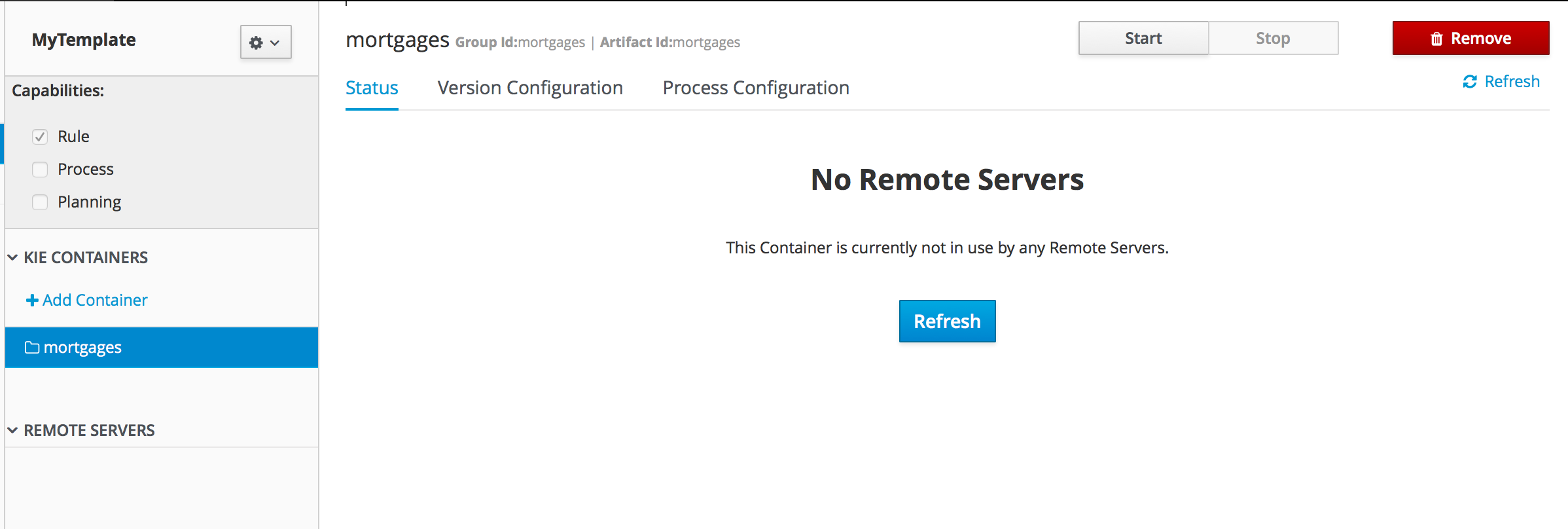
A Container has the following tabs available for management and/or configuration:
-
Status
-
Version Configuration
-
Process Configuration
Status tab lists all the Remote Servers that are running the active Container. Each Remote Server is rendered as a Card, which displays to users status and endpoint.
Only started Containers are deployed to remote servers. |
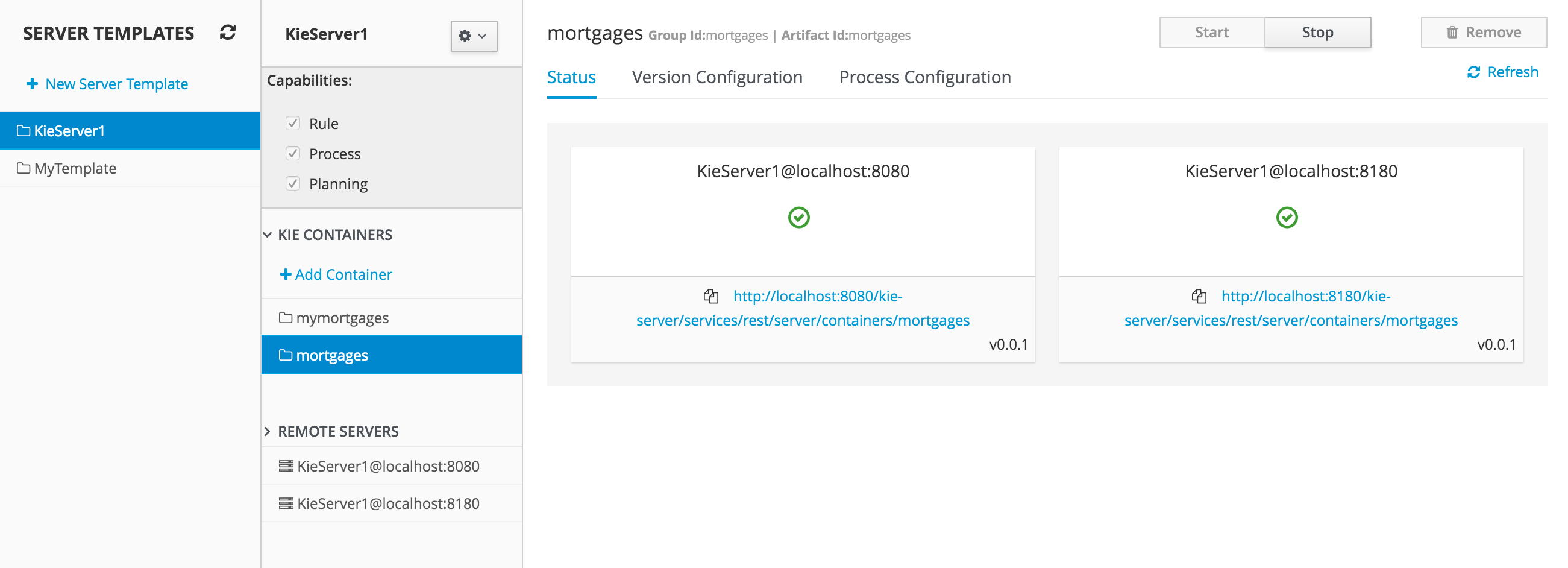
For containers that do not have process capability the Version Configuration tab allows users to change the current version of the Container. Users can upgrade manually to a specific version using the "Upgrade" button or enable/disable the Scanner. It’s also possible to execute a Scan Now operation that will scan for new versions only once.
To redeploy SNAPSHOT kjars with your latest changes all existing containers with that version must first be removed. Executing 'build and deploy' will then create a container with the latest SNAPSHOT kjar. However, this is not possible for release versions. Following maven release convensions if the GAV of a kjar is anthing but SNAPSHOT, the GAV will need to be updated to the newer release version and deployed to its own container. The new release version can also be used to upgrade an existing container as describe previously provided the container does not have process capability.
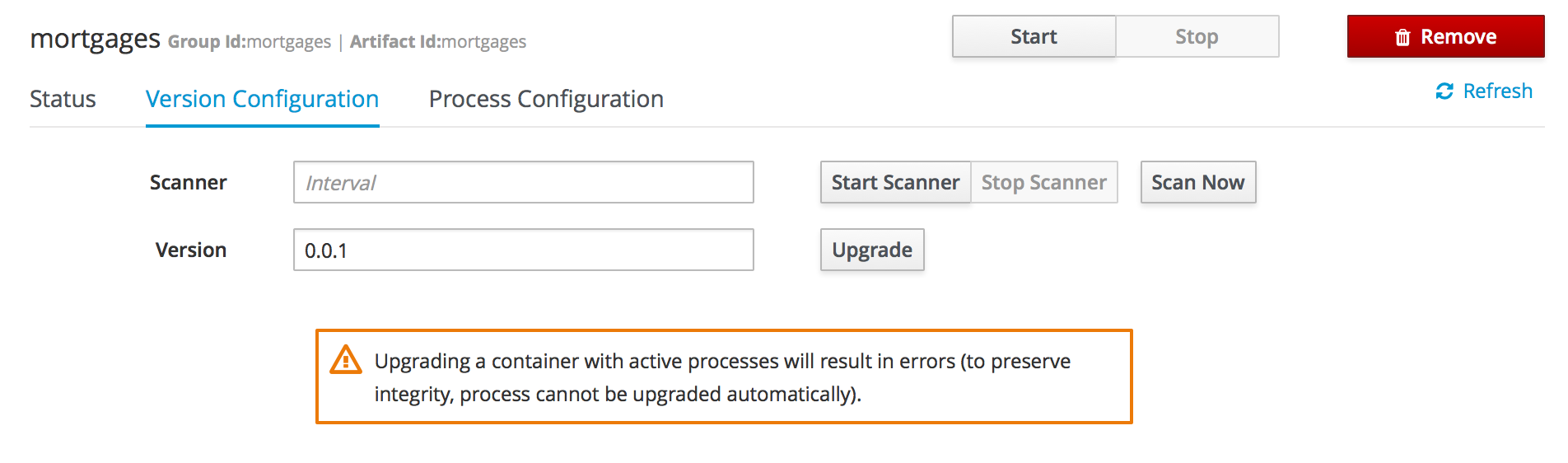
Process Configuration is the same form that is displayed during New Container Wizard for Template Servers that have Process Capability. If Template Server doesn’t have such capability, the action buttons will be disabled.
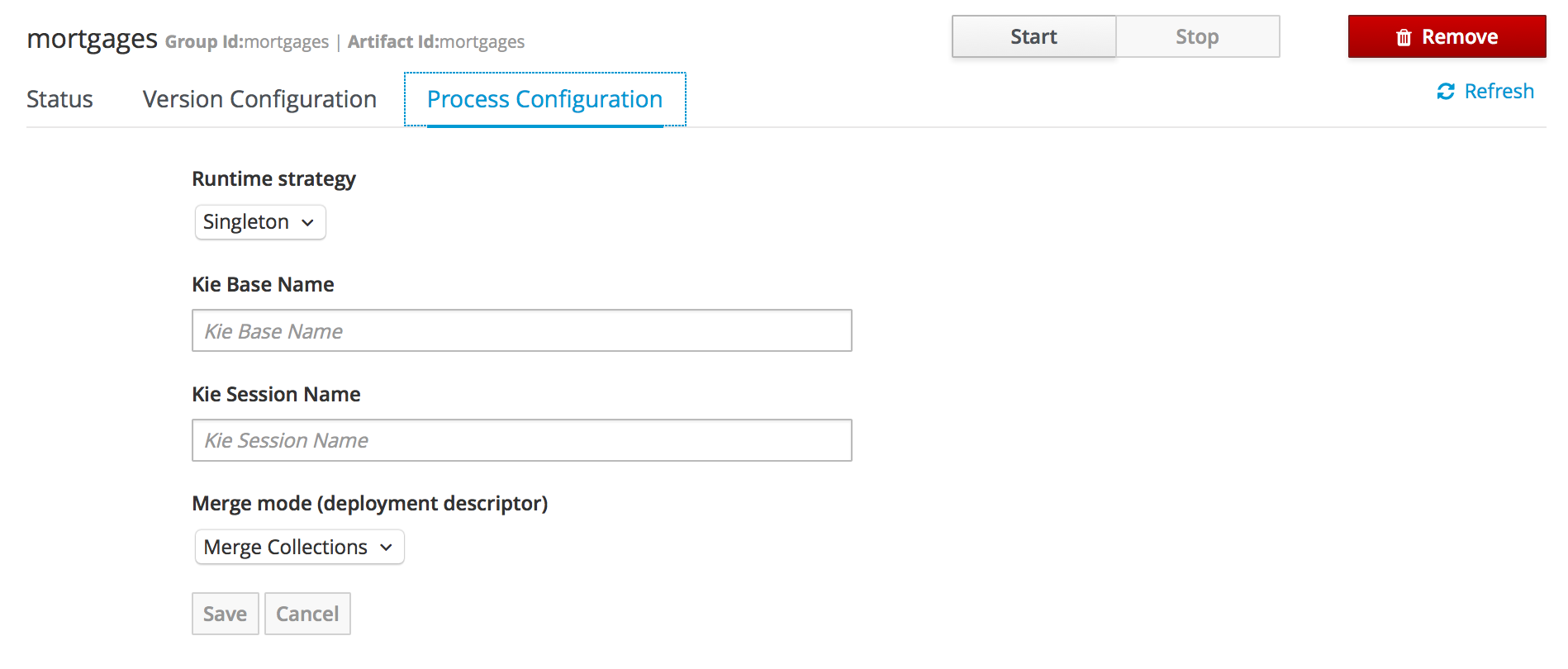
18.10.3. Remote Server
Remote Server is a Managed KIE Server instance running that has a Drools controller configured.
By default, Business Central comes with a Drools controller embedded. |
The list of Remote Servers are displayed just under the list of Containers. Once selected the screens reveals the Remote Server details and a list of cards, each card represents a running Container.
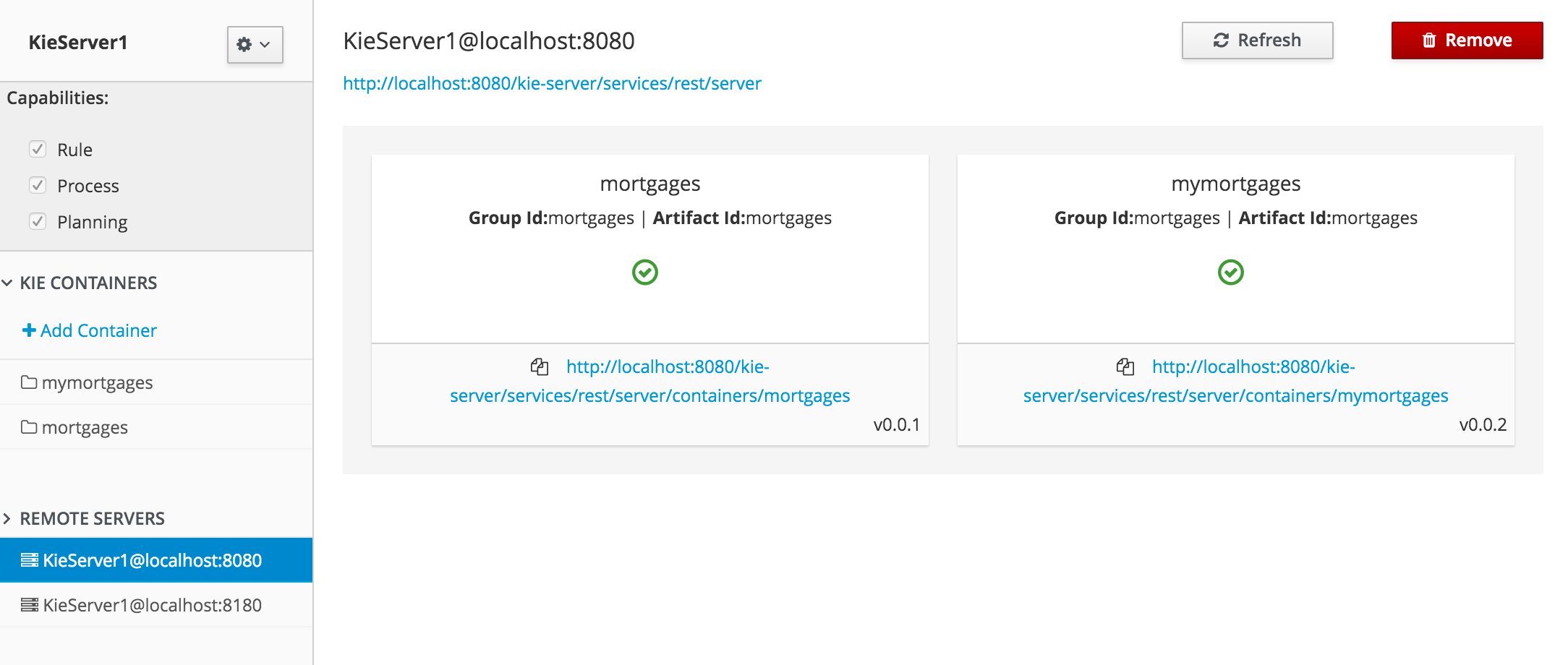
18.11. Experimental Features Framework
This section describes the Experimental Features Framework functionality and how to use it.
18.11.1. Introduction
The Experimental Features Framework is a platform service that allows developers to deliver features which are still not part of Business Central (for example, ongoing developments, tech previews, POCs…) and expose these features to users to let them have a preview of what is comming in the future.
The Experimental Features Framework provides the following features:
-
New Editor UI, accessible on the Admin page, where users can enable and disable Experimental Features.
-
Support for user-level features (stored as system preferences for each user) and global features (only available to
admin
users, in the editor) -
Ability to dynamically handle the visibility for different Experimental Resources on Business Central.
-
Business Central Perspectives
-
Business Central Screens
-
Business Central Editors
-
Library Asset Types
-
Page Builder Layout Components
-
18.11.2. Types of Experimental Features
There are two types of Experimental Features, each with different scopes:
-
User: This type of feature can be enabled or disabled for any platform user, making the feature available for a single user without affecting other users, storing the feature state as a user preference.
-
Global: This type of feature is global for all users. Only users with administrator permissions user can enable them.
18.11.3. Experimental Features Editor
The Experimental Features Framework provides an editor where users can configure the features that they want to use. To open the editor, navigate to the Admin page and click the Experimental menu option.
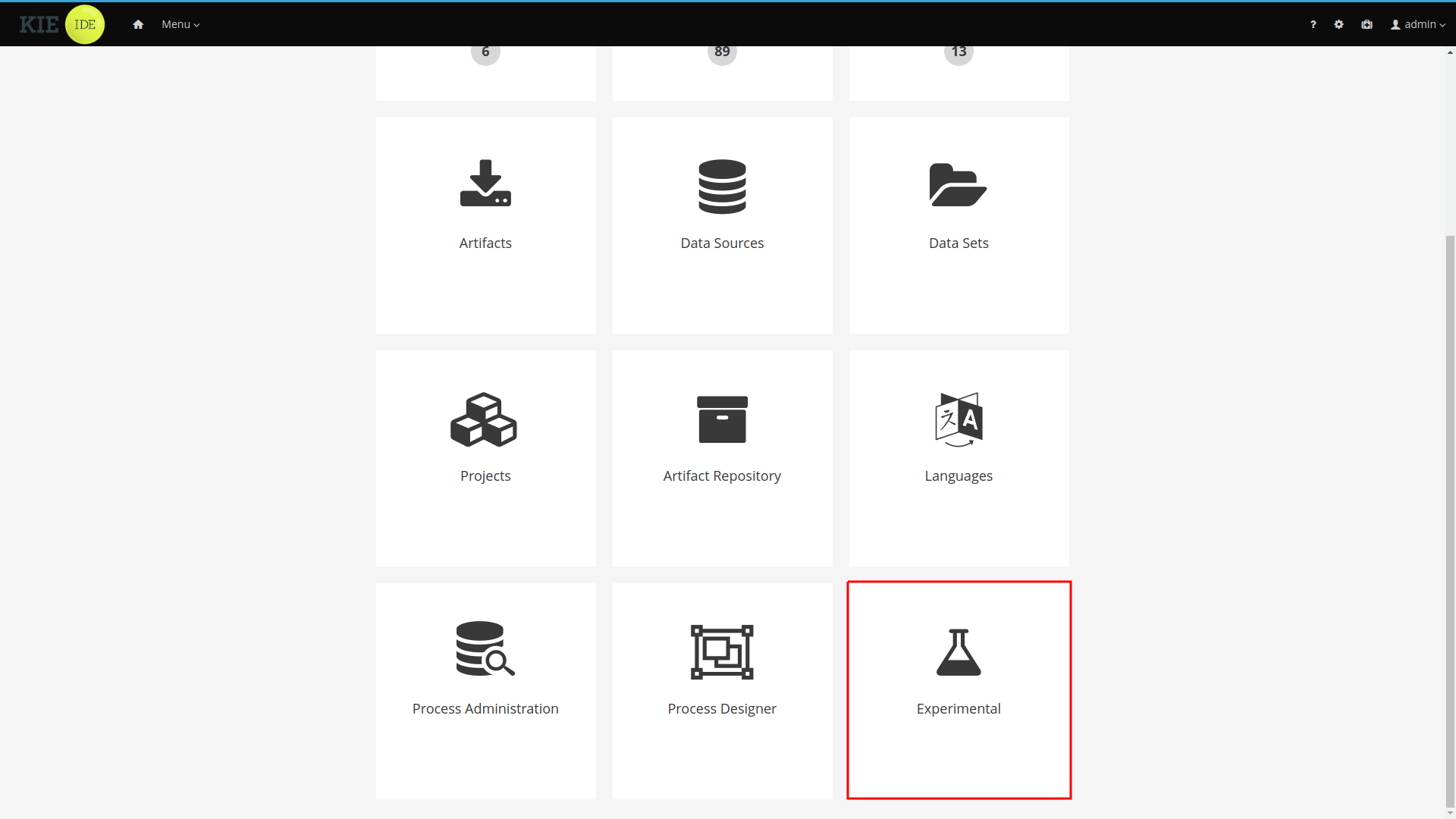
The Experimental menu option only appears if the Experimental Features Framework is enabled and there are Experimental Features installed on Business Central |
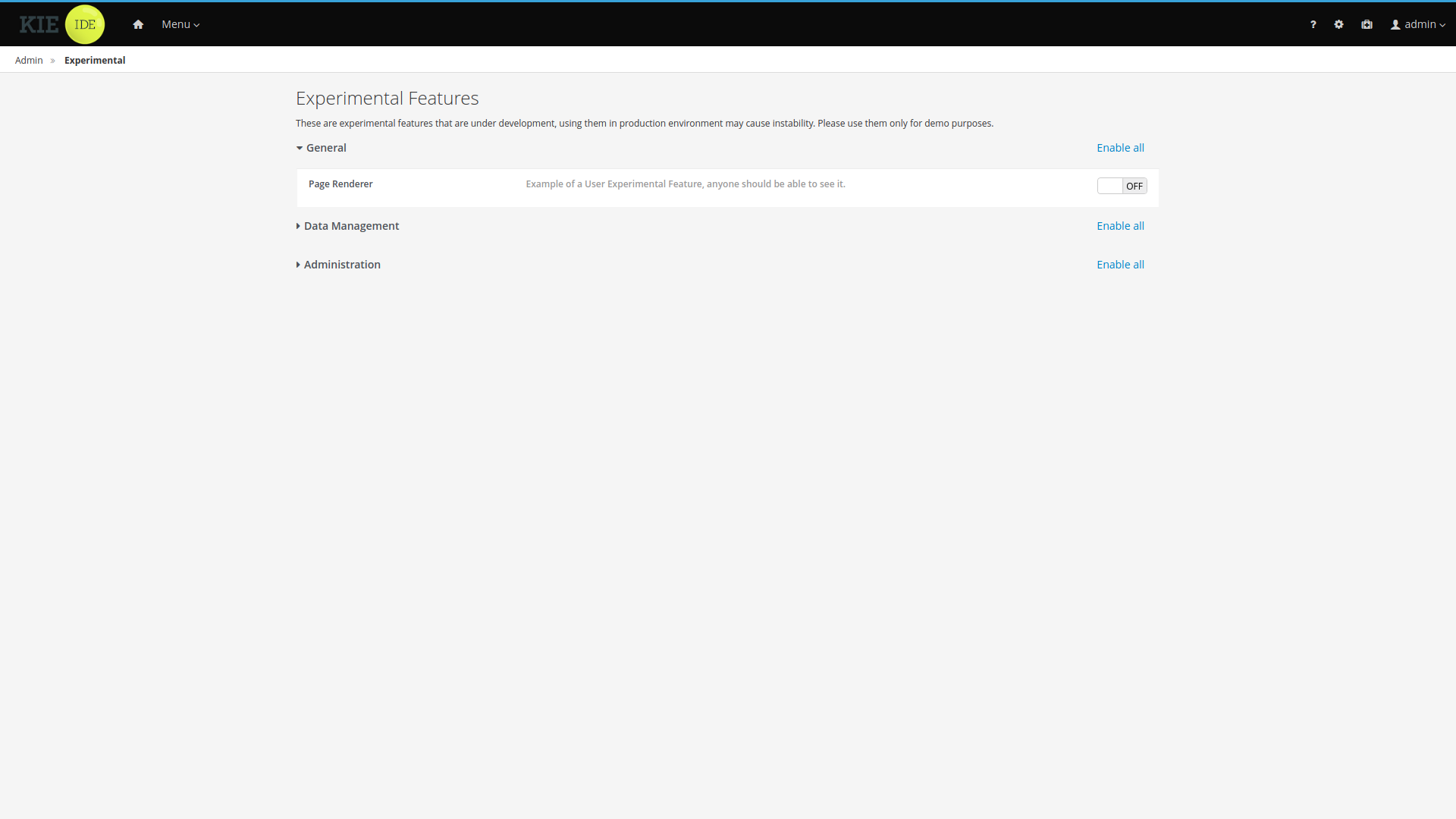
The features and groups displayed on this documentation are examples. |
The Experimental Features Editor displays all the Experimental Features installed on Business Central. For a better user experience these features are organized in collapsible groups. Click a label to expand or collapse a group.

Each row inside of the group corresponds to an experimental feature. Click toggle button to enable or disable the feature.
You can also enable or disable all group features by clicking the group’s *Enable all" / "Disable all" button.

18.11.4. Enabling the Experimental Features Framework
By default, the Experimental Features Framework is disabled. You can enable it by starting Business Central
and setting the system property appformer.experimental.features=true
.
Any Experimental Feature present on Business Central will not be accessible to users while the Experimental Features Framework is disabled.
18.12. Business Central profiles
Starting on 7.15.0.Final, KIE Workbench is renamed to Business Central. Business Central contains all KIE Workbench features. To select between the set of available features, the concept of profiles is introduced. This chapter describes profiles and show how you can configure them in Business Central.
18.12.1. Introduction
When you start the Business Central application, all the features are available to you by default. To configure a set of features, you can select from a list of profile.
A profile is a set of features which contains:
-
Menus
-
Resources that it can handle
-
Specific home page
Currently, we have two profiles: * Full: All workbench features will be enabled (default); * Planner and Rules: Only Optaplanner and Drools features will be available.
18.12.2. Selecting a profile
Profiles can be selected on Administration page, by selecting the Profiles preference.
Only admin users have access to the Profiles preference. |
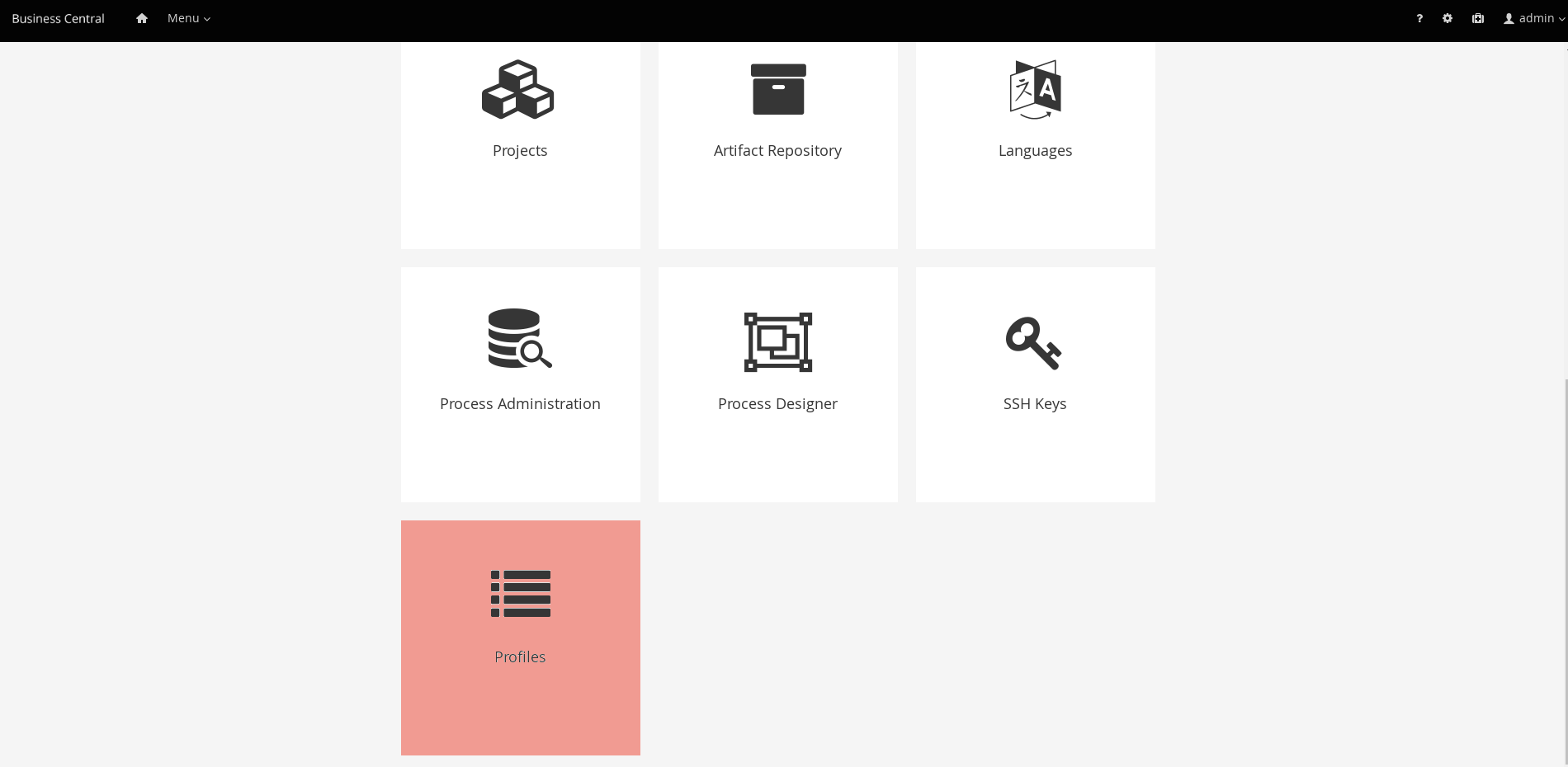
It is also possible to select a profile using the system property org.kie.workbench.profile
, which can have the values FULL
(for Full profile) and PLANNER_AND_RULES
(For Planner and Rules profile).
19. Authoring rule assets
19.1. Creating a package
Configuring packages is generally something that is done once, and by someone with some experience with rules/models. Generally speaking, very few people will need to configure packages, and once they are setup, they can be copied over and over if needed. Package configuration is most definitely a technical task that requires the appropriate expertise.
All assets live in "packages" in Business Central - a package is like a folder (it also serves as a "namespace"). A home folder for rule assets to live in. Rules in particular need to know what the fact model is, what the namespace is etc.
So while rules (and assets in general) can appear in any number of categories, they only live in one package. If you think of Business Central as a file system, then each package is a folder, and the assets live in that folder - as one big happy list of files.
To create an empty package select "Package" from the "Add Asset" menu.
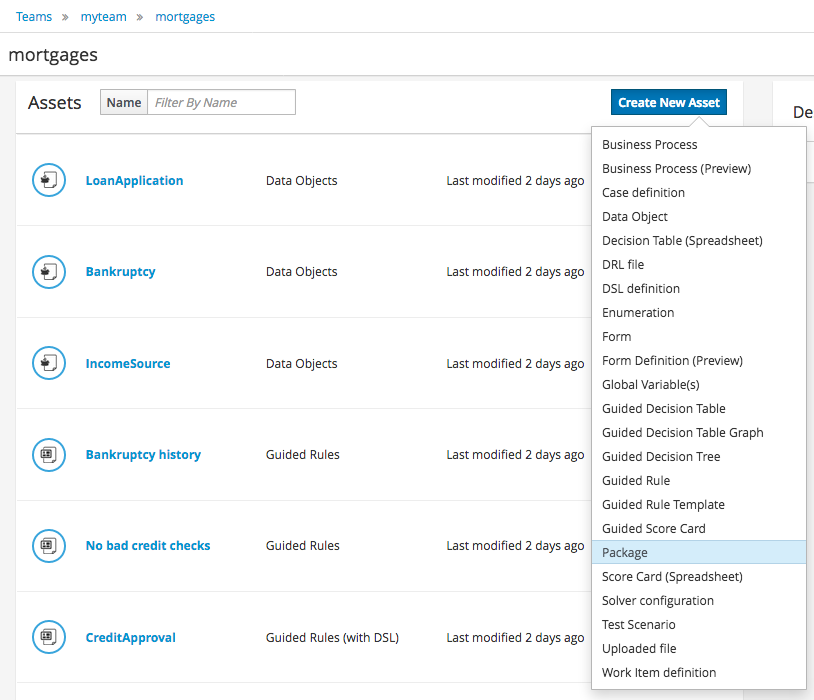
19.1.1. Empty package
An empty package can be created by simply specifying a name.
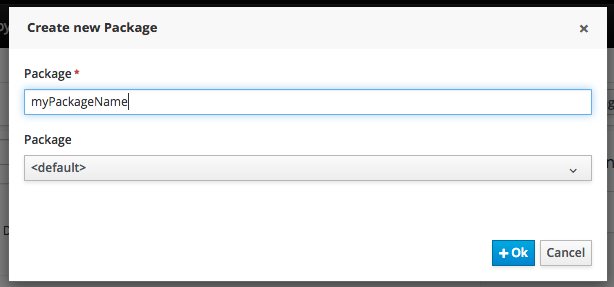
Once the Package has been created it will appear in the Project Explorer.
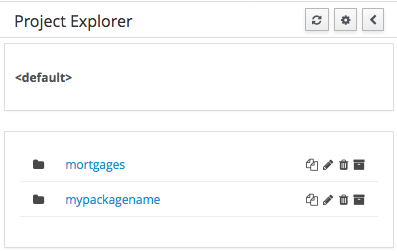
19.1.2. Copy, rename, and delete packages
As already mentioned on Project Explorer section, users can copy, rename or delete a package directly from Project Explorer.
19.2. Guided rules
Guided rules are business rules that you create in a UI-based guided rules designer in Business Central that leads you through the rule-creation process. The guided rules designer provides fields and options for acceptable input based on the data objects for the rule being defined. The guided rules that you define are compiled into Drools Rule Language (DRL) rules as with all other rule assets.
All data objects related to a guided rule must be in the same project package as the guided rule. Assets in the same package are imported by default. After you create the necessary data objects and the guided rule, you can use the Data Objects tab of the guided rules designer to verify that all required data objects are listed or to import other existing data objects by adding a New item.
19.2.1. Creating guided rules
Guided rules enable you to define business rules in a structured format, based on the data objects associated with the rules. You can create and define guided rules individually for your project.
-
In Business Central, go to Menu → Design → Projects and click the project name.
-
Click Add Asset → Guided Rule.
-
Enter an informative Guided Rule name and select the appropriate Package. The package that you specify must be the same package where the required data objects have been assigned or will be assigned.
You can also select Show declared DSL sentences if any domain specific language (DSL) assets have been defined in your project. These DSL assets will then become usable objects for conditions and actions that you define in the guided rules designer.
-
Click Ok to create the rule asset.
The new guided rule is now listed in the Guided Rules panel of the Project Explorer, or in the Guided Rules (with DSL) panel if you selected the Show declared DSL sentences option.
-
Click the Data Objects tab and confirm that all data objects required for your rules are listed. If not, click New item to import data objects from other packages, or create data objects within your package.
-
After all data objects are in place, return to the Model tab of the guided rules designer and use the buttons on the right side of the window to add and define the WHEN (condition) and THEN (action) sections of the rule, based on the available data objects.
Figure 277. The guided rules designerThe WHEN part of the rule contains the conditions that must be met to execute an action. For example, if a bank requires loan applicants to have over 21 years of age, then the WHEN condition of an
Underage
rule would beAge | less than | 21
.The THEN part of the rule contains the actions to be performed when the conditional part of the rule has been met. For example, when the loan applicant is under 21 years old, the THEN action would set
approved
tofalse
, declining the loan because the applicant is under age.You can also specify exceptions for more complex rules, such as if a bank may approve of an under-aged applicant when a guarantor is involved. In that case, you would create or import a guarantor data object and then add the field to the guided rule.
-
After you define all components of the rule, click Validate in the upper-right toolbar of the guided rules designer to validate the guided rule. If the rule validation fails, address any problems described in the error message, review all components in the rule, and try again to validate the rule until the rule passes.
-
Click Save in the guided rules designer to save your work.
19.2.1.1. Adding WHEN conditions in guided rules
The WHEN part of the rule contains the conditions that must be met to execute an action. For example, if a bank requires loan applicants to have over 21 years of age, then the WHEN condition of an Underage
rule would be Age | less than | 21
. You can set simple or complex conditions to determine how and when your rules are applied.
-
All data objects required for your rules have been created or imported and are listed in the Data Objects tab of the guided rules designer.
-
In the guided rules designer, click the plus icon (
) on the right side of the
WHEN
section.The Add a condition to the rule window with the available condition elements opens.
Figure 278. Add a condition to the ruleThe list includes the data objects from the Data Objects tab of the guided rules designer, any DSL objects defined for the package (if you selected Show declared DSL sentences when you created this guided rule), and the following standard options:
-
The following does not exist: Use this to specify facts and constraints that must not exist.
-
The following exists: Use this to specify facts and constraints that must exist. This option is triggered on only the first match, not subsequent matches.
-
Any of the following are true: Use this to list any facts or constraints that must be true.
-
From: Use this to define a
From
conditional element for the rule. -
From Accumulate: Use this to define an
Accumulate
conditional element for the rule. -
From Collect: Use this to define a
Collect
conditional element for the rule. -
From Entry Point: Use this to define an
Entry Point
for the pattern. -
Free form DRL: Use this to insert a free-form DRL field where you can define condition elements freely, without the guided rules designer.
-
-
Choose a condition element (for example, LoanApplication) and click Ok.
-
Click the condition element in the guided rules designer and use the Modify constraints for LoanApplication window to add a restriction on a field, apply multiple field constraints, add a new formula style expression, apply an expression editor, or set a variable name.
Figure 279. Modify a conditionA variable name enables you to identify a fact or field in other constructs within the guided rule. For example, you could set the variable of
LoanApplication
toa
and then referencea
in a separateBankruptcy
constraint that specifies which application the bankruptcy is based on.a : LoanApplication() Bankruptcy( application == a ).
After you select a constraint, the window closes automatically.
-
Choose an operator for the restriction (for example,
greater than
) from the drop-down menu next to the added restriction. -
Click the edit icon (
) to define the field value. The field value can be a literal value, a formula, or a full MVEL expression.
-
To apply multiple field constraints, click the condition and in the Modify constraints for LoanApplication window, select All of(And) or Any of(Or) from the Multiple field constraint drop-down menu.
Figure 280. Add multiple field constraints -
Click the constraint in the guided rules designer and further define the field value.
-
After you define all condition components of the rule, click Validate in the upper-right toolbar of the guided rules designer to validate the guided rule conditions. If the rule validation fails, address any problems described in the error message, review all components in the rule, and try again to validate the rule until the rule passes.
-
Click Save in the guided rules designer to save your work.
19.2.1.2. Adding THEN actions in guided rules
The THEN part of the rule contains the actions to be performed when the WHEN condition of the rule has been met. For example, when a loan applicant is under 21 years old, the THEN action might set approved
to false
, declining the loan because the applicant is under age. You can set simple or complex actions to determine how and when your rules are applied.
-
All data objects required for your rules have been created or imported and are listed in the Data Objects tab of the guided rules designer.
-
In the guided rules designer, click the plus icon (
) on the right side of the
THEN
section.The Add a new action window with the available action elements opens.
Figure 281. Add a new action to the ruleThe list includes insertion and modification options based on the data objects in the Data Objects tab of the guided rules designer, and on any DSL objects defined for the package (if you selected Show declared DSL sentences when you created this guided rule):
-
Change field values of: Use this to set the value of fields on a fact (such as
LoanApplication
) without notifying the Drools engine of the change. -
Delete: Use this to delete a fact.
-
Modify: Use this to specify fields to be modified for a fact and to notify the Drools engine of the change.
-
Insert fact: Use this to insert a fact and define resulting fields and values for the fact.
-
Logically Insert fact: Use this to insert a fact logically into the Drools engine and define resulting fields and values for the fact. The Drools engine is responsible for logical decisions on insertions and retractions of facts. After regular or stated insertions, facts have to be retracted explicitly. After logical insertions, facts are automatically retracted when the conditions that originally asserted the facts are no longer true.
-
Add free form DRL: Use this to insert a free-form DRL field where you can define condition elements freely, without the guided rules designer.
-
Call method on: Use this to invoke a method from another fact.
-
-
Choose an action element (for example, Modify) and click Ok.
-
Click the action element in the guided rules designer and use the Add a field window to select a field.
Figure 282. Add a fieldAfter you select a field, the window closes automatically.
-
Click the edit icon (
) to define the field value. The field value can be a literal value or a formula.
-
After you define all action components of the rule, click Validate in the upper-right toolbar of the guided rules designer to validate the guided rule actions. If the rule validation fails, address any problems described in the error message, review all components in the rule, and try again to validate the rule until the rule passes.
-
Click Save in the guided rules designer to save your work.
19.2.2. User-driven drop-down lists (data enumerations)
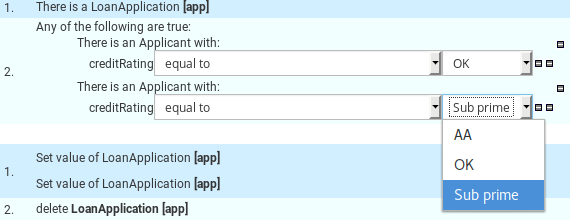
Note that is it possible to limit field values to items in a pre-configured list. This list is either defined by a Java enumeration or configured as part of the package (using a data enumeration to provide values for the drop down list). These values can be a fixed list, or (for example) loaded from a database. This is useful for codes, and other fields where there are set values. It is also possible to have what is displayed on screen, in a drop down, be different to the value (or code) used in a rule. See the section on data enumerations for how these are configured.
It is possible to define a list of values for one field that are dependent upon the value of one or more other fields, on the same Fact (e.g. a list of "Cities" depending on the selected "Country region").
For more information about data enumerations, see Data enumerations (drop-down list configurations).
19.2.3. Augmenting with DSL sentences
If the package the rule is part of has a DSL configuration, when when you add conditions or actions, then it will provide a list of "DSL Sentences" which you can choose from - when you choose one, it will add a row to the rule - where the DSL specifies values come from a user, then a edit box (text) will be shown (so it ends up looking a bit like a form). This is optional, and there is another DSL editor. Please note that the DSL capabilities in this editor are slightly less then the full set of DSL features (basically you can do [when] and [then] sections of the DSL only - which is no different to drools 3 in effect).
The following diagram shows the DSL sentences in action in the guided editor:
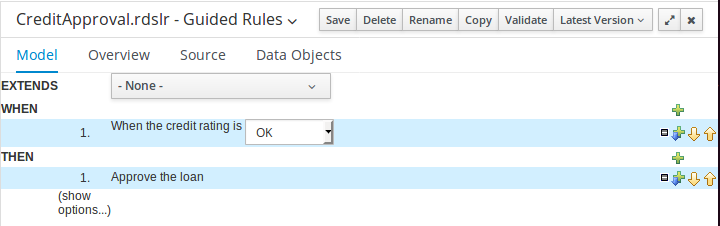
19.2.4. Adding other rule options
You can also use the rule designer to add metadata within a rule, define additional rule attributes (such as salience
and no-loop
), and freeze areas of the rule to restrict modifications to conditions or actions.
-
In the rule designer, click (show options…) under the THEN section.
-
Click the plus icon (
) on the right side of the window to add options.
-
Select an option to be added to the rule:
-
Metadata: Enter a metadata label and click the plus icon (
). Then enter any needed data in the field provided in the rule designer.
-
Attribute: Select from the list of rule attributes. Then further define the value in the field or option displayed in the rule designer.
-
Freeze areas for editing: Select Conditions or Actions to restrict the area from being modified in the rule designer.
Figure 285. Rule options
-
-
Click Save in the rule designer to save your work.
19.2.4.1. Rule attributes
Rule attributes are additional specifications that you can add to business rules to modify rule behavior. The following table lists the names and supported values of the attributes that you can assign to rules:
Attribute | Value |
---|---|
|
An integer defining the priority of the rule. Rules with a higher salience value are given higher priority when ordered in the activation queue. Example: |
|
A Boolean value. When the option is selected, the rule is enabled. When the option is not selected, the rule is disabled. Example: |
|
A string containing a date and time definition. The rule can be activated only if the current date and time is after a Example: |
|
A string containing a date and time definition. The rule cannot be activated if the current date and time is after the Example: |
|
A Boolean value. When the option is selected, the rule cannot be reactivated (looped) if a consequence of the rule re-triggers a previously met condition. When the condition is not selected, the rule can be looped in these circumstances. Example: |
|
A string identifying an agenda group to which you want to assign the rule. Agenda groups allow you to partition the agenda to provide more execution control over groups of rules. Only rules in an agenda group that has acquired a focus are able to be activated. Example: |
|
A string identifying an activation (or XOR) group to which you want to assign the rule. In activation groups, only one rule can be activated. The first rule to fire will cancel all pending activations of all rules in the activation group. Example: |
|
A long integer value defining the duration of time in milliseconds after which the rule can be activated, if the rule conditions are still met. Example: |
|
A string identifying either Example: |
|
A Quartz calendar definition for scheduling the rule. Example: |
|
A Boolean value, applicable only to rules within agenda groups. When the option is selected, the next time the rule is activated, a focus is automatically given to the agenda group to which the rule is assigned. Example: |
|
A Boolean value, applicable only to rules within rule flow groups or agenda groups. When the option is selected, the next time the ruleflow group for the rule becomes active or the agenda group for the rule receives a focus, the rule cannot be activated again until the ruleflow group is no longer active or the agenda group loses the focus. This is a stronger version of the Example: |
|
A string identifying a rule flow group. In rule flow groups, rules can fire only when the group is activated by the associated rule flow. Example: |
|
A string identifying either Example: |
19.2.5. A more complex example:
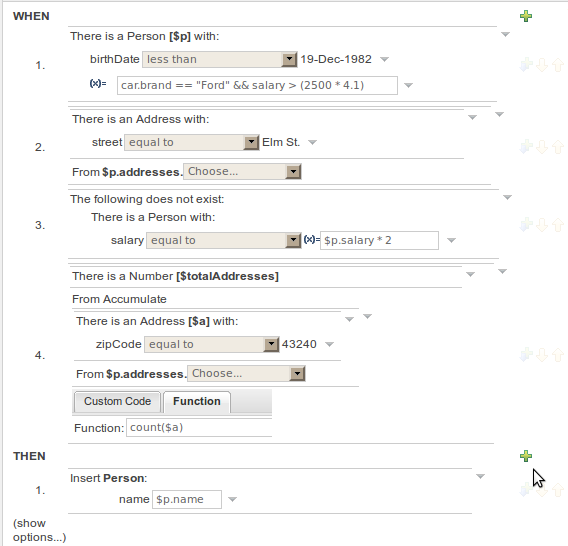
In the above example, you can see how to use a mixture of Conditional Elements, literal values, and formulas. The rule has 4 "top level" Patterns and 1 Action. The "top level" Patterns are:
-
A Fact Pattern on Person. This Pattern contains two field constraints: one for birthDate field and the other one is a formula. Note that the value of the birthDate restriction is selected from a calendar. Another thing to note is that you can make calculations and use nested fields in the formula restriction (i.e. car.brand). Finally, we are setting a variable name ($p) to the Person Fact Type. You can then use this variable in other Patterns.
The generated DRL from this Pattern will be:
$p : Person( birthDate < "19-Dec-1982" , eval( car.brand == "Ford" && salary > (2500 * 4.1) ))
-
A From Pattern. This condition will create a match for every Address whose street name is "Elm St." from the Person’s list of addresses. The left side of the from is a regular Fact Pattern and the right side is an Expression Builder that let us inspect variable’s fields.
The generated DRL from this Pattern will be:
Address( street == "Elm St." ) from $p.addresses
-
A "Not Exist" Conditional Element. This condition will match when its content doesn’t create a match. In this case, its content is a regular Fact Pattern (on Person). In this Fact Pattern you can see how variables ($p) could be used inside a formula value.
The generated DRL from this Pattern will be:
not Person( salary == ( $p.salary * 2 ) )
-
A "From Accumulate" Conditional Element. This is maybe one of the most complex Patterns you can use. It consist in a Left Pattern (It must be a Fact Pattern. In this case is a Number Pattern. The Number is named $totalAddresses), a Source Pattern (Which could be a Fact Pattern, From, Collect or Accumulate conditional elements. In this case is an Address Pattern Restriction with a field restriction in its zip field) and a Formula Section where you can use any built-in or custom Accumulate Function (in this example a count() function is used). Basically, this Conditional Element will count the addresses having a zip code of 43240 from the Person’s list of addresses.
The generated DRL from this Pattern will be:
$totalAddresses : Number() from accumulate ( $a : Address( zipCode == " 43240" ) from $p.addresses, count( $a ) )
19.3. Guided rule templates
Guided rule templates are business rule structures with placeholder values (template keys) that are interchanged with actual values defined in separate data tables. Each row of values defined in the corresponding data table for that template results in a rule. Guided rule templates are ideal when many rules have the same conditions, actions, and other attributes but differ in values of facts or constraints. In such cases, instead of creating many similar guided rules and defining values in each rule, you can create a guided rule template with the rule structure that applies to each rule and then define only the differing values in the data table.
The guided rule templates designer provides fields and options for acceptable template input based on the data objects for the rule template being defined, and a corresponding data table where you add template key values. After you create your guided rule template and add values in the corresponding data table, the rules you defined are compiled into Drools Rule Language (DRL) rules as with all other rule assets.
All data objects related to a guided rule template must be in the same project package as the guided rule template. Assets in the same package are imported by default. After you create the necessary data objects and the guided rule template, you can use the Data Objects tab of the guided rule templates designer to verify that all required data objects are listed or to import other existing data objects by adding a New item.
19.3.1. Creating guided rule templates
You can use guided rule templates to define rule structures with placeholder values (template keys) that correspond to actual values defined in a data table. Guided rule templates are an efficient alternative to defining sets of many guided rules individually that use the same structure.
-
In Business Central, go to Menu → Design → Projects and click the project name.
-
Click Add Asset → Guided Rule Template.
-
Enter an informative Guided Rule Template name and select the appropriate Package. The package that you specify must be the same package where the required data objects have been assigned or will be assigned.
-
Click Ok to create the rule template.
The new guided rule template is now listed in the Guided Rule Templates panel of the Project Explorer.
-
Click the Data Objects tab and confirm that all data objects required for your rules are listed. If not, click New item to import data objects from other packages, or create data objects within your package.
-
After all data objects are in place, return to the Model tab and use the buttons on the right side of the window to add and define the WHEN (condition) and THEN (action) sections of the rule template, based on the available data objects. For the field values that vary per rule, use template keys in the format
$key
in the rule designer or in the format@{key}
in free form DRL (if used).Figure 287. Sample guided rule templateNote on template keysTemplate keys are fundamental in guided rule templates. Template keys are what enable field values in the templates to be interchanged with actual values that you define in the corresponding data table to generate different rules from the same template. You can use other value types, such as Literal or Formula, for values that are part of the rule structure of all rules based on that template. However, for any values that differ among the rules, use the Template key field type with a specified key. Without template keys in a guided rule template, the corresponding data table is not generated in the template designer and the template essentially functions as an individual guided rule.
The WHEN part of the rule template is the condition that must be met to execute an action. For example, if a telecommunications company charges customers based on the services they subscribe to (Internet, phone, and TV), then one of the WHEN conditions would be
internetService | equal to | $hasInternetService
. The template key$hasInternetService
is interchanged with an actual Boolean value (true
orfalse
) defined in the data table for the template.The THEN part of the rule template is the action to be performed when the conditional part of the rule has been met. For example, if a customer subscribes to only Internet service, a THEN action for
RecurringPayment
with a template key$amount
would set the actual monthly amount to the integer value defined for Internet service charges in the data table. -
After you define all components of the rule, click Save in the guided rule templates designer to save your work.
19.3.1.1. Adding WHEN conditions in guided rule templates
The WHEN part of the rule contains the conditions that must be met to execute an action. For example, if a telecommunications company charges customers based on the services they subscribe to (Internet, phone, and TV), then one of the WHEN conditions would be internetService | equal to | $hasInternetService
. The template key $hasInternetService
is interchanged with an actual Boolean value (true
or false
) defined in the data table for the template.
-
All data objects required for your rules have been created or imported and are listed in the Data Objects tab of the guided rule templates designer.
-
In the guided rule templates designer, click the plus icon (
) on the right side of the
WHEN
section.The Add a condition to the rule window with the available condition elements opens.
Figure 288. Add a condition to the ruleThe list includes the data objects from the Data Objects tab of the guided rule templates designer, any DSL objects defined for the package, and the following standard options:
-
The following does not exist: Use this to specify facts and constraints that must not exist.
-
The following exists: Use this to specify facts and constraints that must exist. This option is triggered on only the first match, not subsequent matches.
-
Any of the following are true: Use this to list any facts or constraints that must be true.
-
From: Use this to define a
From
conditional element for the rule. -
From Accumulate: Use this to define an
Accumulate
conditional element for the rule. -
From Collect: Use this to define a
Collect
conditional element for the rule. -
From Entry Point: Use this to define an
Entry Point
for the pattern. -
Free form DRL: Use this to insert a free-form DRL field where you can define condition elements freely, without the guided rules designer. For template keys in free form DRL, use the format
@{key}
.
-
-
Choose a condition element (for example, Customer) and click Ok.
-
Click the condition element in the guided rule templates designer and use the Modify constraints for Customer window to add a restriction on a field, apply multiple field constraints, add a new formula style expression, apply an expression editor, or set a variable name.
Figure 289. Modify a conditionA variable name enables you to identify a fact or field in other constructs within the guided rule. For example, you could set the variable of
Customer
toc
and then referencec
in a separateApplicant
constraint that specifies that theCustomer
is theApplicant
.c : Customer() Applicant( this == c )
After you select a constraint, the window closes automatically.
-
Choose an operator for the restriction (for example,
equal to
) from the drop-down menu next to the added restriction. -
Click the edit icon (
) to define the field value.
-
Select Template key and add a template key in the format
$key
if this value varies among the rules that are based on this template. This allows the field value to be interchanged with actual values that you define in the corresponding data table to generate different rules from the same template. For field values that do not vary among the rules and are part of the rule template, you can use any other value type. -
To apply multiple field constraints, click the condition and in the Modify constraints for Customer window, select All of(And) or Any of(Or) from the Multiple field constraint drop-down menu.
Figure 290. Add multiple field constraints -
Click the constraint in the guided rule templates designer and further define the field values.
-
After you define all condition elements, click Save in the guided rule templates designer to save your work.
19.3.1.2. Adding THEN actions in guided rule templates
The THEN part of the rule template is the action to be performed when the conditional part of the rule has been met. For example, if a customer subscribes to only Internet service, a THEN action for RecurringPayment
with a template key $amount
would set the actual monthly amount to the integer value defined for Internet service charges in the data table.
-
All data objects required for your rules have been created or imported and are listed in the Data Objects tab of the guided rule templates designer.
-
In the guided rule templates designer, click the plus icon (
) on the right side of the
THEN
section.The Add a new action window with the available action elements opens.
Figure 291. Add a new action to the ruleThe list includes insertion and modification options based on the data objects in the Data Objects tab of the guided rule templates designer, and on any DSL objects defined for the package:
-
Insert fact: Use this to insert a fact and define resulting fields and values for the fact.
-
Logically Insert fact: Use this to insert a fact logically into the Drools engine and define resulting fields and values for the fact. The Drools engine is responsible for logical decisions on insertions and retractions of facts. After regular or stated insertions, facts have to be retracted explicitly. After logical insertions, facts are automatically retracted when the conditions that originally asserted the facts are no longer true.
-
Add free form DRL: Use this to insert a free-form DRL field where you can define condition elements freely, without the guided rules designer. For template keys in free form DRL, use the format
@{key}
.
-
-
Choose an action element (for example, Logically Insert fact RecurringPayment) and click Ok.
-
Click the action element in the guided rule templates designer and use the Add a field window to select a field.
Figure 292. Add a fieldAfter you select a field, the window closes automatically.
-
Click the edit icon (
) to define the field value.
-
Select Template key and add a template key in the format
$key
if this value varies among the rules that are based on this template. This allows the field value to be interchanged with actual values that you define in the corresponding data table to generate different rules from the same template. For field values that do not vary among the rules and are part of the rule template, you can use any other value type. -
After you define all action elements, click Save in the guided rule templates designer to save your work.
19.3.1.3. Adding other rule options
You can also use the rule designer to add metadata within a rule, define additional rule attributes (such as salience
and no-loop
), and freeze areas of the rule to restrict modifications to conditions or actions.
-
In the rule designer, click (show options…) under the THEN section.
-
Click the plus icon (
) on the right side of the window to add options.
-
Select an option to be added to the rule:
-
Metadata: Enter a metadata label and click the plus icon (
). Then enter any needed data in the field provided in the rule designer.
-
Attribute: Select from the list of rule attributes. Then further define the value in the field or option displayed in the rule designer.
-
Freeze areas for editing: Select Conditions or Actions to restrict the area from being modified in the rule designer.
Figure 293. Rule options
-
-
Click Save in the rule designer to save your work.
Rule attributes
Rule attributes are additional specifications that you can add to business rules to modify rule behavior. The following table lists the names and supported values of the attributes that you can assign to rules:
Attribute | Value |
---|---|
|
An integer defining the priority of the rule. Rules with a higher salience value are given higher priority when ordered in the activation queue. Example: |
|
A Boolean value. When the option is selected, the rule is enabled. When the option is not selected, the rule is disabled. Example: |
|
A string containing a date and time definition. The rule can be activated only if the current date and time is after a Example: |
|
A string containing a date and time definition. The rule cannot be activated if the current date and time is after the Example: |
|
A Boolean value. When the option is selected, the rule cannot be reactivated (looped) if a consequence of the rule re-triggers a previously met condition. When the condition is not selected, the rule can be looped in these circumstances. Example: |
|
A string identifying an agenda group to which you want to assign the rule. Agenda groups allow you to partition the agenda to provide more execution control over groups of rules. Only rules in an agenda group that has acquired a focus are able to be activated. Example: |
|
A string identifying an activation (or XOR) group to which you want to assign the rule. In activation groups, only one rule can be activated. The first rule to fire will cancel all pending activations of all rules in the activation group. Example: |
|
A long integer value defining the duration of time in milliseconds after which the rule can be activated, if the rule conditions are still met. Example: |
|
A string identifying either Example: |
|
A Quartz calendar definition for scheduling the rule. Example: |
|
A Boolean value, applicable only to rules within agenda groups. When the option is selected, the next time the rule is activated, a focus is automatically given to the agenda group to which the rule is assigned. Example: |
|
A Boolean value, applicable only to rules within rule flow groups or agenda groups. When the option is selected, the next time the ruleflow group for the rule becomes active or the agenda group for the rule receives a focus, the rule cannot be activated again until the ruleflow group is no longer active or the agenda group loses the focus. This is a stronger version of the Example: |
|
A string identifying a rule flow group. In rule flow groups, rules can fire only when the group is activated by the associated rule flow. Example: |
|
A string identifying either Example: |
19.3.2. Defining data tables for guided rule templates
After you create a guided rule template and add template keys for field values, a data table is displayed in the Data table of the guided rule templates designer. Each column in the data table corresponds to a template key that you added in the guided rule template. Use this table to define values for each template key row by row. Each row of values that you define in the data table for that template results in a rule.
-
In the guided rule templates designer, click the Data tab to view the data table. Each column in the data table corresponds to a template key that you added in the guided rule template.
If you did not add any template keys to the rule template, then this data table does not appear and the template does not function as a genuine template but essentially as an individual guided rule. For this reason, template keys are fundamental in creating guided rule templates. -
Click Add row and define the data values for each template key column to generate that rule (row).
-
Continue adding rows and defining data values for each rule that will be generated. You can click Add row for each new row, or click the plus icon (
) or minus icon to add or remove rows.
Figure 294. Sample data table for a guided rule templateTo view the DRL code, click the Source tab in the guided rule templates designer.
Example:
rule "PaymentRules_6" dialect "mvel" when Customer( internetService == false , phoneService == false , TVService == true ) then RecurringPayment fact0 = new RecurringPayment(); fact0.setAmount( 5 ); insertLogical( fact0 ); end rule "PaymentRules_5" dialect "mvel" when Customer( internetService == false , phoneService == true , TVService == false ) then RecurringPayment fact0 = new RecurringPayment(); fact0.setAmount( 5 ); insertLogical( fact0 ); end ... //Other rules omitted for brevity.
-
As a visual aid, click the grid icon in the upper-left corner of the data table to toggle cell merging on and off, if needed. Cells in the same column with identical values are merged into a single cell.
Figure 295. Merge cells in a data tableYou can then use the expand/collapse icon [+/-] in the upper-left corner of each newly merged cell to collapse the rows corresponding to the merged cell, or to re-expand the collapsed rows.
Figure 296. Collapse merged cells -
After you define the template key data for all rules and adjust the table display as needed, click Validate in the upper-right toolbar of the guided rule templates designer to validate the guided rule template. If the rule template validation fails, address any problems described in the error message, review all components in the rule template and data defined in the data table, and try again to validate the rule template until the rule template passes.
-
Click Save in the guided rule templates designer to save your work.
19.4. Guided decision tables
Guided decision tables are a wizard-led alternative to spreadsheet decision tables for defining business rules in a tabular format. With guided decision tables, you are led by a UI-based wizard in Business Central that helps you define rule attributes, metadata, conditions, and actions based on specified data objects in your project. After you create your guided decision tables, the rules you defined are compiled into Drools Rule Language (DRL) rules as with all other rule assets.
All data objects related to a guided decision table must be in the same project package as the guided decision table. Assets in the same package are imported by default. After you create the necessary data objects and the guided decision table, you can use the Data Objects tab of the guided decision tables designer to verify that all required data objects are listed or to import other existing data objects by adding a New item.
19.4.1. Creating guided decision tables
You can use guided decision tables to define rule attributes, metadata, conditions, and actions in a tabular format that can be added to your business rules project.
-
In Business Central, go to Menu → Design → Projects and click the project name.
-
Click Add Asset → Guided Decision Table.
-
Enter an informative Guided Decision Table name and select the appropriate Package. The package that you specify must be the same package where the required data objects have been assigned or will be assigned.
-
Select Use Wizard to finish setting up the table in the wizard, or leave this option unselected to finish creating the table and specify remaining configurations in the guided decision tables designer.
-
Select the hit policy that you want your rows of rules in the table to conform to. For details, see Hit policies for guided decision tables.
-
Specify whether you want the Extended entry or Limited entry table. For details, see Types of guided decision tables.
-
Click Ok to complete the setup. If you have selected Use Wizard, the Guided Decision Table wizard is displayed. If you did not select the Use Wizard option, this prompt does not appear and you are taken directly to the table designer.
Figure 297. Create guided decision table -
If you are using the wizard, add any available imports, fact patterns, constraints, and actions, and select whether table columns should expand. Click Finish to close the wizard and view the table designer.
Figure 298. Guided Decision Table wizard
In the guided decision tables designer, you can add or edit columns and rows, and make other final adjustments.
19.4.2. Hit policies for guided decision tables
Hit policies determine the order in which rules (rows) in a guided decision table are applied, whether top to bottom, per specified priority, or other options.
The following hit policies are available:
-
None: (Default hit policy) Multiple rows can be executed and the verification warns about rows that conflict. Any decision tables that have been uploaded (using a non-guided decision table spreadsheet) will adopt this hit policy.
-
Resolved Hit: Only one row at a time can be executed according to specified priority, regardless of list order (you can give row 10 priority over row 5, for example). This means you can keep the order of the rows you want for visual readability, but specify priority exceptions.
-
Unique Hit: Only one row at a time can be executed, and each row must be unique, with no overlap of conditions being met. If more than one row is executed, then the verification produces a warning at development time.
-
First Hit: Only one row at a time can be executed in the order listed in the table, top to bottom.
-
Rule Order: Multiple rows can be executed and verification does not report conflicts between the rows since they are expected to happen.
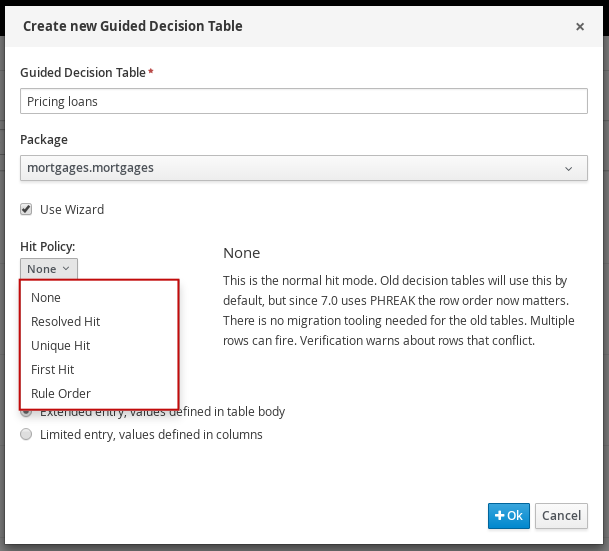
19.4.2.1. Hit policy examples: Decision table for discounts on movie tickets
The following is part of a decision table for discounts on movie tickets based on customer age, student status, or military status, or all three.
Row Number | Discount Type | Discount |
---|---|---|
1 |
Senior citizen (age 60+) |
10% |
2 |
Student |
10% |
3 |
Military |
10% |
The total discount to be applied in the end will vary depending on the hit policy specified for the table, as follows.
-
None/Rule Order: With both None and Rule Order hit policies, all applicable rules are incorporated, in this case allowing discounts to be stacked for each customer.
Example: A senior citizen who is also a student and a military veteran will receive all three discounts, totaling 30%.
Key difference: With None, warnings are created for multiple rows applied. With Rule Order, those warnings are not created.
-
First Hit/Resolved Hit: With both First Hit and Resolved Hit policies, only one of the discounts can be applied.
For First Hit, the discount that is satisfied first in the list is applied and the others are ignored.
Example: A senior citizen who is also a student and a military veteran will receive only the senior citizen discount of 10%, since that is listed first in the table.
For Resolved Hit, a modified table is required. The discount that you assign a priority exception to in the table, regardless of listed order, will be applied first. To assign this exception, include a new column that specifies the priority of one discount (row) over others.
Example: If military discounts are prioritized higher than age or student discounts, despite the listed order, then a senior citizen who is also a student and a military veteran will receive only the military discount of 10%, regardless of age or student status.
Consider the following modified decision table that accommodates a Resolved Hit policy:
Table 46. Modified decision table that accommodates a Resolved Hit policy Row Number Discount Type Has Priority over Row Discount 1
Senior citizen (age 60+)
10%
2
Student
10%
3
Military
1
10%
In this modified table, the military discount is essentially the new row 1 and therefore takes priority over both age and student discounts, and any other discounts added later. You do not need to specify priority over rows "1 and 2", only over row "1". This changes the row hit order to 3 → 1 → 2 → … and so on as the table grows.
The row order would be changed in the same way if you actually moved the military discount to row 1 and applied a First Hit policy to the table instead. However, if you want the rules listed in a certain way and applied differently, such as in an alphabetized table, the Resolved Hit policy is useful. Key difference: With First Hit, rules are applied strictly in the listed order. With Resolved Hit, rules are applied in the listed order unless priority exceptions are specified.
-
Unique Hit: A modified table is required. With a Unique Hit policy, rows must be created in a way that it is impossible to satisfy multiple rules at one time. However, you can still specify row-by-row whether to apply one rule or multiple. In this way, with a Unique Hit policy you can make decision tables more granular and prevent overlap warnings.
Consider the following modified decision table that accommodates a Unique Hit policy:
Table 47. Modified decision table that accommodates a Unique Hit policy Row Number Is Senior Citizen (age 65+) Is Student Is Military Discount 1
yes
no
no
10%
2
no
yes
no
10%
3
no
no
yes
10%
4
yes
yes
no
20%
5
yes
no
yes
20%
6
no
yes
yes
20%
7
yes
yes
yes
30%
In this modified table, each row is unique, with no allowance of overlap, and any single discount or any combination of discounts is accommodated.
Types of guided decision tables
Two types of decision tables are supported in Drools: Extended entry and Limited entry tables.
-
Extended entry: An Extended Entry decision table is one for which the column definitions specify Pattern, Field, and Operator but not value. The values, or states, are themselves held in the body of the decision table.
-
Limited entry: A Limited Entry decision table is one for which the column definitions specify value in addition to Pattern, Field, and Operator. The decision table states, held in the body of the table, are boolean where a positive value (a marked check box) has the effect of meaning the column should apply, or be matched. A negative value (a cleared check box) means the column does not apply.
19.4.3. Adding columns to guided decision tables
After you have created the guided decision table, you can define and add various types of columns within the guided decision tables designer.
-
Any data objects that will be used for column parameters, such as Facts and Fields, have been created within the same package where the guided decision table is found, or have been imported from another package in Data Objects → New item of the guided decision tables designer.
For descriptions of these column parameters, see the "Required column parameters" segments for each column type in Types of columns in guided decision tables.
For details about creating data objects, see Data Modeller.
-
In the guided decision tables designer, click Columns → Insert Column.
-
Click Include advanced options to view the full list of column options.
Figure 300. Add columns -
Select the column type that you want to add, click Next, and follow the steps in the wizard to specify the data required to add the column.
For descriptions of each column type and required parameters for setup, see Types of columns in guided decision tables.
-
Click Finish to add the configured column.
After all columns are added, you can begin adding rows of rules correlating to your columns to complete the decision table. For details, see Adding rows and defining rules in guided decision tables.

19.4.4. Types of columns in guided decision tables
The Add a new column wizard for guided decision tables provides the following column options. (Select Include advanced options to view all options.)
These column types and the parameters required for each in the Add a new column wizard are described in the sections that follow.
IMPORTANT: Required data objects for column parameters
Some of the column parameters described in this section, such as Fact Patterns and Fields, provide drop-down options consisting only of data objects already defined within the same package where the guided decision table is found. Available data objects for the package are listed in the Data Objects panel of the Project Explorer and in the Data Objects tab of the guided decision tables designer. You can create additional data objects within the package as needed, or import them from another package in Data Objects → New item of the guided decision tables designer. For details about creating data objects, see Data Modeller. |
19.4.4.1. "Add a Condition"
Conditions represent fact patterns defined in the left ("WHEN") portion of a rule. With this column option, you can define one or more condition columns that check for the presence or absence of data objects with certain field values, and that affect the action ("THEN") portion of the rule. You can define a binding for the fact in the condition table, or select one that has previously been defined. You can also choose to negate the pattern.
Example:
when
$i : IncomeSource( type == "Asset" ) // Binds the IncomeSource object to the $i variable
then
...
end
when
not IncomeSource( type == "Asset" ) // Negates matching pattern
then
...
end
After a binding is specified, you can define field constraints. If two or more columns are defined using the same fact pattern binding, the field constraints become composite field constraints on the same pattern. If you define multiple bindings for a single model class, each binding becomes a separate model class in the condition ("WHEN") side of the rule.
The following parameters are required in the Add a new column wizard to set up this column type:
-
Pattern: Select from the list of fact patterns already used in conditions in your table or create a new fact pattern. A fact pattern is a combination of an available data object in the package (see the note on Required data objects for details) and a model class binding that you specify. (Examples:
LoanApplication [application]
orIncomeSource [income]
where the bracketed portion is the binding to the given fact type) -
Entry point: Define the entry point for the fact pattern, if applicable. An entry point is a gate or stream through which facts enter the Drools engine, if specified. (Examples:
Application Stream
,Credit Check Stream
) -
Calculation type: Select one of the following calculation types:
-
Literal value: The value in the cell will be compared with the field using the operator.
-
Formula: The expression in the cell will be evaluated and then compared with the field.
-
Predicate: No field is needed; the expression will be evaluated to
true
orfalse
.
-
-
Field: Select a field from the previously specified fact pattern. The field options are defined in the fact file in the Data Objects panel of your project. (Examples:
amount
orlengthYears
fields within theLoanApplication
fact type) -
Binding (optional): Define a binding for the previously selected field, if needed. (Example: For pattern
LoanApplication [application]
, fieldamount
, and operatorequal to
, if binding is set to$amount
, the end condition will beapplication : LoanAppplication($amount : amount == [value])
.) -
Operator: Select the operator to be applied to the fact pattern and field previously specified.
-
Value list (optional): Enter a list of value options, delimited by a comma and space, to limit table input data for the condition ("WHEN") portion of the rule. When this value list is defined, the values will be provided in the table cells for that column as a drop-down list, from which users can select only one option. (Example list:
Monday, Wednesday, Friday
to specify only these three options) -
Default value (optional): Select one of the previously defined value options as the default value that will appear in the cell automatically in a new row. If the default value is not specified, the table cell will be blank by default. You can also select a default value from any previously configured data enumerations in the project, listed in the Enumeration Definitions panel of the Project Explorer. (You can create enumerations in Menu → Design → Projects → [select project] → Add Asset → Enumeration.)
-
Header (description): Add header text for the column.
-
Hide column: Select this to hide the column, or clear this to display the column.
Inserting an any other
value in condition column cells
For simple condition columns in guided decision tables, you can apply an any other
value to table cells within the column if the following parameters are set:
-
Calculation type for the condition column has been set to
Literal value
. -
Operator has been set as equality
==
or inequality!=
.
The any other
value enables a rule to be defined for any other field values not explicitly defined in the rules already in the table.
Example:
when
IncomeSource( type not in ("Asset", "Job") )
...
then
...
end
-
Select a cell of a condition column that uses the
==
or!=
operator. -
In the upper-right toolbar of the table designer, click Edit → Insert "any other" value.
19.4.4.2. "Add a Condition BRL fragment"
A Business Rule Language (BRL) fragment is a section of a rule created using the guided rules designer. The condition BRL fragment is the "WHEN" portion of the rule, and the action BRL fragment is the "THEN" portion of the rule. With this column option, you can define a condition BRL fragment to be used in the left ("WHEN") side of a rule. Simpler column types can refer to Facts and Fact fields bound in the BRL fragment and vice-versa.
Example condition BRL fragment for a loan application:
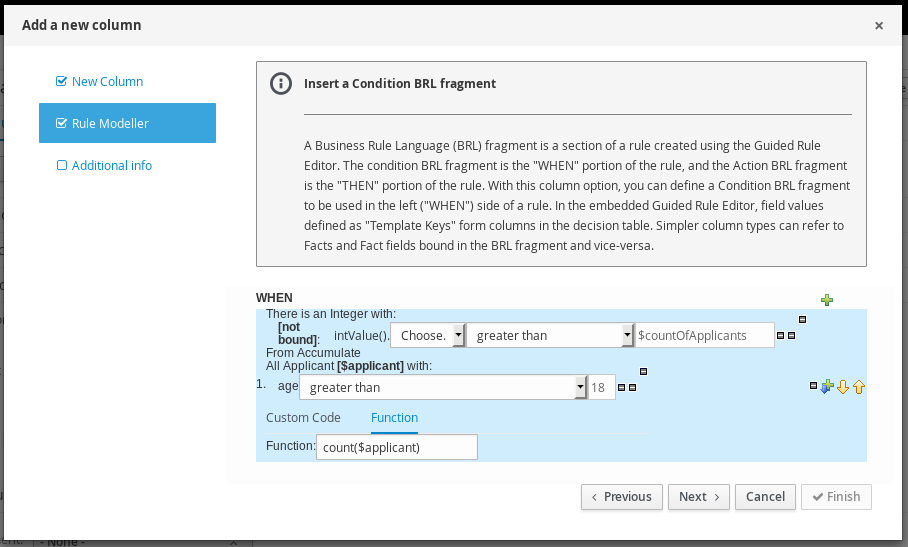
You can also select Free form DRL from the list of condition options to define the condition BRL fragment without the embedded guided rules designer.
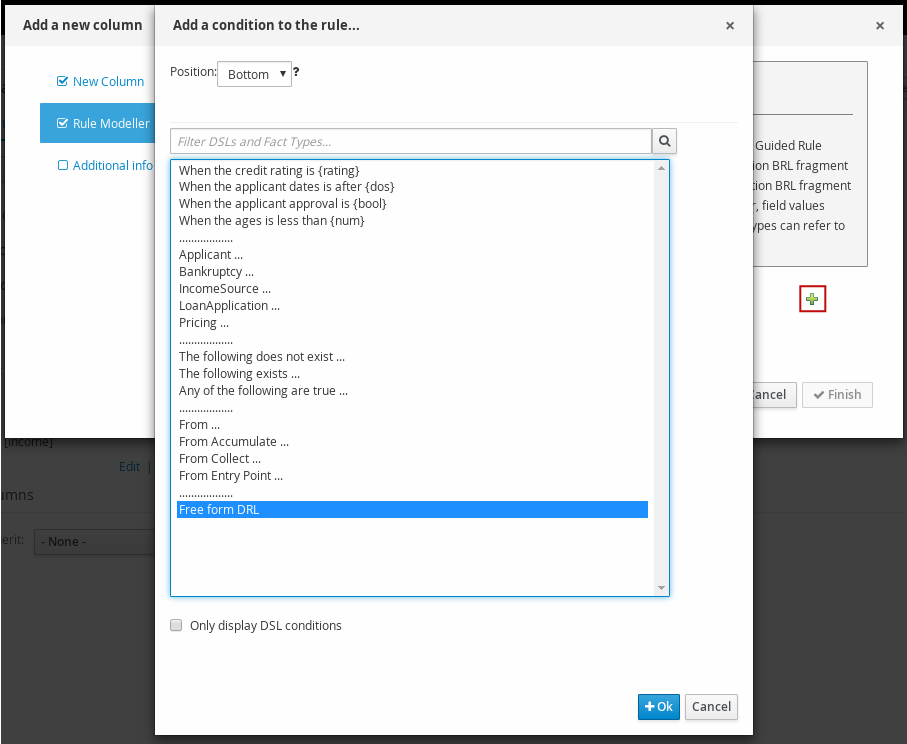
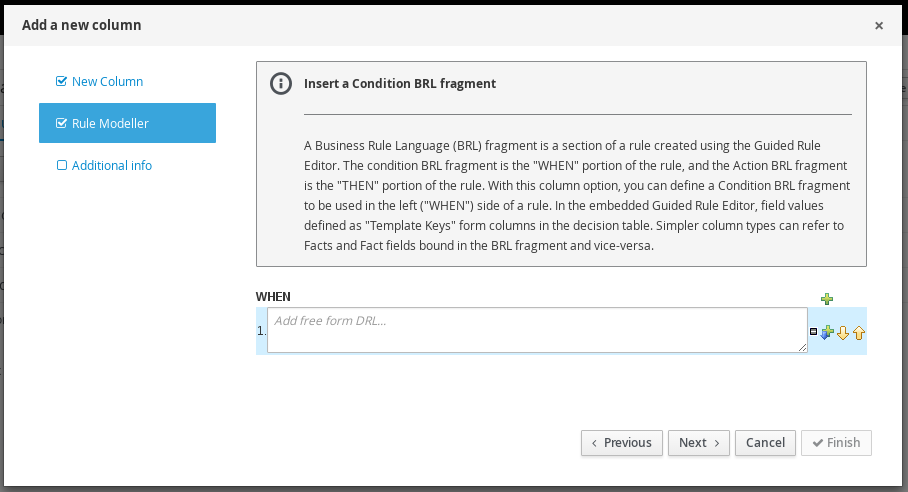
Template keys
When you add a field for a condition BRL fragment, one of the value options is Template key (as opposed to Literal or Formula). Template keys are placeholder variables that are interchanged with a specified value when the guided decision table is generated, and form separate columns in the table for each template key value specified. While Literal and Formula values are static in a decision table, Template key values can be modified as needed. In the embedded guided rules designer, you can add a template key value to a field by selecting the Template key field option and entering the value in the editor in the format In free form DRL, you can add a template key value to facts in the format The data type is String for new columns added using template keys. |
The following parameters are required in the Add a new column wizard to set up this column type:
-
Rule Modeller: Define the condition BRL fragment ("WHEN" portion) for the rule.
-
Header (description): Add header text for the column.
-
Hide column: Select this to hide the column, or clear this to display the column.
19.4.4.3. "Add a Metadata column"
With this column option, you can define a metadata element as a column in your decision table. Each column represents the normal metadata annotation in DRL rules. By default, the metadata column is hidden. To display the column, click Edit Columns in the guided decision tables designer and clear the Hide column check box.
The following parameter is required in the Add a new column wizard to set up this column type:
-
Metadata: Enter the name of the metadata item in Java variable form (that is, it cannot start with a number or contain spaces or special characters).
19.4.4.4. "Add an Action BRL fragment"
A Business Rule Language (BRL) fragment is a section of a rule created using the guided rules designer. The condition BRL fragment is the "WHEN" portion of the rule, and the action BRL fragment is the "THEN" portion of the rule. With this column option you can define an action BRL fragment to be used in the right ("THEN") side of a rule. Simpler column types can refer to Facts and Fact fields bound in the BRL fragment and vice-versa.
Example action BRL fragment for a loan application:
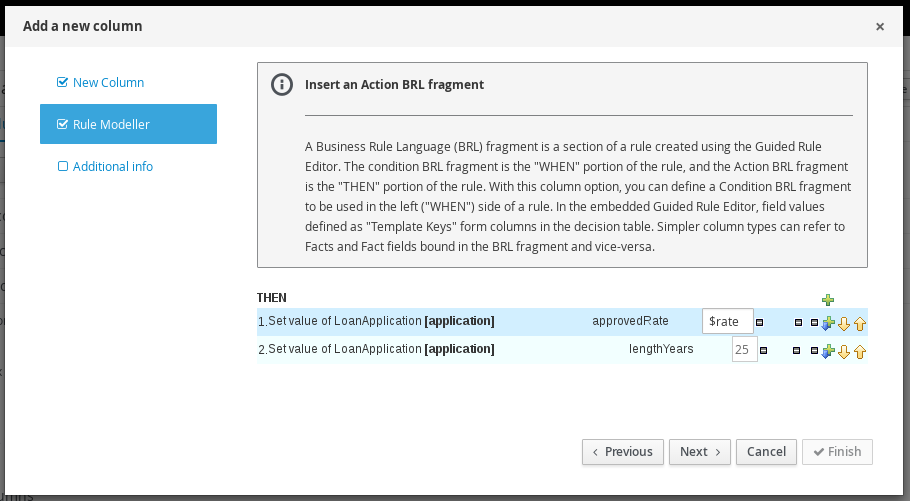
You can also select Add free form DRL from the list of action options to define the action BRL fragment without the embedded guided rules designer.
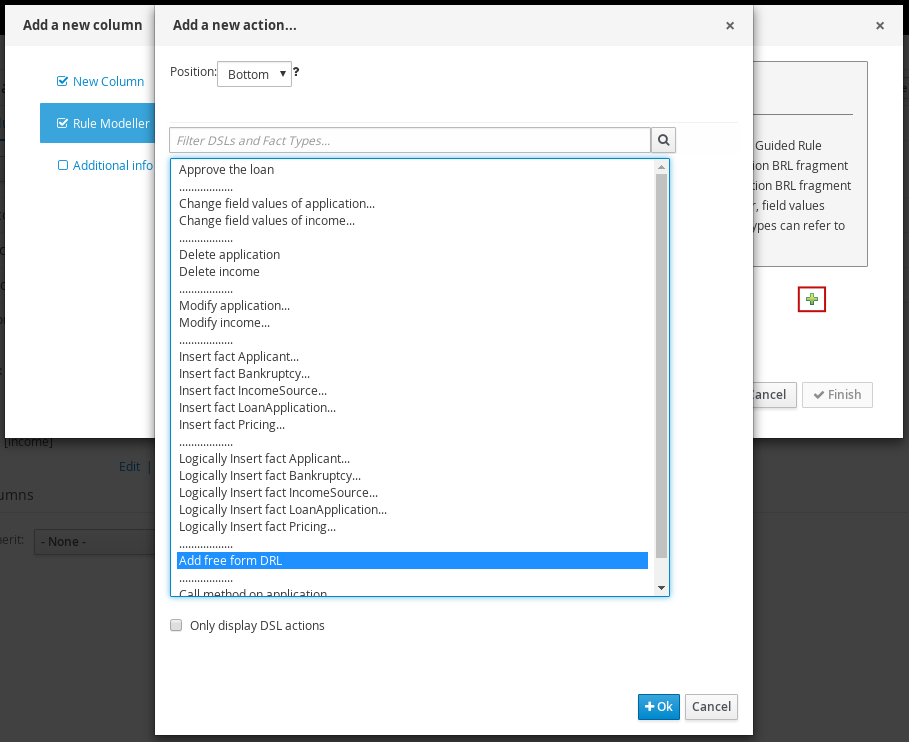
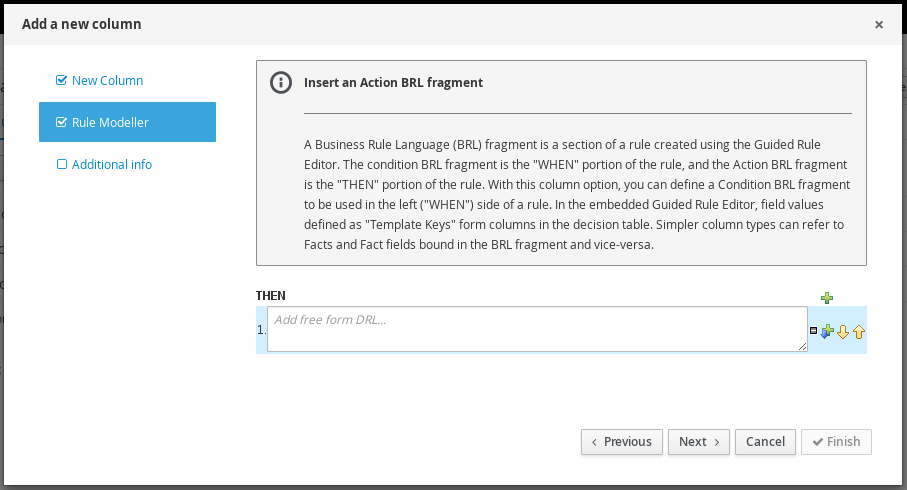
Template keys
When you add a field for an action BRL fragment, one of the value options is Template key (as opposed to Literal or Formula). Template keys are placeholder variables that are interchanged with a specified value when the guided decision table is generated, and form separate columns in the table for each template key value specified. While Literal and Formula values are static in a decision table, Template key values can be modified as needed. In the embedded guided rules designer, you can add a template key value to a field by selecting the Template key field option and entering the value in the editor in the format In free form DRL, you can add a template key value to facts in the format The data type is String for new columns added using template keys. |
The following parameters are required in the Add a new column wizard to set up this column type:
-
Rule Modeller: Define the action BRL fragment ("THEN" portion) for the rule.
-
Header (description): Add header text for the column.
-
Hide column: Select this to hide the column, or clear this to display the column.
19.4.4.5. "Add an Attribute column"
With this column option, you can add one or more attribute columns representing any of the DRL rule attributes, such as Saliance, Enabled, Date-Effective, and others.
Example:
rule "Rule1"
salience 100 // This rule has the highest priority
when
$i : IncomeSource( type == "Asset" )
then
...
end
For descriptions of each attribute, select the attribute from the list in the wizard.
Hit policies and attributes
Note that depending on the hit policy that you have defined for the decision table, some attributes may be disabled because they are internally used by the hit policy. For example, if you have assigned the Resolved Hit policy to this table so that rows (rules) are applied according to a priority order specified in the table, then the Salience attribute would be obsolete. The reason is that the Salience attribute escalates rule priority according to a defined salience value, and that value would be overridden by the Resolved Hit policy in the table. |
The following parameter is required in the Add a new column wizard to set up this column type:
-
Attribute: Select the attribute to be applied to the column.
19.4.4.6. "Delete an existing fact"
With this column option, you can implement an action to delete a fact that was added previously as a fact pattern in the table. When this column is created, the fact types are provided in the table cells for that column as a drop-down list, from which users can select only one option.
The following parameters are required in the Add a new column wizard to set up this column type:
-
Header (description): Add header text for the column.
-
Hide column: Select this to hide the column, or clear this to display the column.
19.4.4.7. "Execute a Work Item"
With this column option, you can execute a work item handler, based on your predefined work item definitions in Business Central. (You can create work items in Menu → Design → Projects → [select project] → Add Asset → Work Item definition.)
The following parameters are required in the Add a new column wizard to set up this column type:
-
Work Item: Select from the list of your predefined work items.
-
Header (description): Add header text for the column.
-
Hide column: Select this to hide the column, or clear this to display the column.
19.4.4.8. "Set the value of a field"
With this column option, you can implement an action to set the value of a field on a previously bound fact for the "THEN" portion of the rule. You have the option to notify the Drools engine of the modified values which could lead to other rules being reactivated.
The following parameters are required in the Add a new column wizard to set up this column type:
-
Pattern: Select from the list of fact patterns already used in conditions or condition BRL fragments in your table or create a new fact pattern. A fact pattern is a combination of an available data object in the package (see the note on Required data objects for details) and a model class binding that you specify. (Examples:
LoanApplication [application]
orIncomeSource [income]
where the bracketed portion is the binding to the given fact type) -
Field: Select a field from the previously specified fact pattern. The field options are defined in the fact file in the Data Objects panel of your project. (Examples:
amount
orlengthYears
fields within theLoanApplication
fact type) -
Value list (optional): Enter a list of value options, delimited by a comma and space, to limit table input data for the action ("THEN") portion of the rule. When this value list is defined, the values will be provided in the table cells for that column as a drop-down list, from which users can select only one option. (Example list:
Accepted, Declined, Pending
) -
Default value (optional): Select one of the previously defined value options as the default value that will appear in the cell automatically in a new row. If the default value is not specified, the table cell will be blank by default. You can also select a default value from any previously configured data enumerations in the project, listed in the Enumeration Definitions panel of the Project Explorer. (You can create enumerations in Menu → Design → Projects → [select project] → Add Asset → Enumeration.)
-
Header (description): Add header text for the column.
-
Hide column: Select this to hide the column, or clear this to display the column.
-
Logically insert: This option appears when the selected Fact Pattern is not currently used in another column in the guided decision table (see the next field description). Select this to insert the fact pattern logically into the Drools engine, or clear this to insert it regularly. The Drools engine is responsible for logical decisions on insertions and retractions of facts. After regular or stated insertions, facts have to be retracted explicitly. After logical insertions, facts are automatically retracted when the conditions that asserted the facts in the first place are no longer true.
-
Update engine with changes: This option appears when the selected Fact Pattern is already used in another column in the guided decision table. Select this to update the Drools engine with the modified field values, or clear this to not update the Drools engine.
19.4.4.9. "Set the value of a field with a Work Item result"
With this column option, you can implement an action to set the value of a previously defined fact field to the value of a result of a work item handler for the "THEN" portion of the rule. The work item must define a result parameter of the same data type as a field on a bound fact in order for you to set the field to the return parameter. (You can create work items in Menu → Design → Projects → [select project] → Add Asset → Work Item definition.)
An Execute a Work Item column must already be created in the table for this column option to be created.
The following parameters are required in the Add a new column wizard to set up this column type:
-
Pattern: Select from the list of fact patterns already used in your table or create a new fact pattern. A fact pattern is a combination of an available data object in the package (see the note on Required data objects for details) and a model class binding that you specify. (Examples:
LoanApplication [application]
orIncomeSource [income]
where the bracketed portion is the binding to the given fact type) -
Field: Select a field from the previously specified fact pattern. The field options are defined in the fact file in the Data Objects panel of your project. (Examples:
amount
orlengthYears
fields within theLoanApplication
fact type) -
Work Item: Select from the list of your predefined work items. (The work item must define a result parameter of the same data type as a field on a bound fact in order for you to set the field to the return parameter.)
-
Header (description): Add header text for the column.
-
Hide column: Select this to hide the column, or clear this to display the column.
-
Logically insert: This option appears when the selected Fact Pattern is not currently used in another column in the guided decision table (see the next field description). Select this to insert the fact pattern logically into the Drools engine, or clear this to insert it regularly. The Drools engine is responsible for logical decisions on insertions and retractions of facts. After regular or stated insertions, facts have to be retracted explicitly. After logical insertions, facts are automatically retracted when the conditions that asserted the facts in the first place are no longer true.
-
Update engine with changes: This option appears when the selected Fact Pattern is already used in another column in the guided decision table. Select this to update the Drools engine with the modified field values, or clear this to not update the Drools engine.
19.4.5. Editing or deleting columns in guided decision tables
You can edit or delete the columns you have created at any time in the guided decision tables designer.
-
In the guided decision tables designer, click Columns.
-
Expand the appropriate section and click Edit or Delete next to the column name.
Figure 306. Edit or delete columnsA condition column cannot be deleted if an existing action column uses the same pattern-matching parameters as the condition column. -
After any column changes, click Finish in the wizard to save.
19.4.6. Adding rows and defining rules in guided decision tables
After you have created your columns in the guided decision table, you can add rows and define rules within the guided decision tables designer.
-
Columns for the guided decision table have been added as described in Adding columns to guided decision tables.
-
In the guided decision tables designer, click Insert → Append row or one of the Insert row options. (You can also click Insert column to open the column wizard and define a new column.)
Figure 307. Add Rows -
Double-click each cell and enter data. For cells with specified values, select from the cell drop-down options.
Figure 308. Enter input data in each cell -
After you define all rows of data in the guided decision table, click Validate in the upper-right toolbar of the guided decision tables designer to validate the table. If the table validation fails, address any problems described in the error message, review all components in the table, and try again to validate the table until the table passes.
Although guided decision tables have real-time verification and validation, you should still manually validate the completed decision table to ensure optimal results. -
Click Save in the table designer to save your changes.
19.4.7. Real-time verification and validation of guided decision tables
Business Central provides a real-time verification and validation feature for guided decision tables to ensure that your tables are complete and error free. Guided decision tables are validated after each cell change. If a problem in logic is detected, an error notification appears and describes the problem.
19.4.7.1. Types of problems in guided decision tables
The validation and verification feature detects the following types of problems:
- Redundancy
-
Redundancy occurs when two rows in a decision table execute the same consequences for the same set of facts. For example, two rows checking a client’s birthday and providing a birthday discount may result in double discount.
- Subsumption
-
Subsumption is similar to redundancy and occurs when two rules execute the same consequences, but one executes on a subset of facts of the other. For example, consider these two rules:
-
when Person age > 10 then Increase Counter
-
when Person age > 20 then Increase Counter
In this case, if a person is 15 years old, only one rule fires and if a person is 20 years old, both rules fire. Such cases cause similar trouble during runtime as redundancy.
-
- Conflicts
-
A conflicting situation occurs when two similar conditions have different consequences. Conflicts can occur between two rows (rules) or two cells in a decision table.
The following example illustrates conflict between two rows in a decision table:
-
when Deposit > 20000 then Approve Loan
-
when Deposit > 20000 then Refuse Loan
In this case, there is no way to know if the loan will be approved or not.
The following example illustrates conflict between two cells in a decision table:
-
when Age > 25
-
when Age < 25
A row with conflicting cells never executes.
-
- Broken Unique Hit Policy
-
When the Unique Hit policy is applied to a decision table, only one row at a time can be executed and each row must be unique, with no overlap of conditions being met. If more than one row is executed, then the verification report identifies the broken hit policy. For example, consider the following conditions in a table that determines eligibility for a price discount:
-
when Is Student = true
-
when Is Military = true
If a customer is both a student and in the military, both conditions apply and break the Unique Hit policy. Rows in this type of table must therefore be created in a way that does not allow multiple rules to fire at one time. For details about hit policies, see Hit policies for guided decision tables.
-
- Deficiency
-
Deficiency is similar to a conflict and occurs the logic of a rule in a decision table is incomplete. For example, consider the following two deficient rules:
-
when Age > 20 then Approve Loan
-
when Deposit < 20000 then Refuse Loan
These two rules may lead to confusion for a person who is over 20 years old and has deposited less than 20000. You can add more constraints to avoid the conflict.
-
- Missing Columns
-
When deleted columns result in incomplete or incorrect logic, rules cannot fire properly. This is detected so that you can address the missing columns, or adjust the logic to not rely on intentionally deleted conditions or actions.
- Incomplete Ranges
-
Ranges of field values are incomplete if a table contains constraints against possible field values but does not define all possible values. The verification report identifies any incomplete ranges provided. For example, if your table has a check for if an application is approved, the verification report reminds you to make sure you also handle situations where the application was not approved.
19.4.7.2. Types of notifications
The verification and validation feature uses three types of notifications:
-
Error: A serious problem that may lead to the guided decision table failing to work as designed at run time. Conflicts, for example, are reported as errors.
-
Warning: Likely a serious problem that may not prevent the guided decision table from working but requires attention. Subsumptions, for example, are reported as warnings.
-
Information: A moderate or minor problem that may not prevent the guided decision table from working but requires attention. Missing columns, for example, are reported as information.
Business Central verification and validation does not prevent you from saving an incorrect change. The feature only reports issues while editing and you can still continue to overlook those and save your changes. |
19.4.7.3. Disabling verification and validation of guided decision tables
The decision table verification and validation feature of Business Central is enabled by default. You can disable it by setting the org.kie.verification.disable-dtable-realtime-verification
system property value to true
in your Red Hat JBoss EAP directory.
Navigate to $EAP_HOME/standalone/configuration/standalone-full.xml
and add the following system property:
<property name="org.kie.verification.disable-dtable-realtime-verification" value="true"/>
19.5. Guided decision table graphs
Whilst it is possible to author single Guided Decision Tables it is also possible to author a graph of related tables where an action of one may provide a potential match for a condition of another. In this scenario the tables are said to be related.
19.5.1. Editor
The editor itself preserves all the features of the Guided Decision Table Editor merely adding the ability to visualise multiple tables and their relationships in a single view. Selecting a single table leads to the column definitions panel, Source and Data Objects tabs being updated to reflect the selected table. Validation & Verification reporting is also updated to reflect the selected table. The Overview tab maintains state of the graph as a whole.
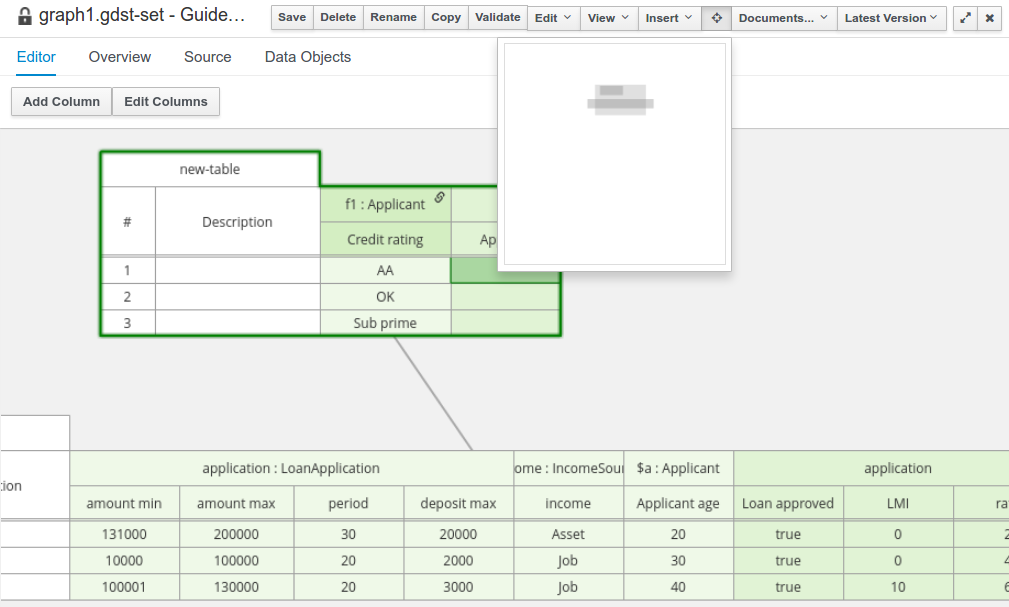
19.5.2. Creating a new graph
When a new "Guided Decision Table Graph" is first created the target package is scanned for existing single Guided Decision Tables. Those found are checked for relationships and, if any exist, added automatically to the new graph.
19.5.3. Adding new tables to the graph
Tables can be added to the graph by clicking on the "Documents…" menu entry. This reveals a tool panel that lists the tables already part of the graph. The same panel gives the User the ability to either add a new table or open an existing table.
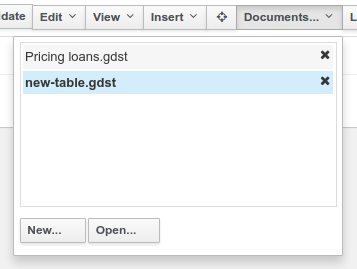
19.5.3.1. Adding new tables
Clicking on "New…" launches the New Guided Decision Table Wizard. On completion of the Wizard the new table will be added to the graph and any relationships automatically added.
19.5.3.2. Adding existing tables
Clicking on "Open…" reveals a file selector wherefrom one or more existing Guided Decision Tables can be selected and added to the graph. If no additional Guided Decision Tables are found a message is shown to the User.
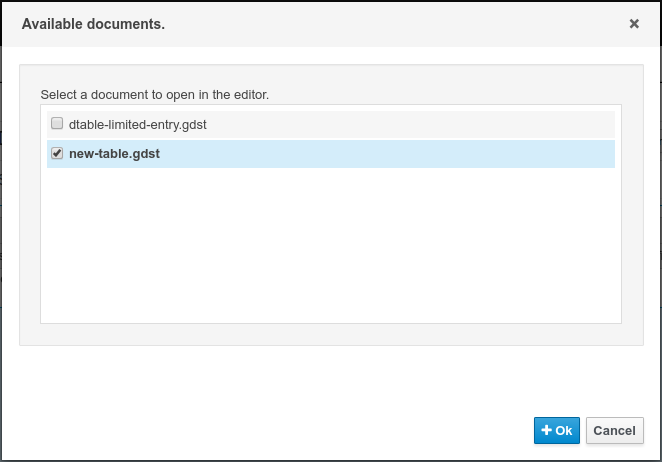
19.5.4. Removing tables
Tables can be removed from the graph by opening the "Documents…" panel and clicking on the "X" icon beside the table. Tables removed from the graph are not deleted from the Project and can continue to be edited by the Gudied Decision Table Editor.
19.6. Guided decision trees
Business Central supports authoring of simple Decision Trees.
The editor does not support nested Data Objects at present. It is therefore advised to only use Guided Decision Trees with flat Data Object models. |
19.6.1. The initial editor layout
When a new Guided Decision Tree is created the editor is initially blank.
The left-hand side is a palette of available Data Objects, their fields and Actions.
The right-hand side is the area where you can drag and drop Data Objects, their fields or Actions to build a tree.
The editor will show a connector between the node being dragged and applicable children to which it can be attached. When the drag is complete the new node will be attached to the applicable child. Root nodes will not have a connector shown when being dragged to an empty tree. Completing the drag positions the root node in the centre of the editor.
There are various restrictions when composing a tree:-
-
A tree must have a Data Object at the root.
-
A tree can only have one root.
-
Data Objects can have either other Data Objects, field constraints or Actions as children.
The field constraints must be on fields of the same Data Object as the parent node.
-
Field constraints can have either other field constraints or Actions as children.
The field constraints must be on fields of the same Data Object as the parent node.
-
Actions can only have other Actions as children.
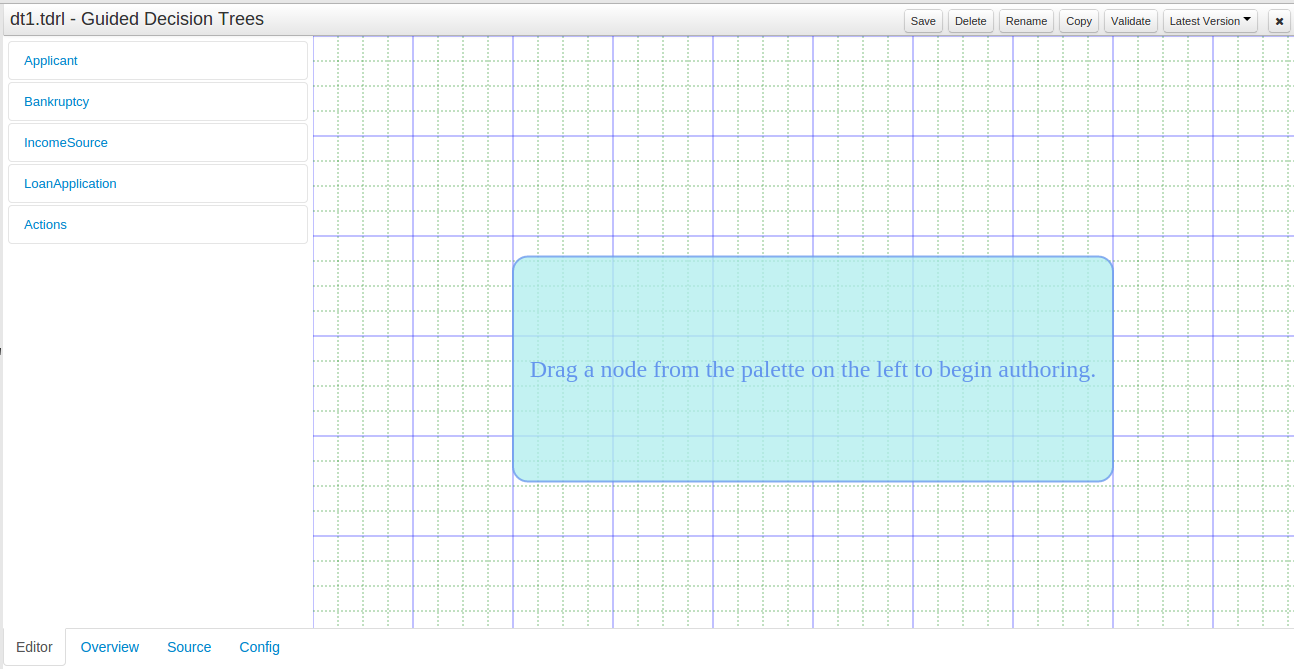
Expanding the palette reveals Tree Nodes for the Data Object and its fields.
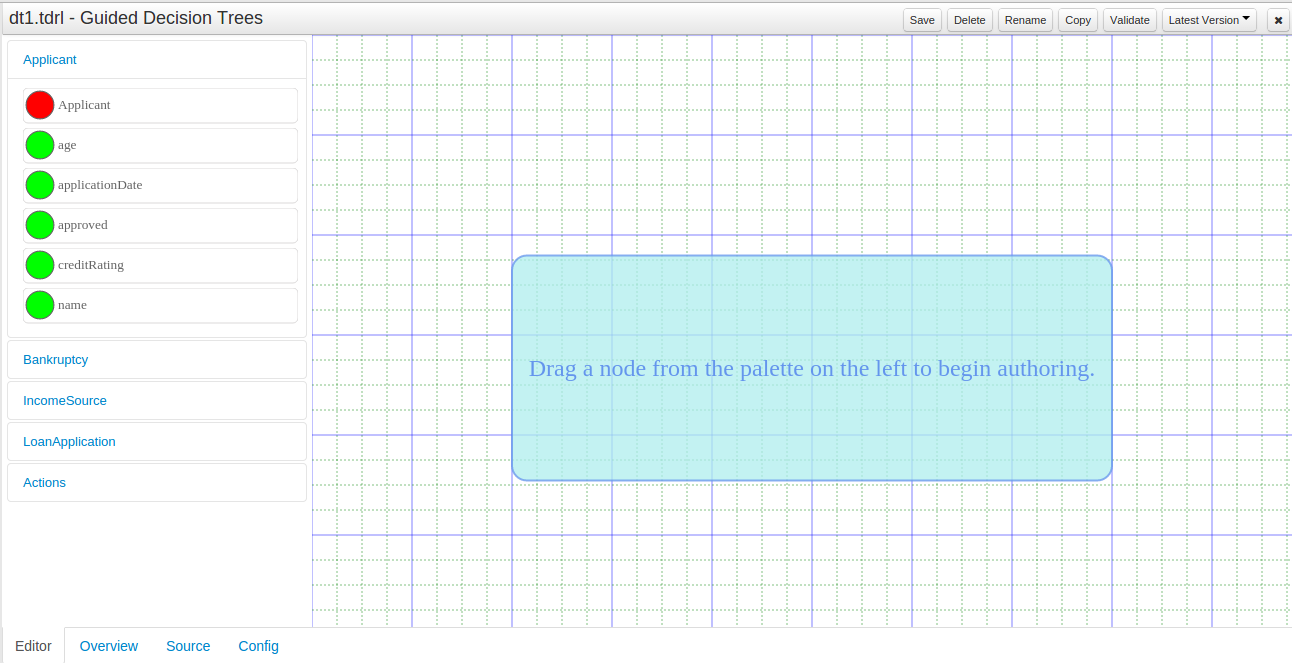
19.6.2. First steps
Drag a Data Object on to the tree authoring area.
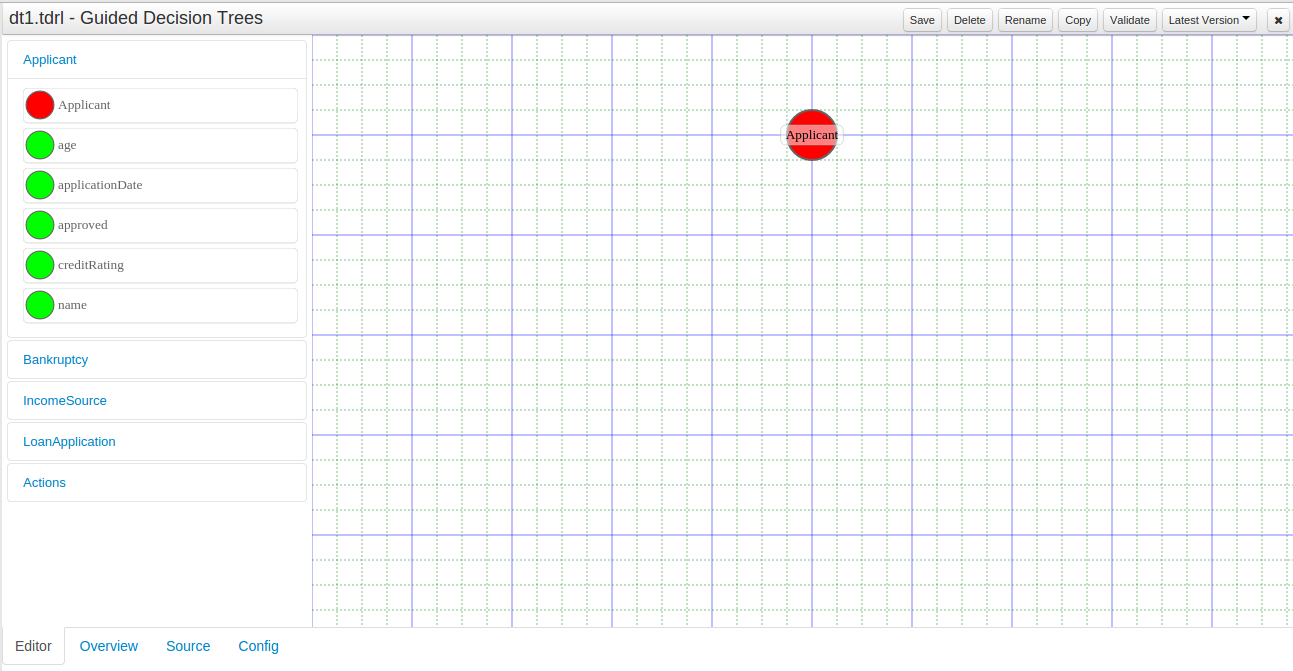
Clicking on a node selects it.
Icons to manipulate the node appear when the node is selected.
The icons are:
-
Delete
Deleting a node will also delete all children.
-
Edit
Collapsed nodes cannot be edited as they contain numerous children.
-
Collapse
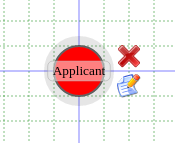
19.6.3. Editing Data Object nodes
Selecting a Data Object node and clicking the edit icon shows a popup to manage the node.
The popup shows the Data Object type and allows it to be bound to a variable. Bound Data Objects can be modified or retracted by Actions.
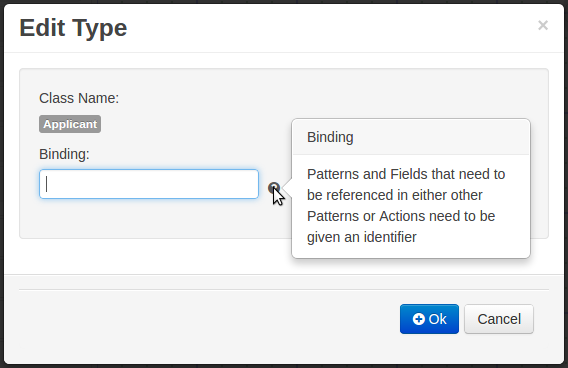
19.6.4. Editing Field Constraint nodes
Selecting a Field Constraint node and clicking the edit icon shows a popup to manage the node.
The popup shows the Data Object type and field and allows the field to be bound to a variable. An operator, applicable to the Data Model field’s data-type, can be selected and a corresponding value entered.
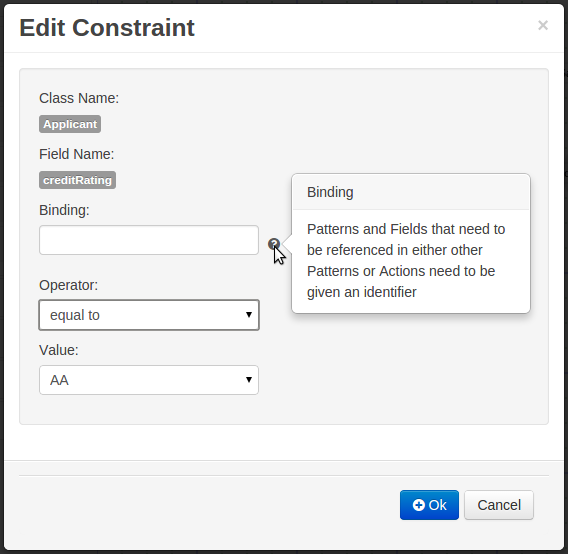
19.6.5. Editing Action nodes
Selecting an Insert Action node and clicking the edit icon shows a popup to manage the node.
The popup allows selection of the Data Object to be inserted and whether it’s insertion is "logical". Please refer to Drools documentation regarding Truth Maintenance for more information. Fields for the new Data Object can have values set.
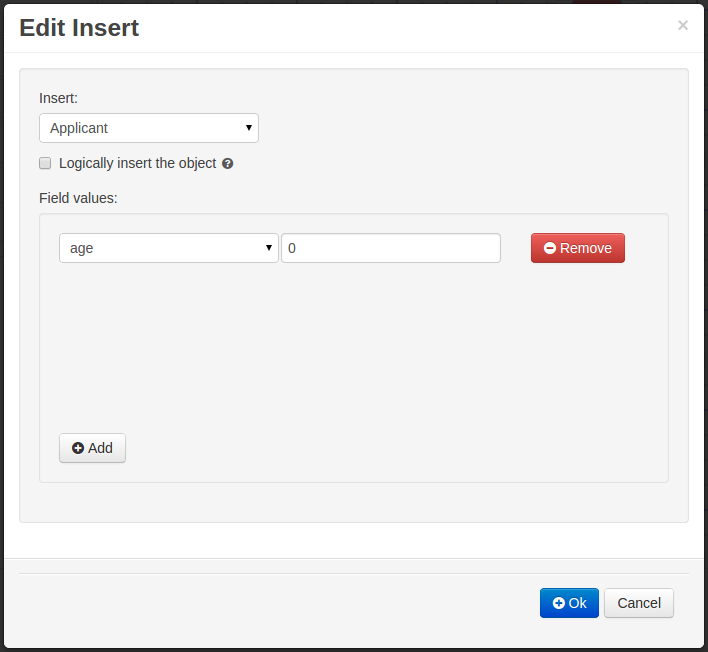
Selecting an Insert Retract node and clicking the edit icon shows a popup to manage the node.
The popup allows any Data Object bound in the path from the selected node to the root node to be selected for retraction.
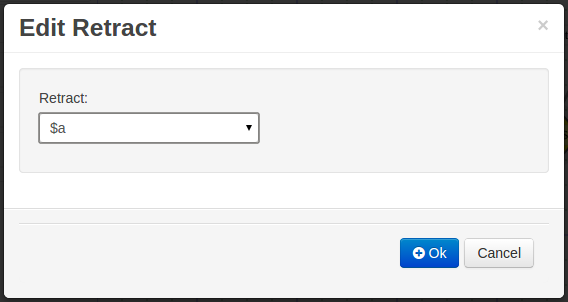
Selecting an Insert Update node and clicking the edit icon shows a popup to manage the node.
The popup allows any Data Object bound in the path from the selected node to the root node to be modified. Fields for the modified Data Object can have values set.
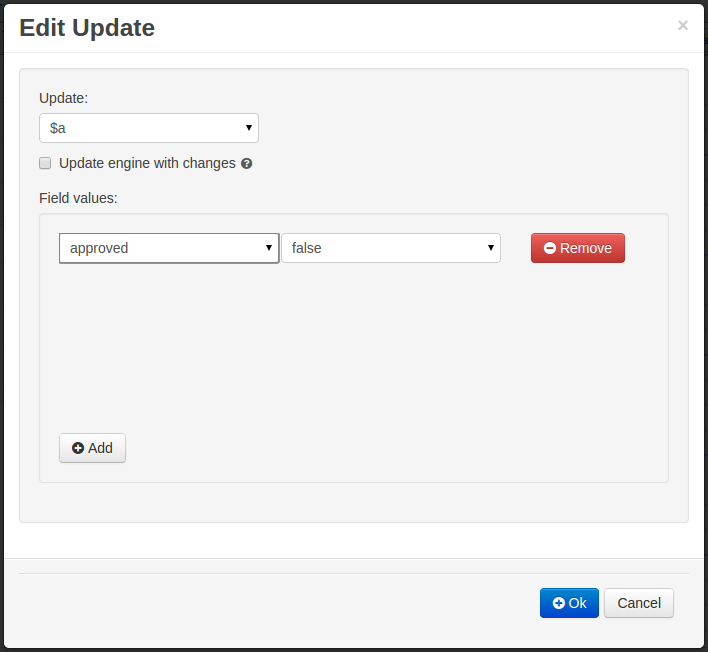
19.6.6. Managing the tree
Even simple trees can grow in size and become difficult to maintain.
It is therefore possible to collapse parts of the tree, giving more space in the user interface to maintain different parts of the tree.
If a node has children, when selected, it will have an icon to collapse the children. Clicking this icon will collapse children.
A collapsed node can equally be expanded by selecting it and clicking on the exapnd icon. A collapsed node cannot be edited as it contains numerous children. Deleting a collapsed node deletes all children too.
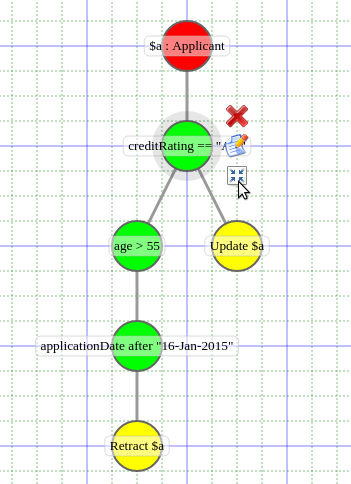
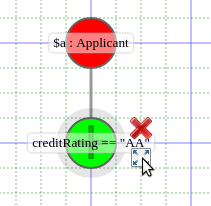
19.7. Spreadsheet decision tables
Spreadsheet decision tables are XLS or XLSX spreadsheets that contain business rules defined in a tabular format. You can include spreadsheet decision tables with standalone Drools projects or upload them to projects in Business Central. Each row in a decision table is a rule, and each column is a condition, an action, or another rule attribute. After you create and upload your spreadsheet decision tables, the rules you defined are compiled into Drools Rule Language (DRL) rules as with all other rule assets.
All data objects related to a spreadsheet decision table must be in the same project package as the spreadsheet decision table. Assets in the same package are imported by default. Existing assets in other packages can be imported with the decision table.
19.7.1. Decision table use case
An online shopping site lists the shipping charges for ordered items. The site provides free shipping under the following conditions:
-
The number of items ordered is 4 or more and the checkout total is $300 or more.
-
Standard shipping is selected (4 or 5 business days from the date of purchase).
The following are the shipping rates under these conditions:
Number of items | Delivery day | Shipping charge in USD, N = Number of items |
---|---|---|
3 or fewer |
Next day 2nd day Standard |
35 15 10 |
4 or more |
Next day 2nd day Standard |
N*7.50 N*3.50 N*2.50 |
Number of items | Delivery day | Shipping charge in USD, N = Number of items |
---|---|---|
3 or fewer |
Next day 2nd day Standard |
25 10 N*1.50 |
4 or more |
Next day 2nd day Standard |
N*5 N*2 FREE |
These conditions and rates are shown in the following example spreadsheet decision table:
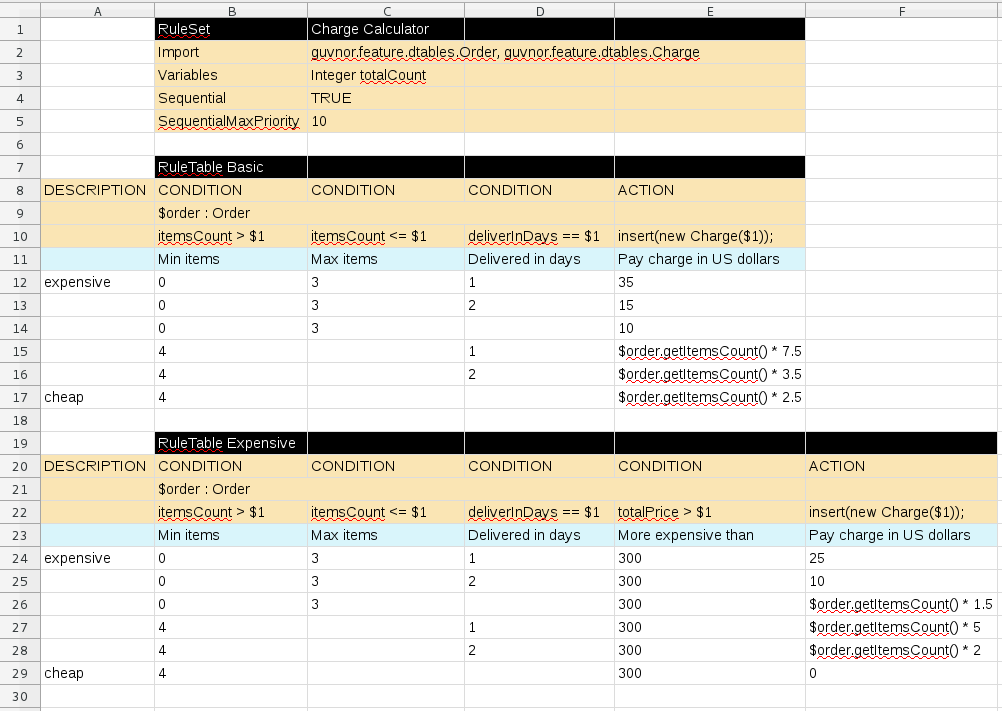
In order for a decision table to be uploaded in Business Central, the table must comply with certain structure and syntax requirements, within an XLS or XLSX spreadsheet, as shown in this example. For more information, see Defining spreadsheet decision tables.
19.7.2. Defining spreadsheet decision tables
Spreadsheet decision tables (XLS or XLSX) require two key areas that define rule data: a RuleSet
area and a RuleTable
area. The RuleSet
area of the spreadsheet defines elements that you want to apply globally to all rules in the same package (not only the spreadsheet), such as a rule set name or universal rule attributes. The RuleTable
area defines the actual rules (rows) and the conditions, actions, and other rule attributes (columns) that constitute that rule table within the specified rule set. A spreadsheet of decision tables can contain multiple RuleTable
areas, but only one RuleSet
area.
You should typically upload only one spreadsheet of decision tables, containing all necessary RuleTable definitions, per rule package in Business Central. You can upload separate decision table spreadsheets for separate packages, but uploading multiple spreadsheets in the same package can cause compilation errors from conflicting RuleSet or RuleTable attributes and is therefore not recommended.
|
Refer to the following sample spreadsheet as you define your decision table:
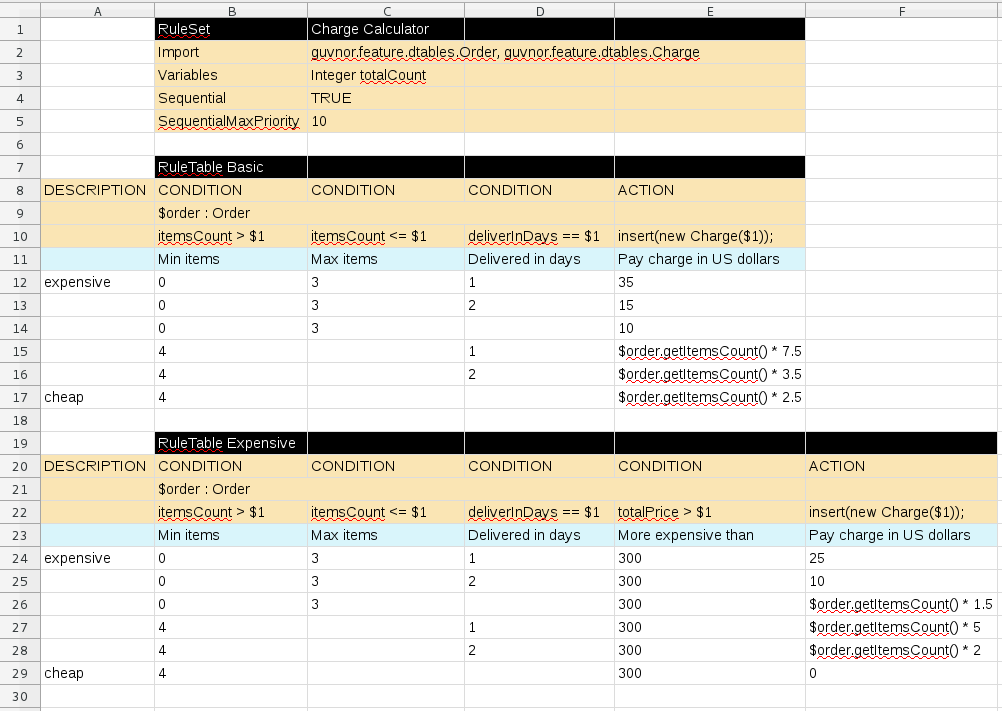
-
In a new XLS or XLSX spreadsheet, go to the second or third column and label a cell
RuleSet
(row 1 in example). Reserve the column or columns to the left for descriptive metadata (optional). -
In the next cell to the right, enter a name for the
RuleSet
. This named rule set will contain allRuleTable
rules defined in the rule package. -
Under the
RuleSet
cell, define any rule attributes (one per cell) that you want to apply globally to all rule tables in the package. Specify attribute values in the cells to the right. For example, you can enter anImport
label and in the cell to the right, specify relevant data objects from other packages that you want to import into the package for the decision table (in the formatpackage.name.object.name
). For supported cell labels and values, see RuleSet definitions. -
Below the
RuleSet
area and in the same column as theRuleSet
cell, skip a row and label a new cellRuleTable
(row 7 in example) and enter a table name in the same cell. The name is used as the initial part of the name for all rules derived from this rule table, with the row number appended for distinction. You can override this automatic naming by inserting aNAME
attribute column. -
Use the next four rows to define the following elements as needed (rows 8-11 in example):
-
Rule attributes: Conditions, actions, or other attributes. For supported cell labels and values, see RuleTable definitions.
-
Object types: The data objects to which the rule attributes apply. If the same object type applies to multiple columns, merge the object cells into one cell across multiple columns (as shown in the sample decision table), instead of repeating the object type in multiple cells. When an object type is merged, all columns below the merged range will be combined into one set of constraints within a single pattern for matching a single fact at a time. When an object is repeated in separate columns, the separate columns can create different patterns, potentially matching different or identical facts.
-
Constraints: Constraints on the object types.
-
Column label: (Optional) Any descriptive label for the column, as a visual aid. Leave blank if unused.
As an alternative to populating both the object type and constraint cells, you can leave the object type cell or cells empty and enter the full expression in the corresponding constraint cell or cells. For example, instead of Order
as the object type anditemsCount > $1
as a constraint (separate cells), you can leave the object type cell empty and enterOrder( itemsCount > $1 )
in the constraint cell, and then do the same for other constraint cells.
-
-
After you have defined all necessary rule attributes (columns), enter values for each column as needed, row by row, to generate rules (rows 12-17 in example). Cells with no data are ignored (such as when a condition or action does not apply).
If you need to add more rule tables to this decision table spreadsheet, skip a row after the last rule in the previous table, label another
RuleTable
cell in the same column as the previousRuleTable
andRuleSet
cells, and create the new table following the same steps in this section (rows 19-29 in example). -
Save your XLS or XLSX spreadsheet to finish.
Only the first worksheet in a spreadsheet workbook will be processed as a decision table when you upload the spreadsheet in Business Central. Each RuleSet name combined with the RuleTable name must be unique across all decision table files in the same package.
|
After you upload the decision table in Business Central, the rules are rendered as DRL rules like the following example, from the sample spreadsheet:
//row 12 rule "Basic_12" salience 10 when $order : Order( itemsCount > 0, itemsCount <= 3, deliverInDays == 1 ) then insert( new Charge( 35 ) ); end
Enabling white space used in cell values
By default, any white space before or after values in decision table cells is removed before the decision table is processed by the Drools engine. To retain white space that you use intentionally before or after values in cells, set the |
19.7.2.1. RuleSet definitions
Entries in the RuleSet
area of a decision table define DRL constructs and rule attributes that you want to apply to all rules in a package (not only in the spreadsheet). Entries must be in a vertically stacked sequence of cell pairs, where the first cell contains a label and the cell to the right contains the value. A decision table spreadsheet can have only one RuleSet
area.
The following table lists the supported labels and values for RuleSet
definitions:
Label | Value | Usage |
---|---|---|
|
The package name for the generated DRL file. Optional, the default is |
Must be the first entry. |
|
|
Optional, at most once. If omitted, no firing order is imposed. |
|
Integer numeric value |
Optional, at most once. In sequential mode, this option is used to set the start value of the salience. If omitted, the default value is 65535. |
|
Integer numeric value |
Optional, at most once. In sequential mode, this option is used to check if this minimum salience value is not violated. If omitted, the default value is 0. |
|
|
Optional, at most once. If omitted, quotation marks are escaped. |
|
A comma-separated list of Java classes to import from another package. |
Optional, may be used repeatedly. |
|
Declarations of DRL globals (a type followed by a variable name). Multiple global definitions must be separated by commas. |
Optional, may be used repeatedly. |
|
One or more function definitions, according to DRL syntax. |
Optional, may be used repeatedly. |
|
One or more query definitions, according to DRL syntax. |
Optional, may be used repeatedly. |
|
One or more declarative types, according to DRL syntax. |
Optional, may be used repeatedly. |
In some cases, Microsoft Office, LibreOffice, and OpenOffice might encode a double quotation mark differently, causing a compilation error. For example, “A” will fail, but "A" will pass.
|
19.7.2.2. RuleTable definitions
Entries in the RuleTable
area of a decision table define conditions, actions, and other rule attributes for the rules in that rule table. A spreadsheet of decision tables can contain multiple RuleTable
areas.
The following table lists the supported labels (column headers) and values for RuleTable
definitions. For column headers, you can use either the given labels or any custom labels that begin with the letters listed in the table.
Label | Or custom label that begins with | Value | Usage |
---|---|---|---|
|
N |
Provides the name for the rule generated from that row. The default is constructed from the text following the |
At most one column. |
|
I |
Results in a comment within the generated rule. |
At most one column. |
|
C |
Code snippet and interpolated values for constructing a constraint within a pattern in a condition. |
At least one per rule table. |
|
A |
Code snippet and interpolated values for constructing an action for the consequence of the rule. |
At least one per rule table. |
|
@ |
Code snippet and interpolated values for constructing a metadata entry for the rule. |
Optional, any number of columns. |
The following sections provide more details about how condition, action, and metadata columns use cell data:
- Conditions
-
For columns headed
CONDITION
, the cells in consecutive lines result in a conditional element:-
First cell: Text in the first cell below
CONDITION
develops into a pattern for the rule condition, and uses the snippet in the next line as a constraint. If the cell is merged with one or more neighboring cells, a single pattern with multiple constraints is formed. All constraints are combined into a parenthesized list and appended to the text in this cell.If this cell is empty, the code snippet in the cell below it must result in a valid conditional element on its own. For example, instead of
Order
as the object type anditemsCount > $1
as a constraint (separate cells), you can leave the object type cell empty and enterOrder( itemsCount > $1 )
in the constraint cell, and then do the same for any other constraint cells.To include a pattern without constraints, you can write the pattern in front of the text of another pattern, with or without an empty pair of parentheses. You can also append a
from
clause to the pattern.If the pattern ends with
eval
, code snippets produce boolean expressions for inclusion into a pair of parentheses aftereval
. -
Second cell: Text in the second cell below
CONDITION
is processed as a constraint on the object reference in the first cell. The code snippet in this cell is modified by interpolating values from cells farther down in the column. If you want to create a constraint consisting of a comparison using==
with the value from the cells below, then the field selector alone is sufficient. Any other comparison operator must be specified as the last item within the snippet, and the value from the cells below is appended. For all other constraint forms, you must mark the position for including the contents of a cell with the symbol$param
. Multiple insertions are possible if you use the symbols$1
,$2
, and so on, and a comma-separated list of values in the cells below. However, do not separate$1
,$2
, and so on, by commas, or the table will fail to process.To expand a text according to the pattern
forall($delimiter){$snippet}
, repeat the$snippet
once for each of the values of the comma-separated list in each of the cells below, insert the value in place of the symbol$
, and join these expansions by the given$delimiter
. Note that theforall
construct may be surrounded by other text.If the first cell contains an object, the completed code snippet is added to the conditional element from that cell. A pair of parentheses is provided automatically, as well as a separating comma if multiple constraints are added to a pattern in a merged cell. If the first cell is empty, the code snippet in this cell must result in a valid conditional element on its own. For example, instead of
Order
as the object type anditemsCount > $1
as a constraint (separate cells), you can leave the object type cell empty and enterOrder( itemsCount > $1 )
in the constraint cell, and then do the same for any other constraint cells. -
Third cell: Text in the third cell below
CONDITION
is a descriptive label that you define for the column, as a visual aid. -
Fourth cell: From the fourth row on, non-blank entries provide data for interpolation. A blank cell omits the condition or constraint for this rule.
-
- Actions
-
For columns headed
ACTION
, the cells in consecutive lines result in an action statement:-
First cell: Text in the first cell below
ACTION
is optional. If present, the text is interpreted as an object reference. -
Second cell: Text in the second cell below
ACTION
is a code snippet that is modified by interpolating values from cells farther down in the column. For a singular insertion, mark the position for including the contents of a cell with the symbol$param
. Multiple insertions are possible if you use the symbols$1
,$2
, and so on, and a comma-separated list of values in the cells below. However, do not separate$1
,$2
, and so on, by commas, or the table will fail to process.A text without any marker symbols can execute a method call without interpolation. In this case, use any non-blank entry in a row below the cell to include the statement. The
forall
construct is supported.If the first cell contains an object, then the cell text (followed by a period), the text in the second cell, and a terminating semicolon are strung together, resulting in a method call that is added as an action statement for the consequence. If the first cell is empty, the code snippet in this cell must result in a valid action element on its own.
-
Third cell: Text in the third cell below
ACTION
is a descriptive label that you define for the column, as a visual aid. -
Fourth cell: From the fourth row on, non-blank entries provide data for interpolation. A blank cell omits the condition or constraint for this rule.
-
- Metadata
-
For columns headed
METADATA
, the cells in consecutive lines result in a metadata annotation for the generated rules:-
First cell: Text in the first cell below
METADATA
is ignored. -
Second cell: Text in the second cell below
METADATA
is subject to interpolation, using values from the cells in the rule rows. The metadata marker character@
is prefixed automatically, so you do not need to include that character in the text for this cell. -
Third cell: Text in the third cell below
METADATA
is a descriptive label that you define for the column, as a visual aid. -
Fourth cell: From the fourth row on, non-blank entries provide data for interpolation. A blank cell results in the omission of the metadata annotation for this rule.
-
19.7.2.3. Additional rule attributes for RuleSet or RuleTable definitions
The RuleSet
and RuleTable
areas also support labels and values for other rule attributes, such as PRIORITY
or NO-LOOP
. Rule attributes specified in a RuleSet
area will affect all rule assets in the same package (not only in the spreadsheet). Rule attributes specified in a RuleTable
area will affect only the rules in that rule table. You can use each rule attribute only once in a RuleSet
area and once in a RuleTable
area. If the same attribute is used in both RuleSet
and RuleTable
areas within the spreadsheet, then RuleTable
takes priority and the attribute in the RuleSet
area is overridden.
The following table lists the supported labels (column headers) and values for additional RuleSet
or RuleTable
definitions. For column headers, you can use either the given labels or any custom labels that begin with the letters listed in the table.
Label | Or custom label that begins with | Value |
---|---|---|
|
P |
An integer defining the Example: |
|
V |
A string containing a date and time definition. The rule can be activated only if the current date and time is after a Example: |
|
Z |
A string containing a date and time definition. The rule cannot be activated if the current date and time is after the Example: |
|
U |
A Boolean value. When this option is set to Example: |
|
G |
A string identifying an agenda group to which you want to assign the rule. Agenda groups allow you to partition the agenda to provide more execution control over groups of rules. Only rules in an agenda group that has acquired a focus are able to be activated. Example: |
|
X |
A string identifying an activation (or XOR) group to which you want to assign the rule. In activation groups, only one rule can be activated. The first rule to fire will cancel all pending activations of all rules in the activation group. Example: |
|
D |
A long integer value defining the duration of time in milliseconds after which the rule can be activated, if the rule conditions are still met. Example: |
|
T |
A string identifying either Example: |
|
E |
A Quartz calendar definition for scheduling the rule. Example: |
|
F |
A Boolean value, applicable only to rules within agenda groups. When this option is set to Example: |
|
L |
A Boolean value, applicable only to rules within rule flow groups or agenda groups. When this option is set to Example: |
|
R |
A string identifying a rule flow group. In rule flow groups, rules can fire only when the group is activated by the associated rule flow. Example: |
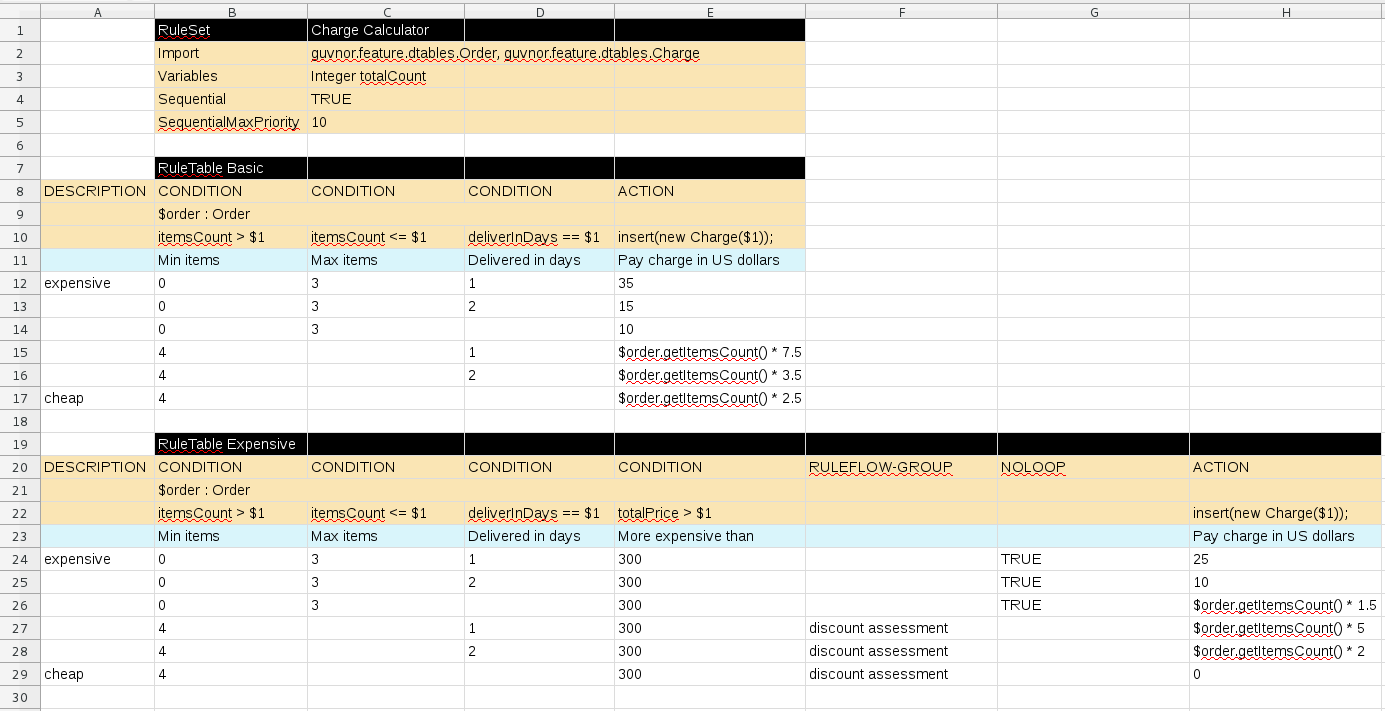
19.7.2.4. Examples of decision table data interpolation
The various interpolations of data in decision tables are illustrated in the following example.
If the template is Foo(bar == $param)
and the cell is 42
, then the result is Foo(bar == 42)
.
If the template is Foo(bar < $1, baz == $2)
and the cell contains 42,43
, the result will be Foo(bar < 42, baz ==43)
.
The template forall(&&){bar != $}
with a cell containing 42,43
results in bar != 42 && bar != 43
.
The next example demonstrates the joint effect of a cell defining the pattern type and the code snippet below it.
RuleTable Cheese fans | |
---|---|
CONDITION |
CONDITION |
Person |
|
age |
type |
Persons age |
Cheese type |
42 |
stilton |
21 |
cheddar |
This spreadsheet section shows how the Person
type declaration spans 2 columns, and thus both constraints will appear as Person(age == …, type == …)
.
Since only the field names are present in the snippet, they imply an equality test.
In the following example the marker symbol $param
is used.
CONDITION |
Person |
age == $param |
Persons age |
42 |
The result of this column is the pattern Person(age == 42))
.
You may have noticed that the marker and the operator "==" are redundant.
The next example illustrates that a trailing insertion marker can be omitted.
CONDITION |
Person |
age < |
Persons age |
42 |
Here, appending the value from the cell is implied, resulting in Person(age < 42)).
You can provide the definition of a binding variable, as in the example below.
CONDITION |
c : Cheese |
type |
Cheese type |
stilton |
Here, the result is c: Cheese(type == "stilton").
Note that the quotes are provided automatically.
Actually, anything can be placed in the object type row.
Apart from the definition of a binding variable, it could also be an additional pattern that is to be inserted literally.
A simple construction of an action statement with the insertion of a single value is shown below.
ACTION |
list.add("$param"); |
Log |
Old man stilton |
The cell below the ACTION header is left blank. Using this style, anything can be placed in the consequence, not just a single method call. (The same technique is applicable within a CONDITION column as well.)
Below is a comprehensive example, showing the use of various column headers. It is not an error to have no value below a column header (as in the NO-LOOP column): here, the attribute will not be applied in any of the rules.
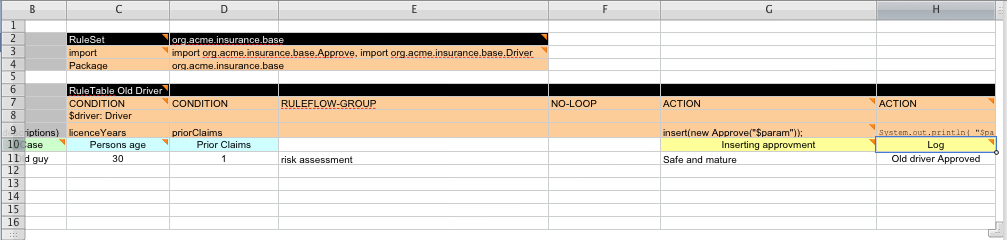
And, finally, here is an example of Import and Functions.
RuleSet |
Cheese Price |
Import |
com.sample.Cheese, com.sample.Person |
Functions |
function int computePrice(Cheese cheese) { if (cheese.getType() == "cheddar") { return 10; } else if (cheese.getType() == "stilton") { return 15; } else { return 20; } } function boolean hasDiscount(Person person) { if (person.getAge() > 60) { return true; } else { return false; } } |
Multiple package names within the same cell must be separated by a comma. Also, the pairs of type and variable names must be comma-separated. Functions, however, must be written as they appear in a DRL file. This should appear in the same column as the "RuleSet" keyword; it could be above, between or below all the rule rows.
It may be more convenient to use Import, Variables, Functions and Queries repeatedly rather than packing several definitions into a single cell. |
19.7.3. Creating and integrating spreadsheet decision tables
The API to use spreadsheet decision tables is in the drools-decisiontables
module.
There is really only one class to look at: SpreadsheetCompiler
.
This class will take spreadsheets in various formats, and generate rules in DRL (which you can then use in the normal way). The SpreadsheetCompiler
can just be used to generate partial rule files if it is wished, and assemble it into a complete rule package after the fact (this allows the separation of technical and non-technical aspects of the rules if needed).
To get started, a sample spreadsheet can be used as a base. Alternatively, if the plug-in is being used, the wizard can generate a spreadsheet from a template (to edit it an xls compatible spreadsheet editor will need to be used).
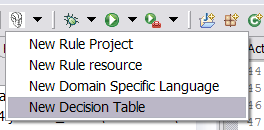
19.7.4. Decision table workflow and collaboration
Spreadsheets are well established business tools (in use for over 25 years). Decision tables lend themselves to close collaboration between IT and domain experts, while making the business rules clear to business analysts, it is an ideal separation of concerns.
Typically, the whole process of authoring rules (coming up with a new decision table) would be something like:
-
Business analyst takes a template decision table (from a repository, or from IT)
-
Decision table business language descriptions are entered in the table(s)
-
Decision table rules (rows) are entered (roughly)
-
Decision table is handed to a technical resource, who maps the business language (descriptions) to scripts (this may involve software development of course, if it is a new application or data model)
-
Technical person hands back and reviews the modifications with the business analyst.
-
The business analyst can continue editing the rule rows as needed (moving columns around is also fine etc).
-
In parallel, the technical person can develop test cases for the rules (liaising with business analysts) as these test cases can be used to verify rules and rule changes once the system is running.
19.7.5. Using spreadsheet features
Features of applications like Excel can be used to provide assistance in entering data into spreadsheets, such as validating fields. Lists that are stored in other worksheets can be used to provide valid lists of values for cells, like in the following diagram.
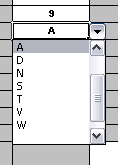
Some applications provide a limited ability to keep a history of changes, but it is recommended to use an alternative means of revision control. When changes are being made to rules over time, older versions are archived (many open source solutions exist for this, such as Subversion or Git).
19.7.6. Rule templates
Related to decision tables (but not necessarily requiring a spreadsheet) are "Rule Templates" (in the drools-templates module). These use any tabular data source as a source of rule data - populating a template to generate many rules. This can allow both for more flexible spreadsheets, but also rules in existing databases for instance (at the cost of developing the template up front to generate the rules).
With Rule Templates the data is separated from the rule and there are no restrictions on which part of the rule is data-driven. So whilst you can do everything you could do in decision tables you can also do the following:
-
store your data in a database (or any other format)
-
conditionally generate rules based on the values in the data
-
use data for any part of your rules (e.g. condition operator, class name, property name)
-
run different templates over the same data
As an example, a more classic decision table is shown, but without any hidden rows for the rule meta data (so the spreadsheet only contains the raw data to generate the rules).
Case |
Person age |
Cheese type |
Log |
Old guy |
42 |
stilton |
Old man stilton |
Young guy |
21 |
cheddar |
Young man cheddar |
See the ExampleCheese.xls in the examples download for the above spreadsheet.
If this was a regular decision table there would be hidden rows before row 1 and between rows 1 and 2 containing rule metadata. With rule templates the data is completely separate from the rules. This has two handy consequences - you can apply multiple rule templates to the same data and your data is not tied to your rules at all. So what does the template look like?
1 template header
2 age
3 type
4 log
5
6 package org.drools.examples.templates;
7
8 global java.util.List list;
9
10 template "cheesefans"
11
12 rule "Cheese fans_@{row.rowNumber}"
13 when
14 Person(age == @{age})
15 Cheese(type == "@{type}")
16 then
17 list.add("@{log}");
18 end
19
20 end template
Annotations to the preceding program listing:
-
Line 1: All rule templates start with
template header
. -
Lines 2-4: Following the header is the list of columns in the order they appear in the data. In this case we are calling the first column
age
, the secondtype
and the thirdlog
. -
Line 5: An empty line signifies the end of the column definitions.
-
Lines 6-9: Standard rule header text. This is standard rule DRL and will appear at the top of the generated DRL. Put the package statement and any imports and global and function definitions into this section.
-
Line 10: The keyword
template
signals the start of a rule template. There can be more than one template in a template file, but each template should have a unique name. -
Lines 11-18: The rule template - see below for details.
-
Line 20: The keywords
end template
signify the end of the template.
The rule templates rely on MVEL to do substitution using the syntax @{token_name}. There is currently one built-in expression, @{row.rowNumber} which gives a unique number for each row of data and enables you to generate unique rule names. For each row of data a rule will be generated with the values in the data substituted for the tokens in the template.
A rule template has to be included in a file with extension .drt and associated to the corresponding decision table when defining the kbase in the kmodule.xml file as in the following example
<?xml version="1.0" encoding="UTF-8"?>
<kmodule xmlns="http://drools.org/xsd/kmodule">
<kbase name="TemplatesKB" packages="org.drools.examples.templates">
<ruleTemplate dtable="org/drools/examples/templates/ExampleCheese.xls"
template="org/drools/examples/templates/Cheese.drt"
row="2" col="2"/>
<ksession name="TemplatesKS"/>
</kbase>
</kmodule>
With the example data above the following rule file would be generated:
package org.drools.examples.templates;
global java.util.List list;
rule "Cheese fans_1"
when
Person( age == 42 )
Cheese( type == "stilton" )
then
list.add( "Old man stilton" );
end
rule "Cheese fans_2"
when
Person( age == 21 )
Cheese( type == "cheddar" )
then
list.add( "Young man cheddar" );
end
At this point the KieSession
named "TemplatesKS" and containing the rules generated from the template can be simply created from the KieContainer
and used as any other KieSession
.
KieSession ksession = kc.newKieSession("TemplatesKS");
// now create some test data
ksession.insert(new Cheese("stilton", 42));
ksession.insert(new Person("michael", "stilton", 42));
final List<String> list = new ArrayList<String>();
ksession.setGlobal("list", list);
ksession.fireAllRules();
19.7.7. Uploading spreadsheet decision tables
After you define your rules in an external XLS or XLSX spreadsheet of decision tables, you can upload the spreadsheet file to your project in Business Central.
You should typically upload only one spreadsheet of decision tables, containing all necessary RuleTable definitions, per rule package in Business Central. You can upload separate decision table spreadsheets for separate packages, but uploading multiple spreadsheets in the same package can cause compilation errors from conflicting RuleSet or RuleTable attributes and is therefore not recommended.
|
-
In Business Central, go to Menu → Design → Projects and click the project name.
-
Click Add Asset → Decision Table (Spreadsheet).
-
Enter an informative Decision Table name and select the appropriate Package.
-
Select the file type (xls or xlsx), click the Choose File icon, and select the spreadsheet. Click Ok to upload.
-
In the decision tables designer, click Validate in the upper-right toolbar to validate the table. If the table validation fails, open the XLS or XLSX file and address any syntax errors. For syntax help, see Defining spreadsheet decision tables.
Converting a spreadsheet decision table to a guided decision table
To convert a spreadsheet decision table to a guided decision table, click Convert in the upper-right toolbar in the decision tables designer. Converting the spreadsheet to a guided decision table enables you to edit the table data directly in Business Central. For more information about guided decision tables, see Guided decision tables. |
19.8. DRL (Drools Rule Language) rules
DRL (Drools Rule Language) rules are business rules that you define directly in .drl
text files. These DRL files are the source in which all other rule assets in Business Central are ultimately rendered. You can create and manage DRL files within the Business Central interface, or create them externally using Red Hat Developer Studio, Java objects, or Maven archetypes. A DRL file can contain one or more rules that define at minimum the rule conditions (when
) and actions (then
). The DRL designer in Business Central provides syntax highlighting for Java, DRL, and XML.
All data objects related to a DRL rule must be in the same project package as the DRL rule in Business Central. Assets in the same package are imported by default. Existing assets in other packages can be imported with the DRL rule.
19.8.1. Creating DRL rules in Business Central
You can create and manage DRL rules for your project in Business Central. In each DRL rule file, you define rule conditions, actions, and other components related to the rule, based on the data objects you create or import in the package.
-
In Business Central, go to Menu → Design → Projects and click the project name.
-
Click Add Asset → DRL file.
-
Enter an informative DRL file name and select the appropriate Package. The package that you specify must be the same package where the required data objects have been assigned or will be assigned.
You can also select Show declared DSL sentences if any domain specific language (DSL) assets have been defined in your project. These DSL assets will then become usable objects for conditions and actions that you define in the DRL designer.
-
Click Ok to create the rule asset.
The new DRL file is now listed in the DRL panel of the Project Explorer, or in the DSLR panel if you selected the Show declared DSL sentences option. The package to which you assigned this DRL file is listed at the top of the file.
-
In the Fact types list in the left panel of the DRL designer, confirm that all data objects and data object fields (expand each) required for your rules are listed. If not, you can either import relevant data objects from other packages by using
import
statements in the DRL file, or create data objects within your package. -
After all data objects are in place, return to the Model tab of the DRL designer and define the DRL file with any of the following components:
Components of a DRL filepackage //automatic import function //optional query //optional declare //optional rule rule ...
-
package
: (automatic) This was defined for you when you created the DRL file and selected the package. -
import
: Use this to identify the data objects from either this package or another package that you want to use in the DRL file. Specify the package and data object in the formatpackage.name.object.name
, one import per line.Importing data objectsimport mortgages.mortgages.LoanApplication;
-
function
: (optional) Use this to include a function to be used by rules in the DRL file. Functions put semantic code in your rule source file. Functions are especially useful if an action (then
) part of a rule is used repeatedly and only the parameters differ for each rule. Above the rules in the DRL file, you can declare the function or import a static method as a function, and then use the function by name in an action (then
) part of the rule.Declaring and using a function with a rule (option 1)function String hello(String applicantName) { return "Hello " + applicantName + "!"; } rule "Using a function" when eval( true ) then System.out.println( hello( "James" ) ); end
Importing and using the function with a rule (option 2)import function my.package.applicant.hello; rule "Using a function" when eval( true ) then System.out.println( hello( "James" ) ); end
-
query
: (optional) Use this to search the Drools engine for facts related to the rules in the DRL file. Queries search for a set of defined conditions and do not requirewhen
orthen
specifications. Query names are global to the KIE base and therefore must be unique among all other rule queries in the project. To return the results of a query, construct a traditionalQueryResults
definition usingksession.getQueryResults("name")
, where"name"
is the query name. This returns a list of query results, which enable you to retrieve the objects that matched the query. Define the query and query results parameters above the rules in the DRL file.Query and query results for people under the age of 21, with a rulequery "people under the age of 21" person : Person( age < 21 ) end QueryResults results = ksession.getQueryResults( "people under the age of 21" ); System.out.println( "we have " + results.size() + " people under the age of 21" ); rule "Underage" when application : LoanApplication( ) Applicant( age < 21 ) then application.setApproved( false ); application.setExplanation( "Underage" ); end
-
declare
: (optional) Use this to declare a new fact type to be used by rules in the DRL file. The default fact type in thejava.lang
package of Drools isObject
, but you can declare other types in DRL files as needed. Declaring fact types in DRL files enables you to define a new fact model directly in the Drools engine, without creating models in a lower-level language like Java.Declaring and using a new fact typedeclare Person name : String dateOfBirth : java.util.Date address : Address end rule "Using a declared type" when $p : Person( name == "James" ) then // Insert Mark, who is a customer of James. Person mark = new Person(); mark.setName( "Mark" ); insert( mark ); end
-
rule
: Use this to define each rule in the DRL file. Rules consist of a rule name in the formatrule "name"
, followed by optional attributes that define rule behavior (such assalience
orno-loop
), followed bywhen
andthen
definitions. The same rule name cannot be used more than once in the same package. Thewhen
part of the rule contains the conditions that must be met to execute an action. For example, if a bank requires loan applicants to have over 21 years of age, then thewhen
condition for anUnderage
rule would beApplicant( age < 21 )
. Thethen
part of the rule contains the actions to be performed when the conditional part of the rule has been met. For example, when the loan applicant is under 21 years old, thethen
action would besetApproved( false )
, declining the loan because the applicant is under age. Conditions (when
) and actions (then
) consist of a series of stated fact patterns with optional constraints, bindings, and other supported DRL elements, based on the available data objects in the package. These patterns determine how defined objects are affected by the rule.Rule for loan application age limitrule "Underage" salience 15 dialect "mvel" when application : LoanApplication( ) Applicant( age < 21 ) then application.setApproved( false ); application.setExplanation( "Underage" ); end
At minimum, each DRL file must specify the
package
,import
, andrule
components. All other components are optional.Figure 328. Sample DRL file with required components and optional rule attributes
-
-
After you define all components of the rule, click Validate in the upper-right toolbar of the DRL designer to validate the DRL file. If the file validation fails, address any problems described in the error message, review all syntax and components in the DRL file, and try again to validate the file until the file passes.
-
Click Save in the DRL designer to save your work.
19.8.1.1. Adding WHEN conditions in DRL rules
The when
part of the rule contains the conditions that must be met to execute an action. For example, if a bank requires loan applicants to have over 21 years of age, then the when
condition of an Underage
rule would be Applicant( age < 21 )
. Conditions consist of a series of stated patterns and constraints, with optional bindings and other supported DRL elements, based on the available data objects in the package.
-
The
package
is defined at the top of the DRL file. This should have been done for you when you created the file. -
The
import
list of data objects used in the rule is defined below thepackage
line of the DRL file. Data objects can be from this package or from another package in Business Central. -
The
rule
name is defined in the formatrule "name"
below thepackage
,import
, and other lines that apply to the entire DRL file. The same rule name cannot be used more than once in the same package. Optional rule attributes (such assalience
orno-loop
) that define rule behavior are below the rule name, before thewhen
section.
-
In the DRL designer, enter
when
within the rule to begin adding condition statements. Thewhen
section consists of zero or more fact patterns that define conditions for the rule.If the
when
section is empty, then actions in thethen
section are executed every time afireAllRules()
call is made in the Drools engine. This is useful if you want to use rules to set up the Drools engine state.Rule without conditionsrule "bootstrap" when // empty then // actions to be executed once insert( new Applicant() ); end // The above rule is internally rewritten as: rule "bootstrap" when eval( true ) then insert( new Applicant() ); end
-
Enter a pattern for the first condition to be met, with optional constraints, bindings, and other supported DRL elements. A basic pattern format is
patternBinding : patternType ( constraints )
. Patterns are based on the available data objects in the package and define the conditions to be met in order to trigger actions in thethen
section.-
Simple pattern: A simple pattern with no constraints matches against a fact of the given type. For example, the following condition is only that the applicant exists.
when Applicant( )
-
Pattern with constraints: A pattern with constraints matches against a fact of the given type and the additional restrictions in parentheses that are true or false. For example, the following condition is that the applicant is under the age of 21.
when Applicant( age < 21 )
-
Pattern with binding: A binding on a pattern is a shorthand reference that other components of the rule can use to refer back to the defined pattern. For example, the following binding
a
onLoanApplication
is used in a related action for underage applicants.when a : LoanApplication( ) Applicant( age < 21 ) then a.setApproved( false ); a.setExplanation( "Underage" )
-
-
Continue defining all condition patterns that apply to this rule. The following are some of the keyword options for defining DRL conditions:
-
and
: Use this to group conditional components into a logical conjunction. Infix and prefixand
are supported. By default, all listed conditions or actions are combined withand
when no conjunction is specified.a : LoanApplication( ) and Applicant( age < 21 ) a : LoanApplication( ) and Applicant( age < 21 ) a : LoanApplication( ) Applicant( age < 21 ) // All of the above are the same.
-
or
: Use this to group conditional components into a logical disjunction. Infix and prefixor
are supported.Bankruptcy( amountOwed == 100000 ) or IncomeSource( amount == 20000 ) Bankruptcy( amountOwed == 100000 ) or IncomeSource( amount == 20000 )
-
exists
: Use this to specify facts and constraints that must exist. Note that this does not mean that a fact exists, but that a fact must exist. This option is triggered on only the first match, not subsequent matches.exists ( Bankruptcy( yearOfOccurrence > 1990 || amountOwed > 10000 ) )
-
not
: Use this to specify facts and constraints that must not exist.not ( Applicant( age < 21 ) )
-
forall
: Use this to set up a construct where all facts that match the first pattern match all the remaining patterns.forall( app : Applicant( age < 21 ) Applicant( this == app, status = 'underage' ) )
-
from
: Use this to specify a source for data to be matched by the conditional pattern.Applicant( ApplicantAddress : address ) Address( zipcode == "23920W" ) from ApplicantAddress
-
entry-point
: Use this to define anEntry Point
corresponding to a data source for the pattern. Typically used withfrom
.Applicant( ) from entry-point "LoanApplication"
-
collect
: Use this to define a collection of objects that the construct can use as part of the condition. In the example, all pending applications in the Drools engine for each given mortgage are grouped inArrayLists
. If three or more pending applications are found, the rule is executed.m : Mortgage() a : ArrayList( size >= 3 ) from collect( LoanApplication( Mortgage == m, status == 'pending' ) )
-
accumulate
: Use this to iterate over a collection of objects, execute custom actions for each of the elements, and return one or more result objects (if the constraints evaluate totrue
). This option is a more flexible and powerful form ofcollect
. Use the formataccumulate( <source pattern>; <functions> [;<constraints>] )
. In the example,min
,max
, andaverage
are accumulate functions that calculate the minimum, maximum and average temperature values over all the readings for each sensor. Other supported functions includecount
,sum
,variance
,standardDeviation
,collectList
, andcollectSet
.s : Sensor() accumulate( Reading( sensor == s, temp : temperature ); min : min( temp ), max : max( temp ), avg : average( temp ); min < 20, avg > 70 )
Advanced DRL optionsThese are examples of basic keyword options and pattern constructs for defining conditions. For more advanced DRL options and syntax supported in the DRL designer, see Rule Language Reference.
-
-
After you define all condition components of the rule, click Validate in the upper-right toolbar of the DRL designer to validate the DRL file. If the file validation fails, address any problems described in the error message, review all syntax and components in the DRL file, and try again to validate the file until the file passes.
-
Click Save in the DRL designer to save your work.
19.8.1.2. Adding THEN actions in DRL rules
The then
part of the rule contains the actions to be performed when the conditional part of the rule has been met. For example, when a loan applicant is under 21 years old, the then
action of an Underage
rule would be setApproved( false )
, declining the loan because the applicant is under age. Actions execute consequences based on the rule conditions and on available data objects in the package.
-
The
package
is defined at the top of the DRL file. This should have been done for you when you created the file. -
The
import
list of data objects used in the rule is defined below thepackage
line of the DRL file. Data objects can be from this package or from another package in Business Central. -
The
rule
name is defined in the formatrule "name"
below thepackage
,import
, and other lines that apply to the entire DRL file. The same rule name cannot be used more than once in the same package. Optional rule attributes (such assalience
orno-loop
) that define rule behavior are below the rule name, before thewhen
section.
-
In the DRL designer, enter
then
after thewhen
section of the rule to begin adding action statements. -
Enter one or more actions to be executed on fact patterns based on the conditions for the rule.
The following are some of the keyword options for defining DRL actions:
-
and
: Use this to group action components into a logical conjunction. Infix and prefixand
are supported. By default, all listed conditions or actions are combined withand
when no conjunction is specified.application.setApproved ( false ) and application.setExplanation( "has been bankrupt" ); application.setApproved ( false ); and application.setExplanation( "has been bankrupt" ); application.setApproved ( false ); application.setExplanation( "has been bankrupt" ); // All of the above are the same.
-
set
: Use this to set the value of a field.application.setApproved ( false ); application.setExplanation( "has been bankrupt" );
-
modify
: Use this to specify fields to be modified for a fact and to notify the Drools engine of the change.modify( LoanApplication ) { setAmount( 100 ) }
-
update
: Use this to specify fields and the entire related fact to be modified and to notify the Drools engine of the change. After a fact has changed, you must callupdate
before changing another fact that might be affected by the updated values. Themodify
keyword avoids this added step.update( LoanApplication ) { setAmount( 100 ) }
-
delete
: Use this to remove an object from the Drools engine. The keywordretract
is also supported in the DRL designer and executes the same action, butdelete
is preferred for consistency with the keywordinsert
.delete( LoanApplication );
-
insert
: Use this to insert anew
fact and define resulting fields and values as needed for the fact.insert( new Applicant() );
-
insertLogical
: Use this to insert anew
fact logically into the Drools engine and define resulting fields and values as needed for the fact. The Drools engine is responsible for logical decisions on insertions and retractions of facts. After regular or stated insertions, facts have to be retracted explicitly. After logical insertions, facts are automatically retracted when the conditions that originally asserted the facts are no longer true.insertLogical( new Applicant() );
Advanced DRL optionsThese are examples of basic keyword options and pattern constructs for defining actions. For more advanced DRL options and syntax supported in the DRL designer, see Rule Language Reference.
-
-
After you define all action components of the rule, click Validate in the upper-right toolbar of the DRL designer to validate the DRL file. If the file validation fails, address any problems described in the error message, review all syntax and components in the DRL file, and try again to validate the file until the file passes.
-
Click Save in the DRL designer to save your work.
Rule attributes
Rule attributes are additional specifications that you can add to business rules to modify rule behavior. The following table lists the names and supported values of the attributes that you can assign to rules:
Attribute | Value |
---|---|
|
An integer defining the priority of the rule. Rules with a higher salience value are given higher priority when ordered in the activation queue. Example: |
|
A Boolean value. When the option is selected, the rule is enabled. When the option is not selected, the rule is disabled. Example: |
|
A string containing a date and time definition. The rule can be activated only if the current date and time is after a Example: |
|
A string containing a date and time definition. The rule cannot be activated if the current date and time is after the Example: |
|
A Boolean value. When the option is selected, the rule cannot be reactivated (looped) if a consequence of the rule re-triggers a previously met condition. When the condition is not selected, the rule can be looped in these circumstances. Example: |
|
A string identifying an agenda group to which you want to assign the rule. Agenda groups allow you to partition the agenda to provide more execution control over groups of rules. Only rules in an agenda group that has acquired a focus are able to be activated. Example: |
|
A string identifying an activation (or XOR) group to which you want to assign the rule. In activation groups, only one rule can be activated. The first rule to fire will cancel all pending activations of all rules in the activation group. Example: |
|
A long integer value defining the duration of time in milliseconds after which the rule can be activated, if the rule conditions are still met. Example: |
|
A string identifying either Example: |
|
A Quartz calendar definition for scheduling the rule. Example: |
|
A Boolean value, applicable only to rules within agenda groups. When the option is selected, the next time the rule is activated, a focus is automatically given to the agenda group to which the rule is assigned. Example: |
|
A Boolean value, applicable only to rules within rule flow groups or agenda groups. When the option is selected, the next time the ruleflow group for the rule becomes active or the agenda group for the rule receives a focus, the rule cannot be activated again until the ruleflow group is no longer active or the agenda group loses the focus. This is a stronger version of the Example: |
|
A string identifying a rule flow group. In rule flow groups, rules can fire only when the group is activated by the associated rule flow. Example: |
|
A string identifying either Example: |
19.9. DSL (domain specific language) rules
The DSL editor allows DSL Sentences to be authored. The reader should take time to explore DSL features in the Drools Expert documentation; as the syntax in Business Central’s DSL Editor is identical. The normal syntax is extended to provide "hints" to control how the DSL variable is rendered and validated within the user-interface.
The following "hints" are supported:-
-
{<varName>:<regular expression>}
This will render a text field in place of the DSL variable when the DSL Sentence is used in the guided editor. The content of the text field will be validated against the regular expression.
-
{<varName>:ENUM:<factType.fieldName>}
This will render an enumeration in place of the DSL variable when the DSL Sentence is used in the guided editor. <factType.fieldName> binds the enumeration to the model Fact and Field enumeration definition. This could be either a "Business Central enumeration" (i.e. defined within Business Central) or a Java enumeration (i.e. defined in a model POJO JAR file).
-
{<varName>:DATE:<dateFormat>}
This will render a Date selector in place of the DSL variable when the DSL Sentence is used in the guided editor.
-
{<varName>:BOOLEAN:<[checked | unchecked]>}
This will render a dropdown selector in place of the DSL variable, providing boolean choices, when the DSL Sentence is used in the guided editor.
-
{<varName>:CF:<factType.fieldName>}
This will render a button that will allow you to set the value of this variable using a Custom Form. In order to use this feature, a Working-Set containing a Custom Form Configuration for factType.fieldName must be active. If there is no such Working-Set, a simple text box is used (just like a regular variable).
For more information, please read more about Working-Sets and Custom Form Configurations.

19.10. Scorecards
A scorecard is a graphical representation of a formula used to calculate an overall score. A scorecard can be used to predict the likelihood or probability of a certain outcome. Drools now supports additive scorecards. An additive scorecard calculates an overall score by adding all partial scores assigned to individual rule conditions.
Additionally, Drools Scorecards will allows for reason codes to be set, which help in identifying the specific rules (buckets) that have contributed to the overall score. Drools Scorecards will be based on the PMML 4.1 Standard.
The New Item menu now allows for creation of scorecard assets.
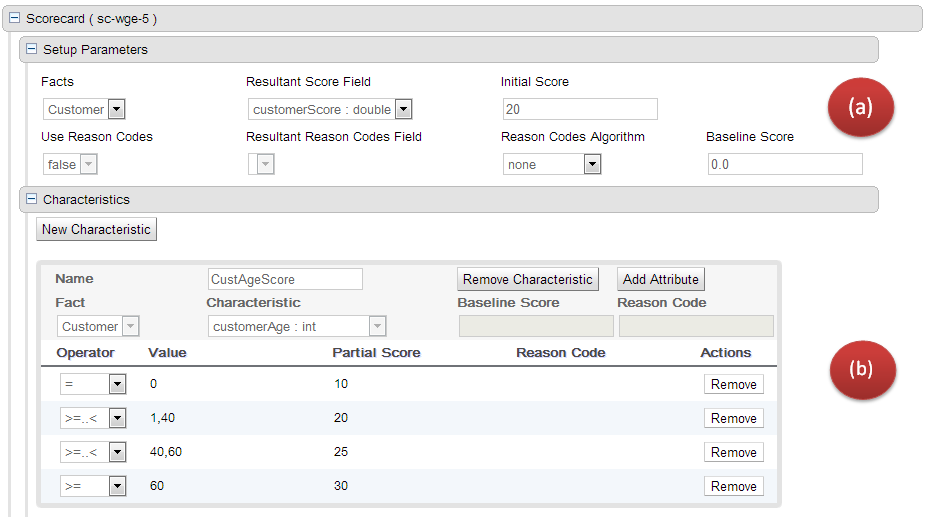
The above image shows a scorecard with one characteristic. Each scorecard consists of two sections (a) Setup Parameters (b) Characteristic Section
19.10.1. (a) Setup parameters
The setup section consits of parameters that define the overall behaviour of this scorecard.
-
Facts: This dropdown shows a list of facts that are visible for this asset.
-
Resultant Score Field: Shows a list of fields from the selected fact. Only fields of type 'double' are shown. If this dropdown is empty double check your fact model. The final calculated score will be stored in this field.
-
Initial Score: Numeric Text Field to capture the initial score. The generated rules will initialize the 'Resultant Score Field' with this score and then is added to the overall score whenever partial scores are summed up.
-
Use Reason Codes: Boolean indicator to compute reason codes along with the final score. Selecting Yes/No in this field will enable/disable the 'Resultant Reason Codes Field', 'Reason Code Algorithm' and the 'Baseline Score' field.
-
Resultant Reason Codes Field: Shows a list of fields from the selected fact. Only fields of type 'java.util.List' are shown. This collection will hold the reason codes selected by this scorecard.
-
Reason Code Algorithm: May be "none", "pointsAbove" or "pointsBelow", describing how reason codes shall be ranked, relative to the baseline score of each Characteristic, or as set at the top-level scorecard.
-
Baseline Score: A single value to use as the baseline comparison score for all characteristics, when determining reason code ranking. Alternatively, unique baseline scores may be set for each individual Characteristic as shown below. This value is required only when UseReasonCodes is "true" and baselineScore is not given for each Characteristic.
If UseReasonCodes is "true", then BaselineScore must be defined at the Scorecard level or for each Characteristic, and ReasonCode must be provided for each Characteristic or for each of its input Attributes. If UseReasonCodes is "false", then baselineScore and reasonCode are not required. |
19.10.2. (b) Characteristics
On Clicking the 'New Characteristic' button, a new empty characteristic editor is added to the scorecard. Defines the point allocation strategy for each scorecard characteristic (numeric or categorical). Each scorecard characteristic is assigned a single partial score which is used to compute the overall score. The overall score is simply the sum of all partial scores. Partial scores are assumed to be continuous values of type "double".
19.10.2.1. Creating characterstics
Every scorecard must have at least one characteristic
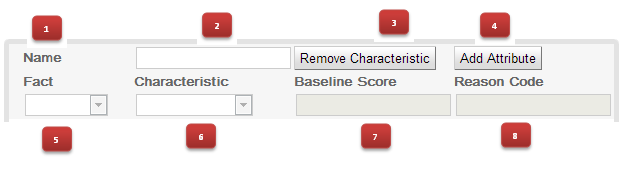
-
Name: Descriptive name for this characteristic. For informational reasons only.
-
Remove Charteristic: Will remove this characteristic from the scorecard after a confirmation dialog is shown.
-
Add Attribute: Will add a line entry for an attribute (bin).
-
Fact: Select the class which will be evaluated for calculating the partial score.
-
Characteristic: Shows the list of fields from the selected Fact. Only fields of type "String", "int", "double", "boolean" are shown.
-
Baseline Score: Sets the characteristic’s baseline score against which to compare the actual partial score when determining the ranking of reason codes. This value is required when useReasonCodes attribute is "true" and baselineScore is not defined in element Scorecard. Whenever baselineScore is defined for a Characteristic, it takes precedence over the baselineScore value defined in element Scorecard.
-
Reason Code: Contains the characteristic’s reason code, usually associated with an adverse decision.
19.10.2.2. Creating attributes
On Clicking the 'New Attribute' button, a new empty attribute editor. In scorecard models, all the elements defining the Attributes for a particular Characteristic must all reference a single field.

-
Operator: The condition upon which the mapping between input attribute and partial score takes place. The operator dropdown will show different values depending on the datatype of the selected Field.
-
DataType Strings: "=", "in".
-
DataType Integers: "=", ">", "<", ">=", "⇐", ">..<", ">=..<", ">=..⇐", ">..⇐".
-
DataType Boolean: "true", "false".
Refer to the next sub-section (values) for more details.
-
-
Value: Basis the operator selected the value specified can either be a single value or a set of values separated by comma (","). The value field is disabled for operator type boolean.
Table 56. Operators / Values Data Type
Operator
Value
Remarks
String
=
Single Value
will look for an exact match
String
in
Comma Separated Values (a,b,c,…)
The operator 'in' indicates an evaluation to TRUE if the field value is contained in the comma separated list of values
Boolean
is true
N/A
Value Field is uneditable (readonly)
Boolean
is false
N/A
Value Field is uneditable (readonly)
Numeric
=
Single Value
Equals Operator
Numeric
>
Single Value
Greator Than Operator
Numeric
<
Single Value
Less Than Operator
Numeric
>=
Single Value
Greater than or equal To
Numeric
<=
Single Value
Less than or equal To
Numeric
>..<
Comma Separated Values (a,b)
(Greater than Value 'a') and (less than value 'b')
Numeric
>=..<
Comma Separated Values (a,b)
(Greater than or equal to Value 'a') and (less than value 'b')
Numeric
>=..<=
Comma Separated Values (a,b)
(Greater than or equal to Value 'a') and (less than or equal to value 'b')
Numeric
>..<=
Comma Separated Values (a,b)
(Greater than Value 'a') and (less than or equal to value 'b')
-
Partial Score: Defines the score points awarded to the Attribute.
-
Reason Code: Defines the attribute’s reason code. If the reasonCode attribute is used in this level, it takes precedence over the ReasonCode associated with the Characteristic element.
-
Actions: Delete this attribute. Prompts the user for confirmation.
If Use Reason Codes is "true", then Baseline Score must be defined at the Scorecard level or for each Characteristic, and Reason Code must be provided for each Characteristic or for each of its input Attributes. If Use Reason Codes is "false", then BaselineScore and ReasonCode are not required. |
19.11. Data enumerations (drop-down list configurations)
Data enumerations are an optional asset type that technical folk can configure to provide drop down lists for the guided editor. These are stored and edited just like any other asset, and apply to the package that they belong to.
The contents of an enum config are a mapping of Fact.field
to a list of values to be used in a drop down.
The strings are either a value to be shown in a drop down, or a mapping from the code value (what ends up used in the rule) and a display value.
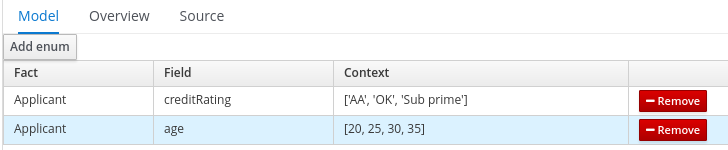
If you wish to use a mapping between value used in the rule and the value shown in the UI you need to separate the code value and display value with an equals sign. For example:
'Person.gender' : ['M=Male','F=Female']
In this example F
will be used in the rules but Female
shown in the UI.
Drop down lists can also depend on other field values.
Lets imagine a simple fact model, we have a class called Vehicle, which has 2 fields: engineType
and fuelType
.
We want to have a choice for the engineType
of "Petrol" or "Diesel". Now, obviously the choice type for fuel must be dependent on the engine type (so for Petrol we have ULP and PULP, and for Diesel we have BIO and NORMAL). We can express this dependency in an enumeration as:
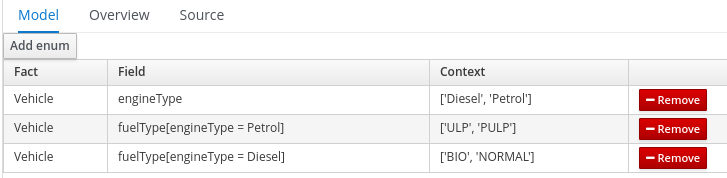
This shows how it is possible to make the choices dependent on other field values. Note that once you pick the engineType, the choice list for the fuelType will be determined.
Choices are not dependent across rule conditions and actions. If the rule condition checks engine type then the guided editor will not offer dependent fuel types in the rule actions. |
19.11.1. Advanced enumeration concepts
There are a few other advanced things you can do with data enumerations.
19.11.1.1. External data-sources
Instead of defining a static list you can retrieve the list from an external data-source by using a helper class.
The helper class must be on the Project’s classpath; by adding a JAR containng the class as a project dependency.
The helper class must be instantiable and have a non-static method that returns a java.util.List
.
Population of the list is implementation specific.
For example:
'Person.age' : (new org.yourco.DataHelper()).getListOfAges()
In some other cases you may want to load the enumeration data entirely from an external data source (such as a relational database). To do this, you can implement a class that returns a Map<String, List<String>>
.
The key of the map is the Fact.field
and the value is a java.util.List<String>
of values to be used.
public class SampleDataSource2 {
public Map<String>, List<String> loadData() {
Map data = new HashMap();
List d = new ArrayList();
d.add("value1");
d.add("value2");
data.put("Fact.field", d);
return data;
}
}
The enumeration definition would simply be as follows (with no reference to fact or field names):
=(new SampleDataSource2()).loadData()
The =
operator informs Business Central to load all enumeration data from the helper class.
The helper methods will be statically evaluated when the enumeration definition is requested for use in an editor. |
19.11.1.2. Dynamic lookup
All of the above cases retrieve the list of values by statically evaluating the enumeration definition when enumerations are required by an editor.
It is also possible to load dependent enumeration definitions dynamically from a helper class by enclosing the call to the helper class within quotation marks. For example:
'Country.region[countryCode]' : '(new org.yourco.DataHelper()).getListOfRegions("@{countryCode}")'
19.12. Test scenarios
Test scenarios in Drools enable you to validate the functionality of business rules and business rule data (for rules-based test scenarios) or of DMN models (for DMN-based test scenarios) before deploying them into a production environment. With a test scenario, you use data from your project to set given conditions and expected results based on one or more defined business rules. When you run the scenario, the expected results and actual results of the rule instance are compared. If the expected results match the actual results, the test is successful. If the expected results do not match the actual results, then the test fails.
Drools currently supports both the new Test Scenarios designer and the former Test Scenarios (Legacy) designer. The default designer is the new test scenarios designer, which supports testing of both rules and DMN models and provides an enhanced overall user experience with test scenarios. The new test scenarios designer currently can use only the default KIE session. If required, you can continue to use the legacy test scenarios designer, which supports rule-based test scenarios only.
With the test scenarios designer, you execute all scenarios from the .scesim
file at one time, whereas with the legacy test scenarios designer, you can execute test scenarios one at a time or as a group. The group execution contains all the scenarios from one package. Test scenarios are independent, so one scenario cannot affect or modify the other. You can run test scenarios at any time during project development in Business Central. You do not have to compile or deploy your decision service to run test scenarios.
You can import data objects from different packages to the same project package as the test scenario. Assets in the same package are imported by default. After you create the necessary data objects and the test scenario, you can use the Data Objects tab of the test scenarios designer to verify that all required data objects are listed or to import other existing data objects by adding a New item.
Throughout the test scenarios documentation, all references to test scenarios and the test scenarios designer are for the new version, unless explicitly noted as the legacy version. |
19.12.1. Test scenarios designer in Business Central
The test scenarios designer provides a tabular layout that helps you in defining a scenario template and all the associated test cases. The designer layout consists of a table which has a header and the individual rows. The header consists of three parts, the GIVEN and EXPECT row, a row with instances, and a row with corresponding fields. The header is also known as test scenario template and the individual rows are called test scenarios definitions.
The test scenario template or header has the following two parts:
-
GIVEN data objects and their fields - represents the input information
-
EXPECT data objects and their fields - represents the objects and their fields whose exact values are checked based on the given information and which also constitutes the expected result.
The test scenarios definitions represent the separate test cases of a template.
You can access the Project Explorer from the left panel of the designer whereas from the right panel you can access the Test Tools and the Test Report. You can use the Test Tools from the right panel to configure the data object mappings (Test Editor) or for accessing the cheat sheet (Scenario Cheatsheet) that contain notes which you can use as reference.
19.12.1.1. Importing data objects
The test scenarios designer loads all data objects that are located in the same package as the test scenario. You can view all the data objects from the Data Objects tab in the designer. The loaded data objects are also displayed in the Test Editor tab in the Test Tools panel.
You need to close and reopen the designer in case the data objects change (for example, when a new data object is created or when an existing one is deleted). Select a data object from the list to display its fields and the field types.
In case you want to use a data object located in a different package than the test scenario, you need to import the data object first. Follow the procedure below to import a data object for rules-based test scenarios.
You cannot import any data objects while creating DMN-based test scenarios. DMN-based test scenarios does not use any data objects from the project but uses the custom data types defined in the DMN file. |
-
Go to Project Explorer panel in the test scenarios designer.
-
From Test Scenario, select a test scenario.
-
Select Data Objects tab and click New Item.
-
In the Add import window, choose the data object from the drop-down list.
-
Click Ok and then Save.
-
Close and reopen the test scenarios designer to view the new data object from the data objects list.
19.12.1.2. Importing a test scenario
You can import an existing test scenario using the Import Asset button in the Asset tab from the project view.
-
In Business Central, go to Menu → Design → Projects and click the project name.
-
From the project’s Asset tab, click Import Asset.
-
In the Create new Import Asset window,
-
Enter the name of the import asset.
-
Select the package from the Package drop-down list.
-
From Please select a file to upload, click Choose File… to browse to test scenario file.
-
-
Select the file and click Open.
-
Click Ok and the test scenario opens in the review designer.
19.12.1.3. Saving a test scenario
You can save a test scenario at any time while creating a test scenario template or defining the test scenarios.
-
From the test scenarios designer toolbar on the upper-right, click Save.
-
On the Confirm Save window,
-
If you wish to add a comment regarding the test scenario, click add a comment.
-
Click Save again.
-
A message stating that the test scenario was saved successfully appears on the screen.
19.12.1.4. Deleting a test scenario
You can delete existing test scenarios that were created using the test scenarios designer.
-
From the test scenarios designer toolbar on the upper-right, click Delete.
-
In the Confirm Delete window,
-
To add a comment regarding the deletion of the test scenario, click add a comment.
-
Click Delete.
-
A message stating that the test scenario was deleted successfully appears on the screen.
19.12.1.5. Renaming a test scenario
You can rename existing test scenarios by using the Rename button from the upper-right toolbar in the designer.
-
From the test scenarios designer toolbar on the upper-right, click Rename.
-
In the Rename Asset window,
-
Enter a name in the Asset Name field.
-
If you wish to add a comment, click add a comment.
-
Click Rename.
Clicking on Rename simply renames the test scenario file.
-
From the test scenarios designer toolbar on the upper-right, click Save.
-
In the Confirm Save window,
-
If you wish to add a comment, click add a comment.
-
Click Save again.
-
-
Alternately, you could click Save and Rename to save and rename the scenario at the same time.
-
A message stating that the test scenario was renamed successfully appears on the screen.
19.12.1.6. Copying a test scenario
You can copy an existing test scenario to the same package or to some other package by using the Copy button from the upper-right toolbar.
-
From the test scenarios designer toolbar on the upper-right, click Copy.
-
In the Make a Copy window,
-
Enter a name in the New Name field.
-
Select the package you want to copy the test scenario to.
-
Optionally, to add a comment, click add a comment.
-
Click Make a Copy.
-
A message stating that the test scenario was copied successfully appears on the screen.
19.12.1.7. Downloading a test scenario
You can download a copy of the test scenario to your local machine for future reference or as backup.
From the test scenarios designer toolbar on the upper-right, click Download.
The .scesim
file is downloaded to your local machine.
19.12.1.8. Switching between versions of a test scenario
Business Central provides you the ability to switch between the various versions of a test scenario. Every time you save the scenario, a new version of the scenario is listed under Latest Versions. To use this feature, you must save the test scenario file at least once.
-
From the test scenarios designer toolbar on the upper-right, click Latest Version. All the versions of the file are listed under Latest Version, if they exist.
-
Click the version you want to work on.
The selected version of the test scenario opens in the test scenarios designer.
-
From the designer toolbar, click Restore.
-
In the Confirm Restore,
-
To add a comment, click add a comment.
-
Click Restore to confirm.
-
A message stating that the selected version has been reloaded successfully in the designer appears on the screen.
19.12.1.9. View or hide the alerts panel
In the test scenarios designer, the message panel appears at the bottom of the designer with the test results and the Alert messages.
From the designer toolbar on the upper-right, click Hide Alerts/View Alerts to enable or disable the reporting panel.
19.12.1.10. Contextual menu options
The test scenarios designer provides contextual menu options, which enables you to perform basic operations on the table such as adding, deleting, and, duplicating rows and columns. To use the contextual menus, you need to right-click a table element. Menu options differ based on the table element you select.
Table element | Cell label | Available context menu options |
---|---|---|
Header |
# & Scenario description |
Insert row below |
GIVEN & EXPECT |
Insert leftmost column, Insert rightmost column, Insert row below |
|
INSTANCE 1, INSTANCE 2 & PROPERTY 1, PROPERTY 2 |
Insert column left, Insert column right, Delete column, Insert row below |
|
Rows |
Insert column left, Insert column right, Delete column, Insert row above, Insert row below, Duplicate row, Delete row |
Table interaction | Description |
---|---|
Insert leftmost column |
Inserts a new leftmost column (in either the GIVEN or EXPECT section of the table based on user selection). |
Insert rightmost column |
Inserts a new rightmost column (in either the GIVEN or EXPECT section of the table based on user selection). |
Insert column left |
Inserts a new column to the left of the selected column. The new column is of the same type as the selected column (in either the GIVEN or EXPECT section of the table based on user selection). |
Insert column right |
Inserts a new column to the right of the selected column. The new column is of the same type as the selected column (in either the GIVEN or EXPECT section of the table based on user selection). |
Delete column |
Deletes the selected column. |
Insert row above |
Inserts a new row above the selected row. |
Insert row below |
Inserts a new row below the selected row. If invoked from a header cell, inserts a new row with index 1. |
Duplicate row |
Duplicates the selected row. |
Delete row |
Deletes the selected row. |
The Insert column right
or Insert column left
context menu options behave differently,
-
if the selected column does not have a type defined, a new column without a type is added.
-
if the selected column has a type defined, either a new empty column or a column with the parent instance type is created.
-
if the action is performed from an instance header, a new column without a type is created.
-
if the action is performed from a property header, a new column with the parent instance type is created.
19.12.2. Test scenario template
Before specifying test scenario definitions, you need to create a test scenario template. The header of the test scenario table defines the template for each scenario. You need to set the types of the instance and property headers for both the GIVEN and EXPECT sections. Instance headers map to a particular data object (a fact), whereas the property headers map to a particular field of the corresponding data object.
Using the test scenarios designer, you can create test scenario templates for both rule-based and DMN-based test scenarios.
19.12.2.1. Creating a test scenario template for rule-based test scenarios
Create a test scenario template for rule-based test scenarios by following the procedure below to validate your rules and data.
-
In Business Central, go to Menu → Design → Projects and click the project for which you want to create the test scenario.
-
Click Add Asset → Test Scenario.
-
Enter a Test Scenario name and select the appropriate Package. The package you select must contain all the required data objects and rule assets have been assigned or will be assigned.
-
Select RULE as the Source type.
-
Click Ok to create and open the test scenario in the test scenarios designer.
-
To map the GIVEN column header to a data object,
-
Click an instance header in the GIVEN section.
-
Select the data object from the Test Editor tab.
-
Click Add.
-
-
To insert more properties of the data object, right-click the property header and select Insert column right or Insert column left as required.
-
To map a data object field to a property cell,
-
Click a property cell.
-
Select the data object field from the Test Editor tab.
-
Click Add.
-
-
To map the EXPECT column header to a data object,
-
Click an instance header in the EXPECT section.
-
Select the data object from the Test Editor tab.
-
Click Add.
-
-
To insert more properties of the data object, right-click the property header and select Insert column right or Insert column left as required.
-
To map a data object field to a property cell,
-
Click a property cell.
-
Select the data object field from the Test Editor tab.
-
Click Add.
-
Use the contextual menu to add or remove columns as needed.
After you have created and mapped both the GIVEN & EXPECT columns to a data object & its properties, you have to define the test scenario which comes next.
19.12.2.2. Using aliases in rule-based test scenarios
In the test scenarios designer, once you map a header cell with a data object, the data object is removed from the Test Editor tab in the Test Tools panel. You can re-map a data object to another header cell by using an alias. Aliases enable you to specify multiple instances of the same data object in a test scenario. You can also create property aliases to rename the used properties directly in the table.
In the test scenarios designer in Business Central, double-click a header cell and manually change the name. Ensure that the aliases are uniquely named.
The instance now appears in the list of data objects in the Test Editor tab.
19.12.3. Test template for DMN-based test scenarios
Business Central automatically generates the template for every DMN-based test scenario asset and it contains all the specified inputs and decisions of the related DMN model. For each input node in the DMN model, a GIVEN column is added, whereas each decision node is represented by an EXPECT column. You can modify the default template at any time as per your needs. Also, to test only a specific part of the whole DMN model, its possible to remove the generated columns as well as move decision nodes from the EXPECT to the GIVEN section.
19.12.3.1. Creating a test scenario template for DMN-based test scenarios
Create a test scenario template for DMN-based scenarios by following the procedure below to validate your DMN models.
-
In Business Central, go to Menu → Design → Projects and click the project that you want to create the test scenario for.
-
Click Add Asset → Test Scenario.
-
Enter a Test Scenario name and select the appropriate Package.
-
Select DMN as the Source type.
-
Select an existing DMN asset using the Choose DMN asset option.
-
Click Ok to create and open the test scenario in the test scenarios designer.
The template is automatically generated and you can modify it as per your needs.
19.12.4. Defining a test scenario
After creating a test scenario template you have to define the test scenario next. The rows of the test scenario table define the individual test scenarios. A test scenario has a unique index number, description, set of input values (the Given values), and a set of output values (the Expect values).
-
The test scenario template has been created for the selected test scenario.
-
Open the test scenario in the test scenarios designer.
-
Enter a description of the test scenario and fill in required values in each cell of the row.
-
Use the contextual menu to add or remove rows as required.
Double click a cell to start inline editing. To skip a particular cell from test evaluation, leave it empty.
After defining the test scenario, you can run the test next.
19.12.5. Using list and map collections in test scenarios
The test scenarios designer supports list and map collections for both DMN-based as well as rules-based test scenarios. You can define a collection like a list or a map as the value of a particular cell in both GIVEN and EXPECT columns.
-
Set the column type first (use a field whose type is a list or a map).
-
Double click a cell in the column to input a value.
-
In the collection editor popup, click Add new item.
-
Enter the required value and click the check icon
to save each collection item that you add.
-
Click Save.
To delete an item from the collection, click the bin icon in the collection popup editor. Click Remove to delete the collection itself.
19.12.6. Expression syntax in test scenarios
The test scenarios designer supports different expression languages for both rule-based and DMN-based test scenarios. While rule-based test scenarios support a basic expression language, DMN-based test scenarios support the FEEL expression language.
19.12.6.1. Expression syntax in rule-based test scenarios
The following rule-based test scenario definition expressions are supported by the test scenarios designer:
Operator | Description |
---|---|
= |
Specifies equal to a value. This is default for all columns and is the only operator supported by the GIVEN column. |
=, =!, <> |
Specifies inequality of a value. This operator can be combined with other operators. |
<, >, <=, >= |
Specifies a comparison: less than, greater than, less or equals than, and greater or equals than. |
[value1, value2, value3] |
Specifies a list of values. If one or more values are valid, the scenario definition is evaluated as true. |
expression1; expression2; expression3 |
Specifies a list of expressions. If all expressions are valid, the scenario definition is evaluated as true. |
An empty cell is skipped from evaluation. To define an empty string, use |
Expression | Description |
---|---|
-1 |
The actual value is equal to -1. |
< 0 |
The actual value is less than 0. |
! > 0 |
The actual value is not greater than 0. |
[-1, 0, 1] |
The actual value is equal to either -1 or 0 or 1. |
<> [1, -1] |
The actual value is neither equal to 1 nor -1. |
! 100; 0 |
The actual value is not equal to 100 but is equal to 0. |
!= < 0; <> > 1 |
The actual value is neither less than 0 nor greater than 1. |
<> <= 0; >= 1 |
The actual value is neither less than 0 nor equal to 0 but is greater than or equal to 1. |
You can refer to the supported commands and syntax in the Scenario Cheatsheet tab on the Test Tools panel on the right of the test scenarios designer. |
19.12.6.2. Expression syntax in DMN-based scenarios
The following data types are supported by the DMN-based test scenarios in the test scenarios designer:
Supported data types | Description |
---|---|
numbers & strings |
Strings must be delimited by quotation marks, for example, |
boolean values |
|
dates and time |
For example, |
functions |
|
contexts |
For example, |
ranges and lists |
For example, |
You can refer to the supported commands and syntax in the Scenario Cheatsheet tab on the Test Tools panel on the right side of the test scenarios designer. |
19.12.7. Running a test scenario
After creating a test scenario template and defining the test scenarios, you can run the tests to validate your business rules and data.
-
At the top of the test scenarios designer, click Run Test
icon.
-
The Test Report panel pops out from the right of the designer and displays the test result overview and the scenario status.
-
After the tests are executed, click View Alerts to open the Alerts panel at the bottom of the designer for the error messages.
-
In case the tests fails, review the error messages. Messages with a red cross indicate test failures where as those with a green check mark indicate successful tests.
In the designer, erroneous rows or cells are highlighted in red.
-
Make the necessary changes and run the test again till the scenario passes.
19.12.8. Running a test scenario locally
In Drools, you can run tests in two ways. One way is click the Run Test icon at the top of the Test Scenarios designer. The other way is to run the tests locally using the command line.
-
In Business Central, go to Menu → Design → Projects and click the project name.
-
On the Project’s home page, select the Settings tab.
-
Select git URL and click the Clipboard
to copy the git url.
-
Open a command terminal and navigate to the directory where you want to clone the git project.
-
From your project’s directory run the following command:
git clone your_git_project_url
Replace
your_git_project_url
with relevant data likegit://localhost:9418/MySpace/ProjectTestScenarios
. -
Once the project is successfully cloned, navigate to the git project directory and execute the following command:
mvn clean test
Your project’s build information and the test results (such as, the number of tests run and whether the test run was a success or not) are displayed in the command terminal. In case of errors, correct them and run the command again.
19.12.9. Creating test scenario using the sample Mortgages project
This chapter illustrates creating and executing a test scenario from the sample Mortgages project shipped with Business Central using the test scenarios designer. The test scenario example in this chapter is based on the Pricing loans guided decision table from the Mortgages project.
-
In Business Central, go to Menu → Design → Projects and click Mortgages.
-
If the project is not listed under Projects, from MySpace, click the three dots (
) in the upper-right corner of the page.
-
Click Try Samples → Mortgages → OK.
The Assets window appears.
-
Click Add Asset → Test Scenario.
-
Enter
scenario_pricing_loans
as the Test Scenario name and select the defaultmortgages.mortgages
package from the Package drop-down list.The package you select must contain all the required rule assets.
-
Click Ok to create and open the test scenario in the test scenarios designer.
-
Expand Project Explorer and verify the following:
-
Applicant
,Bankruptcy
,IncomeSource
, andLoanApplication
data objects exist. -
Pricing loans
guide decision table exists. -
Verify that the new test scenario is listed under Test Scenario
-
-
After verifying that everything is in place, return to the Model tab of the test scenarios designer and define the GIVEN and EXPECT data for the scenario, based on the available data objects.
Figure 334. A blank test scenarios designer -
Define the GIVEN column details,
-
Click the cell named INSTANCE 1 under the GIVEN column header.
-
From Test Tools → Test Editor, select LoanApplication data object.
-
Click Add.
-
-
To create properties of the data object, right-click the property cell and select Insert column right or Insert column left as required. For this example, you need to create two more property cells under the GIVEN column.
-
Click the first property cell,
-
From Test Tools → Test Editor, select and expand the LoanApplication data object.
-
Click amount and then Add to map the data object field to the property cell.
-
-
Click the second property cell,
-
From Test Tools → Test Editor, select and expand the LoanApplication data object.
-
Click deposit and then Add.
-
-
Click the third property cell,
-
From Test Tools → Test Editor, select and expand the LoanApplication data object.
-
Click lengthYears and then Add.
-
-
Right-click LoanApplication header cell and select Insert column right. A new GIVEN column to the right is created.
-
Click the new header cell,
-
From Test Tools → Test Editor, select the IncomeSource data object.
-
Click Add to map the data object to the header cell.
-
-
Click the property cell below IncomeSource,
-
From Test Tools → Test Editor, select and expand the IncomeSource data object.
-
Click type and then Add to map the data object field to the property cell.
You have now defined all the GIVEN column cells.
-
-
Next, define the EXPECT column details,
-
Click the cell named INSTANCE 2 under the EXPECT column header.
-
From Test Tools → Test Editor, select LoanApplication data object.
-
Click Add.
-
-
To create properties of the data object, right-click the property cell and select Insert column right or Insert column left as required. Create two more property cells under the EXPECT column.
-
Click the first property cell,
-
From Test Tools → Test Editor, select and expand the LoanApplication data object.
-
Click approved and then Add to map the data object field to the property cell.
-
-
Click the second property cell,
-
From Test Tools → Test Editor, select and expand the LoanApplication data object.
-
Click insuranceCost and then Add.
-
-
Click the third property cell,
-
From Test Tools → Test Editor, select and expand the LoanApplication data object.
-
Click approvedRate and then Add.
-
-
Now for defining the test scenario, enter the following data in the first row:
-
Enter
Row 1 test scenario
as the Scenario Description,150000
as the amount,19000
as the deposit,30
as the lenghtYears, andAsset
as the type for the GIVEN column values. -
Enter
true
as approved and0
as the insuranceCost for the EXPECT column values.
-
-
Next enter the following data in the second row:
-
Enter
Row 2 test scenario
as the Scenario Description,100002
as the amount,2999
as the deposit,20
as the lenghtYears, andJob
as the type for the GIVEN column values. -
Enter
true
as approved and10
as the insuranceCost for the EXPECT column values.
-
-
After you have defined all GIVEN, EXPECT, and other data for the scenario, click Save in the test scenarios designer to save your work.
-
Click Run Test in the upper-right corner to run the
.scesim
file.The test result is displayed in the Test Report panel. Click View Alerts to display messages from the Alerts section. If a test fails, refer to the messages in the Alerts section at the bottom of the window, review and correct all components in the scenario, and try again to validate the scenario until the scenario passes.
-
Click Save in the test scenarios designer to save your work after you have made all necessary changes.
19.12.10. Test scenarios (legacy) designer in Business Central
Drools currently supports both the new Test Scenarios designer and the former Test Scenarios (Legacy) designer. The default designer is the new test scenarios designer, which supports testing of both rules and DMN models and provides an enhanced overall user experience with test scenarios. If required, you can continue to use the legacy test scenarios designer, which supports rule-based test scenarios only.
19.12.10.1. Creating and running a test scenario (legacy)
You can create test scenarios in Business Central to test the functionality of business rule data before deployment. A basic test scenario must have at least the following data:
-
Related data objects
-
GIVEN facts
-
EXPECT results
With this data, the test scenario can validate the expected and actual results for that rule instance based on the defined facts. You can also add a CALL METHOD and any available globals to a test scenario, but these scenario settings are optional.
-
In Business Central, go to Menu → Design → Projects and click the project name.
-
Click Add Asset → Test Scenarios (Legacy).
-
Enter an informative Test Scenario name and select the appropriate Package. The package that you specify must be the same package where the required rule assets have been assigned or will be assigned. You can import data objects from any package into the asset’s designer.
-
Click Ok to create the test scenario.
The new test scenario is now listed in the Test Scenarios panel of the Project Explorer,
-
Click the Data Objects tab to verify that all data objects required for the rules that you want to test are listed. If not, click New item to import the needed data objects from other packages, or within your package.
-
After all data objects are in place, return to the Model tab of the test scenarios designer and define the GIVEN and EXPECT data for the scenario, based on the available data objects.
Figure 335. The test scenarios designerThe GIVEN section defines the input facts for the test. For example, if an
Underage
rule in the project declines loan applications for applicants under the age of 21, then the GIVEN facts in the test scenario could beApplicant
withage
set to some integer less than 21.The EXPECT section defines the expected results based on the GIVEN input facts. That is, GIVEN the input facts, EXPECT these other facts to be valid or entire rules to be activated. For example, with the given facts of an applicant under the age of 21 in the scenario, the EXPECT results could be
LoanApplication
withapproved
set tofalse
(as a result of the underage applicant), or could be the activation of theUnderage
rule as a whole. -
Optionally, add a CALL METHOD and any globals to the test scenario:
-
CALL METHOD: Use this to invoke a method from another fact when the rule execution is initiated. Click CALL METHOD, select a fact, and click
to select the method to invoke. You can invoke any Java class methods (such as methods from an ArrayList) from the Java library or from a JAR that was imported for the project (if applicable).
-
globals: Use this to add any global variables in the project that you want to validate in the test scenario. Click globals to select the variable to be validated, and then in the test scenarios designer, click the global name and define field values to be applied to the global variable. If no global variables are available, then they must be created as new assets in Business Central. Global variables are named objects that are visible to the Drools engine but are different from the objects for facts. Changes in the object of a global do not trigger the re-evaluation of rules.
-
-
Click More at the bottom of the test scenarios designer to add other data blocks to the same scenario file as needed.
-
After you have defined all GIVEN, EXPECT, and other data for the scenario, click Save in the test scenarios designer to save your work.
-
Click Run scenario in the upper-right corner to run this
.scenario
file, or click Run all scenarios to run all saved.scenario
files in the project package (if there are multiple). Although the Run scenario option does not require the individual.scenario
file to be saved, the Run all scenarios option does require all.scenario
files to be saved.If the test fails, address any problems described in the Alerts message at the bottom of the window, review all components in the scenario, and try again to validate the scenario until the scenario passes.
-
Click Save in the test scenarios designer to save your work after all changes are complete.
19.12.10.2. Adding GIVEN facts in test scenarios (legacy)
The GIVEN section defines input facts for the test. For example, if an Underage
rule in the project declines loan applications for applicants under the age of 21, then the GIVEN facts in the test scenario could be Applicant
with age
set to some integer less than 21.
-
All data objects required for your test scenario have been created or imported and are listed in the Data Objects tab of the Test Scenarios (Legacy) designer.
-
In the Test Scenarios (Legacy) designer, click GIVEN to open the New input window with the available facts.
Figure 336. Add GIVEN input to the test scenarioThe list includes the following options, depending on the data objects available in the Data Objects tab of the test scenarios designer:
-
Insert a new fact: Use this to add a fact and modify its field values. Enter a variable for the fact as the Fact name.
-
Modify an existing fact: (Appears only after another fact has been added.) Use this to specify a previously inserted fact to be modified in the Drools engine between executions of the scenario.
-
Delete an existing fact: (Appears only after another fact has been added.) Use this to specify a previously inserted fact to be deleted from the Drools engine between executions of the scenario.
-
Activate rule flow group: Use this to specify a rule flow group to be activated so that all rules within that group can be tested.
-
-
Choose a fact for the desired input option and click Add. For example, set Insert a new fact: to Applicant and enter
a
orapp
or any other variable for the Fact name. -
Click the fact in the test scenarios designer and select the field to be modified.
Figure 337. Modify a fact field -
Click the edit icon (
) and select from the following field values:
-
Literal value: Creates an open field in which you enter a specific literal value.
-
Bound variable: Sets the value of the field to the fact bound to a selected variable. The field type must match the bound variable type.
-
Create new fact: Enables you to create a new fact and assign it as a field value of the parent fact. Then you can click the child fact in the test scenarios designer and likewise assign field values or nest other facts similarly.
-
-
Continue adding any other GIVEN input data for the scenario and click Save in the test scenarios designer to save your work.
19.12.10.3. Adding EXPECT results in test scenarios (legacy)
The EXPECT section defines the expected results based on the GIVEN input facts. That is, GIVEN the input facts, EXPECT other specified facts to be valid or entire rules to be activated. For example, with the given facts of an applicant under the age of 21 in the scenario, the EXPECT results could be LoanApplication
with approved
set to false
(as a result of the underage applicant), or could be the activation of the Underage
rule as a whole.
-
All data objects required for your test scenario have been created or imported and are listed in the Data Objects tab of the Test Scenarios (Legacy) designer.
-
In the Test Scenarios (Legacy) designer, click EXPECT to open the New expectation window with the available facts.
Figure 338. Add EXPECT results to the test scenarioThe list includes the following options, depending on the data in the GIVEN section and the data objects available in the Data Objects tab of the test scenarios designer:
-
Rule: Use this to specify a particular rule in the project that is expected to be activated as a result of the GIVEN input. Type the name of a rule that is expected to be activated or select it from the list of rules, and then in the test scenarios designer, specify the number of times the rule should be activated.
-
Fact value: Use this to select a fact and define values for it that are expected to be valid as a result of the facts defined in the GIVEN section. The facts are listed by the Fact name previously defined for the GIVEN input.
-
Any fact that matches: Use this to validate that at least one fact with the specified values exists as a result of the GIVEN input.
-
-
Choose a fact for the desired expectation (such as Fact value:
application
) and click Add or OK. -
Click the fact in the test scenarios designer and select the field to be added and modified.
Figure 339. Modify a fact field -
Set the field values to what is expected to be valid as a result of the GIVEN input (such as
approved
|equals
|false
). -
Continue adding any other EXPECT input data for the scenario and click Save in the test scenarios designer to save your work.
-
After you have defined and saved all GIVEN, EXPECT, and other data for the scenario, click Run scenario in the upper-right corner to run this
.scenario
file, or click Run all scenarios to run all saved.scenario
files in the project package (if there are multiple). Although the Run scenario option does not require the individual.scenario
file to be saved, the Run all scenarios option does require all.scenario
files to be saved.If the test fails, address any problems described in the Alerts message at the bottom of the window, review all components in the scenario, and try again to validate the scenario until the scenario passes.
-
Click Save in the test scenarios designer to save your work after all changes are complete.
20. Business Central integration
20.1. Knowledge Store REST API for Business Central spaces and projects
Drools provides a Knowledge Store REST API that you can use to interact with your projects and spaces in Drools without using the Business Central user interface. The Knowledge Store is the artifact repository for assets in Drools. This API support enables you to facilitate and automate maintenance of Business Central projects and spaces.
With the Knowledge Store REST API, you can perform the following actions:
-
Retrieve information about all projects and spaces
-
Create, update, or delete projects and spaces
-
Build, deploy, and test projects
-
Retrieve information about previous Knowledge Store REST API requests, or jobs
Knowledge Store REST API requests require the following components:
- Authentication
-
The Knowledge Store REST API requires HTTP Basic authentication or token-based authentication for the user role
rest-all
. To view configured user roles for your Drools distribution, navigate to~/$SERVER_HOME/standalone/configuration/application-roles.properties
and~/application-users.properties
.To add a user with the
rest-all
role, navigate to~/$SERVER_HOME/bin
and run the following command:$ ./add-user.sh -a --user <USERNAME> --password <PASSWORD> --role rest-all
For more information about user roles and Drools installation options, see Installing the KIE Server.
- HTTP headers
-
The Knowledge Store REST API requires the following HTTP headers for API requests:
-
Accept
: Data format accepted by your requesting client:-
application/json
(JSON)
-
-
Content-Type
: Data format of yourPOST
orPUT
API request data:-
application/json
(JSON)
-
-
- HTTP methods
-
The Knowledge Store REST API supports the following HTTP methods for API requests:
-
GET
: Retrieves specified information from a specified resource endpoint -
POST
: Creates or updates a resource -
DELETE
: Deletes a resource
-
- Base URL
-
The base URL for Knowledge Store REST API requests is
http://SERVER:PORT/business-central/rest/
, such ashttp://localhost:8080/business-central/rest/
.The REST API base URL for the Knowledge Store and for the Drools controller built in to Business Central are the same because both are considered part of Business Central REST services. - Endpoints
-
Knowledge Store REST API endpoints, such as
/spaces/{spaceName}
for a specified space, are the URIs that you append to the Knowledge Store REST API base URL to access the corresponding resource or type of resource in Drools.Example request URL for/spaces/{spaceName}
endpointhttp://localhost:8080/business-central/rest/spaces/MySpace
- Request data
-
HTTP
POST
requests in the Knowledge Store REST API may require a JSON request body with data to accompany the request.Example POST request URL and JSON request body datahttp://localhost:8080/business-central/rest/spaces/MySpace/projects
{ "name": "Employee_Rostering", "groupId": "employeerostering", "version": "1.0.0-SNAPSHOT", "description": "Employee rostering problem optimisation using Planner. Assigns employees to shifts based on their skill." }
20.1.1. Sending requests with the Knowledge Store REST API using a REST client or curl utility
The Knowledge Store REST API enables you to interact with your projects and spaces in Drools without using the Business Central user interface. You can send Knowledge Store REST API requests using any REST client or curl utility.
-
Business Central is installed and running.
-
You have
rest-all
user role access to Business Central.
-
Identify the relevant API endpoint to which you want to send a request, such as
[GET] /spaces
to retrieve spaces in Business Central. -
In a REST client or curl utility, enter the following components for a
GET
request to/spaces
. Adjust any request details according to your use case.For REST client:
-
Authentication: Enter the user name and password of the Business Central user with the
rest-all
role. -
HTTP Headers: Set the following header:
-
Accept
:application/json
-
-
HTTP method: Set to
GET
. -
URL: Enter the Knowledge Store REST API base URL and endpoint, such as
http://localhost:8080/business-central/rest/spaces
.
For curl utility:
-
-u
: Enter the user name and password of the Business Central user with therest-all
role. -
-H
: Set the following header:-
accept
:application/json
-
-
-X
: Set toGET
. -
URL: Enter the Knowledge Store REST API base URL and endpoint, such as
http://localhost:8080/business-central/rest/spaces
.
curl -u 'baAdmin:password@1' -H "accept: application/json" -X GET "http://localhost:8080/business-central/rest/spaces"
-
-
Execute the request and review the KIE Server response.
Example server response (JSON):
[ { "name": "MySpace", "description": null, "projects": [ { "name": "Employee_Rostering", "spaceName": "MySpace", "groupId": "employeerostering", "version": "1.0.0-SNAPSHOT", "description": "Employee rostering problem optimisation using Planner. Assigns employees to shifts based on their skill.", "publicURIs": [ { "protocol": "git", "uri": "git://localhost:9418/MySpace/example-Employee_Rostering" }, { "protocol": "ssh", "uri": "ssh://localhost:8001/MySpace/example-Employee_Rostering" } ] }, { "name": "Mortgage_Process", "spaceName": "MySpace", "groupId": "mortgage-process", "version": "1.0.0-SNAPSHOT", "description": "Getting started loan approval process in BPMN2, decision table, business rules, and forms.", "publicURIs": [ { "protocol": "git", "uri": "git://localhost:9418/MySpace/example-Mortgage_Process" }, { "protocol": "ssh", "uri": "ssh://localhost:8001/MySpace/example-Mortgage_Process" } ] } ], "owner": "admin", "defaultGroupId": "com.myspace" }, { "name": "MySpace2", "description": null, "projects": [ { "name": "IT_Orders", "spaceName": "MySpace", "groupId": "itorders", "version": "1.0.0-SNAPSHOT", "description": "Case Management IT Orders project", "publicURIs": [ { "protocol": "git", "uri": "git://localhost:9418/MySpace/example-IT_Orders-1" }, { "protocol": "ssh", "uri": "ssh://localhost:8001/MySpace/example-IT_Orders-1" } ] } ], "owner": "admin", "defaultGroupId": "com.myspace" } ]
-
In your REST client or curl utility, send another API request with the following components for a
POST
request to/spaces/{spaceName}/projects
to create a project within a space. Adjust any request details according to your use case.For REST client:
-
Authentication: Enter the user name and password of the Business Central user with the
rest-all
role. -
HTTP Headers: Set the following header:
-
Accept
:application/json
-
Content-Type
:application/json
-
-
HTTP method: Set to
POST
. -
URL: Enter the Knowledge Store REST API base URL and endpoint, such as
http://localhost:8080/business-central/rest/spaces/MySpace/projects
. -
Request body: Add a JSON request body with the identification data for the new project:
{ "name": "Employee_Rostering", "groupId": "employeerostering", "version": "1.0.0-SNAPSHOT", "description": "Employee rostering problem optimisation using Planner. Assigns employees to shifts based on their skill." }
For curl utility:
-
-u
: Enter the user name and password of the Business Central user with therest-all
role. -
-H
: Set the following headers:-
accept
:application/json
-
content-type
:application/json
-
-
-X
: Set toPOST
. -
URL: Enter the Knowledge Store REST API base URL and endpoint, such as
http://localhost:8080/business-central/rest/spaces/MySpace/projects
. -
-d
: Add a JSON request body or file (@file.json
) with the identification data for the new project:
curl -u 'baAdmin:password@1' -H "accept: application/json" -H "content-type: application/json" -X POST "http://localhost:8080/business-central/rest/spaces/MySpace/projects" -d "{ \"name\": \"Employee_Rostering\", \"groupId\": \"employeerostering\", \"version\": \"1.0.0-SNAPSHOT\", \"description\": \"Employee rostering problem optimisation using Planner. Assigns employees to shifts based on their skill.\"}"
curl -u 'baAdmin:password@1' -H "accept: application/json" -H "content-type: application/json" -X POST "http://localhost:8080/business-central/rest/spaces/MySpace/projects" -d @my-project.json
-
-
Execute the request and review the KIE Server response.
Example server response (JSON):
{ "jobId": "1541017411591-6", "status": "APPROVED", "spaceName": "MySpace", "projectName": "Employee_Rostering", "projectGroupId": "employeerostering", "projectVersion": "1.0.0-SNAPSHOT", "description": "Employee rostering problem optimisation using Planner. Assigns employees to shifts based on their skill." }
If you encounter request errors, review the returned error code messages and adjust your request accordingly.
20.1.2. Supported Knowledge Store REST API endpoints
The Knowledge Store REST API provides endpoints for managing spaces and projects in Drools and for retrieving information about previous Knowledge Store REST API requests, or jobs.
20.1.2.1. Spaces
The Knowledge Store REST API supports the following endpoints for managing spaces in Business Central. The Knowledge Store REST API base URL is http://SERVER:PORT/business-central/rest/
. All requests require HTTP Basic authentication or token-based authentication for the rest-all
user role.
- [GET] /spaces
-
Returns all spaces in Business Central.
Example server response (JSON)[ { "name": "MySpace", "description": null, "projects": [ { "name": "Employee_Rostering", "spaceName": "MySpace", "groupId": "employeerostering", "version": "1.0.0-SNAPSHOT", "description": "Employee rostering problem optimisation using Planner. Assigns employees to shifts based on their skill.", "publicURIs": [ { "protocol": "git", "uri": "git://localhost:9418/MySpace/example-Employee_Rostering" }, { "protocol": "ssh", "uri": "ssh://localhost:8001/MySpace/example-Employee_Rostering" } ] }, { "name": "Mortgage_Process", "spaceName": "MySpace", "groupId": "mortgage-process", "version": "1.0.0-SNAPSHOT", "description": "Getting started loan approval process in BPMN2, decision table, business rules, and forms.", "publicURIs": [ { "protocol": "git", "uri": "git://localhost:9418/MySpace/example-Mortgage_Process" }, { "protocol": "ssh", "uri": "ssh://localhost:8001/MySpace/example-Mortgage_Process" } ] } ], "owner": "admin", "defaultGroupId": "com.myspace" }, { "name": "MySpace2", "description": null, "projects": [ { "name": "IT_Orders", "spaceName": "MySpace", "groupId": "itorders", "version": "1.0.0-SNAPSHOT", "description": "Case Management IT Orders project", "publicURIs": [ { "protocol": "git", "uri": "git://localhost:9418/MySpace/example-IT_Orders-1" }, { "protocol": "ssh", "uri": "ssh://localhost:8001/MySpace/example-IT_Orders-1" } ] } ], "owner": "admin", "defaultGroupId": "com.myspace" } ]
- [GET] /spaces/{spaceName}
-
Returns information about a specified space.
Table 62. Request parameters Name Description Type Requirement spaceName
Name of the space to be retrieved
String
Required
Example server response (JSON){ "name": "MySpace", "description": null, "projects": [ { "name": "Mortgage_Process", "spaceName": "MySpace", "groupId": "mortgage-process", "version": "1.0.0-SNAPSHOT", "description": "Getting started loan approval process in BPMN2, decision table, business rules, and forms.", "publicURIs": [ { "protocol": "git", "uri": "git://localhost:9418/MySpace/example-Mortgage_Process" }, { "protocol": "ssh", "uri": "ssh://localhost:8001/MySpace/example-Mortgage_Process" } ] }, { "name": "Employee_Rostering", "spaceName": "MySpace", "groupId": "employeerostering", "version": "1.0.0-SNAPSHOT", "description": "Employee rostering problem optimisation using Planner. Assigns employees to shifts based on their skill.", "publicURIs": [ { "protocol": "git", "uri": "git://localhost:9418/MySpace/example-Employee_Rostering" }, { "protocol": "ssh", "uri": "ssh://localhost:8001/MySpace/example-Employee_Rostering" } ] }, { "name": "Evaluation_Process", "spaceName": "MySpace", "groupId": "evaluation", "version": "1.0.0-SNAPSHOT", "description": "Getting started Business Process for evaluating employees", "publicURIs": [ { "protocol": "git", "uri": "git://localhost:9418/MySpace/example-Evaluation_Process" }, { "protocol": "ssh", "uri": "ssh://localhost:8001/MySpace/example-Evaluation_Process" } ] }, { "name": "IT_Orders", "spaceName": "MySpace", "groupId": "itorders", "version": "1.0.0-SNAPSHOT", "description": "Case Management IT Orders project", "publicURIs": [ { "protocol": "git", "uri": "git://localhost:9418/MySpace/example-IT_Orders" }, { "protocol": "ssh", "uri": "ssh://localhost:8001/MySpace/example-IT_Orders" } ] } ], "owner": "admin", "defaultGroupId": "com.myspace" }
- [POST] /spaces
-
Creates a space in Business Central.
Table 63. Request parameters Name Description Type Requirement body
The
name
,description
,owner
,defaultGroupId
, and any other components of the new spaceRequest body
Required
Example request body (JSON){ "name": "NewSpace", "description": "My new space.", "owner": "admin", "defaultGroupId": "com.newspace" }
Example server response (JSON){ "jobId": "1541016978154-3", "status": "APPROVED", "spaceName": "NewSpace", "owner": "admin", "defaultGroupId": "com.newspace", "description": "My new space." }
- [DELETE] /spaces/{spaceName}
-
Deletes a specified space from Business Central.
Table 64. Request parameters Name Description Type Requirement spaceName
Name of the space to be deleted
String
Required
Example server response (JSON){ "jobId": "1541127032997-8", "status": "APPROVED", "spaceName": "MySpace", "owner": "admin", "description": "My deleted space.", "repositories": null }
20.1.2.2. Projects
The Knowledge Store REST API supports the following endpoints for managing, building, and deploying projects in Business Central. The Knowledge Store REST API base URL is http://SERVER:PORT/business-central/rest/
. All requests require HTTP Basic authentication or token-based authentication for the rest-all
user role.
- [GET] /spaces/{spaceName}/projects
-
Returns projects in a specified space.
Table 65. Request parameters Name Description Type Requirement spaceName
Name of the space for which you are retrieving projects
String
Required
Example server response (JSON)[ { "name": "Mortgage_Process", "spaceName": "MySpace", "groupId": "mortgage-process", "version": "1.0.0-SNAPSHOT", "description": "Getting started loan approval process in BPMN2, decision table, business rules, and forms.", "publicURIs": [ { "protocol": "git", "uri": "git://localhost:9418/MySpace/example-Mortgage_Process" }, { "protocol": "ssh", "uri": "ssh://localhost:8001/MySpace/example-Mortgage_Process" } ] }, { "name": "Employee_Rostering", "spaceName": "MySpace", "groupId": "employeerostering", "version": "1.0.0-SNAPSHOT", "description": "Employee rostering problem optimisation using Planner. Assigns employees to shifts based on their skill.", "publicURIs": [ { "protocol": "git", "uri": "git://localhost:9418/MySpace/example-Employee_Rostering" }, { "protocol": "ssh", "uri": "ssh://localhost:8001/MySpace/example-Employee_Rostering" } ] }, { "name": "Evaluation_Process", "spaceName": "MySpace", "groupId": "evaluation", "version": "1.0.0-SNAPSHOT", "description": "Getting started Business Process for evaluating employees", "publicURIs": [ { "protocol": "git", "uri": "git://localhost:9418/MySpace/example-Evaluation_Process" }, { "protocol": "ssh", "uri": "ssh://localhost:8001/MySpace/example-Evaluation_Process" } ] }, { "name": "IT_Orders", "spaceName": "MySpace", "groupId": "itorders", "version": "1.0.0-SNAPSHOT", "description": "Case Management IT Orders project", "publicURIs": [ { "protocol": "git", "uri": "git://localhost:9418/MySpace/example-IT_Orders" }, { "protocol": "ssh", "uri": "ssh://localhost:8001/MySpace/example-IT_Orders" } ] } ]
- [GET] /spaces/{spaceName}/projects/{projectName}
-
Returns information about a specified project in a specified space.
Table 66. Request parameters Name Description Type Requirement spaceName
Name of the space where the project is located
String
Required
projectName
Name of the project to be retrieved
String
Required
Example server response (JSON){ "name": "Employee_Rostering", "spaceName": "MySpace", "groupId": "employeerostering", "version": "1.0.0-SNAPSHOT", "description": "Employee rostering problem optimisation using Planner. Assigns employees to shifts based on their skill.", "publicURIs": [ { "protocol": "git", "uri": "git://localhost:9418/MySpace/example-Employee_Rostering" }, { "protocol": "ssh", "uri": "ssh://localhost:8001/MySpace/example-Employee_Rostering" } ] }
- [POST] /spaces/{spaceName}/projects
-
Creates a project in a specified space.
Table 67. Request parameters Name Description Type Requirement spaceName
Name of the space in which the new project will be created
String
Required
body
The
name
,groupId
,version
,description
, and any other components of the new projectRequest body
Required
Example request body (JSON){ "name": "Employee_Rostering", "groupId": "employeerostering", "version": "1.0.0-SNAPSHOT", "description": "Employee rostering problem optimisation using Planner. Assigns employees to shifts based on their skill." }
Example server response (JSON){ "jobId": "1541017411591-6", "status": "APPROVED", "spaceName": "MySpace", "projectName": "Employee_Rostering", "projectGroupId": "employeerostering", "projectVersion": "1.0.0-SNAPSHOT", "description": "Employee rostering problem optimisation using Planner. Assigns employees to shifts based on their skill." }
- [DELETE] /spaces/{spaceName}/projects/{projectName}
-
Deletes a specified project from a specified space.
Table 68. Request parameters Name Description Type Requirement spaceName
Name of the space where the project is located
String
Required
projectName
Name of the project to be deleted
String
Required
Example server response (JSON){ "jobId": "1541128617727-10", "status": "APPROVED", "projectName": "Employee_Rostering", "spaceName": "MySpace" }
- [POST] /spaces/{spaceName}/git/clone
-
Clones a project into a specified space from a specified Git address.
Table 69. Request parameters Name Description Type Requirement spaceName
Name of the space to which you are cloning a project
String
Required
body
The
name
,description
, and Git repositoryuserName
,password
, andgitURL
for the project to be clonedRequest body
Required
Example request body (JSON){ "name": "Employee_Rostering", "description": "Employee rostering problem optimisation using Planner. Assigns employees to shifts based on their skill.", "userName": "baAdmin", "password": "password@1", "gitURL": "git://localhost:9418/MySpace/example-Employee_Rostering" }
Example server response (JSON){ "jobId": "1541129488547-13", "status": "APPROVED", "cloneProjectRequest": { "name": "Employee_Rostering", "description": "Employee rostering problem optimisation using Planner. Assigns employees to shifts based on their skill.", "userName": "baAdmin", "password": "password@1", "gitURL": "git://localhost:9418/MySpace/example-Employee_Rostering" }, "spaceName": "MySpace2" }
- [POST] /spaces/{spaceName}/projects/{projectName}/maven/compile
-
Compiles a specified project in a specified space (equivalent to
mvn compile
).Table 70. Request parameters Name Description Type Requirement spaceName
Name of the space where the project is located
String
Required
projectName
Name of the project to be compiled
String
Required
Example server response (JSON){ "jobId": "1541128617727-10", "status": "APPROVED", "projectName": "Employee_Rostering", "spaceName": "MySpace" }
- [POST] /spaces/{spaceName}/projects/{projectName}/maven/test
-
Tests a specified project in a specified space (equivalent to
mvn test
).Table 71. Request parameters Name Description Type Requirement spaceName
Name of the space where the project is located
String
Required
projectName
Name of the project to be tested
String
Required
Example server response (JSON){ "jobId": "1541132591595-19", "status": "APPROVED", "projectName": "Employee_Rostering", "spaceName": "MySpace" }
- [POST] /spaces/{spaceName}/projects/{projectName}/maven/install
-
Installs a specified project in a specified space (equivalent to
mvn install
).Table 72. Request parameters Name Description Type Requirement spaceName
Name of the space where the project is located
String
Required
projectName
Name of the project to be installed
String
Required
Example server response (JSON){ "jobId": "1541132668987-20", "status": "APPROVED", "projectName": "Employee_Rostering", "spaceName": "MySpace" }
- [POST] /spaces/{spaceName}/projects/{projectName}/maven/deploy
-
Deploys a specified project in a specified space (equivalent to
mvn deploy
).Table 73. Request parameters Name Description Type Requirement spaceName
Name of the space where the project is located
String
Required
projectName
Name of the project to be deployed
String
Required
Example server response (JSON){ "jobId": "1541132816435-21", "status": "APPROVED", "projectName": "Employee_Rostering", "spaceName": "MySpace" }
20.1.2.3. Jobs (API requests)
All POST
and DELETE
requests in the Knowledge Store REST API return a job ID associated with each request, in addition to the returned request details. You can use a job ID to view the request status or delete a sent request.
Knowledge Store REST API requests, or jobs, can have the following statuses:
Status | Description |
---|---|
|
The request was accepted and is being processed. |
|
The request contained incorrect content and was not accepted. |
|
The requested resource (path) does not exist. |
|
The resource already exists. |
|
An error occurred in KIE Server. |
|
The request finished successfully. |
|
The request failed. |
|
The request was approved. |
|
The request was denied. |
|
The job ID for the request could not be found due to one of the following reasons:
|
The Knowledge Store REST API supports the following endpoints for retrieving or deleting sent API requests. The Knowledge Store REST API base URL is http://SERVER:PORT/business-central/rest/
. All requests require HTTP Basic authentication or token-based authentication for the rest-all
user role.
- [GET] /jobs/{jobId}
-
Returns the status of a specified job (a previously sent API request).
Table 75. Request parameters Name Description Type Requirement jobId
ID of the job to be retrieved (example:
1541010216919-1
)String
Required
Example server response (JSON){ "status": "SUCCESS", "jobId": "1541010216919-1", "result": null, "lastModified": 1541010218352, "detailedResult": [ "level:INFO, path:null, text:Build of module 'Mortgage_Process' (requested by system) completed.\n Build: SUCCESSFUL" ] }
- [DELETE] /jobs/{jobId}
-
Deletes a specified job (a previously sent API request). If the job is not being processed yet, this request removes the job from the job queue. This request does not cancel or stop an ongoing job.
Table 76. Request parameters Name Description Type Requirement jobId
ID of the job to be deleted (example:
1541010216919-1
)String
Required
Example server response (JSON){ "status": "GONE", "jobId": "1541010216919-1", "result": null, "lastModified": 1541132054916, "detailedResult": [ "level:INFO, path:null, text:Build of module 'Mortgage_Process' (requested by system) completed.\n Build: SUCCESSFUL" ] }
20.2. Embedded Drools controller calls
When running Business Central with the embedded Drools controller mode, a series of endpoints related to managing all aspects of KIE Server templates, instances, and containers are also available. For more details, see Drools controller REST API. A Java client API is also available for interacting with these endpoints.
20.3. Keycloak SSO integration
Single Sign On (SSO) and related token exchange mechanisms are becoming the most common scenario for the authentication and authorization in different environments on the web, specially when moving into the cloud.
This section talks about the integration of Keycloak with jBPM or Drools applications in order to use all the features provided on Keycloak. Keycloak is an integrated SSO and IDM for browser applications and RESTful web services. Lean more about it in the Keycloak’s home page.
The result of the integration with Keycloak has lots of advantages such as:
-
Provide an integrated SSO and IDM environment for different clients, including Business Central
-
Social logins - use your Facebook, Google, LinkedIn, etc accounts
-
User session management
-
And much more…
Next sections cover the following integration points with Keycloak:
-
Business Central authentication through a Keycloak server
It basically consists of securing both web client and remote service clients through the Keycloak SSO. So either web interface or remote service consumers (whether a user or a service) will authenticate into trough KC.
-
Execution server authentication through a Keycloak server
Consists of securing the remote services provided by the execution server (as it does not provide web interface). Any remote service consumer (whether a user or a service) will authenticate trough KC.
-
Consuming remote services
This section describes how a third party clients can consume the remote service endpoints provided by both Business Central and Execution Server, such as the REST API or remote file system services.
-
Keycloak and Business Central’s security administration area
20.3.1. Scenario
Consider the following diagram as the environment for this document’s example:
Keycloak is a standalone process that provides remote authentication, authorization and administration services that can be potentially consumed by one or more jBPM applications over the network.
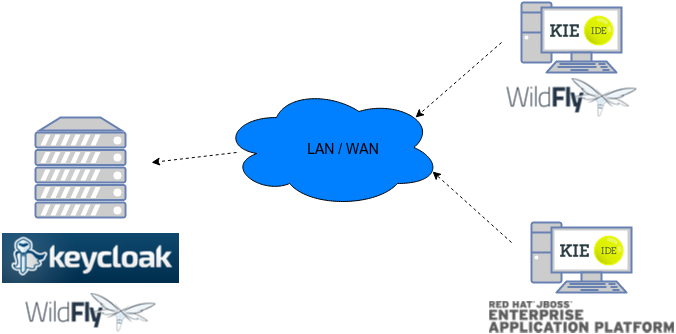
Consider these main steps for building this environment:
-
Install and set up a Keycloak server
-
Create and set up a Realm for this example - Configure realm’s clients, users and roles
-
Install and set up the SSO client adapter & jBPM application
Note: The resulting environment and the different configurations for this document are based on Business Central.
20.3.2. Install and set up a Keycloak server
Keycloak provides an extensive documentation and several articles about the installation on different environments. This section describes the minimal set up for being able to build the integrated environment for the example. Please refer to the Keycloak documentation if you need more information.
Here are the steps for a minimal Keycloak installation and set up:
-
Download latest version of Keycloak from the Downloads section. This example is based on Keycloak 1.9.0.Final
-
Unzip the downloaded distribution of Keycloak into a folder, let’s refer it as
$KC_HOME
-
Run the KC server - This example is based on running both Keycloak and jBPM on same host. In order to avoid port conflicts you can use a port offset for the Keycloak’s server as:
$KC_HOME/bin/standalone.sh -Djboss.socket.binding.port-offset=100
-
Create a Keycloak’s administration 'admin' user by navigating to http://localhost:8180/auth/
The Keycloak administration console will be available at http://localhost:8180/auth/admin/.
20.3.3. Create and set up the demo realm
Security realms are used to restrict the access for the different application’s resources.
Once the Keycloak server is running next step is about creating a realm. This realm will provide the different users, roles, sessions, etc for the jBPM application/s.
Keycloak provides several examples for the realm creation and management, from the official examples to different articles with more examples.
Follow these steps in order to create the demo realm used later in this document:
-
Go to the Keycloak administration console and click on Add realm button. Give it the name demo.
-
Go to the Clients section (from the main admin console menu) and create a new client for the demo realm:
-
Client ID: kie
-
Client protocol: openid-connect
-
Acces type: confidential
-
Root URL: http://localhost:8080
-
Base URL: /business-central-x.y.z.Final
-
Redirect URIs: /business-central-x.y.z.Final/*
-
The resulting kie client settings screen:
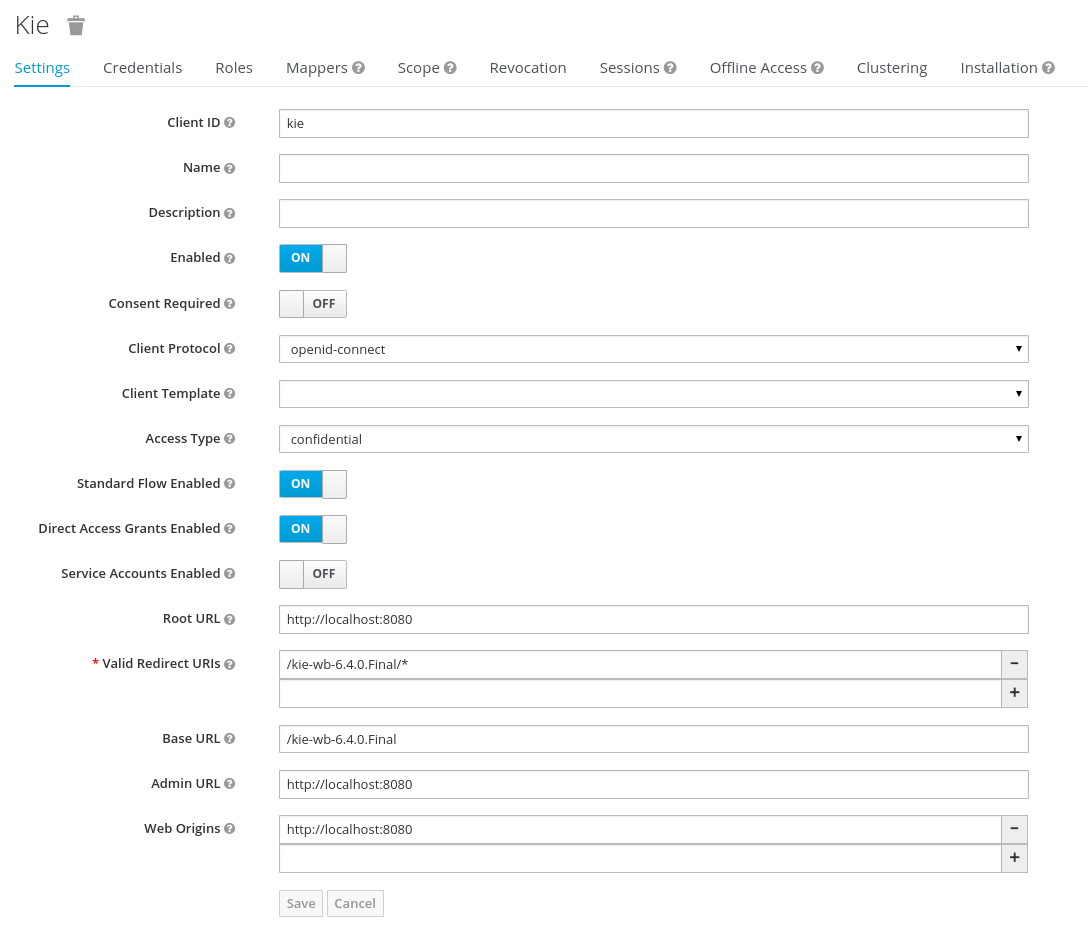
As you can see in the above settings it’s being considered the value business-central-x.y.z.Final for the application’s context path. If your jBPM application will be deployed on a different context path, host or port, just use your concrete settings here. |
Last step for being able to use the demo realm from Business Central is create the application’s user and roles:
-
Go to the Roles section and create the roles admin, kiemgmt and rest-all
-
Go to the Users section and create the admin user. Set the password with value password in the credentials tab, unset the temporary switch.
-
In the Users section navigate to the Role Mappings tab and assign the admin, kiemgmt and rest-all roles to the admin user
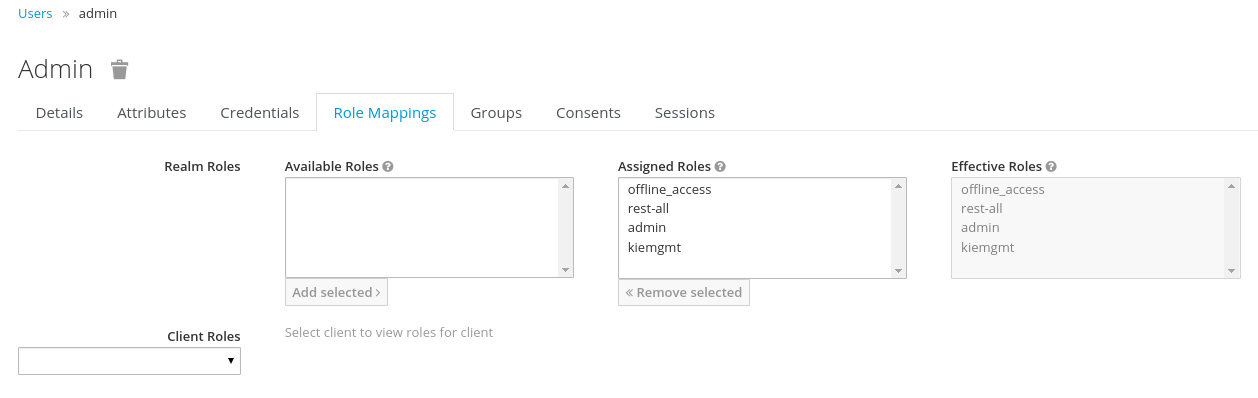
At this point a Keycloak server is running on the host, set up with a minimal configuration set. Let’s move to Business Central set up.
20.3.4. Install and set up Business Central
For this tutorial let’s use a Wildfly as the application server for Business Central, as the jBPM installer does by default.
Let’s assume, after running the jBPM installer, the $JBPM_HOME as the root path for the Wildfly server where the application has been deployed.
20.3.4.1. Install the KC adapter
In order to use the Keycloak’s authentication and authorization modules from the jBPM application, the Keycloak JBoss EAP/Wildfly Adapter must be installed on our server at $JBPM_HOME. Keycloak provides multiple adapters for different containers out of the box, if you are using another container or need to use another adapter, please take a look at the Securing Applications section from the Keycloak docs. Here are the steps to install and set up the adapter for Wildfly 11/10/9:
-
Download the adapter from Keycloak Client Adapter for Wildfly 11/10/9
-
Execute the following commands on your shell:
cd $JBPM_HOME unzip keycloak-wildfly-adapter-dist-3.4.3.Final.zip // Install the KC client adapter cd $JBPM_HOME/bin ./standalone.sh -c standalone-full.xml // set up the KC client adapter. // ** Once server is up, open a new command line terminal and run: cd $JBPM_HOME/bin ./jboss-cli.sh -c --file=adapter-install.cli
20.3.4.2. Configure the KC adapter
Once installed the KC adapter into Wildfly, next step is to configure the adapter in order to specify different settings such as the location for the authentication server, the realm to use and so on.
Keycloak provides two ways of configuring the adapter:
-
Per WAR configuration
-
Via Keycloak subsystem
In this example let’s use the second option, use the Keycloak subsystem, so our WAR is free from this kind of settings. If you want to use the per WAR approach, please take a look Required Per WAR Configuration.
Edit the configuration file $JBPM_HOME/standalone/configuration/standalone-full.xml and locate the subsystem configuration section. Add the following content:
<subsystem xmlns="urn:jboss:domain:keycloak:1.1">
<secure-deployment name="business-central-x.y.z.Final.war">
<realm>demo</realm>
<realm-public-key>MIIBIjANBgkqhkiG9w0BAQEFAAOCA...</realm-public-key>
<auth-server-url>http://localhost:8180/auth</auth-server-url>
<ssl-required>external</ssl-required>
<resource>kie</resource>
<enable-basic-auth>true</enable-basic-auth>
<credential name="secret">925f9190-a7c1-4cfd-8a3c-004f9c73dae6</credential>
<principal-attribute>preferred_username</principal-attribute>
</secure-deployment>
</subsystem>
If you have imported the example json files from this document in step 2, you can just use same configuration as above by using your concrete deployment name. Otherwise please use your values for these configurations:
-
Name for the secure deployment - Use your concrete application’s WAR file name
-
Realm - Is the realm that the applications will use, in our example, the demo realm created the previous step.
-
Realm Public Key - Provide here the public key for the demo realm. It’s not mandatory, if it’s not specified, it will be retrieved from the server. Otherwise, you can find it in the Keycloak admin console → Realm settings (for demo realm) → Keys
-
Authentication server URL - The URL for the Keycloak’s authentication server
-
Resource - The name for the client created on step 2. In our example, use the value kie.
-
Enable basic auth - For this example let’s enable Basic authentication mechanism as well, so clients can use both Token (Bearer) and Basic approaches to perform the requests.
-
Credential - Use the password value for the kie client. You can find it in the Keycloak admin console → Clients → kie → Credentials tab → Copy the value for the secret.
For this example you have to take care about using your concrete values for secure-deployment name, realm-public-key and credential password.
Ensure the following tag is NOT present in the Widfly/EAP profile’s configuration file (eg: standalone.xml):
It’s enabled by default in some server versions. If present, it must be removed/disabled in order to allow Keycloak to properly handle the clients. |
20.3.4.3. Run the environment
At this point a Keycloak server is up and running on the host, and the KC adapter is installed and configured for the jBPM application server. You can run the application using:
$JBPM_HOME/bin/standalone.sh -c standalone-full.xml
You can navigate into the application once the server is up at:
http://localhost:8080/business-central-x.y.z.Final
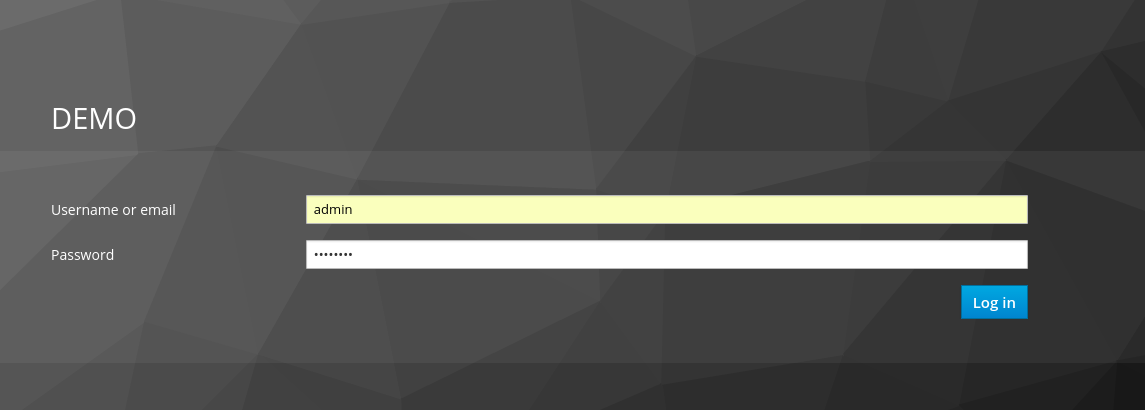
Use your Keycloak’s admin user credentials to login: admin/password.
20.3.5. Securing Business Central remote services via Keycloak
Business Central provides different remote service endpoints that can be consumed by third party clients using the Knowledge Store REST API.
In order to authenticate those services through Keycloak, apply those modifications for the WEB-INF/web.xml file (app deployment descriptor) from jBPM’s WAR file:
-
Constraint the remote services URL patterns as:
<security-constraint> <web-resource-collection> <web-resource-name>remote-services</web-resource-name> <url-pattern>/rest/*</url-pattern> <url-pattern>/maven2/*</url-pattern> <url-pattern>/ws/*</url-pattern> </web-resource-collection> <auth-constraint> <role-name>rest-all</role-name> </auth-constraint> </security-constraint>
The user that consumes the remote services must be member of role rest-all. As on described previous steps, the admin user in this example it’s already a member of the rest-all role. |
20.3.6. Securing Business Central's file system services via Keycloak
In order to consume other remote services such as the file system ones (e.g. remote GIT), a specific Keycloak login module must be used for the application’s security domain in the $JBPM_HOME/standalone/configuration/standalone-full.xml file. By default Business Central uses the other security domain, so the resulting configuration on the $JBPM_HOME/standalone/configuration/standalone-full.xml should be such as:
<security-domain name="other" cache-type="default">
<authentication>
<login-module code="org.keycloak.adapters.jaas.DirectAccessGrantsLoginModule" flag="required">
<!-- Parameter value can be a file system absolute path or a classpath (e.g. "classpath:/some-path/kie-git.json")-->
<module-option name="keycloak-config-file" value="$JBPM_HOME/kie-git.json"/>
</login-module>
</authentication>
</security-domain>
Note that:
-
The login modules on the other security domain in the $JBPM_HOME/standalone/configuration/standalone-full.xml file must be REPLACED by the above given one.
-
Replace $JBPM_HOME/kie-git.json by the path (on file system) or the classpath (e.g. classpath:/some-path/kie-git.json) for the json configuration file used for the remote services client. Please continue reading in order to create this Keycloak client and how to obtain this json file.
At this point, remote services that use JAAS for the authentication process, such as the file system ones (e.g. GIT), are secured by Keycloak using the client specified in the above json configuration file. So let’s create this client on Keycloak and generate the required JSON file:
-
Navigate to the KC administration console and create a new client for the demo realm using kie-git as name.
-
Enable Direct Access Grants Enabled option
-
Disable Standard Flow Enabled option
-
Use a confidential access type for this client. See below image as example:
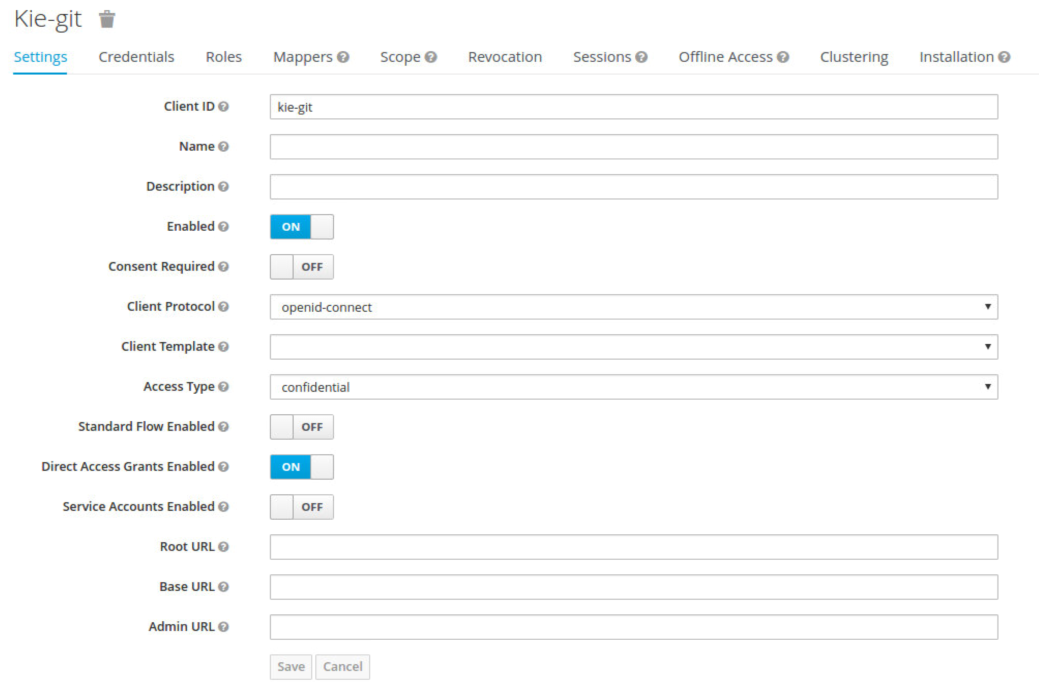
-
Go to the Installation tab in same kie-git client configuration screen and export using the Keycloak OIDC JSON type.
-
Finally copy this generated JSON file into an accessible directory on the server’s file system or add it in the application’s classpath. Use this path value as the keycloak-config-file argument for the above configuration of the org.keycloak.adapters.jaas.DirectAccessGrantsLoginModule login module.
-
More information about Keycloak JAAS Login modules can be found Keycloak JAAS plugin.
At this point, the internal Git repositories can be cloned by all users authenticated via the Keycloak server:
# Command example:
git clone ssh://admin@localhost:8001/system
20.3.7. Execution server
The KIE Execution Server provides a REST API that can be consumed for any third party clients. This this section is about how to integration the KIE Execution Server with the Keycloak SSO in order to delegate the third party clients identity management to the SSO server.
Consider the above environment running, so consider having:
-
A Keycloak server running and listening on http://localhost:8180/auth
-
A realm named demo with a client named kie for Business Central
-
A Business Central running at http://localhost:8080/business-central-x.y.z.Final
Follow these steps in order to add an execution server into this environment:
-
Create the client for the execution server on Keycloak
-
Install set up and the Execution server (with the KC client adapter)
20.3.7.1. Create the execution server’s client on Keycloak
As per each execution server is going to be deployed, you have to create a new client on the demo realm in Keycloak:
-
Go to the KC admin console → Clients → New client
-
Name: kie-execution-server
-
Root URL: http://localhost:8280/
-
Client protocol: openid-connect
-
Access type: confidential (or public if you want so, but not recommended for production environments)
-
Valid redirect URIs: /kie-server-x.y.z.Final/*
-
Base URL: /kie-server-x.y.z.Final
In this example the admin user already created on previous steps is the one used for the client requests. So ensure that the admin user is member of the role kie-server in order to use the execution server’s remote services. If the role does not exist, create it.
Note: This example considers that the execution server will be configured to run using a port offset of 200, so the HTTP port will be available at localhost:8280.
20.3.7.2. Install and set up the KC adapter on the execution server
At this point, a client named kie-execution-server is ready on the KC server to use from the execution server.
Let’s install, set up and deploy the execution server:
-
Install another Wildfly server to use for the execution server and the KC client adapter as well. You can follow above instructions for Business Central or follow the securing applications guide
-
Edit the standalone-full.xml file from the Wildfly server’s configuration path and configure the KC subsystem adapter as:
<secure-deployment name="kie-server-x.y.z.Final.war"> <realm>demo</realm> <realm-public-key>MIGfMA0GCSqGSIb...</realm-public-key> <auth-server-url>http://localhost:8180/auth</auth-server-url> <ssl-required>external</ssl-required> <resource>kie-execution-server</resource> <enable-basic-auth>true</enable-basic-auth> <credential name="secret">e92ec68d-6177-4239-be05-28ef2f3460ff</credential> <principal-attribute>preferred_username</principal-attribute> </secure-deployment>
Consider your concrete environment settings if different from this example:
-
Secure deployment name → use the name of the execution server war file being deployed
-
Public key → Use the demo realm public key or leave it blank, the server will provide one if so
-
Resource → This time, instead of the kie client used in the Business Central configuration, use the kie-execution-server client
-
Enable basic auth → Up to you. You can enable Basic auth for third party service consumers
-
Credential → Use the secret key for the kie-execution-server client. You can find it in the Credentialstab of the KC admin console
20.3.7.3. Deploy and run the execution server
Just deploy the execution server in Wildfly using any of the available mechanisms. Run the execution server using this command:
$EXEC_SERVER_HOME/bin/standalone.sh -c standalone-full.xml -Djboss.socket.binding.port-offset=200 -Dorg.kie.server.id=<ID> -Dorg.kie.server.user=<USER> -Dorg.kie.server.pwd=<PWD> -Dorg.kie.server.location=<LOCATION_URL> -Dorg.kie.server.controller=<CONTROLLER_URL> -Dorg.kie.server.controller.user=<CONTROLLER_USER> -Dorg.kie.server.controller.pwd=<CONTOLLER_PASSWORD>
Example:
$EXEC_SERVER_HOME/bin/standalone.sh -c standalone-full.xml -Djboss.socket.binding.port-offset=200 -Dorg.kie.server.id=kieserver1 -Dorg.kie.server.user=admin -Dorg.kie.server.pwd=password -Dorg.kie.server.location=http://localhost:8280/kie-server-x.y.z.Final/services/rest/server -Dorg.kie.server.controller=http://localhost:8080/business-central-x.y.z.Final/rest/controller -Dorg.kie.server.controller.user=admin -Dorg.kie.server.controller.pwd=password
The users that will consume the execution server remote service endpoints must have the role kie-server assigned. So create and assign this role in the KC admin console for the users that will consume the execution server remote services. |
Once up, you can check the server status as (considered using Basic authentication for this request, see next Consuming remote services for more information):
curl http://admin:password@localhost:8280/kie-server-x.y.z.Final/services/rest/server/
20.3.8. Consuming remote services
In order to use the different remote services provided by Business Central or by an Execution Server, your client must be authenticated on the KC server and have a valid token to perform the requests.
Remember that in order to use the remote services, the authenticated user must have assigned:
-
The role rest-all for using the Business Central remote services
-
The role kie-server for using the Execution Server remote services
Please ensure necessary roles are created and assigned to the users that will consume the remote services on the Keycloak admin console.
You have two options to consume the different remove service endpoints:
-
Using basic authentication, if the application’s client supports it
-
Using Bearer (token) based authentication
20.3.8.1. Using basic authentication
If the KC client adapter configuration has the Basic authentication enabled, as proposed in this guide for both Business Central (step 3.2) and Execution Server, you can avoid the token grant/refresh calls and just call the services as the following examples.
Example for a Business Central remote repositories endpoint:
curl http://admin:password@localhost:8080/business-central-x.y.z.Final/rest/repositories
Example to check the status for the Execution Server:
curl http://admin:password@localhost:8280/kie-server-x.y.z.Final/services/rest/server/
20.3.8.2. Using token based authentication
First step is to create a new client on Keycloak that allows the third party remote service clients to obtain a token. It can be done as:
-
Go to the KC admin console and create a new client using this configuration:
-
Client id: kie-remote
-
Client protocol: openid-connect
-
Access type: public
-
Valid redirect URIs: http://localhost/
-
-
As we are going to manually obtain a token and invoke the service let’s increase the lifespan of tokens slightly. In production access tokens should have a relatively low timeout, ideally less than 5 minutes:
-
Go to the KC admin console
-
Click on your Realm Settings
-
Click on Tokens tab
-
Change the value for Access Token Lifespan to 15 minutes. That should give us plenty of time to obtain a token and invoke the service before it expires.
-
Once a public client for our remote clients has been created, you can now obtain the token by performing an HTTP request to the KC server’s tokens endpoint. Here is an example for command line:
RESULT=`curl --data "grant_type=password&client_id=kie-remote&username=admin&passwordpassword=<the_client_secret>" http://localhost:8180/auth/realms/demo/protocol/openid-connect/token`
TOKEN=`echo $RESULT | sed 's/.*access_token":"//g' | sed 's/".*//g'`
At this point, if you echo the $TOKEN it will output the token string obtained from the KC server, that can be now used to authorize further calls to the remote endpoints. For exmple, if you want to check the internal jBPM repositories:
curl -H "Authorization: bearer $TOKEN" http://localhost:8080/business-central-x.y.z.Final/rest/repositories
20.3.9. Keycloak and the Business Central's security administration area
Business Central provides an administration area which provides user, group and role management features (see Security management).
By default the application’s security management system points to the application’s server realm. For instance, in case of using the packaged distribution for Wildfly, it points to the Wildfly’s ApplicationRealm (properties based). It means the entities from the realm presented in the administration area are not the ones from the Keycloak realm that the application is using. There exist the following options in order to change this default behavior:
-
Disable the user system administration
-
Use the built-in Keycloak security management provider instead of the default one
In order to customize an existing jBPM application (WAR file) for using the Keycloak security management provider please follow the next steps:
-
Add the artifact keycloak-core-x.y.z.Final.jar into WEB-INF/lib
-
Add the artifact keycloak-common-x.y.z.Final.jar into WEB-INF/lib
-
Remove the actual jar artifact for any security management provider in use from WEB-INF/lib (eg: remove WEB-INF/lib/uberfire-security-management-wdilfly-x.y.Z.jar)
-
Replace the content for WEB-INF/classes/security-management.properties by:
org.uberfire.ext.security.management.api.userManagementServices=KCAdapterUserManagementService org.uberfire.ext.security.management.keycloak.authServer=<authz_server_url> # eg: org.uberfire.ext.security.management.keycloak.authServer=http://localhost:8180/auth
-
Update the /META-INF/jboss-deployment-structure.xml in order to include/exclude the following modules:
<deployment> <dependencies> ... <module name="org.jboss.resteasy.resteasy-jackson-provider" services="import"/> ... </dependencies> <exclusions> ... <module name="org.jboss.resteasy.resteasy-jackson2-provider"/> ... </exclusions> </deployment>
The jar artifacts required in the steps above can be either downloaded from JBoss Nexus or either build from sources. |
Once applying the above changes, the security administration area uses the access token present in the user’s session in order to authorize and manage the specific Keycloak realm data.
In order to be able to manage Keycloak realms remotely, please ensure the user has the realm-management client role assigned |
21. Business Central High Availability
21.1. VFS clustering
The VFS repositories (usually git repositories) stores all the assets (such as rules, decision tables, process definitions, forms, etc). If that VFS is located on each local server, then it must be kept in sync between all servers of a cluster.
Use Apache Zookeeper and Apache Helix to accomplish this. Zookeeper glues all the parts together. Helix is the cluster management component that registers all cluster details (nodes, resources and the cluster itself). Uberfire (on top of which Business Central is built) uses those 2 components to provide VFS clustering.
To create a VFS cluster:
-
Download Apache Zookeeper and Apache Helix.
-
Install both:
-
Unzip Zookeeper into a directory (
$ZOOKEEPER_HOME
). -
In
$ZOOKEEPER_HOME
, copyzoo_sample.conf
tozoo.conf
-
Edit
zoo.conf
. Adjust the settings if needed. Usually only these 2 properties are relevant:# the directory where the snapshot is stored. dataDir=/tmp/zookeeper # the port at which the clients will connect clientPort=2181
-
Unzip Helix into a directory (
$HELIX_HOME
).
-
-
Configure the cluster in Zookeeper:
-
Go to its
bin
directory:$ cd $ZOOKEEPER_HOME/bin
-
Start the Zookeeper server:
$ sudo ./zkServer.sh start
If the server fails to start, verify that the
dataDir
(as specified inzoo.conf
) is accessible. -
To review Zookeeper’s activities, open
zookeeper.out
:$ cat $ZOOKEEPER_HOME/bin/zookeeper.out
-
-
Configure the cluster in Helix:
-
Go to its
bin
directory:$ cd $HELIX_HOME/bin
-
Create the cluster:
$ ./helix-admin.sh --zkSvr localhost:2181 --addCluster kie-cluster
The
zkSvr
value must match the used Zookeeper server. The cluster name (kie-cluster
) can be changed as needed. -
Add nodes to the cluster:
# Node 1 $ ./helix-admin.sh --zkSvr localhost:2181 --addNode kie-cluster nodeOne:12345 # Node 2 $ ./helix-admin.sh --zkSvr localhost:2181 --addNode kie-cluster nodeTwo:12346 ...
Usually the number of nodes a in cluster equal the number of application servers in the cluster. The node names (
nodeOne:12345
, …) can be changed as needed.nodeOne:12345
is the unique identifier of the node, which will be referenced later on when configuring application servers. It is not a host and port number, but instead it is used to uniquely identify the logical node. -
Add resources to the cluster:
$ ./helix-admin.sh --zkSvr localhost:2181 --addResource kie-cluster vfs-repo 1 LeaderStandby AUTO_REBALANCE
The resource name (
vfs-repo
) can be changed as needed. -
Rebalance the cluster to initialize it:
$ ./helix-admin.sh --zkSvr localhost:2181 --rebalance kie-cluster vfs-repo 2
-
Start the Helix controller to manage the cluster:
$ ./run-helix-controller.sh --zkSvr localhost:2181 --cluster kie-cluster 2>&1 > /tmp/controller.log &
-
-
Configure the security domain correctly on the application server. For example on WildFly and JBoss EAP:
-
Edit the file
$JBOSS_HOME/domain/configuration/domain.xml
.For simplicity sake, presume we use the default domain configuration which uses the profile
full
that defines two server nodes as part ofmain-server-group
. -
Locate the profile
full
and add a new security domain by copying the other security domain already defined there by default:<security-domain name="kie-ide" cache-type="default"> <authentication> <login-module code="Remoting" flag="optional"> <module-option name="password-stacking" value="useFirstPass"/> </login-module> <login-module code="RealmDirect" flag="required"> <module-option name="password-stacking" value="useFirstPass"/> </login-module> </authentication> </security-domain>
The security-domain name is a magic value.
-
-
Configure the system properties for the cluster on the application server. For example on WildFly and JBoss EAP:
-
Edit the file
$JBOSS_HOME/domain/configuration/host.xml
. -
Locate the XML elements
server
that belong to themain-server-group
and add the necessary system property.For example for nodeOne:
<system-properties> <property name="jboss.node.name" value="nodeOne" boot-time="false"/> <property name="org.uberfire.nio.git.dir" value="/tmp/kie/nodeone" boot-time="false"/> <property name="org.uberfire.metadata.index.dir" value="/tmp/kie/nodeone" boot-time="false"/> <property name="org.uberfire.cluster.id" value="kie-cluster" boot-time="false"/> <property name="org.uberfire.cluster.zk" value="localhost:2181" boot-time="false"/> <property name="org.uberfire.cluster.local.id" value="nodeOne_12345" boot-time="false"/> <property name="org.uberfire.cluster.vfs.lock" value="vfs-repo" boot-time="false"/> <!-- If you're running both nodes on the same machine: --> <property name="org.uberfire.nio.git.daemon.port" value="9418" boot-time="false"/> </system-properties>
And for nodeTwo:
<system-properties> <property name="jboss.node.name" value="nodeTwo" boot-time="false"/> <property name="org.uberfire.nio.git.dir" value="/tmp/kie/nodetwo" boot-time="false"/> <property name="org.uberfire.metadata.index.dir" value="/tmp/kie/nodetwo" boot-time="false"/> <property name="org.uberfire.cluster.id" value="kie-cluster" boot-time="false"/> <property name="org.uberfire.cluster.zk" value="localhost:2181" boot-time="false"/> <property name="org.uberfire.cluster.local.id" value="nodeTwo_12346" boot-time="false"/> <property name="org.uberfire.cluster.vfs.lock" value="vfs-repo" boot-time="false"/> <!-- If you're running both nodes on the same machine: --> <property name="org.uberfire.nio.git.daemon.port" value="9419" boot-time="false"/> </system-properties>
Make sure the cluster, node and resource names match those configured in Helix.
-
21.2. jBPM clustering
In addition to the information above, jBPM clustering requires additional configuration. See this blog post to configure the database etc correctly.
KIE Server
The KIE Server is a standalone execution server for rules.
22. KIE Execution Server
22.1. Overview
KIE Server is a modular, standalone server component that can be used to instantiate and execute rules and processes. It exposes this functionality via REST, JMS and Java interfaces to client application. It also provides seamless integration with the Business Central.
At its core, KIE Server is a configurable web application packaged as a WAR file. Distributions are availables for pure web containers (like Tomcat) and for JEE 6 and JEE 7 containers.
Most capabilities on the Kie Server are configurable, and based on the concepts of extensions. Each extension can be enabled/disabled independently, allowing the user to configure the server to its need.
The current version of the Kie Server ships with two default extensions:
-
BRM: provides support for the execution of Business Rules using the Drools engine.
-
BPM: provides support for the execution of Business Processes using the jBPM engine. It supports:
-
process execution
-
task execution
-
asynchronous job execution
-
Both extensions enabled by default, but can be disabled by setting the corresponding property (see configuration chapter for details).
This server was designed to have a low footprint, with minimal memory consumption, and therefore, to be easily deployable on a cloud environment. Each instance of this server can open and instantiate multiple Kie Containers which allows you to execute multiple services in parallel.
22.1.1. Glossary
-
Kie Server: execution server purely focusing on providing runtime environment for both rules and processes. These capabilities are provided by Kie Server Extensions. More capabilities can be added by further extensions (e.g. customer could add his own extensions in case of missing functionality that will then use infrastructure of the KIE Server). A Kie Server instance is a standalone Kie Server executing on a given application server/web container. A Kie Server instantiates and provides support for multiple Kie Containers.
-
Kie Server Extension: a "plugin" for the Kie Server that adds capabilities to the server. The Kie Server ships with two default kie server extensions: BRM and BPM.
-
Kie Container: an in-memory instantiation of a kjar, allowing for the instantiation and usage of its assets (domain models, processes, rules, etc). A Kie Server exposes Kie Containers through a standard API over transport protocols like REST and JMS.
-
Controller: a server-backed REST endpoint that will be responsible for managing KIE Server instances. Such end point must provide following capabilities:
-
respond to connect requests
-
sync all registered containers on the corresponding Kie Server ID
-
respond to disconnect requests
-
-
Kie Server state: currently known state of given Kie Server instance. This is a local storage (by default in file) that maintains the following information:
-
list of registered Drools controllers
-
list of known containers
-
kie server configuration
The server state is persisted upon receival of events like: Kie Container created, Kie Container is disposed, Drools controller accepts registration of Kie Server instance, etc.
-
-
Kie Server ID: an arbitrary assigned identifier to which configurations are assigned. At boot, each Kie Server Instance is assigned an ID, and that ID is matched to a configuration on the Drools controller. The Kie Server Instance fetches and uses that configuration to setup itself.
22.2. Installing the KIE Server
The KIE Server is distributed as a web application archive (WAR) file. The WAR file comes in three different packagings:
-
webc - WAR for ordinary Web (Servlet) containers like Tomcat
-
ee6 - WAR for JavaEE 6 containers like JBoss EAP 6.x
-
ee7 - WAR for JavaEE 7 containers like WildFly 11.x
To install the KIE Execution Server and verify it is running, complete the following steps:
-
Deploy the WAR file into your web container.
-
Create a user with the role of
kie-server
on the container. -
Test that you can access the KIE Server by navigating to the endpoint in a browser window:
http://SERVER:PORT/CONTEXT/services/rest/server/
. -
When prompted for username/password, type in the username and password that you created in step 2.
-
Once authenticated, you will see an XML response in the form of KIE Server status, similar to this:
Example 204. Sample handshaking server response<response type="SUCCESS" msg="KIE Server info"> <kie-server-info> <version>7.23.0.Final</version> </kie-server-info> </response>
22.2.1. Bootstrap switches
The Kie Server accepts a number of bootstrap switches (system properties) to configure the behaviour of the server. The following is a table of all the supported switches.
Property | Value | Description | Required |
---|---|---|---|
org.drools.server.ext.disabled |
boolean (default is "false") |
If true, disables the BRM support (i.e. rules support). |
No |
org.jbpm.server.ext.disabled |
boolean (default is "false") |
If true, disables the BPM support (i.e. processes support) |
No |
org.jbpm.ui.server.ext.disabled |
boolean (default is "false") |
If true, disables the BPM UI support (i.e. processes image support) |
No |
org.optaplanner.server.ext.disabled |
boolean (default is "false") |
If true, disables the BRP support (i.e. planner support) |
No |
org.kie.executor.disabled |
boolean (default is "false") |
If true, disables the BPM job executor support |
No |
org.kie.server.id |
string |
An arbitrary ID to be assigned to this server. If a headless Drools controller is configured, this is the ID under which the server will connect to the headless Drools controller to fetch the kie container configurations. |
No. If not provided, an ID is automatically generated. |
org.kie.server.user |
string (default is "kieserver") |
User name used to connect with the kieserver from the Drools controller, required when running in managed mode |
No |
org.kie.server.pwd |
string (default is "kieserver1!") |
Password used to connect with the kieserver from the Drools controller, required when running in managed mode |
No |
org.kie.server.controller |
comma separated list of urls |
List of urls to Drools controller REST endpoint. E.g.:
|
Yes when using a Drools controller |
org.kie.server.controller.user |
string (default is "kieserver") |
Username used to connect to the Drools controller REST api |
Yes when using a Drools controller |
org.kie.server.controller.pwd |
string (default is "kieserver1!") |
Password used to connect to the Drools controller REST api |
Yes when using a Drools controller |
org.kie.server.location |
URL location of kie server instance |
The URL used by the Drools controller to call back on this server. E.g.:
|
Yes when using a Drools controller |
org.kie.server.domain |
string |
JAAS LoginContext domain that shall be used to authenticate users when using JMS |
No |
org.kie.server.bypass.auth.user |
boolean (default is "false") |
Allows to bypass the authenticated user for task related operations e.g. queries |
No |
org.kie.server.repo |
valid file system path (default is ".") |
Location on local file system where kie server state files will be stored |
No |
org.kie.server.persistence.ds |
string |
Datasource JNDI name |
Yes when BPM support enabled |
org.kie.server.persistence.tm |
string |
Transaction manager platform for Hibernate properties set |
Yes when BPM support enabled |
org.kie.server.persistence.dialect |
string |
Hibernate dialect to be used |
Yes when BPM support enabled |
org.jbpm.ht.callback |
string |
One of supported callbacks for Task Service (default jaas) |
No |
org.jbpm.ht.custom.callback |
string |
Custom implementation of UserGroupCallback in case org.jbpm.ht.callback was set to ‘custom’ |
No |
kie.maven.settings.custom |
valid file system path |
Location of custom settings.xml for maven configuration |
No |
org.kie.executor.interval |
integer (default is 0) |
Number of time units between polls by executor |
No |
org.kie.executor.pool.size |
integer (default is 1) |
Number of threads in the pool for async work |
No |
org.kie.executor.retry.count |
integer (default is 3) |
Number of retries to handle errors |
No |
org.kie.executor.timeunit |
TimeUnit (default is "SECONDS") |
TimeUnit representing interval |
No |
org.kie.executor.disabled |
boolean (default is "false") |
Disables executor completely |
No |
kie.server.jms.queues.response |
string (default is "queue/KIE.SERVER.RESPONSE") |
JNDI name of response queue for JMS |
No |
org.kie.server.controller.connect |
long (default is 10000) |
Waiting time in milliseconds between repeated attempts to connect kie server to Drools controller when kie server starts up |
No |
org.drools.server.filter.classes |
boolean (default is "false") |
If true, accept only classes which are annotated with @org.kie.api.remote.Remotable or @javax.xml.bind.annotation.XmlRootElement as extra JAXB classes |
No |
If you are running both KIE Server and Business Central you must configure KIE Server to use a different Data Source to Business Central using the org.kie.server.persistence.ds property. Business Central uses a jBPM Executor Service that can conflict with KIE Server if they share the same Data Source. |
22.2.2. Installation details for different containers
22.2.2.1. Tomcat 7.x/8.x
-
Download and unzip the Tomcat distribution. Let’s call the root of the distribution
TOMCAT_HOME
. This directory is named after the Tomcat version, so for exampleapache-tomcat-7.0.55
. -
Download kie-server- -webc.war and place it into
TOMCAT_HOME/webapps
. -
Configure user(s) and role(s). Make sure that file
TOMCAT_HOME/conf/tomcat-users.xml
contains the following username and role definition. You can of course choose different username and password, just make sure that the user has rolekie-server
:Example 205. Username and role definition for Tomcat<role rolename="kie-server"/> <user username="serveruser" password="my.s3cr3t.pass" roles="kie-server"/>
-
Start the server by running
TOMCAT_HOME/bin/startup.[sh|bat]
. You can check out the Tomcat logs inTOMCAT_HOME/logs
to see if the application deployed successfully. Please read the table above for the bootstrap switches that can be used to properly configure the instance. For instance:./startup.sh -Dorg.kie.server.id=first-kie-server -Dorg.kie.server.location=http://localhost:8080/kie-server/services/rest/server
-
Verify the server is running. Go to
http://SERVER:PORT/CONTEXT/services/rest/server/
and type the specified username and password. You should see simple XML message with basic information about the server.
You can not leverage the JMS interface when running with Tomcat, or any other Web container. The Web container version of the WAR contains only the REST interface. |
22.2.2.2. WildFly 11.x
-
Download and unzip the WildFly distribution. Let’s call the root of the distribution
WILDFLY_HOME
. This directory is named after the WildFly version, so for examplewildfly-14.0.1.Final
. -
Download kie-server- -ee7.war and place it into
WILDFLY_HOME/standalone/deployments
. -
Configure user(s) and role(s). Execute the following command
WILDFLY_HOME/bin/add-user.[sh|bat] -a -u 'kieserver' -p 'kieserver1!' -ro 'kie-server'
. You can of course choose different username and password, just make sure that the user has rolekie-server
. -
Start the server by running
WILDFLY_HOME/bin/standalone.[sh|bat] -c standalone-full.xml <bootstrap_switches>
. You can check out the standard output or WildFly logs inWILDFLY_HOME/standalone/logs
to see if the application deployed successfully. Please read the table above for the bootstrap switches that can be used to properly configure the instance. For instance:./standalone.sh --server-config=standalone-full.xml -Djboss.socket.binding.port-offset=150 -Dorg.kie.server.id=first-kie-server -Dorg.kie.server.location=http://localhost:8230/kie-server/services/rest/server
-
Verify the server is running. Go to
http://SERVER:PORT/CONTEXT/services/rest/server/
and type the specified username and password. You should see simple XML message with basic information about the server.
22.3. Kie Server setup
Server setup and registration changed significantly from versions 6.2 and before. The following applies only to version 6.3 and forward. |
22.3.1. Managed Kie Server
A managed instance is one that requires a Drools controller to be available to properly start up the Kie Server instance.
The Drools controller is a component responsible for keeping and managing a Kie Server Configuration in centralized way. Each Drools controller can manager multiple configurations at once and there can be multiple Drools controllers in the environment. Managed KIE Servers can be configured with a list of Drools controllers but will connect to only one at a time.
It’s important to mention that even though there can be multiple Drools controllers they should be kept in sync to make sure that regardless which one of them is contacted by KIE Server instance it will provide same set of configuration. |
At startup, if a Kie Server is configured with a list of Drools controllers, it will try succesivelly to connect to each of them until a connection is successfully stablished with one of them. If for any reason a connection can’t be stablished, the server will not start, even if there is local storage available with configuration. This happens by design in order to ensure consistency. For instance, if the Kie Server was down and the configuration has changed, this restriction guarantees that it will run with up to date configuration or not at all.
In order to run the Kie Server in standalone mode, without connecting to any Drools controllers, please see "Unmanaged Kie Server". |
The configuration sets, among other things:
-
kie containers to be deployed and started
-
configuration items - currently this is a place holder for further enhancements that will allow remotely configure KIE Execution Server components - timers, persistence, etc
The Drools controller, besides providing configuration management, is also responsible for overall management of Kie Servers. It provides a REST api that is divided into two parts:
-
the Drools controller itself that is exposed to interact with KIE Execution Server instances
-
an administration API that allows to remotely manage Kie Server instances:
-
add/remove servers
-
add/remove containers to/from the servers
-
start/stop containers on servers
-
The Drools controller deals only with the Kie Server configuration or definition to put it differently. It does not handle any runtime components of KIE Execution Server instances. They are always considered remote to Drools controller. The Drools controller is responsible for persisting the configuration to preserve restarts of the Drools controller itself. It should manage the synchronization as well in case multiple Drools controllers are configured to keep all definitions up to date on all instances of the Drools controller.
By default Drools controller is shipped with Business Central and provides a fully featured management interface (both REST api and UI). It uses underlying git repository as persistent store and thus when GIT repositories are clustered (using Apache Zookeeper and Apache Helix) it will cover the Drools controllers synchronization as well.
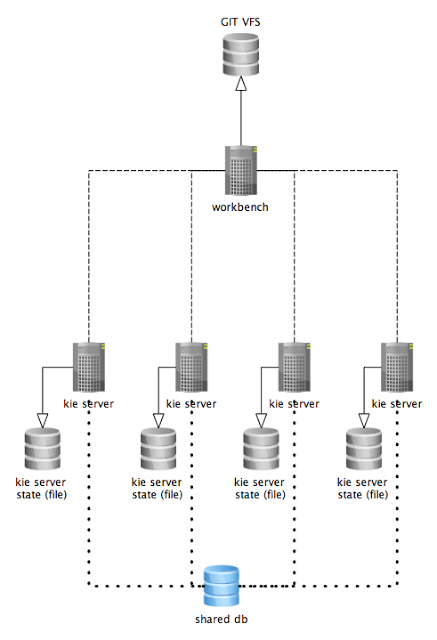
The diagram above illustrates the single Drools controller (Business Central) setup with multiple Kie Server instances managed by it.
The diagram bellow illustrates the clustered setup where there are multiple instances of Drools controller synchronized over Zookeeper.
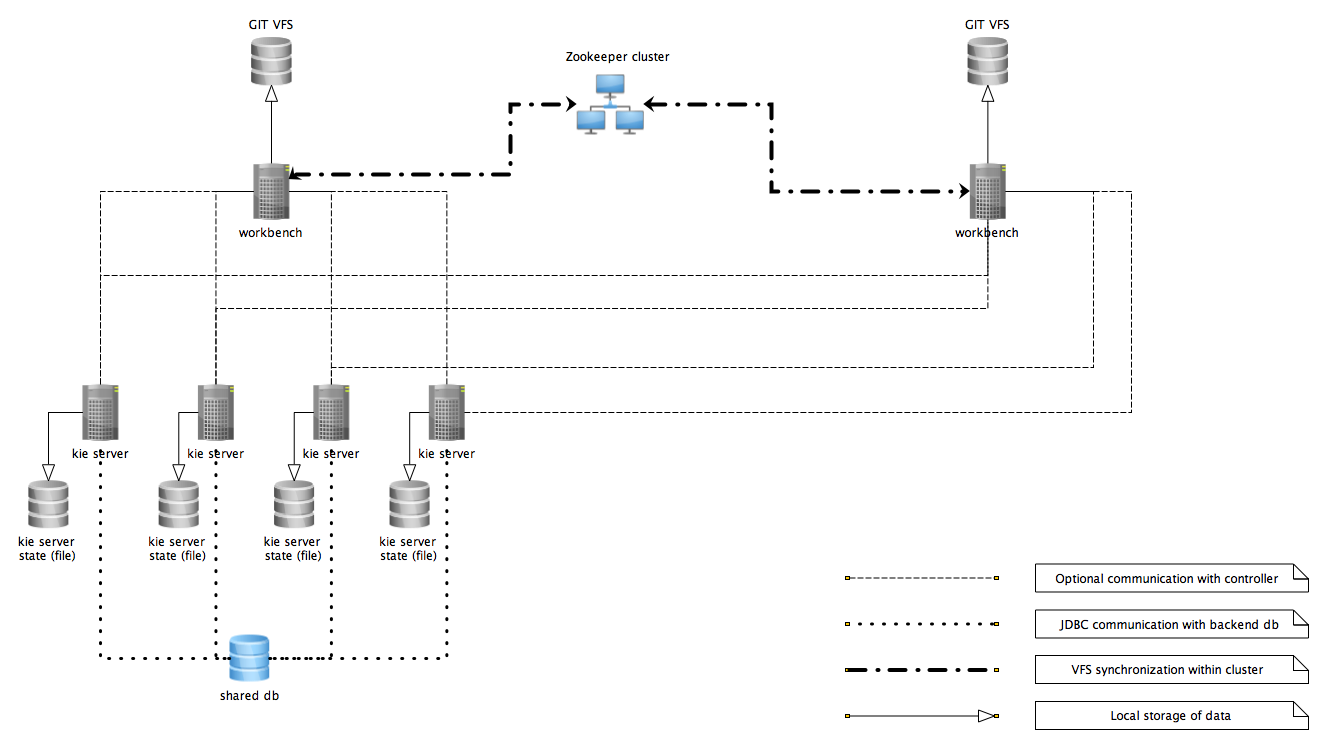
In the above diagram we can see that the Kie Server instances are capable of connecting to any Drools controllers, but they will connect to only one. Each instance will attempt to connect to Drools controller as long as it can reach one. Once connection is established with one of the Drools controllers it will skip the others.
22.3.1.1. Working with managed servers
There are two approaches that users can take when working with managed KIE Server instances:
-
Configuration first: with this approach, a user will start working with the Drools controller (either UI or REST api) and create and configure Kie Server definitions. That consists basically of an identification for the server definition (id and name + optionally version for improved readability) and the configuration for the Kie Containers to run on the server.
-
Registration first: with this approach, the Kie Server instances are started first and auto register themselves on Drools controller. The user then can configure the Kie Containers. This option simply skips the registration step done in the first approach and populates it with server id, name and version directly upon auto registration. There are no other differences between the two approaches.
22.3.2. Unmanaged KIE Execution Server
An unmanaged Kie Server is in turn just a standalone instance, and thus must be configured individually using REST/JMS api from the Kie Server itself. There is no Drools controller involved. The configuration is automatically persisted by the server into a file and that is used as the internal server state, in case of restarts.
The configuration is updated during the following operations:
-
deploy Kie Container
-
undeploy Kie Container
-
start Kie Container
-
stop Kie Container
If the Kie Server is restarted, it will try to restablish the same state that was persisted before shutdown. That means that Kie Containers that were running, will be started, but the ones that were stopped/disposed before, will not. |
In most use cases, the Kie Server should be executed in managed mode as that provides some benefits, like a web user interface (if using Business Central as a Drools controller) and some facilities for clustering.
22.4. Creating a Kie Container
Once your Execution Server is registered, you can start adding Kie Containers to it.
Kie Containers are self contained environments that have been provisioned to hold instances of your packaged and deployed rule instances.
-
Start by clicking the \+ icon next to the Execution Server where you want to deploy your Container. This will bring up the New Container screen.
-
If you know the Group Name, Artifact Id and Version (GAV) of your deployed package, then you can enter those details and click the Ok button to select that instance (and provide a name for the Container);
-
If you don’t know these values, you can search Business Central for all packages that can be deployed. Click the Search button without entering any value in the search field (you can narrow your search by entering any term that you know exists in the package that you want to deploy).
INSERT SCREENSHOT HERE
The figure above shows that there are three deployable packages available to be used as containers on the Execution Server. Select the one that you want by clicking the Select button. This will auto-populate the GAV and you can then click the Ok button to use this deployable as the new Container.
-
Enter a name for this Container at the top and then press the Ok button.
The Container name must be unique inside each execution server and must not contain any spaces.
Just below the GAV row, you will see an uneditable row that shows you the URL for your Container against which you will be able to execute REST commands. |
22.5. Managing Containers
Containers within the Execution Server can be started, stopped and updated from within Business Central.
22.5.1. Starting a Container
Once registered, a Container is in the 'Stopped' mode. It can be started by first selecting it and then clicking the Start button. You can also select multiple Containers and start them all at the same time.
Once the Container is in the 'Running' mode, a green arrow appears next to it. If there are any errors starting the Container(s), red icons appear next to Containers and the Execution Server that they are deployed on.
You should check the logs of both the Execution Server and the current Business Central to see what the errors are before redeploying the Containers (and possibly the Execution Server).
22.5.2. Stopping and Deleting a Container
Similar to starting a Container, select the Container(s) that you want to stop (or delete) and click the Stop button (which replaces the Start button for that Container once it has entered the 'Running' mode) or the Delete button.
22.5.3. Updating a Container
You can update deployed KieContainers
without restarting the Execution Server.
This is useful in cases where the Business Rules change, creating new versions of packages to be provisioned.
You can have multiple versions of the same package provisioned and deployed, each to a different KieContainer
.
To update deployments in a KieContainer
dynamically, click on the icon next to the Container.
This will open up the Container Info screen.
An example of this screen is shown here:
INSERT SCREENSHOT HERE |
The Container Info screen is a useful tool because it not only allows you to see the endpoint for this KieContainer
, but it also allows you to either manually or automatically refresh the provision if an update is available.
The update can be manual or automatic:
Manual Update: To manually update a KieContainer
, enter the new Version number in the Version box and click on the Update button.
You can of course, update the Group Id or the Artifact Id , if these have changed as well.
Once updated, the Execution server updates the container and shows you the resolved GAV attributes at the bottom of the screen in the Resolved Release Id section.
Automatic Update: If you want a deployed Container to always have the latest version of your deployment without manually editing it, you will need to set the Version property to the value of LATEST
and start a Scanner
.
This will ensure that the deployed provision always contains the latest version.
The Scanner can be started just once on demand by clicking the Scan Now button or you can start it in the background with scans happening at a specified interval (in milliseconds).You can also set this value to LATEST
when you are first creating this deployment.
The Resolved Release
Id in this case will show you the actual, latest version number.
22.6. KIE Server REST API for KIE containers and business assets
Drools provides a KIE Server REST API that you can use to interact with your KIE containers and business assets (such as business rules, processes, and solvers) in Drools without using the Business Central user interface. This API support enables you to maintain your Drools resources more efficiently and optimize your integration and development with Drools.
With the KIE Server REST API, you can perform the following actions:
-
Deploy or dispose KIE containers
-
Retrieve and update KIE container information
-
Return KIE Server status and basic information
-
Retrieve and update business asset information
-
Execute business assets (such as rules and processes)
KIE Server REST API requests require the following components:
- Authentication
-
The KIE Server REST API requires HTTP Basic authentication or token-based authentication for the user role
kie-server
. To view configured user roles for your Drools distribution, navigate to~/$SERVER_HOME/standalone/configuration/application-roles.properties
and~/application-users.properties
.To add a user with the
kie-server
role, navigate to~/$SERVER_HOME/bin
and run the following command:$ ./add-user.sh -a --user <USERNAME> --password <PASSWORD> --role kie-server
For more information about user roles and Drools installation options, see Installing the KIE Server.
- HTTP headers
-
The KIE Server REST API requires the following HTTP headers for API requests:
-
Accept
: Data format accepted by your requesting client:-
application/json
(JSON) -
application/xml
(XML, for JAXB or XSTREAM)
-
-
Content-Type
: Data format of yourPOST
orPUT
API request data:-
application/json
(JSON) -
application/xml
(XML, for JAXB or XSTREAM)
-
-
X-KIE-ContentType
: Required header forapplication/xml
XSTREAM API requests and responses:-
XSTREAM
-
-
- HTTP methods
-
The KIE Server REST API supports the following HTTP methods for API requests:
-
GET
: Retrieves specified information from a specified resource endpoint -
POST
: Updates a resource or resource instance -
PUT
: Updates or creates a resource or resource instance -
DELETE
: Deletes a resource or resource instance
-
- Base URL
-
The base URL for KIE Server REST API requests is
http://SERVER:PORT/kie-server/services/rest/
, such ashttp://localhost:8080/kie-server/services/rest/
. - Endpoints
-
KIE Server REST API endpoints, such as
/server/containers/{containerId}
for a specified KIE container, are the URIs that you append to the KIE Server REST API base URL to access the corresponding resource or type of resource in Drools.Example request URL for/server/containers/{containerId}
endpointhttp://localhost:8080/kie-server/services/rest/server/containers/MyContainer
- Request parameters and request data
-
Many KIE Server REST API requests require specific parameters in the request URL path to identify or filter specific resources and to perform specific actions. You can append URL parameters to the endpoint in the format
?<PARAM>=<VALUE>&<PARAM>=<VALUE>
.Example GET request URL with parametershttp://localhost:8080/kie-server/services/rest/server/containers?groupId=com.redhat&artifactId=Project1&version=1.0&status=STARTED
HTTP
POST
andPUT
requests may additionally require a request body or file with data to accompany the request.Example POST request URL and JSON request body datahttp://localhost:8080/kie-server/services/rest/server/containers/MyContainer/release-id
{ "release-id": { "artifact-id": "Project1", "group-id": "com.redhat", "version": "1.1" } }
22.6.1. Sending requests with the KIE Server REST API using a REST client or curl utility
The KIE Server REST API enables you to interact with your KIE containers and business assets (such as business rules, processes, and solvers) in Drools without using the Business Central user interface. You can send KIE Server REST API requests using any REST client or curl utility.
-
KIE Server is installed and running.
-
You have
kie-server
user role access to KIE Server.
-
Identify the relevant API endpoint to which you want to send a request, such as
[GET] /server/containers
to retrieve KIE containers from KIE Server. -
In a REST client or curl utility, enter the following components for a
GET
request to/server/containers
. Adjust any request details according to your use case.For REST client:
-
Authentication: Enter the user name and password of the KIE Server user with the
kie-server
role. -
HTTP Headers: Set the following header:
-
Accept
:application/json
-
-
HTTP method: Set to
GET
. -
URL: Enter the KIE Server REST API base URL and endpoint, such as
http://localhost:8080/kie-server/services/rest/server/containers
.
For curl utility:
-
-u
: Enter the user name and password of the KIE Server user with thekie-server
role. -
-H
: Set the following header:-
accept
:application/json
-
-
-X
: Set toGET
. -
URL: Enter the KIE Server REST API base URL and endpoint, such as
http://localhost:8080/kie-server/services/rest/server/containers
.
curl -u 'baAdmin:password@1' -H "accept: application/json" -X GET "http://localhost:8080/kie-server/services/rest/server/containers"
-
-
Execute the request and review the KIE Server response.
Example server response (JSON):
{ "type": "SUCCESS", "msg": "List of created containers", "result": { "kie-containers": { "kie-container": [ { "container-id": "itorders_1.0.0-SNAPSHOT", "release-id": { "group-id": "itorders", "artifact-id": "itorders", "version": "1.0.0-SNAPSHOT" }, "resolved-release-id": { "group-id": "itorders", "artifact-id": "itorders", "version": "1.0.0-SNAPSHOT" }, "status": "STARTED", "scanner": { "status": "DISPOSED", "poll-interval": null }, "config-items": [], "container-alias": "itorders" } ] } } }
-
For this example, copy or note the project
group-id
,artifact-id
, andversion
(GAV) data from one of the deployed KIE containers returned in the response. -
In your REST client or curl utility, send another API request with the following components for a
PUT
request to/server/containers/{containerId}
to deploy a new KIE container with the copied project GAV data. Adjust any request details according to your use case.For REST client:
-
Authentication: Enter the user name and password of the KIE Server user with the
kie-server
role. -
HTTP Headers: Set the following headers:
-
Accept
:application/json
-
Content-Type
:application/json
-
-
HTTP method: Set to
PUT
. -
URL: Enter the KIE Server REST API base URL and endpoint, such as
http://localhost:8080/kie-server/services/rest/server/containers/MyContainer
. -
Request body: Add a JSON request body with the configuration items for the new KIE container:
{ "config-items": [ { "itemName": "RuntimeStrategy", "itemValue": "SINGLETON", "itemType": "java.lang.String" }, { "itemName": "MergeMode", "itemValue": "MERGE_COLLECTIONS", "itemType": "java.lang.String" }, { "itemName": "KBase", "itemValue": "", "itemType": "java.lang.String" }, { "itemName": "KSession", "itemValue": "", "itemType": "java.lang.String" } ], "release-id": { "group-id": "itorders", "artifact-id": "itorders", "version": "1.0.0-SNAPSHOT" }, "scanner": { "poll-interval": "5000", "status": "STARTED" } }
For curl utility:
-
-u
: Enter the user name and password of the KIE Server user with thekie-server
role. -
-H
: Set the following headers:-
accept
:application/json
-
content-type
:application/json
-
-
-X
: Set toPUT
. -
URL: Enter the KIE Server REST API base URL and endpoint, such as
http://localhost:8080/kie-server/services/rest/server/containers/MyContainer
. -
-d
: Add a JSON request body or file (@file.json
) with the configuration items for the new KIE container:
curl -u 'baAdmin:password@1' -H "accept: application/json" -H "content-type: application/json" -X PUT "http://localhost:8080/kie-server/services/rest/server/containers/MyContainer" -d "{ \"config-items\": [ { \"itemName\": \"RuntimeStrategy\", \"itemValue\": \"SINGLETON\", \"itemType\": \"java.lang.String\" }, { \"itemName\": \"MergeMode\", \"itemValue\": \"MERGE_COLLECTIONS\", \"itemType\": \"java.lang.String\" }, { \"itemName\": \"KBase\", \"itemValue\": \"\", \"itemType\": \"java.lang.String\" }, { \"itemName\": \"KSession\", \"itemValue\": \"\", \"itemType\": \"java.lang.String\" } ], \"release-id\": { \"group-id\": \"itorders\", \"artifact-id\": \"itorders\", \"version\": \"1.0.0-SNAPSHOT\" }, \"scanner\": { \"poll-interval\": \"5000\", \"status\": \"STARTED\" }}"
curl -u 'baAdmin:password@1' -H "accept: application/json" -H "content-type: application/json" -X PUT "http://localhost:8080/kie-server/services/rest/server/containers/MyContainer" -d @my-container-configs.json
-
-
Execute the request and review the KIE Server response.
Example server response (JSON):
{ "type": "SUCCESS", "msg": "Container MyContainer successfully deployed with module itorders:itorders:1.0.0-SNAPSHOT.", "result": { "kie-container": { "container-id": "MyContainer", "release-id": { "group-id": "itorders", "artifact-id": "itorders", "version": "1.0.0-SNAPSHOT" }, "resolved-release-id": { "group-id": "itorders", "artifact-id": "itorders", "version": "1.0.0-SNAPSHOT" }, "status": "STARTED", "scanner": { "status": "STARTED", "poll-interval": 5000 }, "config-items": [], "messages": [ { "severity": "INFO", "timestamp": { "java.util.Date": 1540584717937 }, "content": [ "Container MyContainer successfully created with module itorders:itorders:1.0.0-SNAPSHOT." ] } ], "container-alias": null } } }
If you encounter request errors, review the returned error code messages and adjust your request accordingly.
22.6.2. Sending requests with the KIE Server REST API using the Swagger interface
The KIE Server REST API supports a Swagger web interface that you can use instead of a standalone REST client or curl utility to interact with your KIE containers and business assets (such as business rules, processes, and solvers) in Drools without using the Business Central user interface.
-
KIE Server is installed and running.
-
You have
kie-server
user role access to KIE Server.
-
In a web browser, navigate to
http://SERVER:PORT/kie-server/docs
, such ashttp://localhost:8080/kie-server/docs
, and log in with the user name and password of the KIE Server user with thekie-server
role. -
In the Swagger page, select the relevant API endpoint to which you want to send a request, such as KIE Server and KIE containers → [GET] /server/containers to retrieve KIE containers from KIE Server.
-
Click Try it out and provide any optional parameters by which you want to filter results, if needed.
-
In the Response content type drop-down menu, select the desired format of the server response, such as application/json for JSON format.
-
Click Execute and review the KIE Server response.
Example server response (JSON):
{ "type": "SUCCESS", "msg": "List of created containers", "result": { "kie-containers": { "kie-container": [ { "container-id": "itorders_1.0.0-SNAPSHOT", "release-id": { "group-id": "itorders", "artifact-id": "itorders", "version": "1.0.0-SNAPSHOT" }, "resolved-release-id": { "group-id": "itorders", "artifact-id": "itorders", "version": "1.0.0-SNAPSHOT" }, "status": "STARTED", "scanner": { "status": "DISPOSED", "poll-interval": null }, "config-items": [], "container-alias": "itorders" } ] } } }
-
For this example, copy or note the project
group-id
,artifact-id
, andversion
(GAV) data from one of the deployed KIE containers returned in the response. -
In the Swagger page, navigate to the KIE Server and KIE containers → [PUT] /server/containers/{containerId} endpoint to send another request to deploy a new KIE container with the copied project GAV data. Adjust any request details according to your use case.
-
Click Try it out and enter the following components for the request:
-
containerId: Enter the ID of the new KIE container, such as
MyContainer
. -
body: Set the Parameter content type to the desired request body format, such as application/json for JSON format, and add a request body with the configuration items for the new KIE container:
{ "config-items": [ { "itemName": "RuntimeStrategy", "itemValue": "SINGLETON", "itemType": "java.lang.String" }, { "itemName": "MergeMode", "itemValue": "MERGE_COLLECTIONS", "itemType": "java.lang.String" }, { "itemName": "KBase", "itemValue": "", "itemType": "java.lang.String" }, { "itemName": "KSession", "itemValue": "", "itemType": "java.lang.String" } ], "release-id": { "group-id": "itorders", "artifact-id": "itorders", "version": "1.0.0-SNAPSHOT" }, "scanner": { "poll-interval": "5000", "status": "STARTED" } }
-
-
In the Response content type drop-down menu, select the desired format of the server response, such as application/json for JSON format.
-
Click Execute and review the KIE Server response.
Example server response (JSON):
{ "type": "SUCCESS", "msg": "Container MyContainer successfully deployed with module itorders:itorders:1.0.0-SNAPSHOT.", "result": { "kie-container": { "container-id": "MyContainer", "release-id": { "group-id": "itorders", "artifact-id": "itorders", "version": "1.0.0-SNAPSHOT" }, "resolved-release-id": { "group-id": "itorders", "artifact-id": "itorders", "version": "1.0.0-SNAPSHOT" }, "status": "STARTED", "scanner": { "status": "STARTED", "poll-interval": 5000 }, "config-items": [], "messages": [ { "severity": "INFO", "timestamp": { "java.util.Date": 1540584717937 }, "content": [ "Container MyContainer successfully created with module itorders:itorders:1.0.0-SNAPSHOT." ] } ], "container-alias": null } } }
If you encounter request errors, review the returned error code messages and adjust your request accordingly.
22.6.3. Supported KIE Server REST API endpoints
The KIE Server REST API provides endpoints for the following types of resources in Drools:
-
KIE Server and KIE containers
-
KIE session assets (for runtime commands)
-
DMN assets
-
Planning solvers
The KIE Server REST API base URL is http://SERVER:PORT/kie-server/services/rest/
. All requests require HTTP Basic authentication or token-based authentication for the kie-server
user role.
For the full list of KIE Server REST API endpoints and descriptions, use one of the following resources:
-
Execution Server REST API on the jBPM Documentation page (static)
-
Swagger UI for the KIE Server REST API at
http://SERVER:PORT/kie-server/docs
(dynamic, requires running KIE Server)
22.7. KIE Server Java client API for KIE containers and business assets
Drools provides a KIE Server Java client API that enables you to connect to KIE Server using REST protocol from your Java client application. You can use the KIE Server Java client API as an alternative to the KIE Server REST API to interact with your KIE containers and business assets (such as business rules, processes, and solvers) in Drools without using the Business Central user interface. This API support enables you to maintain your Drools resources more efficiently and optimize your integration and development with Drools.
With the KIE Server Java client API, you can perform the following actions also supported by the KIE Server REST API:
-
Deploy or dispose KIE containers
-
Retrieve and update KIE container information
-
Return KIE Server status and basic information
-
Retrieve and update business asset information
-
Execute business assets (such as rules and processes)
KIE Server Java client API requests require the following components:
- Authentication
-
The KIE Server Java client API requires HTTP Basic authentication for the user role
kie-server
. To view configured user roles for your Drools distribution, navigate to~/$SERVER_HOME/standalone/configuration/application-roles.properties
and~/application-users.properties
.To add a user with the
kie-server
role, navigate to~/$SERVER_HOME/bin
and run the following command:$ ./add-user.sh -a --user <USERNAME> --password <PASSWORD> --role kie-server
For more information about user roles and Drools installation options, see Installing the KIE Server.
- Project dependencies
-
The KIE Server Java client API requires the following dependencies on the relevant classpath of your Java project:
<!-- For remote execution on KIE Server --> <dependency> <groupId>org.kie.server</groupId> <artifactId>kie-server-client</artifactId> <version>${drools.version}</version> </dependency> <!-- For runtime commands --> <dependency> <groupId>org.drools</groupId> <artifactId>drools-compiler</artifactId> <scope>runtime</scope> <version>${drools.version}</version> </dependency> <!-- For debug logging (optional) --> <dependency> <groupId>ch.qos.logback</groupId> <artifactId>logback-classic</artifactId> <version>${logback.version}</version> </dependency>
The
<version>
for Drools dependencies is the Maven artifact version for Drools currently used in your project (for example, 7.23.0.Final-redhat-00002). - Client request configuration
-
All Java client requests with the KIE Server Java client API must define at least the following server communication components:
-
Credentials of the
kie-server
user -
KIE Server location, such as
http://localhost:8080/kie-server/services/rest/server
-
Marshalling format for API requests and responses (JSON, JAXB, or XSTREAM)
-
A
KieServicesConfiguration
object and aKieServicesClient
object, which serve as the entry point for starting the server communication using the Java client API -
A
KieServicesFactory
object defining REST protocol and user access -
Any other client services used, such as
RuleServicesClient
,ProcessServicesClient
, orQueryServicesClient
The following are examples of basic and advanced client configurations with these components:
Basic client configuration exampleimport org.kie.server.api.marshalling.MarshallingFormat; import org.kie.server.client.KieServicesClient; import org.kie.server.client.KieServicesConfiguration; import org.kie.server.client.KieServicesFactory; public class MyConfigurationObject { private static final String URL = "http://localhost:8080/kie-server/services/rest/server"; private static final String USER = "baAdmin"; private static final String PASSWORD = "password@1"; private static final MarshallingFormat FORMAT = MarshallingFormat.JSON; private static KieServicesConfiguration conf; private static KieServicesClient kieServicesClient; public static void initialize() { conf = KieServicesFactory.newRestConfiguration(URL, USER, PASSWORD); //If you use custom classes, such as Obj.class, add them to the configuration. Set<Class<?>> extraClassList = new HashSet<Class<?>>(); extraClassList.add(Obj.class); conf.addExtraClasses(extraClassList); conf.setMarshallingFormat(FORMAT); kieServicesClient = KieServicesFactory.newKieServicesClient(conf); } }
Advanced client configuration example with additional client servicesimport org.kie.server.api.marshalling.MarshallingFormat; import org.kie.server.client.CaseServicesClient; import org.kie.server.client.DMNServicesClient; import org.kie.server.client.DocumentServicesClient; import org.kie.server.client.JobServicesClient; import org.kie.server.client.KieServicesClient; import org.kie.server.client.KieServicesConfiguration; import org.kie.server.client.KieServicesFactory; import org.kie.server.client.ProcessServicesClient; import org.kie.server.client.QueryServicesClient; import org.kie.server.client.RuleServicesClient; import org.kie.server.client.SolverServicesClient; import org.kie.server.client.UIServicesClient; import org.kie.server.client.UserTaskServicesClient; import org.kie.server.api.model.instance.ProcessInstance; import org.kie.server.api.model.KieContainerResource; import org.kie.server.api.model.ReleaseId; public class MyAdvancedConfigurationObject { // REST API base URL, credentials, and marshalling format private static final String URL = "http://localhost:8080/kie-server/services/rest/server"; private static final String USER = "baAdmin"; private static final String PASSWORD = "password@1";; private static final MarshallingFormat FORMAT = MarshallingFormat.JSON; private static KieServicesConfiguration conf; // KIE client for common operations private static KieServicesClient kieServicesClient; // Rules client private static RuleServicesClient ruleClient; // Process automation clients private static CaseServicesClient caseClient; private static DocumentServicesClient documentClient; private static JobServicesClient jobClient; private static ProcessServicesClient processClient; private static QueryServicesClient queryClient; private static UIServicesClient uiClient; private static UserTaskServicesClient userTaskClient; // DMN client private static DMNServicesClient dmnClient; // Planning client private static SolverServicesClient solverClient; public static void main(String[] args) { initializeKieServerClient(); initializeDroolsServiceClients(); initializeJbpmServiceClients(); initializeSolverServiceClients(); } public static void initializeKieServerClient() { conf = KieServicesFactory.newRestConfiguration(URL, USER, PASSWORD); conf.setMarshallingFormat(FORMAT); kieServicesClient = KieServicesFactory.newKieServicesClient(conf); } public static void initializeDroolsServiceClients() { ruleClient = kieServicesClient.getServicesClient(RuleServicesClient.class); dmnClient = kieServicesClient.getServicesClient(DMNServicesClient.class); } public static void initializeJbpmServiceClients() { caseClient = kieServicesClient.getServicesClient(CaseServicesClient.class); documentClient = kieServicesClient.getServicesClient(DocumentServicesClient.class); jobClient = kieServicesClient.getServicesClient(JobServicesClient.class); processClient = kieServicesClient.getServicesClient(ProcessServicesClient.class); queryClient = kieServicesClient.getServicesClient(QueryServicesClient.class); uiClient = kieServicesClient.getServicesClient(UIServicesClient.class); userTaskClient = kieServicesClient.getServicesClient(UserTaskServicesClient.class); } public static void initializeSolverServiceClients() { solverClient = kieServicesClient.getServicesClient(SolverServicesClient.class); } }
-
22.7.1. Sending requests with the KIE Server Java client API
The KIE Server Java client API enables you to connect to KIE Server using REST protocol from your Java client application. You can use the KIE Server Java client API as an alternative to the KIE Server REST API to interact with your KIE containers and business assets (such as business rules, processes, and solvers) in Drools without using the Business Central user interface.
-
KIE Server is installed and running.
-
You have
kie-server
user role access to KIE Server. -
You have a Java project with Drools resources.
-
In your client application, ensure that the following dependencies have been added to the relevant classpath of your Java project:
<!-- For remote execution on KIE Server --> <dependency> <groupId>org.kie.server</groupId> <artifactId>kie-server-client</artifactId> <version>${drools.version}</version> </dependency> <!-- For runtime commands --> <dependency> <groupId>org.drools</groupId> <artifactId>drools-compiler</artifactId> <scope>runtime</scope> <version>${drools.version}</version> </dependency> <!-- For debug logging (optional) --> <dependency> <groupId>ch.qos.logback</groupId> <artifactId>logback-classic</artifactId> <version>${logback.version}</version> </dependency>
-
In the
~/kie/server/client
folder of the Java client API in GitHub , identify the relevant Java client for the request you want to send, such asKieServicesClient
to access client services for KIE containers and other assets in KIE Server. -
In your client application, create a
.java
class for the API request. The class must contain the necessary imports, KIE Server location and user credentials, aKieServicesClient
object, and the client method to execute, such ascreateContainer
anddisposeContainer
from theKieServicesClient
client. Adjust any configuration details according to your use case.Creating and disposing a containerimport org.kie.server.api.marshalling.MarshallingFormat; import org.kie.server.client.KieServicesClient; import org.kie.server.client.KieServicesConfiguration; import org.kie.server.client.KieServicesFactory; import org.kie.server.api.model.KieContainerResource; import org.kie.server.api.model.ServiceResponse; public class MyConfigurationObject { private static final String URL = "http://localhost:8080/kie-server/services/rest/server"; private static final String USER = "baAdmin"; private static final String PASSWORD = "password@1"; private static final MarshallingFormat FORMAT = MarshallingFormat.JSON; private static KieServicesConfiguration conf; private static KieServicesClient kieServicesClient; public static void initialize() { conf = KieServicesFactory.newRestConfiguration(URL, USER, PASSWORD); public void disposeAndCreateContainer() { System.out.println("== Disposing and creating containers =="); // Retrieve list of KIE containers List<KieContainerResource> kieContainers = kieServicesClient.listContainers().getResult().getContainers(); if (kieContainers.size() == 0) { System.out.println("No containers available..."); return; } // Dispose KIE container KieContainerResource container = kieContainers.get(0); String containerId = container.getContainerId(); ServiceResponse<Void> responseDispose = kieServicesClient.disposeContainer(containerId); if (responseDispose.getType() == ResponseType.FAILURE) { System.out.println("Error disposing " + containerId + ". Message: "); System.out.println(responseDispose.getMsg()); return; } System.out.println("Success Disposing container " + containerId); System.out.println("Trying to recreate the container..."); // Re-create KIE container ServiceResponse<KieContainerResource> createResponse = kieServicesClient.createContainer(containerId, container); if(createResponse.getType() == ResponseType.FAILURE) { System.out.println("Error creating " + containerId + ". Message: "); System.out.println(responseDispose.getMsg()); return; } System.out.println("Container recreated with success!"); } } }
You define service responses using the
org.kie.server.api.model.ServiceResponse<T>
object, whereT
represents the type of returned response. TheServiceResponse
object has the following attributes:-
String message
: Returns the response message -
ResponseType type
: Returns eitherSUCCESS
orFAILURE
-
T result
: Returns the requested object
In this example, when you dispose a container, the
ServiceResponse
returns aVoid
response. When you create a container, theServiceResponse
returns aKieContainerResource
object.A conversation between a client and a specific KIE Server container in a clustered environment is secured by a unique conversationID
. TheconversationID
is transferred using theX-KIE-ConversationId
REST header. If you update the container, unset the previousconversationID
. UseKieServiesClient.completeConversation()
to unset theconversationID
for Java API. -
-
Run the configured
.java
class from your project directory to execute the request, and review the KIE Server response.If you enabled debug logging, KIE Server responds with a detailed response according to your configured marshalling format, such as JSON.
Example server response for a new KIE container (log):
10:23:35.194 [main] INFO o.k.s.a.m.MarshallerFactory - Marshaller extensions init 10:23:35.396 [main] DEBUG o.k.s.client.balancer.LoadBalancer - Load balancer RoundRobinBalancerStrategy{availableEndpoints=[http://localhost:8080/kie-server/services/rest/server]} selected url 'http://localhost:8080/kie-server/services/rest/server' 10:23:35.398 [main] DEBUG o.k.s.c.i.AbstractKieServicesClientImpl - About to send GET request to 'http://localhost:8080/kie-server/services/rest/server' 10:23:35.440 [main] DEBUG o.k.s.c.i.AbstractKieServicesClientImpl - About to deserialize content: '{ "type" : "SUCCESS", "msg" : "Kie Server info", "result" : { "kie-server-info" : { "id" : "default-kieserver", "version" : "7.11.0.Final-redhat-00003", "name" : "default-kieserver", "location" : "http://localhost:8080/kie-server/services/rest/server", "capabilities" : [ "KieServer", "BRM", "BPM", "CaseMgmt", "BPM-UI", "BRP", "DMN", "Swagger" ], "messages" : [ { "severity" : "INFO", "timestamp" : { "java.util.Date" : 1540814906533 }, "content" : [ "Server KieServerInfo{serverId='default-kieserver', version='7.11.0.Final-redhat-00003', name='default-kieserver', location='http://localhost:8080/kie-server/services/rest/server', capabilities=[KieServer, BRM, BPM, CaseMgmt, BPM-UI, BRP, DMN, Swagger], messages=null}started successfully at Mon Oct 29 08:08:26 EDT 2018" ] } ] } } }' into type: 'class org.kie.server.api.model.ServiceResponse' 10:23:35.653 [main] DEBUG o.k.s.c.impl.KieServicesClientImpl - KieServicesClient connected to: default-kieserver version 7.11.0.Final-redhat-00003 10:23:35.653 [main] DEBUG o.k.s.c.impl.KieServicesClientImpl - Supported capabilities by the server: [KieServer, BRM, BPM, CaseMgmt, BPM-UI, BRP, DMN, Swagger] 10:23:35.653 [main] DEBUG o.k.s.c.impl.KieServicesClientImpl - Building services client for server capability KieServer 10:23:35.653 [main] DEBUG o.k.s.c.impl.KieServicesClientImpl - No builder found for 'KieServer' capability 10:23:35.654 [main] DEBUG o.k.s.c.impl.KieServicesClientImpl - Building services client for server capability BRM 10:23:35.654 [main] DEBUG o.k.s.c.impl.KieServicesClientImpl - Builder 'org.kie.server.client.helper.DroolsServicesClientBuilder@6b927fb' for capability 'BRM' 10:23:35.655 [main] DEBUG o.k.s.c.impl.KieServicesClientImpl - Capability implemented by {interface org.kie.server.client.RuleServicesClient=org.kie.server.client.impl.RuleServicesClientImpl@4a94ee4} 10:23:35.655 [main] DEBUG o.k.s.c.impl.KieServicesClientImpl - Building services client for server capability BPM 10:23:35.656 [main] DEBUG o.k.s.c.impl.KieServicesClientImpl - Builder 'org.kie.server.client.helper.JBPMServicesClientBuilder@4cc451f2' for capability 'BPM' 10:23:35.672 [main] DEBUG o.k.s.c.impl.KieServicesClientImpl - Capability implemented by {interface org.kie.server.client.JobServicesClient=org.kie.server.client.impl.JobServicesClientImpl@1189dd52, interface org.kie.server.client.admin.ProcessAdminServicesClient=org.kie.server.client.admin.impl.ProcessAdminServicesClientImpl@36bc55de, interface org.kie.server.client.DocumentServicesClient=org.kie.server.client.impl.DocumentServicesClientImpl@564fabc8, interface org.kie.server.client.admin.UserTaskAdminServicesClient=org.kie.server.client.admin.impl.UserTaskAdminServicesClientImpl@16d04d3d, interface org.kie.server.client.QueryServicesClient=org.kie.server.client.impl.QueryServicesClientImpl@49ec71f8, interface org.kie.server.client.ProcessServicesClient=org.kie.server.client.impl.ProcessServicesClientImpl@1d2adfbe, interface org.kie.server.client.UserTaskServicesClient=org.kie.server.client.impl.UserTaskServicesClientImpl@36902638} 10:23:35.672 [main] DEBUG o.k.s.c.impl.KieServicesClientImpl - Building services client for server capability CaseMgmt 10:23:35.672 [main] DEBUG o.k.s.c.impl.KieServicesClientImpl - Builder 'org.kie.server.client.helper.CaseServicesClientBuilder@223d2c72' for capability 'CaseMgmt' 10:23:35.676 [main] DEBUG o.k.s.c.impl.KieServicesClientImpl - Capability implemented by {interface org.kie.server.client.admin.CaseAdminServicesClient=org.kie.server.client.admin.impl.CaseAdminServicesClientImpl@2b662a77, interface org.kie.server.client.CaseServicesClient=org.kie.server.client.impl.CaseServicesClientImpl@7f0eb4b4} 10:23:35.676 [main] DEBUG o.k.s.c.impl.KieServicesClientImpl - Building services client for server capability BPM-UI 10:23:35.676 [main] DEBUG o.k.s.c.impl.KieServicesClientImpl - Builder 'org.kie.server.client.helper.JBPMUIServicesClientBuilder@5c33f1a9' for capability 'BPM-UI' 10:23:35.677 [main] DEBUG o.k.s.c.impl.KieServicesClientImpl - Capability implemented by {interface org.kie.server.client.UIServicesClient=org.kie.server.client.impl.UIServicesClientImpl@223191a6} 10:23:35.678 [main] DEBUG o.k.s.c.impl.KieServicesClientImpl - Building services client for server capability BRP 10:23:35.678 [main] DEBUG o.k.s.c.impl.KieServicesClientImpl - Builder 'org.kie.server.client.helper.OptaplannerServicesClientBuilder@49139829' for capability 'BRP' 10:23:35.679 [main] DEBUG o.k.s.c.impl.KieServicesClientImpl - Capability implemented by {interface org.kie.server.client.SolverServicesClient=org.kie.server.client.impl.SolverServicesClientImpl@77fbd92c} 10:23:35.679 [main] DEBUG o.k.s.c.impl.KieServicesClientImpl - Building services client for server capability DMN 10:23:35.679 [main] DEBUG o.k.s.c.impl.KieServicesClientImpl - Builder 'org.kie.server.client.helper.DMNServicesClientBuilder@67c27493' for capability 'DMN' 10:23:35.680 [main] DEBUG o.k.s.c.impl.KieServicesClientImpl - Capability implemented by {interface org.kie.server.client.DMNServicesClient=org.kie.server.client.impl.DMNServicesClientImpl@35e2d654} 10:23:35.680 [main] DEBUG o.k.s.c.impl.KieServicesClientImpl - Building services client for server capability Swagger 10:23:35.680 [main] DEBUG o.k.s.c.impl.KieServicesClientImpl - No builder found for 'Swagger' capability 10:23:35.681 [main] DEBUG o.k.s.client.balancer.LoadBalancer - Load balancer RoundRobinBalancerStrategy{availableEndpoints=[http://localhost:8080/kie-server/services/rest/server]} selected url 'http://localhost:8080/kie-server/services/rest/server' 10:23:35.701 [main] DEBUG o.k.s.c.i.AbstractKieServicesClientImpl - About to send PUT request to 'http://localhost:8080/kie-server/services/rest/server/containers/employee-rostering3' with payload '{ "container-id" : null, "release-id" : { "group-id" : "employeerostering", "artifact-id" : "employeerostering", "version" : "1.0.0-SNAPSHOT" }, "resolved-release-id" : null, "status" : null, "scanner" : null, "config-items" : [ ], "messages" : [ ], "container-alias" : null }' 10:23:38.071 [main] DEBUG o.k.s.c.i.AbstractKieServicesClientImpl - About to deserialize content: '{ "type" : "SUCCESS", "msg" : "Container employee-rostering3 successfully deployed with module employeerostering:employeerostering:1.0.0-SNAPSHOT.", "result" : { "kie-container" : { "container-id" : "employee-rostering3", "release-id" : { "group-id" : "employeerostering", "artifact-id" : "employeerostering", "version" : "1.0.0-SNAPSHOT" }, "resolved-release-id" : { "group-id" : "employeerostering", "artifact-id" : "employeerostering", "version" : "1.0.0-SNAPSHOT" }, "status" : "STARTED", "scanner" : { "status" : "DISPOSED", "poll-interval" : null }, "config-items" : [ ], "messages" : [ { "severity" : "INFO", "timestamp" : { "java.util.Date" : 1540909418069 }, "content" : [ "Container employee-rostering3 successfully created with module employeerostering:employeerostering:1.0.0-SNAPSHOT." ] } ], "container-alias" : null } } }' into type: 'class org.kie.server.api.model.ServiceResponse'
If you encounter request errors, review the returned error code messages and adjust your Java configurations accordingly.
22.7.2. Supported KIE Server Java clients
The following are some of the Java client services available in the org.kie.server.client
package of your Drools distribution. You can use these services to interact with related resources in KIE Server similarly to the KIE Server REST API.
-
KieServicesClient
: Used as the entry point for other KIE Server Java clients, and used to interact with KIE containers -
JobServicesClient
: Used to schedule, cancel, re-queue, and get job requests -
RuleServicesClient
: Used to send commands to the server to perform rule-related operations, such as executing rules or inserting objects into the KIE session -
SolverServicesClient
: Used to perform all OptaPlanner operations, such as getting the solver state and the best solution, or disposing a solver
The getServicesClient
method provides access to any of these clients:
RuleServicesClient rulesClient = kieServicesClient.getServicesClient(RuleServicesClient.class);
For the full list of available KIE Server Java clients, see the Java client API source in GitHub.
22.7.3. Example requests with the KIE Server Java client API
The following are examples of KIE Server Java client API requests for basic interactions with KIE Server. For the full list of available KIE Server Java clients, see the Java client API source in GitHub.
- Listing KIE Server capabilities
-
You can use the
org.kie.server.api.model.KieServerInfo
object to identify server capabilities. TheKieServicesClient
client requires the server capability information to correctly produce service clients. You can specify the capabilities globally inKieServicesConfiguration
; otherwise they are automatically retrieved from KIE Server.Example request to return KIE Server capabilitiespublic void listCapabilities() { KieServerInfo serverInfo = kieServicesClient.getServerInfo().getResult(); System.out.print("Server capabilities:"); for (String capability : serverInfo.getCapabilities()) { System.out.print(" " + capability); } System.out.println(); }
- Listing KIE containers in KIE Server
-
KIE containers are represented by the
org.kie.server.api.model.KieContainerResource
object. The list of resources is represented by theorg.kie.server.api.model.KieContainerResourceList
object.Example request to return KIE containers from KIE Serverpublic void listContainers() { KieContainerResourceList containersList = kieServicesClient.listContainers().getResult(); List<KieContainerResource> kieContainers = containersList.getContainers(); System.out.println("Available containers: "); for (KieContainerResource container : kieContainers) { System.out.println("\t" + container.getContainerId() + " (" + container.getReleaseId() + ")"); } }
You can optionally filter the KIE container results using an instance of the
org.kie.server.api.model.KieContainerResourceFilter
class, which is passed to theorg.kie.server.client.KieServicesClient.listContainers()
method.Example request to return KIE containers by release ID and statuspublic void listContainersWithFilter() { // Filter containers by releaseId "org.example:container:1.0.0.Final" and status FAILED KieContainerResourceFilter filter = new KieContainerResourceFilter.Builder() .releaseId("org.example", "container", "1.0.0.Final") .status(KieContainerStatus.FAILED) .build(); // Using previously created KieServicesClient KieContainerResourceList containersList = kieServicesClient.listContainers(filter).getResult(); List<KieContainerResource> kieContainers = containersList.getContainers(); System.out.println("Available containers: "); for (KieContainerResource container : kieContainers) { System.out.println("\t" + container.getContainerId() + " (" + container.getReleaseId() + ")"); } }
- Creating and disposing KIE containers in KIE Server
-
You can use the
createContainer
anddisposeContainer
methods in theKieServicesClient
client to dispose and create KIE containers. In this example, when you dispose a container, theServiceResponse
returns aVoid
response. When you create a container, theServiceResponse
returns aKieContainerResource
object.Example request to dispose and re-create a KIE containerpublic void disposeAndCreateContainer() { System.out.println("== Disposing and creating containers =="); // Retrieve list of KIE containers List<KieContainerResource> kieContainers = kieServicesClient.listContainers().getResult().getContainers(); if (kieContainers.size() == 0) { System.out.println("No containers available..."); return; } // Dispose KIE container KieContainerResource container = kieContainers.get(0); String containerId = container.getContainerId(); ServiceResponse<Void> responseDispose = kieServicesClient.disposeContainer(containerId); if (responseDispose.getType() == ResponseType.FAILURE) { System.out.println("Error disposing " + containerId + ". Message: "); System.out.println(responseDispose.getMsg()); return; } System.out.println("Success Disposing container " + containerId); System.out.println("Trying to recreate the container..."); // Re-create KIE container ServiceResponse<KieContainerResource> createResponse = kieServicesClient.createContainer(containerId, container); if(createResponse.getType() == ResponseType.FAILURE) { System.out.println("Error creating " + containerId + ". Message: "); System.out.println(responseDispose.getMsg()); return; } System.out.println("Container recreated with success!"); }
- Executing runtime commands in KIE Server
-
Drools supports runtime commands that you can send to KIE Server for asset-related operations, such as inserting or retracting objects in a KIE session or firing all rules. The full list of supported runtime commands is located in the
org.drools.core.command.runtime
package in your Drools instance.You can use the
org.kie.api.command.KieCommands
class to insert commands, and useorg.kie.api.KieServices.get().getCommands()
to instantiate theKieCommands
class. If you want to add multiple commands, use theBatchExecutionCommand
wrapper.Example request to insert an object and fire all rulesimport org.kie.api.command.Command; import org.kie.api.command.KieCommands; import org.kie.server.api.model.ServiceResponse; import org.kie.server.client.RuleServicesClient; import org.kie.server.client.KieServicesClient; import org.kie.api.KieServices; import java.util.Arrays; ... public void executeCommands() { String containerId = "hello"; System.out.println("== Sending commands to the server =="); RuleServicesClient rulesClient = kieServicesClient.getServicesClient(RuleServicesClient.class); KieCommands commandsFactory = KieServices.Factory.get().getCommands(); Command<?> insert = commandsFactory.newInsert("Some String OBJ"); Command<?> fireAllRules = commandsFactory.newFireAllRules(); Command<?> batchCommand = commandsFactory.newBatchExecution(Arrays.asList(insert, fireAllRules)); ServiceResponse<String> executeResponse = rulesClient.executeCommands(containerId, batchCommand); if(executeResponse.getType() == ResponseType.SUCCESS) { System.out.println("Commands executed with success! Response: "); System.out.println(executeResponse.getResult()); } else { System.out.println("Error executing rules. Message: "); System.out.println(executeResponse.getMsg()); } }
A conversation between a client and a specific KIE Server container in a clustered environment is secured by a unique conversationID
. TheconversationID
is transferred using theX-KIE-ConversationId
REST header. If you update the container, unset the previousconversationID
. UseKieServiesClient.completeConversation()
to unset theconversationID
for Java API.
22.8. KIE Server and KIE container commands in Drools
Drools supports server commands that you can send to KIE Server for server-related or container-related operations, such as retrieving server information or creating or deleting a container. The full list of supported KIE Server configuration commands is located in the org.kie.server.api.commands
package in your Drools instance.
In the KIE Server REST API, you use the org.kie.server.api.commands
commands as the request body for POST
requests to http://SERVER:PORT/kie-server/services/rest/server/config
. For more information about using the KIE Server REST API, see KIE Server REST API for KIE containers and business assets.
In the KIE Server Java client API, you use the corresponding method in the parent KieServicesClient
Java client as an embedded API request in your Java application. All KIE Server commands are executed by methods provided in the Java client API, so you do not need to embed the actual KIE Server commands in your Java application. For more information about using the KIE Server Java client API, see KIE Server Java client API for KIE containers and business assets.
22.8.1. Sample KIE Server and KIE container commands
The following are sample KIE Server commands that you can use with the KIE Server REST API or Java client API for server-related or container-related operations in KIE Server:
-
GetServerInfoCommand
-
GetServerStateCommand
-
CreateContainerCommand
-
GetContainerInfoCommand
-
ListContainersCommand
-
CallContainerCommand
-
DisposeContainerCommand
-
GetScannerInfoCommand
-
UpdateScannerCommand
-
UpdateReleaseIdCommand
For the full list of supported KIE Server configuration and management commands, see the org.kie.server.api.commands
package in your Drools instance.
You can run KIE Server commands individually or together as a batch REST API request or batch Java API request:
{
"commands": [
{
"create-container": {
"container": {
"status": "STARTED",
"container-id": "command-script-container",
"release-id": {
"version": "1.0",
"group-id": "com.redhat",
"artifact-id": "Project1"
}
}
}
},
{
"call-container": {
"payload": "{\n \"commands\" : [ {\n \"fire-all-rules\" : {\n \"max\" : -1,\n \"out-identifier\" : null\n }\n } ]\n}",
"container-id": "command-script-container"
}
},
{
"dispose-container": {
"container-id": "command-script-container"
}
}
]
}
public void disposeAndCreateContainer() {
System.out.println("== Disposing and creating containers ==");
// Retrieve list of KIE containers
List<KieContainerResource> kieContainers = kieServicesClient.listContainers().getResult().getContainers();
if (kieContainers.size() == 0) {
System.out.println("No containers available...");
return;
}
// Dispose KIE container
KieContainerResource container = kieContainers.get(0);
String containerId = container.getContainerId();
ServiceResponse<Void> responseDispose = kieServicesClient.disposeContainer(containerId);
if (responseDispose.getType() == ResponseType.FAILURE) {
System.out.println("Error disposing " + containerId + ". Message: ");
System.out.println(responseDispose.getMsg());
return;
}
System.out.println("Success Disposing container " + containerId);
System.out.println("Trying to recreate the container...");
// Re-create KIE container
ServiceResponse<KieContainerResource> createResponse = kieServicesClient.createContainer(containerId, container);
if(createResponse.getType() == ResponseType.FAILURE) {
System.out.println("Error creating " + containerId + ". Message: ");
System.out.println(responseDispose.getMsg());
return;
}
System.out.println("Container recreated with success!");
}
Each command in this section includes a REST request body example (JSON) for the KIE Server REST API and an embedded method example from the KieServicesClient
Java client for the KIE Server Java client API.
- GetServerInfoCommand
-
Returns information about the KIE Server.
Example REST request body (JSON){ "commands" : [ { "get-server-info" : { } } ] }
Example Java client methodKieServerInfo serverInfo = kieServicesClient.getServerInfo();
Example server response (JSON){ "response": [ { "type": "SUCCESS", "msg": "Kie Server info", "result": { "kie-server-info": { "id": "default-kieserver", "version": "7.11.0.Final-redhat-00001", "name": "default-kieserver", "location": "http://localhost:8080/kie-server/services/rest/server", "capabilities": [ "KieServer", "BRM", "BPM", "CaseMgmt", "BPM-UI", "BRP", "DMN", "Swagger" ], "messages": [ { "severity": "INFO", "timestamp": { "java.util.Date": 1538502533321 }, "content": [ "Server KieServerInfo{serverId='default-kieserver', version='7.11.0.Final-redhat-00001', name='default-kieserver', location='http://localhost:8080/kie-server/services/rest/server', capabilities=[KieServer, BRM, BPM, CaseMgmt, BPM-UI, BRP, DMN, Swagger], messages=null}started successfully at Tue Oct 02 13:48:53 EDT 2018" ] } ] } } } ] }
- GetServerStateCommand
-
Returns information about the current state and configurations of the KIE Server.
Example REST request body (JSON){ "commands" : [ { "get-server-state" : { } } ] }
Example Java client methodKieServerStateInfo serverStateInfo = kieServicesClient.getServerState();
Example server response (JSON){ "response": [ { "type": "SUCCESS", "msg": "Successfully loaded server state for server id default-kieserver", "result": { "kie-server-state-info": { "controller": [ "http://localhost:8080/business-central/rest/controller" ], "config": { "config-items": [ { "itemName": "org.kie.server.location", "itemValue": "http://localhost:8080/kie-server/services/rest/server", "itemType": "java.lang.String" }, { "itemName": "org.kie.server.controller.user", "itemValue": "controllerUser", "itemType": "java.lang.String" }, { "itemName": "org.kie.server.controller", "itemValue": "http://localhost:8080/business-central/rest/controller", "itemType": "java.lang.String" } ] }, "containers": [ { "container-id": "employee-rostering", "release-id": { "group-id": "employeerostering", "artifact-id": "employeerostering", "version": "1.0.0-SNAPSHOT" }, "resolved-release-id": null, "status": "STARTED", "scanner": { "status": "STOPPED", "poll-interval": null }, "config-items": [ { "itemName": "KBase", "itemValue": "", "itemType": "BPM" }, { "itemName": "KSession", "itemValue": "", "itemType": "BPM" }, { "itemName": "MergeMode", "itemValue": "MERGE_COLLECTIONS", "itemType": "BPM" }, { "itemName": "RuntimeStrategy", "itemValue": "SINGLETON", "itemType": "BPM" } ], "messages": [], "container-alias": "employeerostering" } ] } } } ] }
- CreateContainerCommand
-
Creates a KIE container in the KIE Server.
Table 78. Command attributes Name Description Requirement container
Map containing the
container-id
,release-id
data (group ID, artifact ID, version),status
, and any other components of the new KIE containerRequired
Example REST request body (JSON){ "commands" : [ { "create-container" : { "container" : { "status" : null, "messages" : [ ], "container-id" : "command-script-container", "release-id" : { "version" : "1.0", "group-id" : "com.redhat", "artifact-id" : "Project1" }, "config-items" : [ ] } } } ] }
Example Java client methodServiceResponse<KieContainerResource> response = kieServicesClient.createContainer("command-script-container", resource);
Example server response (JSON){ "response": [ { "type": "SUCCESS", "msg": "Container command-script-container successfully deployed with module com.redhat:Project1:1.0.", "result": { "kie-container": { "container-id": "command-script-container", "release-id": { "version" : "1.0", "group-id" : "com.redhat", "artifact-id" : "Project1" }, "resolved-release-id": { "version" : "1.0", "group-id" : "com.redhat", "artifact-id" : "Project1" }, "status": "STARTED", "scanner": { "status": "DISPOSED", "poll-interval": null }, "config-items": [], "messages": [ { "severity": "INFO", "timestamp": { "java.util.Date": 1538762455510 }, "content": [ "Container command-script-container successfully created with module com.redhat:Project1:1.0." ] } ], "container-alias": null } } } ] }
- GetContainerInfoCommand
-
Returns information about a specified KIE container in KIE Server.
Table 79. Command attributes Name Description Requirement container-id
ID of the KIE container
Required
Example REST request body (JSON){ "commands" : [ { "get-container-info" : { "container-id" : "command-script-container" } } ] }
Example Java client methodServiceResponse<KieContainerResource> response = kieServicesClient.getContainerInfo("command-script-container");
Example server response (JSON){ "response": [ { "type": "SUCCESS", "msg": "Info for container command-script-container", "result": { "kie-container": { "container-id": "command-script-container", "release-id": { "group-id": "com.redhat", "artifact-id": "Project1", "version": "1.0" }, "resolved-release-id": { "group-id": "com.redhat", "artifact-id": "Project1", "version": "1.0" }, "status": "STARTED", "scanner": { "status": "DISPOSED", "poll-interval": null }, "config-items": [ ], "container-alias": null } } } ] }
- ListContainersCommand
-
Returns a list of KIE containers that have been created in the KIE Server.
Table 80. Command attributes Name Description Requirement kie-container-filter
Optional map containing
release-id-filter
,container-status-filter
, and any other KIE container properties by which you want to filter resultsOptional
Example REST request body (JSON){ "commands" : [ { "list-containers" : { "kie-container-filter" : { "release-id-filter" : { }, "container-status-filter" : { "accepted-status" : ["FAILED"] } } } } ] }
Example Java client methodKieContainerResourceFilter filter = new KieContainerResourceFilter.Builder() .status(KieContainerStatus.FAILED) .build(); KieContainerResourceList containersList = kieServicesClient.listContainers(filter);
Example server response (JSON){ "response": [ { "type": "SUCCESS", "msg": "List of created containers", "result": { "kie-containers": { "kie-container": [ { "container-id": "command-script-container", "release-id": { "group-id": "com.redhat", "artifact-id": "Project1", "version": "1.0" }, "resolved-release-id": { "group-id": "com.redhat", "artifact-id": "Project1", "version": "1.0" }, "status": "STARTED", "scanner": { "status": "STARTED", "poll-interval": 5000 }, "config-items": [ { "itemName": "RuntimeStrategy", "itemValue": "SINGLETON", "itemType": "java.lang.String" }, { "itemName": "MergeMode", "itemValue": "MERGE_COLLECTIONS", "itemType": "java.lang.String" }, { "itemName": "KBase", "itemValue": "", "itemType": "java.lang.String" }, { "itemName": "KSession", "itemValue": "", "itemType": "java.lang.String" } ], "messages": [ { "severity": "INFO", "timestamp": { "java.util.Date": 1538504619749 }, "content": [ "Container command-script-container successfully created with module com.redhat:Project1:1.0." ] } ], "container-alias": null } ] } } } ] }
- CallContainerCommand
-
Calls a KIE container and executes one or more runtime commands. For information about Drools runtime commands, see Runtime commands in Drools.
Table 81. Command attributes Name Description Requirement container-id
ID of the KIE container to be called
Required
payload
One or more commands in a
BatchExecutionCommand
wrapper to be executed on the KIE containerRequired
Example REST request body (JSON){ "commands" : [ { "call-container" : { "payload" : "{\n \"lookup\" : \"defaultKieSession\",\n \"commands\" : [ {\n \"fire-all-rules\" : {\n \"max\" : -1,\n \"out-identifier\" : null\n }\n } ]\n}", "container-id" : "command-script-container" } } ] }
Example Java client methodList<Command<?>> commands = new ArrayList<Command<?>>(); BatchExecutionCommand batchExecution1 = commandsFactory.newBatchExecution(commands, "defaultKieSession"); commands.add(commandsFactory.newFireAllRules()); ServiceResponse<ExecutionResults> response1 = ruleClient.executeCommandsWithResults("command-script-container", batchExecution1);
Example server response (JSON){ "response": [ { "type": "SUCCESS", "msg": "Container command-script-container successfully called.", "result": "{\n \"results\" : [ ],\n \"facts\" : [ ]\n}" } ] }
- DisposeContainerCommand
-
Disposes a specified KIE container in the KIE Server.
Table 82. Command attributes Name Description Requirement container-id
ID of the KIE container to be disposed
Required
Example REST request body (JSON){ "commands" : [ { "dispose-container" : { "container-id" : "command-script-container" } } ] }
Example Java client methodServiceResponse<Void> response = kieServicesClient.disposeContainer("command-script-container");
Example server response (JSON){ "response": [ { "type": "SUCCESS", "msg": "Container command-script-container successfully disposed.", "result": null } ] }
- GetScannerInfoCommand
-
Returns information about the KIE scanner used for automatic updates in a specified KIE container, if applicable.
Table 83. Command attributes Name Description Requirement container-id
ID of the KIE container where the KIE scanner is used
Required
Example REST request body (JSON){ "commands" : [ { "get-scanner-info" : { "container-id" : "command-script-container" } } ] }
Example Java client methodServiceResponse<KieScannerResource> response = kieServicesClient.getScannerInfo("command-script-container");
Example server response (JSON){ "response": [ { "type": "SUCCESS", "msg": "Scanner info successfully retrieved", "result": { "kie-scanner": { "status": "DISPOSED", "poll-interval": null } } } ] }
- UpdateScannerCommand
-
Starts or stops a KIE scanner that controls polling for updated KIE container deployments.
Avoid using a KIE scanner with business processes. Using a KIE scanner with processes can lead to unforeseen updates that can then cause errors in long-running processes when changes are not compatible with running process instances. Table 84. Command attributes Name Description Requirement container-id
ID of the KIE container where the KIE scanner is used
Required
status
Status to be set on the KIE scanner (
STARTED
,STOPPED
)Required
poll-interval
Permitted polling duration in milliseconds
Required only when starting scanner
Example REST request body (JSON){ "commands" : [ { "update-scanner" : { "scanner" : { "status" : "STARTED", "poll-interval" : 10000 }, "container-id" : "command-script-container" } } ] }
Example Java client methodKieScannerResource scannerResource = new KieScannerResource(); scannerResource.setPollInterval(10000); scannerResource.setStatus(KieScannerStatus. STARTED); ServiceResponse<KieScannerResource> response = kieServicesClient.updateScanner("command-script-container", scannerResource);
Example server response (JSON){ "response": [ { "type": "SUCCESS", "msg": "Kie scanner successfully created.", "result": { "kie-scanner": { "status": "STARTED", "poll-interval": 10000 } } } ] }
- UpdateReleaseIdCommand
-
Updates the release ID data (group ID, artifact ID, version) for a specified KIE container.
Table 85. Command attributes Name Description Requirement container-id
ID of the KIE container to be updated
Required
releaseId
Updated GAV (group ID, artifact ID, version) data to be applied to the KIE container
Required
Example REST request body (JSON){ "commands" : [ { "update-release-id" : { "releaseId" : { "version" : "1.1", "group-id" : "com.redhat", "artifact-id" : "Project1" }, "container-id" : "command-script-container" } } ] }
Example Java client methodServiceResponse<ReleaseId> response = kieServicesClient.updateReleaseId("command-script-container", "com.redhat:Project1:1.1");
Example server response (JSON){ "response": [ { "type": "SUCCESS", "msg": "Release id successfully updated", "result": { "release-id": { "group-id": "com.redhat", "artifact-id": "Project1", "version": "1.1" } } } ] }
22.9. Runtime commands in Drools
Drools supports runtime commands that you can send to KIE Server for asset-related operations, such as executing all rules or inserting or retracting objects in a KIE session. The full list of supported runtime commands is located in the org.drools.core.command.runtime
package in your Drools instance.
In the KIE Server REST API, you use the global org.drools.core.command.runtime
commands or the rule-specific org.drools.core.command.runtime.rule
commands as the request body for POST
requests to http://SERVER:PORT/kie-server/services/rest/server/containers/instances/{containerId}
. For more information about using the KIE Server REST API, see KIE Server REST API for KIE containers and business assets.
In the KIE Server Java client API, you can embed these commands in your Java application along with the relevant Java client. For example, for rule-related commands, you use the RuleServicesClient
Java client with the embedded commands. For more information about using the KIE Server Java client API, see KIE Server Java client API for KIE containers and business assets.
22.9.1. Sample runtime commands in Drools
The following are sample runtime commands that you can use with the KIE Server REST API or Java client API for asset-related operations in KIE Server:
-
BatchExecutionCommand
-
InsertObjectCommand
-
RetractCommand
-
ModifyCommand
-
GetObjectCommand
-
GetObjectsCommand
-
InsertElementsCommand
-
FireAllRulesCommand
-
QueryCommand
-
SetGlobalCommand
-
GetGlobalCommand
For the full list of supported runtime commands, see the org.drools.core.command.runtime
package in your Drools instance.
Each command in this section includes a REST request body example (JSON) for the KIE Server REST API and an embedded Java command example for the KIE Server Java client API. The Java examples use an object org.drools.compiler.test.Person
with the fields name
(String) and age
(Integer).
- BatchExecutionCommand
-
Contains multiple commands to be executed together.
Table 86. Command attributes Name Description Requirement commands
List of commands to be executed.
Required
lookup
Sets the KIE session ID on which the commands will be executed. For stateless KIE sessions, this attribute is required. For stateful KIE sessions, this attribute is optional and if not specified, the default KIE session is used.
Required for stateless KIE session, optional for stateful KIE session
Example JSON request body{ "lookup": "ksession1", "commands": [ { "insert": { "object": { "org.drools.compiler.test.Person": { "name": "john", "age": 25 } } } }, { "fire-all-rules": { "max": 10, "out-identifier": "firedActivations" } } ] }
Example Java commandBatchExecutionCommand command = new BatchExecutionCommand(); command.setLookup("ksession1"); InsertObjectCommand insertObjectCommand = new InsertObjectCommand(new Person("john", 25)); FireAllRulesCommand fireAllRulesCommand = new FireAllRulesCommand(); command.getCommands().add(insertObjectCommand); command.getCommands().add(fireAllRulesCommand); ksession.execute(command);
Example server response (JSON){ "response": [ { "type": "SUCCESS", "msg": "Container command-script-container successfully called.", "result": { "execution-results": { "results": [ { "value": 0, "key": "firedActivations" } ], "facts": [] } } } ] }
- InsertObjectCommand
-
Inserts an object into the KIE session.
Table 87. Command attributes Name Description Requirement object
The object to be inserted
Required
out-identifier
ID of the
FactHandle
created from the object insertion and added to the execution resultsOptional
return-object
Boolean to determine whether the object must be returned in the execution results (default:
true
)Optional
entry-point
Entry point for the insertion
Optional
Example JSON request body{ "commands": [ { "insert": { "entry-point": "my stream", "object": { "org.drools.compiler.test.Person": { "age": 25, "name": "john" } }, "out-identifier": "john", "return-object": false } } ] }
Example Java commandCommand insertObjectCommand = CommandFactory.newInsert(new Person("john", 25), "john", false, null); ksession.execute(insertObjectCommand);
Example server response (JSON){ "response": [ { "type": "SUCCESS", "msg": "Container command-script-container successfully called.", "result": { "execution-results": { "results": [], "facts": [ { "value": { "org.drools.core.common.DefaultFactHandle": { "external-form": "0:4:436792766:-2127720265:4:DEFAULT:NON_TRAIT:java.util.LinkedHashMap" } }, "key": "john" } ] } } } ] }
- RetractCommand
-
Retracts an object from the KIE session.
Table 88. Command attributes Name Description Requirement fact-handle
The
FactHandle
associated with the object to be retractedRequired
Example JSON request body{ "commands": [ { "retract": { "fact-handle": "0:4:436792766:-2127720265:4:DEFAULT:NON_TRAIT:java.util.LinkedHashMap" } } ] }
Example Java command: UseFactHandleFromString
RetractCommand retractCommand = new RetractCommand(); retractCommand.setFactHandleFromString("123:234:345:456:567");
Example Java command: UseFactHandle
from inserted objectRetractCommand retractCommand = new RetractCommand(factHandle);
Example server response (JSON){ "response": [ { "type": "SUCCESS", "msg": "Container employee-rostering successfully called.", "result": { "execution-results": { "results": [], "facts": [] } } } ] }
- ModifyCommand
-
Modifies a previously inserted object in the KIE session.
Table 89. Command attributes Name Description Requirement fact-handle
The
FactHandle
associated with the object to be modifiedRequired
setters
List of setters for object modifications
Required
Example JSON request body{ "commands": [ { "modify": { "fact-handle": "0:4:436792766:-2127720265:4:DEFAULT:NON_TRAIT:java.util.LinkedHashMap", "setters": { "accessor": "age", "value": 25 } } } ] }
Example Java commandModifyCommand modifyCommand = new ModifyCommand(factHandle); List<Setter> setters = new ArrayList<Setter>(); setters.add(new SetterImpl("age", "25")); modifyCommand.setSetters(setters);
Example server response (JSON){ "response": [ { "type": "SUCCESS", "msg": "Container employee-rostering successfully called.", "result": { "execution-results": { "results": [], "facts": [] } } } ] }
- GetObjectCommand
-
Retrieves an object from a KIE session.
Table 90. Command attributes Name Description Requirement fact-handle
The
FactHandle
associated with the object to be retrievedRequired
out-identifier
ID of the
FactHandle
created from the object insertion and added to the execution resultsOptional
Example JSON request body{ "commands": [ { "get-object": { "fact-handle": "0:4:436792766:-2127720265:4:DEFAULT:NON_TRAIT:java.util.LinkedHashMap", "out-identifier": "john" } } ] }
Example Java commandGetObjectCommand getObjectCommand = new GetObjectCommand(); getObjectCommand.setFactHandleFromString("123:234:345:456:567"); getObjectCommand.setOutIdentifier("john");
Example server response (JSON){ "response": [ { "type": "SUCCESS", "msg": "Container command-script-container successfully called.", "result": { "execution-results": { "results": [ { "value": null, "key": "john" } ], "facts": [] } } } ] }
- GetObjectsCommand
-
Retrieves all objects from the KIE session as a collection.
Table 91. Command attributes Name Description Requirement object-filter
Filter for the objects returned from the KIE session
Optional
out-identifier
Identifier to be used in the execution results
Optional
Example JSON request body{ "commands": [ { "get-objects": { "out-identifier": "objects" } } ] }
Example Java commandGetObjectsCommand getObjectsCommand = new GetObjectsCommand(); getObjectsCommand.setOutIdentifier("objects");
Example server response (JSON){ "response": [ { "type": "SUCCESS", "msg": "Container command-script-container successfully called.", "result": { "execution-results": { "results": [ { "value": [ { "org.apache.xerces.dom.ElementNSImpl": "<?xml version=\"1.0\" encoding=\"UTF-16\"?>\n<object xmlns:xsi=\"http://www.w3.org/2001/XMLSchema-instance\" xsi:type=\"person\"><age>25</age><name>john</name>\n <\/object>" }, { "org.drools.compiler.test.Person": { "name": "john", "age": 25 } } ], "key": "objects" } ], "facts": [] } } } ] }
- InsertElementsCommand
-
Inserts a list of objects into the KIE session.
Table 92. Command attributes Name Description Requirement objects
The list of objects to be inserted into the KIE session
Required
out-identifier
ID of the
FactHandle
created from the object insertion and added to the execution resultsOptional
return-object
Boolean to determine whether the object must be returned in the execution results. Default value:
true
.Optional
entry-point
Entry point for the insertion
Optional
Example JSON request body{ "commands": [ { "insert-elements": { "objects": [ { "containedObject": { "@class": "org.drools.compiler.test.Person", "age": 25, "name": "john" } }, { "containedObject": { "@class": "Person", "age": 35, "name": "sarah" } } ] } } ] }
Example Java commandList<Object> objects = new ArrayList<Object>(); objects.add(new Person("john", 25)); objects.add(new Person("sarah", 35)); Command insertElementsCommand = CommandFactory.newInsertElements(objects);
Example server response (JSON){ "response": [ { "type": "SUCCESS", "msg": "Container command-script-container successfully called.", "result": { "execution-results": { "results": [], "facts": [ { "value": { "org.drools.core.common.DefaultFactHandle": { "external-form": "0:4:436792766:-2127720265:4:DEFAULT:NON_TRAIT:java.util.LinkedHashMap" } }, "key": "john" }, { "value": { "org.drools.core.common.DefaultFactHandle": { "external-form": "0:4:436792766:-2127720266:4:DEFAULT:NON_TRAIT:java.util.LinkedHashMap" } }, "key": "sarah" } ] } } } ] }
- FireAllRulesCommand
-
Executes all rules in the KIE session.
Table 93. Command attributes Name Description Requirement max
Maximum number of rules to be executed. The default is
-1
and does not put any restriction on execution.Optional
out-identifier
ID to be used for retrieving the number of fired rules in execution results.
Optional
agenda-filter
Agenda Filter to be used for rule execution.
Optional
Example JSON request body{ "commands" : [ { "fire-all-rules": { "max": 10, "out-identifier": "firedActivations" } } ] }
Example Java commandFireAllRulesCommand fireAllRulesCommand = new FireAllRulesCommand(); fireAllRulesCommand.setMax(10); fireAllRulesCommand.setOutIdentifier("firedActivations");
Example server response (JSON){ "response": [ { "type": "SUCCESS", "msg": "Container command-script-container successfully called.", "result": { "execution-results": { "results": [ { "value": 0, "key": "firedActivations" } ], "facts": [] } } } ] }
- QueryCommand
-
Executes a query defined in the KIE base.
Table 94. Command attributes Name Description Requirement name
Query name.
Required
out-identifier
ID of the query results. The query results are added in the execution results with this identifier.
Optional
arguments
List of objects to be passed as a query parameter.
Optional
Example JSON request body{ "commands": [ { "query": { "name": "persons", "arguments": [], "out-identifier": "persons" } } ] }
Example Java commandQueryCommand queryCommand = new QueryCommand(); queryCommand.setName("persons"); queryCommand.setOutIdentifier("persons");
Example server response (JSON){ "type": "SUCCESS", "msg": "Container stateful-session successfully called.", "result": { "execution-results": { "results": [ { "value": { "org.drools.core.runtime.rule.impl.FlatQueryResults": { "idFactHandleMaps": { "type": "LIST", "componentType": null, "element": [ { "type": "MAP", "componentType": null, "element": [ { "value": { "org.drools.core.common.DisconnectedFactHandle": { "id": 1, "identityHashCode": 1809949690, "objectHashCode": 1809949690, "recency": 1, "object": { "org.kie.server.testing.Person": { "fullname": "John Doe", "age": 47 } }, "entryPointId": "DEFAULT", "traitType": "NON_TRAIT", "external-form": "0:1:1809949690:1809949690:1:DEFAULT:NON_TRAIT:org.kie.server.testing.Person" } }, "key": "$person" } ] } ] }, "idResultMaps": { "type": "LIST", "componentType": null, "element": [ { "type": "MAP", "componentType": null, "element": [ { "value": { "org.kie.server.testing.Person": { "fullname": "John Doe", "age": 47 } }, "key": "$person" } ] } ] }, "identifiers": { "type": "SET", "componentType": null, "element": [ "$person" ] } } }, "key": "persons" } ], "facts": [] } } }
- SetGlobalCommand
-
Sets an object to a global state.
Table 95. Command attributes Name Description Requirement identifier
ID of the global variable defined in the KIE base
Required
object
Object to be set into the global variable
Optional
out
Boolean to exclude the global variable you set from the execution results
Optional
out-identifier
ID of the global execution result
Optional
Example JSON request body{ "commands": [ { "set-global": { "identifier": "helper", "object": { "org.kie.server.testing.Person": { "fullname": "kyle", "age": 30 } }, "out-identifier": "output" } } ] }
Example Java commandSetGlobalCommand setGlobalCommand = new SetGlobalCommand(); setGlobalCommand.setIdentifier("helper"); setGlobalCommand.setObject(new Person("kyle", 30)); setGlobalCommand.setOut(true); setGlobalCommand.setOutIdentifier("output");
Example server response (JSON){ "type": "SUCCESS", "msg": "Container stateful-session successfully called.", "result": { "execution-results": { "results": [ { "value": { "org.kie.server.testing.Person": { "fullname": "kyle", "age": 30 } }, "key": "output" } ], "facts": [] } } }
- GetGlobalCommand
-
Retrieves a previously defined global object.
Table 96. Command attributes Name Description Requirement identifier
ID of the global variable defined in the KIE base
Required
out-identifier
ID to be used in the execution results
Optional
Example JSON request body{ "commands": [ { "get-global": { "identifier": "helper", "out-identifier": "helperOutput" } } ] }
Example Java commandGetGlobalCommand getGlobalCommand = new GetGlobalCommand(); getGlobalCommand.setIdentifier("helper"); getGlobalCommand.setOutIdentifier("helperOutput");
Example server response (JSON){ "response": [ { "type": "SUCCESS", "msg": "Container command-script-container successfully called.", "result": { "execution-results": { "results": [ { "value": null, "key": "helperOutput" } ], "facts": [] } } } ] }
22.10. Drools controller REST API for KIE Server templates and instances
Drools provides a Drools controller REST API that you can use to interact with your KIE Server templates (configurations), KIE Server instances (remote servers), and associated KIE containers (deployment units) in Drools without using the Business Central user interface. This API support enables you to maintain your Drools servers and resources more efficiently and optimize your integration and development with Drools.
With the Drools controller REST API, you can perform the following actions:
-
Retrieve information about KIE Server templates, instances, and associated KIE containers
-
Update, start, or stop KIE containers associated with KIE Server templates and instances
-
Create, update, or delete KIE Server templates
-
Create, update, or delete KIE Server instances
Requests to the Drools controller REST API require the following components:
- Authentication
-
The Drools controller REST API requires HTTP Basic authentication or token-based authentication for the following user roles, depending on controller type:
-
rest-all
user role if you installed Business Central and you want to use the built-in Drools controller -
kie-server
user role if you installed the headless Drools controller separately from Business Central
To view configured user roles for your Drools distribution, navigate to
~/$SERVER_HOME/standalone/configuration/application-roles.properties
and~/application-users.properties
.To add a user with the
kie-server
role or therest-all
role or both, navigate to~/$SERVER_HOME/bin
and run the following command with the role or roles specified:$ ./add-user.sh -a --user <USERNAME> --password <PASSWORD> --role kie-server,rest-all
To configure the
kie-server
orrest-all
user with Drools controller access, navigate to~/$SERVER_HOME/standalone/configuration/standalone-full.xml
, uncomment theorg.kie.server
properties (if applicable), and add the controller user login credentials and controller location (if needed):<property name="org.kie.server.location" value="http://localhost:8080/kie-server/services/rest/server"/> <property name="org.kie.server.controller" value="http://localhost:8080/business-central/rest/controller"/> <property name="org.kie.server.controller.user" value="baAdmin"/> <property name="org.kie.server.controller.pwd" value="password@1"/> <property name="org.kie.server.id" value="default-kieserver"/>
For more information about user roles and Drools installation options, see Installing the KIE Server.
-
- HTTP headers
-
The Drools controller REST API requires the following HTTP headers for API requests:
-
Accept
: Data format accepted by your requesting client:-
application/json
(JSON) -
application/xml
(XML, for JAXB)
-
-
Content-Type
: Data format of yourPOST
orPUT
API request data:-
application/json
(JSON) -
application/xml
(XML, for JAXB)
-
-
- HTTP methods
-
The Drools controller REST API supports the following HTTP methods for API requests:
-
GET
: Retrieves specified information from a specified resource endpoint -
POST
: Updates a resource or resource instance -
PUT
: Creates a resource or resource instance -
DELETE
: Deletes a resource or resource instance
-
- Base URL
-
The base URL for Drools controller REST API requests is
http://SERVER:PORT/CONTROLLER/rest/
, such ashttp://localhost:8080/business-central/rest/
if you are using the Drools controller built in to Business Central. - Endpoints
-
Drools controller REST API endpoints, such as
/controller/management/servers/{serverTemplateId}
for a specified KIE Server template, are the URIs that you append to the Drools controller REST API base URL to access the corresponding server resource or type of server resource in Drools.Example request URL for/controller/management/servers/{serverTemplateId}
endpointhttp://localhost:8080/business-central/rest/controller/management/servers/default-kieserver
- Request parameters and request data
-
Some Drools controller REST API requests require specific parameters in the request URL path to identify or filter specific resources and to perform specific actions. You can append URL parameters to the endpoint in the format
?<PARAM>=<VALUE>&<PARAM>=<VALUE>
.Example DELETE request URL with parametershttp://localhost:8080/business-central/rest/controller/server/new-kieserver-instance?location=http://localhost:8080/kie-server/services/rest/server
HTTP
POST
andPUT
requests may additionally require a request body or file with data to accompany the request.Example PUT request URL and JSON request body datahttp://localhost:8080/business-central/rest/controller/management/servers/new-kieserver
{ "server-id": "new-kieserver", "server-name": "new-kieserver", "container-specs": [], "server-config": {}, "capabilities": [ "RULE", "PROCESS", "PLANNING" ] }
22.10.1. Sending requests with the Drools controller REST API using a REST client or curl utility
The Drools controller REST API enables you to interact with your KIE Server templates (configurations), KIE Server instances (remote servers), and associated KIE containers (deployment units) in Drools without using the Business Central user interface. You can send Drools controller REST API requests using any REST client or curl utility.
-
KIE Server is installed and running.
-
The Drools controller or headless Drools controller is installed and running.
-
You have
rest-all
user role access to the Drools controller if you installed Business Central, orkie-server
user role access to the headless Drools controller installed separately from Business Central.
-
Identify the relevant API endpoint to which you want to send a request, such as
[GET] /controller/management/servers
to retrieve KIE Server templates from the Drools controller. -
In a REST client or curl utility, enter the following components for a
GET
request tocontroller/management/servers
. Adjust any request details according to your use case.For REST client:
-
Authentication: Enter the user name and password of the Drools controller user with the
rest-all
role or the headless Drools controller user with thekie-server
role. -
HTTP Headers: Set the following header:
-
Accept
:application/json
-
-
HTTP method: Set to
GET
. -
URL: Enter the Drools controller REST API base URL and endpoint, such as
http://localhost:8080/business-central/rest/controller/management/servers
.
For curl utility:
-
-u
: Enter the user name and password of the Drools controller user with therest-all
role or the headless Drools controller user with thekie-server
role. -
-H
: Set the following header:-
accept
:application/json
-
-
-X
: Set toGET
. -
URL: Enter the Drools controller REST API base URL and endpoint, such as
http://localhost:8080/business-central/rest/controller/management/servers
.
curl -u 'baAdmin:password@1' -H "accept: application/json" -X GET "http://localhost:8080/business-central/rest/controller/management/servers"
-
-
Execute the request and review the Drools controller response.
Example server response (JSON):
{ "server-template": [ { "server-id": "default-kieserver", "server-name": "default-kieserver", "container-specs": [ { "container-id": "employeerostering_1.0.0-SNAPSHOT", "container-name": "employeerostering", "server-template-key": { "server-id": "default-kieserver", "server-name": "default-kieserver" }, "release-id": { "group-id": "employeerostering", "artifact-id": "employeerostering", "version": "1.0.0-SNAPSHOT" }, "configuration": { "RULE": { "org.kie.server.controller.api.model.spec.RuleConfig": { "pollInterval": null, "scannerStatus": "STOPPED" } }, "PROCESS": { "org.kie.server.controller.api.model.spec.ProcessConfig": { "runtimeStrategy": "SINGLETON", "kbase": "", "ksession": "", "mergeMode": "MERGE_COLLECTIONS" } } }, "status": "STARTED" }, { "container-id": "mortgage-process_1.0.0-SNAPSHOT", "container-name": "mortgage-process", "server-template-key": { "server-id": "default-kieserver", "server-name": "default-kieserver" }, "release-id": { "group-id": "mortgage-process", "artifact-id": "mortgage-process", "version": "1.0.0-SNAPSHOT" }, "configuration": { "RULE": { "org.kie.server.controller.api.model.spec.RuleConfig": { "pollInterval": null, "scannerStatus": "STOPPED" } }, "PROCESS": { "org.kie.server.controller.api.model.spec.ProcessConfig": { "runtimeStrategy": "PER_PROCESS_INSTANCE", "kbase": "", "ksession": "", "mergeMode": "MERGE_COLLECTIONS" } } }, "status": "STARTED" } ], "server-config": {}, "server-instances": [ { "server-instance-id": "default-kieserver-instance@localhost:8080", "server-name": "default-kieserver-instance@localhost:8080", "server-template-id": "default-kieserver", "server-url": "http://localhost:8080/kie-server/services/rest/server" } ], "capabilities": [ "RULE", "PROCESS", "PLANNING" ] } ] }
-
In your REST client or curl utility, send another API request with the following components for a
PUT
request to/controller/management/servers/{serverTemplateId}
to create a new KIE Server template. Adjust any request details according to your use case.For REST client:
-
Authentication: Enter the user name and password of the Drools controller user with the
rest-all
role or the headless Drools controller user with thekie-server
role. -
HTTP Headers: Set the following headers:
-
Accept
:application/json
-
Content-Type
:application/json
-
-
HTTP method: Set to
PUT
. -
URL: Enter the Drools controller REST API base URL and endpoint, such as
http://localhost:8080/business-central/rest/controller/management/servers/new-kieserver
. -
Request body: Add a JSON request body with the configurations for the new KIE Server template:
{ "server-id": "new-kieserver", "server-name": "new-kieserver", "container-specs": [], "server-config": {}, "capabilities": [ "RULE", "PROCESS", "PLANNING" ] }
For curl utility:
-
-u
: Enter the user name and password of the Drools controller user with therest-all
role or the headless Drools controller user with thekie-server
role. -
-H
: Set the following headers:-
accept
:application/json
-
content-type
:application/json
-
-
-X
: Set toPUT
. -
URL: Enter the Drools controller REST API base URL and endpoint, such as
http://localhost:8080/business-central/rest/controller/management/servers/new-kieserver
. -
-d
: Add a JSON request body or file (@file.json
) with the configurations for the new KIE Server template:
curl -u 'baAdmin:password@1' -H "accept: application/json" -H "content-type: application/json" -X PUT "http://localhost:8080/business-central/rest/controller/management/servers/new-kieserver" -d "{ \"server-id\": \"new-kieserver\", \"server-name\": \"new-kieserver\", \"container-specs\": [], \"server-config\": {}, \"capabilities\": [ \"RULE\", \"PROCESS\", \"PLANNING\" ]}"
curl -u 'baAdmin:password@1' -H "accept: application/json" -H "content-type: application/json" -X PUT "http://localhost:8080/business-central/rest/controller/management/servers/new-kieserver" -d @my-server-template-configs.json
-
-
Execute the request and confirm the successful Drools controller response.
If you encounter request errors, review the returned error code messages and adjust your request accordingly.
22.10.2. Sending requests with the Drools controller REST API using the Swagger interface
The Drools controller REST API supports a Swagger web interface that you can use instead of a standalone REST client or curl utility to interact with your KIE Server templates, instances, and associated KIE containers in Drools without using the Business Central user interface.
-
The Drools controller is installed and running.
-
You have
rest-all
user role access to the Drools controller if you installed Business Central, orkie-server
user role access to the headless Drools controller installed separately from Business Central.
-
In a web browser, navigate to
http://SERVER:PORT/CONTROLLER/docs
, such ashttp://localhost:8080/business-central/docs
, and log in with the user name and password of the Drools controller user with therest-all
role or the headless Drools controller user with thekie-server
role.If you are using the Drools controller built in to Business Central, the Swagger page associated with the Drools controller is identified as the "Business Central API" for Business Central REST services. If you are using the headless Drools controller without Business Central, the Swagger page associated with the headless Drools controller is identified as the "Controller API". The Drools controller REST API endpoints in both cases are the same. -
In the Swagger page, select the relevant API endpoint to which you want to send a request, such as Controller :: KIE Server templates and KIE containers → [GET] /controller/management/servers to retrieve KIE Server templates from the Drools controller.
-
Click Try it out and provide any optional parameters by which you want to filter results, if applicable.
-
In the Response content type drop-down menu, select the desired format of the server response, such as application/json for JSON format.
-
Click Execute and review the KIE Server response.
Example server response (JSON):
{ "server-template": [ { "server-id": "default-kieserver", "server-name": "default-kieserver", "container-specs": [ { "container-id": "employeerostering_1.0.0-SNAPSHOT", "container-name": "employeerostering", "server-template-key": { "server-id": "default-kieserver", "server-name": "default-kieserver" }, "release-id": { "group-id": "employeerostering", "artifact-id": "employeerostering", "version": "1.0.0-SNAPSHOT" }, "configuration": { "RULE": { "org.kie.server.controller.api.model.spec.RuleConfig": { "pollInterval": null, "scannerStatus": "STOPPED" } }, "PROCESS": { "org.kie.server.controller.api.model.spec.ProcessConfig": { "runtimeStrategy": "SINGLETON", "kbase": "", "ksession": "", "mergeMode": "MERGE_COLLECTIONS" } } }, "status": "STARTED" }, { "container-id": "mortgage-process_1.0.0-SNAPSHOT", "container-name": "mortgage-process", "server-template-key": { "server-id": "default-kieserver", "server-name": "default-kieserver" }, "release-id": { "group-id": "mortgage-process", "artifact-id": "mortgage-process", "version": "1.0.0-SNAPSHOT" }, "configuration": { "RULE": { "org.kie.server.controller.api.model.spec.RuleConfig": { "pollInterval": null, "scannerStatus": "STOPPED" } }, "PROCESS": { "org.kie.server.controller.api.model.spec.ProcessConfig": { "runtimeStrategy": "PER_PROCESS_INSTANCE", "kbase": "", "ksession": "", "mergeMode": "MERGE_COLLECTIONS" } } }, "status": "STARTED" } ], "server-config": {}, "server-instances": [ { "server-instance-id": "default-kieserver-instance@localhost:8080", "server-name": "default-kieserver-instance@localhost:8080", "server-template-id": "default-kieserver", "server-url": "http://localhost:8080/kie-server/services/rest/server" } ], "capabilities": [ "RULE", "PROCESS", "PLANNING" ] } ] }
-
In the Swagger page, navigate to the Controller :: KIE Server templates and KIE containers → [GET] /controller/management/servers/{serverTemplateId} endpoint to send another request to create a new KIE Server template. Adjust any request details according to your use case.
-
Click Try it out and enter the following components for the request:
-
serverTemplateId: Enter the ID of the new KIE Server template, such as
new-kieserver
. -
body: Set the Parameter content type to the desired request body format, such as application/json for JSON format, and add a request body with the configurations for the new KIE Server template:
{ "server-id": "new-kieserver", "server-name": "new-kieserver", "container-specs": [], "server-config": {}, "capabilities": [ "RULE", "PROCESS", "PLANNING" ] }
-
-
In the Response content type drop-down menu, select the desired format of the server response, such as application/json for JSON format.
-
Click Execute and confirm the successful Drools controller response.
If you encounter request errors, review the returned error code messages and adjust your request accordingly.
22.10.3. Supported Drools controller REST API endpoints
The Drools controller REST API provides endpoints for interacting with KIE Server templates (configurations), KIE Server instances (remote servers), and associated KIE containers (deployment units). The Drools controller REST API base URL is http://SERVER:PORT/CONTROLLER/rest/
. All requests require HTTP Basic authentication or token-based authentication for the rest-all
user role if you installed Business Central and you want to use the built-in Drools controller, or the kie-server
user role if you installed the headless Drools controller separately from Business Central.
For the full list of Drools controller REST API endpoints and descriptions, use one of the following resources:
-
Controller REST API on the jBPM Documentation page (static)
-
Swagger UI for the Drools controller REST API at
http://SERVER:PORT/CONTROLLER/docs
(dynamic, requires running Drools controller)If you are using the Drools controller built in to Business Central, the Swagger page associated with the Drools controller is identified as the "Business Central API" for Business Central REST services. If you are using the headless Drools controller without Business Central, the Swagger page associated with the headless Drools controller is identified as the "Controller API". The Drools controller REST API endpoints in both cases are the same.
22.11. Drools controller Java client API for KIE Server templates and instances
Drools provides a Drools controller Java client API that enables you to connect to the Drools controller using REST or WebSocket protocol from your Java client application. You can use the Drools controller Java client API as an alternative to the Drools controller REST API to interact with your KIE Server templates (configurations), KIE Server instances (remote servers), and associated KIE containers (deployment units) in Drools without using the Business Central user interface. This API support enables you to maintain your Drools servers and resources more efficiently and optimize your integration and development with Drools.
With the Drools controller Java client API, you can perform the following actions also supported by the Drools controller REST API:
-
Retrieve information about KIE Server templates, instances, and associated KIE containers
-
Update, start, or stop KIE containers associated with KIE Server templates and instances
-
Create, update, or delete KIE Server templates
-
Create, update, or delete KIE Server instances
Drools controller Java client API requests require the following components:
- Authentication
-
The Drools controller Java client API requires HTTP Basic authentication for the following user roles, depending on controller type:
-
rest-all
user role if you installed Business Central and you want to use the built-in Drools controller -
kie-server
user role if you installed the headless Drools controller separately from Business Central
To view configured user roles for your Drools distribution, navigate to
~/$SERVER_HOME/standalone/configuration/application-roles.properties
and~/application-users.properties
.To add a user with the
kie-server
role or therest-all
role or both, navigate to~/$SERVER_HOME/bin
and run the following command with the role or roles specified:$ ./add-user.sh -a --user <USERNAME> --password <PASSWORD> --role kie-server,rest-all
To configure the
kie-server
orrest-all
user with Drools controller access, navigate to~/$SERVER_HOME/standalone/configuration/standalone-full.xml
, uncomment theorg.kie.server
properties (if applicable), and add the controller user login credentials and controller location (if needed):<property name="org.kie.server.location" value="http://localhost:8080/kie-server/services/rest/server"/> <property name="org.kie.server.controller" value="http://localhost:8080/business-central/rest/controller"/> <property name="org.kie.server.controller.user" value="baAdmin"/> <property name="org.kie.server.controller.pwd" value="password@1"/> <property name="org.kie.server.id" value="default-kieserver"/>
For more information about user roles and Drools installation options, see Installing the KIE Server.
-
- Project dependencies
-
The Drools controller Java client API requires the following dependencies on the relevant classpath of your Java project:
<!-- For remote execution on controller --> <dependency> <groupId>org.kie.server</groupId> <artifactId>kie-server-controller-client</artifactId> <version>${drools.version}</version> </dependency> <!-- For REST client --> <dependency> <groupId>org.jboss.resteasy</groupId> <artifactId>resteasy-client</artifactId> <version>${resteasy.version}</version> </dependency> <!-- For WebSocket client --> <dependency> <groupId>io.undertow</groupId> <artifactId>undertow-websockets-jsr</artifactId> <version>${undertow.version}</version> </dependency> <!-- For debug logging (optional) --> <dependency> <groupId>ch.qos.logback</groupId> <artifactId>logback-classic</artifactId> <version>${logback.version}</version> </dependency>
The
<version>
for Drools dependencies is the Maven artifact version for Drools currently used in your project (for example, 7.23.0.Final-redhat-00002). - Client request configuration
-
All Java client requests with the Drools controller Java client API must define at least the following controller communication components:
-
Credentials of the
rest-all
user if you installed Business Central, or thekie-server
user if you installed the headless Drools controller separately from Business Central -
Drools controller location for REST or WebSocket protocol:
-
Example REST URL:
http://localhost:8080/business-central/rest/controller
-
Example WebSocket URL:
ws://localhost:8080/headless-controller/websocket/controller
-
-
Marshalling format for API requests and responses (JSON or JAXB)
-
A
KieServerControllerClient
object, which serves as the entry point for starting the server communication using the Java client API -
A
KieServerControllerClientFactory
defining REST or WebSocket protocol and user access -
The Drools controller client service or services used, such as
listServerTemplates
,getServerTemplate
, orgetServerInstances
The following are examples of REST and WebSocket client configurations with these components:
Client configuration example with RESTimport org.kie.server.api.marshalling.MarshallingFormat; import org.kie.server.controller.api.model.spec.ServerTemplateList; import org.kie.server.controller.client.KieServerControllerClient; import org.kie.server.controller.client.KieServerControllerClientFactory; public class ListServerTemplatesExample { private static final String URL = "http://localhost:8080/business-central/rest/controller"; private static final String USER = "baAdmin"; private static final String PASSWORD = "password@1"; private static final MarshallingFormat FORMAT = MarshallingFormat.JSON; public static void main(String[] args) { KieServerControllerClient client = KieServerControllerClientFactory.newRestClient(URL, USER, PASSWORD); final ServerTemplateList serverTemplateList = client.listServerTemplates(); System.out.println(String.format("Found %s server template(s) at controller url: %s", serverTemplateList.getServerTemplates().length, URL)); } }
Client configuration example with WebSocketimport org.kie.server.api.marshalling.MarshallingFormat; import org.kie.server.controller.api.model.spec.ServerTemplateList; import org.kie.server.controller.client.KieServerControllerClient; import org.kie.server.controller.client.KieServerControllerClientFactory; public class ListServerTemplatesExample { private static final String URL = "ws://localhost:8080/my-controller/websocket/controller"; private static final String USER = "baAdmin"; private static final String PASSWORD = "password@1"; private static final MarshallingFormat FORMAT = MarshallingFormat.JSON; public static void main(String[] args) { KieServerControllerClient client = KieServerControllerClientFactory.newWebSocketClient(URL, USER, PASSWORD); final ServerTemplateList serverTemplateList = client.listServerTemplates(); System.out.println(String.format("Found %s server template(s) at controller url: %s", serverTemplateList.getServerTemplates().length, URL)); } }
-
22.11.1. Sending requests with the Drools controller Java client API
The Drools controller Java client API enables you to connect to the Drools controller using REST or WebSocket protocols from your Java client application. You can use the Drools controller Java client API as an alternative to the Drools controller REST API to interact with your KIE Server templates (configurations), KIE Server instances (remote servers), and associated KIE containers (deployment units) in Drools without using the Business Central user interface.
-
KIE Server is installed and running.
-
The Drools controller or headless Drools controller is installed and running.
-
You have
rest-all
user role access to the Drools controller if you installed Business Central, orkie-server
user role access to the headless Drools controller installed separately from Business Central. -
You have a Java project with Drools resources.
-
In your client application, ensure that the following dependencies have been added to the relevant classpath of your Java project:
<!-- For remote execution on controller --> <dependency> <groupId>org.kie.server</groupId> <artifactId>kie-server-controller-client</artifactId> <version>${drools.version}</version> </dependency> <!-- For REST client --> <dependency> <groupId>org.jboss.resteasy</groupId> <artifactId>resteasy-client</artifactId> <version>${resteasy.version}</version> </dependency> <!-- For WebSocket client --> <dependency> <groupId>io.undertow</groupId> <artifactId>undertow-websockets-jsr</artifactId> <version>${undertow.version}</version> </dependency> <!-- For debug logging (optional) --> <dependency> <groupId>ch.qos.logback</groupId> <artifactId>logback-classic</artifactId> <version>${logback.version}</version> </dependency>
-
In the
~/kie/server/controller/client
folder of the Java client API in GitHub , identify the relevant Java client implementation for the request you want to send, such as theRestKieServerControllerClient
implementation to access client services for KIE Server templates and KIE containers in REST protocol. -
In your client application, create a
.java
class for the API request. The class must contain the necessary imports, the Drools controller location and user credentials, aKieServerControllerClient
object, and the client method to execute, such ascreateServerTemplate
andcreateContainer
from theRestKieServerControllerClient
implementation. Adjust any configuration details according to your use case.Creating and interacting with a KIE Server template and KIE containersimport java.util.Arrays; import java.util.HashMap; import java.util.Map; import org.kie.server.api.marshalling.MarshallingFormat; import org.kie.server.api.model.KieContainerStatus; import org.kie.server.api.model.KieScannerStatus; import org.kie.server.api.model.ReleaseId; import org.kie.server.controller.api.model.spec.*; import org.kie.server.controller.client.KieServerControllerClient; import org.kie.server.controller.client.KieServerControllerClientFactory; public class RestTemplateContainerExample { private static final String URL = "http://localhost:8080/business-central/rest/controller"; private static final String USER = "baAdmin"; private static final String PASSWORD = "password@1"; private static KieServerControllerClient client; public static void main(String[] args) { KieServerControllerClient client = KieServerControllerClientFactory.newRestClient(URL, USER, PASSWORD, MarshallingFormat.JSON); // Create server template and KIE container, start and stop KIE container, and delete server template ServerTemplate serverTemplate = createServerTemplate(); ContainerSpec container = createContainer(serverTemplate); client.startContainer(container); client.stopContainer(container); client.deleteServerTemplate(serverTemplate.getId()); } // Re-create and configure server template protected static ServerTemplate createServerTemplate() { ServerTemplate serverTemplate = new ServerTemplate(); serverTemplate.setId("example-client-id"); serverTemplate.setName("example-client-name"); serverTemplate.setCapabilities(Arrays.asList(Capability.PROCESS.name(), Capability.RULE.name(), Capability.PLANNING.name())); client.saveServerTemplate(serverTemplate); return serverTemplate; } // Re-create and configure KIE containers protected static ContainerSpec createContainer(ServerTemplate serverTemplate){ Map<Capability, ContainerConfig> containerConfigMap = new HashMap(); ProcessConfig processConfig = new ProcessConfig("PER_PROCESS_INSTANCE", "kieBase", "kieSession", "MERGE_COLLECTION"); containerConfigMap.put(Capability.PROCESS, processConfig); RuleConfig ruleConfig = new RuleConfig(500l, KieScannerStatus.SCANNING); containerConfigMap.put(Capability.RULE, ruleConfig); ReleaseId releaseId = new ReleaseId("org.kie.server.testing", "stateless-session-kjar", "1.0.0-SNAPSHOT"); ContainerSpec containerSpec = new ContainerSpec("example-container-id", "example-client-name", serverTemplate, releaseId, KieContainerStatus.STOPPED, containerConfigMap); client.saveContainerSpec(serverTemplate.getId(), containerSpec); return containerSpec; } }
-
Run the configured
.java
class from your project directory to execute the request, and review the Drools controller response.If you enabled debug logging, KIE Server responds with a detailed response according to your configured marshalling format, such as JSON. If you encounter request errors, review the returned error code messages and adjust your Java configurations accordingly.
22.11.2. Supported Drools controller Java clients
The following are some of the Java client services available in the org.kie.server.controller.client
package of your Drools distribution. You can use these services to interact with related resources in the Drools controller similarly to the Drools controller REST API.
-
KieServerControllerClient
: Used as the entry point for communicating with the Drools controller -
RestKieServerControllerClient
: Implementation used to interact with KIE Server templates and KIE containers in REST protocol (found in~/org/kie/server/controller/client/rest
) -
WebSocketKieServerControllerClient
: Implementation used to interact with KIE Server templates and KIE containers in WebSocket protocol (found in~/org/kie/server/controller/client/websocket
)
For the full list of available Drools controller Java clients, see the Java client API source in GitHub.
22.11.3. Example requests with the Drools controller Java client API
The following are examples of Drools controller Java client API requests for basic interactions with the Drools controller. For the full list of available Drools controller Java clients, see the Java client API source in GitHub.
- Creating and interacting with KIE Server templates and KIE containers
-
You can use the
ServerTemplate
andContainerSpec
services in the REST or WebSocket Drools controller clients to create, dispose, and update KIE Server templates and KIE containers, and to start and stop KIE containers, as illustrated in this example.Example request to create and interact with a KIE Server template and KIE containersimport java.util.Arrays; import java.util.HashMap; import java.util.Map; import org.kie.server.api.marshalling.MarshallingFormat; import org.kie.server.api.model.KieContainerStatus; import org.kie.server.api.model.KieScannerStatus; import org.kie.server.api.model.ReleaseId; import org.kie.server.controller.api.model.spec.*; import org.kie.server.controller.client.KieServerControllerClient; import org.kie.server.controller.client.KieServerControllerClientFactory; public class RestTemplateContainerExample { private static final String URL = "http://localhost:8080/business-central/rest/controller"; private static final String USER = "baAdmin"; private static final String PASSWORD = "password@1"; private static KieServerControllerClient client; public static void main(String[] args) { KieServerControllerClient client = KieServerControllerClientFactory.newRestClient(URL, USER, PASSWORD, MarshallingFormat.JSON); // Create server template and KIE container, start and stop KIE container, and delete server template ServerTemplate serverTemplate = createServerTemplate(); ContainerSpec container = createContainer(serverTemplate); client.startContainer(container); client.stopContainer(container); client.deleteServerTemplate(serverTemplate.getId()); } // Re-create and configure server template protected static ServerTemplate createServerTemplate() { ServerTemplate serverTemplate = new ServerTemplate(); serverTemplate.setId("example-client-id"); serverTemplate.setName("example-client-name"); serverTemplate.setCapabilities(Arrays.asList(Capability.PROCESS.name(), Capability.RULE.name(), Capability.PLANNING.name())); client.saveServerTemplate(serverTemplate); return serverTemplate; } // Re-create and configure KIE containers protected static ContainerSpec createContainer(ServerTemplate serverTemplate){ Map<Capability, ContainerConfig> containerConfigMap = new HashMap(); ProcessConfig processConfig = new ProcessConfig("PER_PROCESS_INSTANCE", "kieBase", "kieSession", "MERGE_COLLECTION"); containerConfigMap.put(Capability.PROCESS, processConfig); RuleConfig ruleConfig = new RuleConfig(500l, KieScannerStatus.SCANNING); containerConfigMap.put(Capability.RULE, ruleConfig); ReleaseId releaseId = new ReleaseId("org.kie.server.testing", "stateless-session-kjar", "1.0.0-SNAPSHOT"); ContainerSpec containerSpec = new ContainerSpec("example-container-id", "example-client-name", serverTemplate, releaseId, KieContainerStatus.STOPPED, containerConfigMap); client.saveContainerSpec(serverTemplate.getId(), containerSpec); return containerSpec; } }
- Listing KIE Server templates and specifying connection timeout (REST)
-
When you use REST protocol for Drools controller Java client API requests, you can provide your own
javax.ws.rs.core.Configuration
specification to modify the underlying REST client API, such as connection timeout.Example REST request to return server templates and specify connection timeoutimport java.util.concurrent.TimeUnit; import javax.ws.rs.core.Configuration; import org.jboss.resteasy.client.jaxrs.ResteasyClientBuilder; import org.kie.server.api.marshalling.MarshallingFormat; import org.kie.server.controller.api.model.spec.ServerTemplateList; import org.kie.server.controller.client.KieServerControllerClient; import org.kie.server.controller.client.KieServerControllerClientFactory; public class RESTTimeoutExample { private static final String URL = "http://localhost:8080/business-central/rest/controller"; private static final String USER = "baAdmin"; private static final String PASSWORD = "password@1"; public static void main(String[] args) { // Specify connection timeout final Configuration configuration = new ResteasyClientBuilder() .establishConnectionTimeout(10, TimeUnit.SECONDS) .socketTimeout(60, TimeUnit.SECONDS) .getConfiguration(); KieServerControllerClient client = KieServerControllerClientFactory.newRestClient(URL, USER, PASSWORD, MarshallingFormat.JSON, configuration); // Retrieve list of server templates final ServerTemplateList serverTemplateList = client.listServerTemplates(); System.out.println(String.format("Found %s server template(s) at controller url: %s", serverTemplateList.getServerTemplates().length, URL)); } }
- Listing KIE Server templates and specifying event notifications (WebSocket)
-
When you use WebSocket protocol for Drools controller Java client API requests, you can enable event notifications based on changes that happen in the particular Drools controller to which the client API is connected. For example, you can receive notifications when KIE Server templates or instances are connected to or updated in the Drools controller.
Example WebSocket request to return server templates and specify event notificationsimport org.kie.server.api.marshalling.MarshallingFormat; import org.kie.server.controller.api.model.events.*; import org.kie.server.controller.api.model.spec.ServerTemplateList; import org.kie.server.controller.client.KieServerControllerClient; import org.kie.server.controller.client.KieServerControllerClientFactory; import org.kie.server.controller.client.event.EventHandler; public class WebSocketEventsExample { private static final String URL = "ws://localhost:8080/my-controller/websocket/controller"; private static final String USER = "baAdmin"; private static final String PASSWORD = "password@1"; public static void main(String[] args) { KieServerControllerClient client = KieServerControllerClientFactory.newWebSocketClient(URL, USER, PASSWORD, MarshallingFormat.JSON, new TestEventHandler()); // Retrieve list of server templates final ServerTemplateList serverTemplateList = client.listServerTemplates(); System.out.println(String.format("Found %s server template(s) at controller url: %s", serverTemplateList.getServerTemplates().length, URL)); try { Thread.sleep(60 * 1000); } catch (Exception e) { e.printStackTrace(); } } // Set up event notifications static class TestEventHandler implements EventHandler { @Override public void onServerInstanceConnected(ServerInstanceConnected serverInstanceConnected) { System.out.println("serverInstanceConnected = " + serverInstanceConnected); } @Override public void onServerInstanceDeleted(ServerInstanceDeleted serverInstanceDeleted) { System.out.println("serverInstanceDeleted = " + serverInstanceDeleted); } @Override public void onServerInstanceDisconnected(ServerInstanceDisconnected serverInstanceDisconnected) { System.out.println("serverInstanceDisconnected = " + serverInstanceDisconnected); } @Override public void onServerTemplateDeleted(ServerTemplateDeleted serverTemplateDeleted) { System.out.println("serverTemplateDeleted = " + serverTemplateDeleted); } @Override public void onServerTemplateUpdated(ServerTemplateUpdated serverTemplateUpdated) { System.out.println("serverTemplateUpdated = " + serverTemplateUpdated); } @Override public void onServerInstanceUpdated(ServerInstanceUpdated serverInstanceUpdated) { System.out.println("serverInstanceUpdated = " + serverInstanceUpdated); } @Override public void onContainerSpecUpdated(ContainerSpecUpdated containerSpecUpdated) { System.out.println("onContainerSpecUpdated = " + containerSpecUpdated); } } }
22.12. Securing password using key store
KIE server is using for some communication (e.g. REST api) basic authentication with passwords. From the security perspective it is not safe to store such passwords in clear text form on the disc. For this purpose a mechanism was developed to store passwords in a key store and then use it in the application.
22.12.1. Simple usecase
User wants to secure his password for communicating via REST client. He creates new keystore where he will put his password, he will setup sytem variables with the info to the keystore and KIE will automatically load the keystore and will use the password for securing the communication.
22.12.2. Implementation and business logic
Current implementation is using key store if it is defined. If not, the funcionality is falling back to old behavior using config parameters.
22.12.3. System requirements
To use a key store we need to create it first. As JKS is not supporting symetric keys we have to create JCEKS key store. Moreover, password can be stored in a key store only for Java 8 and above. For generating a key store you can use standard tool KeyTool which is part of JDK instalation.
22.12.4. Initialization of a key store
For keystore initialization we recommend to use keytool. Syntax is the following:
$ keytool -importpassword -keystore _keystore_url_ -keypass _alias_key_password_ -alias _password_alias_ -storepass _keystore_password_ -storetype JCEKS
-
alias - alias name of the entry to process
-
keypass - key password
-
keystore - keystore name
-
storepass - keystore password
-
storetype - keystore type
After running this command user will be asked for entering the password which he wants to store.
22.12.5. System parameters for loading key store
-
kie.keystore.keyStoreURL - URL to a keystore which should be used
-
kie.keystore.keyStorePwd - password to a keystore
-
kie.keystore.key.server.alias - alias of the key for REST services where password is stored
-
kie.keystore.key.server.pwd - password of an alias for REST services with stored password
-
kie.keystore.key.ctrl.alias - alias of the key for default REST Drools controller where password is stored
-
kie.keystore.key.ctrl.pwd - password of an alias for default REST Drools controller with stored password
22.12.6. Example
-
create user and password in application server (it has to have kie-server role)
${EAP_HOME}/add-user.sh -a -e -u kieserver -p "kiePassword1!" -g kie-server
-
use key tool to create keystore with password in it
$ keytool -importpassword -keystore /home/kie/keystores/droolsServer.jceks -keypass keypwd -alias droolsKey -storepass serverpwd -storetype JCEKS
Enter the password to be stored:
Re-enter password:
$ keytool -importpassword -keystore /home/kie/keystores/droolsServer.jceks -keypass keypwd -alias restKey -storepass serverpwd -storetype JCEKS
Enter the password to be stored:
Re-enter password:
-
set following system properties on application server that will let the KIE Server or Drools controller to read password from keystore
<system-properties> <property name="kie.keystore.keyStoreURL" value="droolsServer.jceks"/> <property name="kie.keystore.keyStorePwd" value="serverpwd"/> <property name="kie.keystore.key.server.alias" value="restKey"/> <property name="kie.keystore.key.server.pwd" value="keypwd"/> <property name="kie.keystore.key.ctrl.alias" value="droolsKey"/> <property name="kie.keystore.key.ctrl.pwd" value="keypwd"/> </system-properties>
-
start server to verify configuration
22.13. Prometheus metrics monitoring in Drools
Prometheus is an open-source systems monitoring toolkit that you can use with Drools to collect and store metrics related to the execution of business rules, processes, Decision Model and Notation (DMN) models, and other Drools assets. You can access the stored metrics through a REST API call to the KIE Server, through the Prometheus expression browser, or using a data-graphing tool such as Grafana.
You can configure Prometheus metrics monitoring for an on-premise KIE Server instance or for your KIE Server deployment on Red Hat OpenShift Container Platform.
For the list of available metrics that KIE Server exposes with Prometheus, see the KIE Server Prometheus Extension page on GitHub.
22.13.1. Configuring Prometheus metrics monitoring for KIE Server
You can configure your KIE Server instances to use Prometheus to collect and store metrics related to your business asset activity in Drools. For the list of available metrics that KIE Server exposes with Prometheus, see the KIE Server Prometheus Extension page on GitHub.
-
KIE Server is installed.
-
You have
kie-server
user role access to KIE Server. -
Prometheus is installed. For information about downloading and using Prometheus, see the Prometheus documentation page.
-
In your KIE Server instance, set the
org.kie.prometheus.server.ext.disabled
system property tofalse
to enable the Prometheus extension. You can define this property when you start KIE Server or in thestandalone.xml
orstandalone-full.xml
file of Drools distribution. -
If you are running Drools on Spring Boot, add the following dependencies in the
pom.xml
file of your Maven project and configure the required key in theapplication.properties
system property:Spring Boot pom.xml dependencies for Prometheus<dependency> <groupId>org.kie.server</groupId> <artifactId>kie-server-services-prometheus</artifactId> <version>${drools.version}</version> </dependency> <dependency> <groupId>org.kie.server</groupId> <artifactId>kie-server-rest-prometheus</artifactId> <version>${drools.version}</version> </dependency>
Spring Boot application.properties key for Drools and Prometheuskieserver.drools.enabled=true kieserver.dmn.enabled=true kieserver.prometheus.enabled=true
-
In the
prometheus.yaml
file of your Prometheus distribution, add the following settings in thescrape_configs
section to configure Prometheus to scrape metrics from KIE Server:Scrape configurations in prometheus.yaml filescrape_configs: job_name: 'kie-server' metrics_path: /SERVER_PATH/services/rest/metrics Basic_auth: username: USER_NAME password: PASSWORD static_configs: - targets: ["HOST:PORT"]
Scrape configurations in prometheus.yaml file for Spring Boot (if applicable)scrape_configs: job_name: 'kie' metrics_path: /rest/metrics static_configs: - targets: ["HOST:PORT"]
Replace the values according to your KIE Server location and settings.
-
Start the KIE Server instance.
After you start the configured KIE Server instance, Prometheus begins collecting metrics and KIE Server publishes the metrics to the REST API endpoint
http://HOST:PORT/SERVER/services/rest/metrics
(or on Spring Boot, tohttp://HOST:PORT/rest/metrics
). -
In a REST client or curl utility, send a REST API request with the following components to verify that KIE Server is publishing the metrics:
For REST client:
-
Authentication: Enter the user name and password of the KIE Server user with the
kie-server
role. -
HTTP Headers: Set the following header:
-
Accept
:application/json
-
-
HTTP method: Set to
GET
. -
URL: Enter the KIE Server REST API base URL and metrics endpoint, such as
http://localhost:8080/kie-server/services/rest/metrics
(or on Spring Boot,http://localhost:8080/rest/metrics
).
For curl utility:
-
-u
: Enter the user name and password of the KIE Server user with thekie-server
role. -
-H
: Set the following header:-
accept
:application/json
-
-
-X
: Set toGET
. -
URL: Enter the KIE Server REST API base URL and metrics endpoint, such as
http://localhost:8080/kie-server/services/rest/metrics
(or on Spring Boot,http://localhost:8080/rest/metrics
).
curl -u 'baAdmin:password@1' -H "accept: application/json" -X GET "http://localhost:8080/kie-server/services/rest/metrics"
Example server response# HELP kie_server_container_started_total Kie Server Started Containers # TYPE kie_server_container_started_total counter kie_server_container_started_total{container_id="task-assignment-kjar-1.0",} 1.0 # HELP solvers_running Number of solvers currently running # TYPE solvers_running gauge solvers_running 0.0 # HELP dmn_evaluate_decision_nanosecond DMN Evaluation Time # TYPE dmn_evaluate_decision_nanosecond histogram # HELP solver_duration_seconds Time in seconds it took solver to solve the constraint problem # TYPE solver_duration_seconds summary solver_duration_seconds_count{solver_id="100tasks-5employees.xml",} 1.0 solver_duration_seconds_sum{solver_id="100tasks-5employees.xml",} 179.828255925 solver_duration_seconds_count{solver_id="24tasks-8employees.xml",} 1.0 solver_duration_seconds_sum{solver_id="24tasks-8employees.xml",} 179.995759653 # HELP drl_match_fired_nanosecond Drools Firing Time # TYPE drl_match_fired_nanosecond histogram # HELP dmn_evaluate_failed_count DMN Evaluation Failed # TYPE dmn_evaluate_failed_count counter # HELP kie_server_start_time Kie Server Start Time # TYPE kie_server_start_time gauge kie_server_start_time{name="myapp-kieserver",server_id="myapp-kieserver",location="http://myapp-kieserver-demo-monitoring.127.0.0.1.nip.io:80/services/rest/server",version="7.4.0.redhat-20190428",} 1.557221271502E12 # HELP kie_server_container_running_total Kie Server Running Containers # TYPE kie_server_container_running_total gauge kie_server_container_running_total{container_id="task-assignment-kjar-1.0",} 1.0 # HELP solver_score_calculation_speed Number of moves per second for a particular solver solving the constraint problem # TYPE solver_score_calculation_speed summary solver_score_calculation_speed_count{solver_id="100tasks-5employees.xml",} 1.0 solver_score_calculation_speed_sum{solver_id="100tasks-5employees.xml",} 6997.0 solver_score_calculation_speed_count{solver_id="24tasks-8employees.xml",} 1.0 solver_score_calculation_speed_sum{solver_id="24tasks-8employees.xml",} 19772.0
If the metrics are not available in KIE Server, review and verify the KIE Server and Prometheus configurations described in this section.
You can also interact with your collected metrics in the Prometheus expression browser at
http://HOST:PORT/graph
, or integrate your Prometheus data source with a data-graphing tool such as Grafana:Figure 340. Prometheus expression browser with KIE Server metricsFigure 341. Prometheus expression browser with KIE Server targetFigure 342. Grafana dashboard with KIE Server metrics for DMN modelsFigure 343. Grafana dashboard with KIE Server metrics for solvers -
Drools Examples
Examples to help you learn Drools
23. Examples
23.1. Example decisions in Drools for an IDE
Drools provides example decisions distributed as Java classes that you can import into your integrated development environment (IDE). You can use these examples to better understand Drools engine capabilities or use them as a reference for the decisions that you define in your own Drools projects.
The following example decision sets are some of the examples available in Drools:
-
Hello World example: Demonstrates basic rule execution and use of debug output
-
State example: Demonstrates forward chaining and conflict resolution through rule salience and agenda groups
-
Fibonacci example: Demonstrates recursion and conflict resolution through rule salience
-
Banking example: Demonstrates pattern matching, basic sorting, and calculation
-
Pet Store example: Demonstrates rule agenda groups, global variables, callbacks, and GUI integration
-
Sudoku example: Demonstrates complex pattern matching, problem solving, callbacks, and GUI integration
-
House of Doom example: Demonstrates backward chaining and recursion
For optimization examples provided with OptaPlanner, see the OptaPlanner User Guide. |
23.2. Importing and executing Drools example decisions in an IDE
You can import Drools example decisions into your integrated development environment (IDE) and execute them to explore how the rules and code function. You can use these examples to better understand Drools engine capabilities or use them as a reference for the decisions that you define in your own Drools projects.
-
Java 8 or later is installed.
-
Maven 3.5.x or later is installed.
-
An IDE is installed, such as Eclipse with the JBoss Tools plugin.
-
Download and unzip the source from the Drools repository in GitHub.
For the Conway’s Game of Life example decision, also download and unzip the source from the Drools and jBPM integration repository in GitHub.
-
Open your IDE and select File → Import → Maven → Existing Maven Projects, or the equivalent option for importing a Maven project.
-
Click Browse, navigate to
~/drools-master/drools-examples
(or, for the Conway’s Game of Life example,~/droolsjbpm-integration-examples
), and import the project. -
Navigate to the example package that you want to run and find the Java class with the
main
method. -
Right-click the Java class and select Run As → Java Application to run the example.
To run all examples through a basic user interface, run the
DroolsExamplesApp.java
class (or, for Conway’s Game of Life, theDroolsJbpmIntegrationExamplesApp.java
class) in theorg.drools.examples
main class.Figure 344. Interface for all examples in drools-examples (DroolsExamplesApp.java)Figure 345. Interface for all examples in droolsjbpm-integration-examples (DroolsJbpmIntegrationExamplesApp.java)
23.3. Hello World example decisions (basic rules and debugging)
The Hello World example decision set demonstrates how to insert objects into the Drools engine working memory, how to match the objects using rules, and how to configure logging to trace the internal activity of the Drools engine.
The following is an overview of the Hello World example:
-
Name:
helloworld
-
Main class:
org.drools.examples.helloworld.HelloWorldExample
(insrc/main/java
) -
Module:
drools-examples
-
Type: Java application
-
Rule file:
org.drools.examples.helloworld.HelloWorld.drl
(insrc/main/resources
) -
Objective: Demonstrates basic rule execution and use of debug output
In the Hello World example, a KIE session is generated to enable rule execution. All rules require a KIE session for execution.
KieServices ks = KieServices.Factory.get(); (1)
KieContainer kc = ks.getKieClasspathContainer(); (2)
KieSession ksession = kc.newKieSession("HelloWorldKS"); (3)
1 | Obtains the KieServices factory. This is the main interface that applications use to interact with the Drools engine. |
2 | Creates a KieContainer from the project class path. This detects a /META-INF/kmodule.xml file from which it configures and instantiates a KieContainer with a KieModule . |
3 | Creates a KieSession based on the "HelloWorldKS" KIE session configuration defined in the /META-INF/kmodule.xml file. |
For more information about Drools project packaging, see Build, Deploy, Utilize and Run. |
Drools has an event model that exposes internal engine activity. Two default debug listeners, DebugAgendaEventListener
and DebugWorkingMemoryEventListener
, print debug event information to the System.err
output. The KieRuntimeLogger
provides execution auditing, the result of which you can view in a graphical viewer.
// Set up listeners.
ksession.addEventListener( new DebugAgendaEventListener() );
ksession.addEventListener( new DebugRuleRuntimeEventListener() );
// Set up a file-based audit logger.
KieRuntimeLogger logger = KieServices.get().getLoggers().newFileLogger( ksession, "./target/helloworld" );
// Set up a ThreadedFileLogger so that the audit view reflects events while debugging.
KieRuntimeLogger logger = ks.getLoggers().newThreadedFileLogger( ksession, "./target/helloworld", 1000 );
The logger is a specialized implementation built on the Agenda
and RuleRuntime
listeners. When the Drools engine has finished executing, logger.close()
is called.
The example creates a single Message
object with the message "Hello World"
, inserts the status HELLO
into the KieSession
, executes rules with fireAllRules()
.
// Insert facts into the KIE session.
final Message message = new Message();
message.setMessage( "Hello World" );
message.setStatus( Message.HELLO );
ksession.insert( message );
// Fire the rules.
ksession.fireAllRules();
Rule execution uses a data model to pass data as inputs and outputs to the KieSession
. The data model in this example has two fields: the message
, which is a String
, and the status
, which can be HELLO
or GOODBYE
.
public static class Message {
public static final int HELLO = 0;
public static final int GOODBYE = 1;
private String message;
private int status;
...
}
The two rules are located in the file src/main/resources/org/drools/examples/helloworld/HelloWorld.drl
.
The when
condition of the "Hello World"
rule states that the rule is activated for each Message
object inserted into the KIE session that has the status Message.HELLO
. Additionally, two variable bindings are created: the variable message
is bound to the message
attribute and the variable m
is bound to the matched Message
object itself.
The then
action of the rule is written using the MVEL expression language, as declared by the rule dialect
attribute. After printing the content of the bound variable message
to System.out
, the rule changes the values of the message
and status
attributes of the Message
object bound to m
. The rule uses the MVEL modify
statement to apply a block of assignments in one statement and to notify the Drools engine of the changes at the end of the block.
rule "Hello World"
dialect "mvel"
when
m : Message( status == Message.HELLO, message : message )
then
System.out.println( message );
modify ( m ) { message = "Goodbye cruel world",
status = Message.GOODBYE };
end
The "Good Bye"
rule, which specifies the java
dialect, is similar to the "Hello World"
rule except that it matches Message
objects that have the status Message.GOODBYE
.
rule "Good Bye"
dialect "java"
when
Message( status == Message.GOODBYE, message : message )
then
System.out.println( message );
end
To execute the example, run the org.drools.examples.helloworld.HelloWorldExample
class as a Java application in your IDE. The rule writes to System.out
, the debug listener writes to System.err
, and the audit logger creates a log file in target/helloworld.log
.
Hello World
Goodbye cruel world
==>[ActivationCreated(0): rule=Hello World;
tuple=[fid:1:1:org.drools.examples.helloworld.HelloWorldExample$Message@17cec96]]
[ObjectInserted: handle=[fid:1:1:org.drools.examples.helloworld.HelloWorldExample$Message@17cec96];
object=org.drools.examples.helloworld.HelloWorldExample$Message@17cec96]
[BeforeActivationFired: rule=Hello World;
tuple=[fid:1:1:org.drools.examples.helloworld.HelloWorldExample$Message@17cec96]]
==>[ActivationCreated(4): rule=Good Bye;
tuple=[fid:1:2:org.drools.examples.helloworld.HelloWorldExample$Message@17cec96]]
[ObjectUpdated: handle=[fid:1:2:org.drools.examples.helloworld.HelloWorldExample$Message@17cec96];
old_object=org.drools.examples.helloworld.HelloWorldExample$Message@17cec96;
new_object=org.drools.examples.helloworld.HelloWorldExample$Message@17cec96]
[AfterActivationFired(0): rule=Hello World]
[BeforeActivationFired: rule=Good Bye;
tuple=[fid:1:2:org.drools.examples.helloworld.HelloWorldExample$Message@17cec96]]
[AfterActivationFired(4): rule=Good Bye]
To better understand the execution flow of this example, you can load the audit log file from target/helloworld.log
into your IDE debug view or Audit View, if available (for example, in Window → Show View in some IDEs).
In this example, the Audit view shows that the object is inserted, which creates an activation for the "Hello World"
rule. The activation is then executed, which updates the Message
object and causes the "Good Bye"
rule to activate. Finally, the "Good Bye"
rule is executed. When you select an event in the Audit View, the origin event, which is the "Activation created"
event in this example, is highlighted in green.
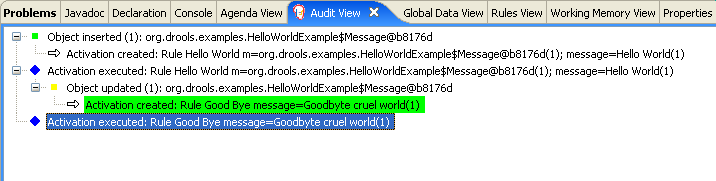
23.4. State example decisions (forward chaining and conflict resolution)
The State example decision set demonstrates how the Drools engine uses forward chaining and any changes to facts in the working memory to resolve execution conflicts for rules in a sequence. The example focuses on resolving conflicts through salience values or through agenda groups that you can define in rules.
The following is an overview of the State example:
-
Name:
state
-
Main classes:
org.drools.examples.state.StateExampleUsingSalience
,org.drools.examples.state.StateExampleUsingAgendaGroup
(insrc/main/java
) -
Module:
drools-examples
-
Type: Java application
-
Rule files:
org.drools.examples.state.*.drl
(insrc/main/resources
) -
Objective: Demonstrates forward chaining and conflict resolution through rule salience and agenda groups
A forward-chaining rule system is a data-driven system that starts with a fact in the working memory of the Drools engine and reacts to changes to that fact. When objects are inserted into working memory, any rule conditions that become true as a result of the change are scheduled for execution by the agenda.
In contrast, a backward-chaining rule system is a goal-driven system that starts with a conclusion that the Drools engine attempts to satisfy, often using recursion. If the system cannot reach the conclusion or goal, it searches for subgoals, which are conclusions that complete part of the current goal. The system continues this process until either the initial conclusion is satisfied or all subgoals are satisfied.
The Drools engine in Drools uses both forward and backward chaining to evaluate rules.
The following diagram illustrates how the Drools engine evaluates rules using forward chaining overall with a backward-chaining segment in the logic flow:
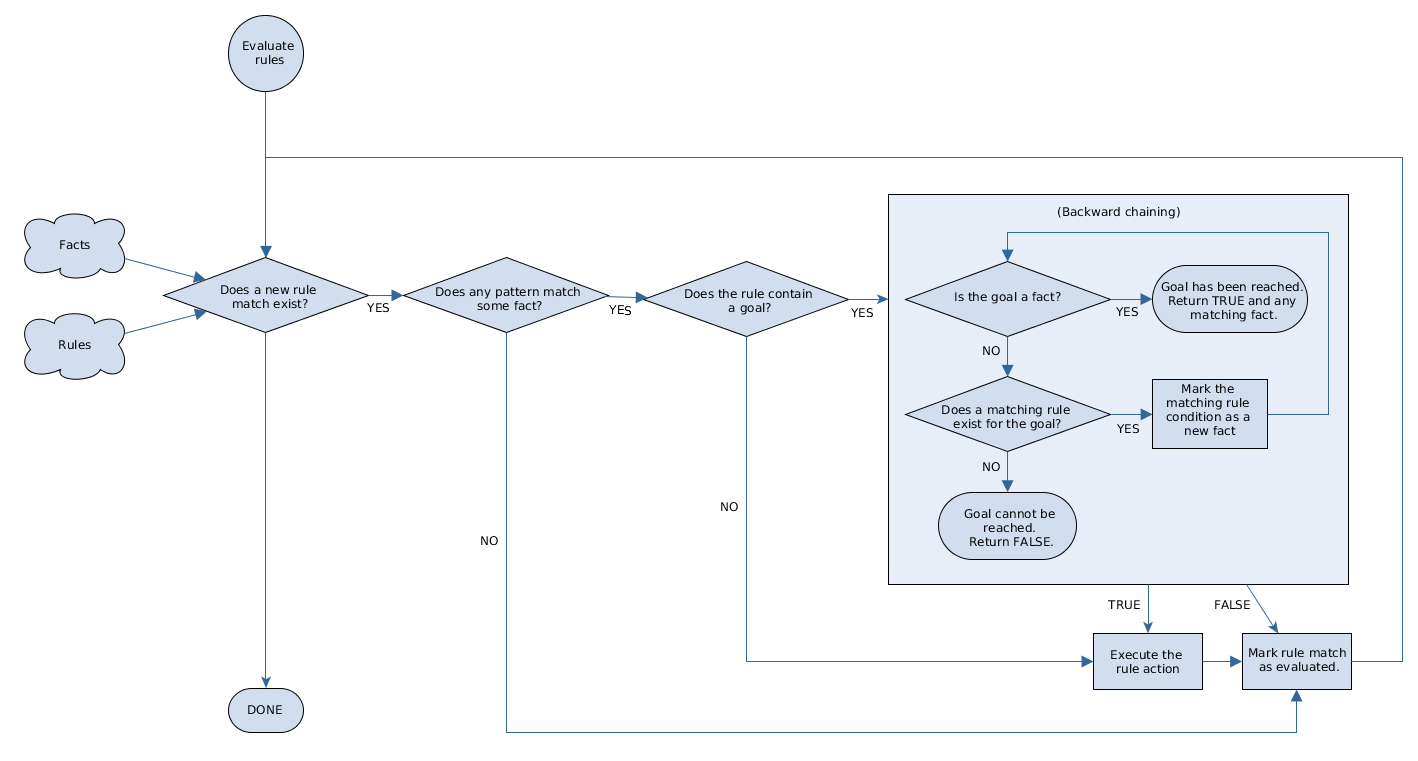
In the State example, each State
class has fields for its name and its current state (see the class org.drools.examples.state.State
). The following states are the two possible states for each object:
-
NOTRUN
-
FINISHED
public class State {
public static final int NOTRUN = 0;
public static final int FINISHED = 1;
private final PropertyChangeSupport changes =
new PropertyChangeSupport( this );
private String name;
private int state;
... setters and getters go here...
}
The State example contains two versions of the same example to resolve rule execution conflicts:
-
A
StateExampleUsingSalience
version that resolves conflicts by using rule salience -
A
StateExampleUsingAgendaGroups
version that resolves conflicts by using rule agenda groups
Both versions of the state example involve four State
objects: A
, B
, C
, and D
. Initially, their states are set to NOTRUN
, which is the default value for the constructor that the example uses.
State example using salience
The StateExampleUsingSalience
version of the State example uses salience values in rules to resolve rule execution conflicts. Rules with a higher salience value are given higher priority when ordered in the activation queue.
The example inserts each State
instance into the KIE session and then calls fireAllRules()
.
final State a = new State( "A" );
final State b = new State( "B" );
final State c = new State( "C" );
final State d = new State( "D" );
ksession.insert( a );
ksession.insert( b );
ksession.insert( c );
ksession.insert( d );
ksession.fireAllRules();
// Dispose KIE session if stateful (not required if stateless).
ksession.dispose();
To execute the example, run the org.drools.examples.state.StateExampleUsingSalience
class as a Java application in your IDE.
After the execution, the following output appears in the IDE console window:
A finished
B finished
C finished
D finished
Four rules are present.
First, the "Bootstrap"
rule fires, setting A
to state FINISHED
, which then causes B
to change its state to FINISHED
. Objects C
and D
are both dependent on B
, causing a conflict that is resolved by the salience values.
To better understand the execution flow of this example, you can load the audit log file from target/state.log
into your IDE debug view or Audit View, if available (for example, in Window → Show View in some IDEs).
In this example, the Audit View shows that the assertion of the object A
in the state NOTRUN
activates the "Bootstrap"
rule, while the assertions of the other objects have no immediate effect.
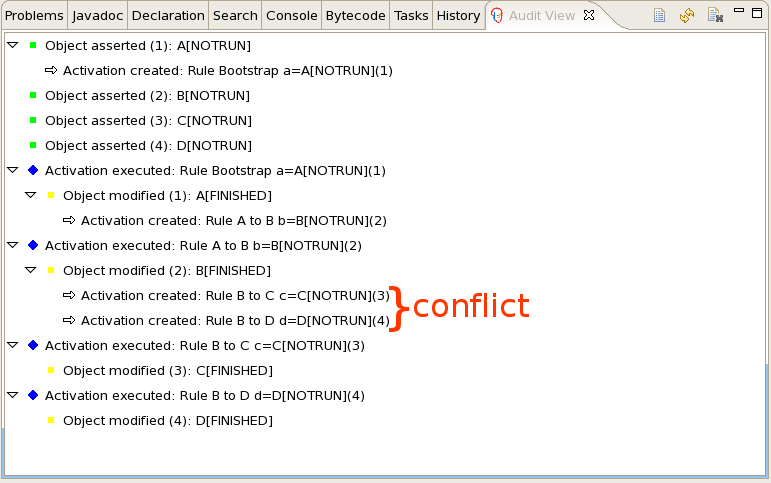
rule "Bootstrap"
when
a : State(name == "A", state == State.NOTRUN )
then
System.out.println(a.getName() + " finished" );
a.setState( State.FINISHED );
end
The execution of the "Bootstrap"
rule changes the state of A
to FINISHED
, which activates rule "A to B"
.
rule "A to B"
when
State(name == "A", state == State.FINISHED )
b : State(name == "B", state == State.NOTRUN )
then
System.out.println(b.getName() + " finished" );
b.setState( State.FINISHED );
end
The execution of rule "A to B"
changes the state of B
to FINISHED
, which activates both rules "B to C"
and "B to D"
, placing their activations onto the Drools engine agenda.
rule "B to C"
salience 10
when
State(name == "B", state == State.FINISHED )
c : State(name == "C", state == State.NOTRUN )
then
System.out.println(c.getName() + " finished" );
c.setState( State.FINISHED );
end
rule "B to D"
when
State(name == "B", state == State.FINISHED )
d : State(name == "D", state == State.NOTRUN )
then
System.out.println(d.getName() + " finished" );
d.setState( State.FINISHED );
end
From this point on, both rules may fire and, therefore, the rules are in conflict. The conflict resolution strategy enables the Drools engine agenda to decide which rule to fire. Rule "B to C"
has the higher salience value (10
versus the default salience value of 0
), so it fires first, modifying object C
to state FINISHED
.
The Audit View in your IDE shows the modification of the State
object in the rule "A to B"
, which results in two activations being in conflict.
You can also use the Agenda View in your IDE to investigate the state of the Drools engine agenda. In this example, the Agenda View shows the breakpoint in the rule "A to B"
and the state of the agenda with the two conflicting rules. Rule "B to D"
fires last, modifying object D
to state FINISHED
.
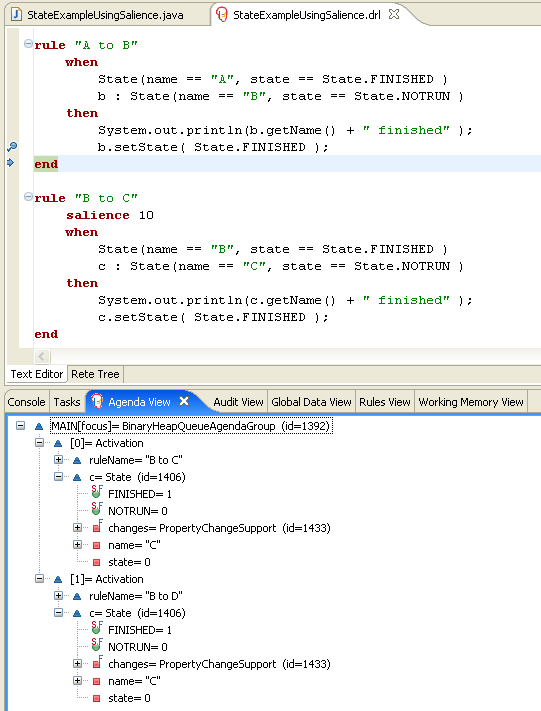
State example using agenda groups
The StateExampleUsingAgendaGroups
version of the State example uses agenda groups in rules to resolve rule execution conflicts. Agenda groups enable you to partition the Drools engine agenda to provide more execution control over groups of rules. By default, all rules are in the agenda group MAIN
. You can use the agenda-group
attribute to specify a different agenda group for the rule.
Initially, a working memory has its focus on the agenda group MAIN
. Rules in an agenda group only fire when the group receives the focus. You can set the focus either by using the method setFocus()
or the rule attribute auto-focus
. The auto-focus
attribute enables the rule to be given a focus automatically for its agenda group when the rule is matched and activated.
In this example, the auto-focus
attribute enables rule "B to C"
to fire before "B to D"
.
rule "B to C"
agenda-group "B to C"
auto-focus true
when
State(name == "B", state == State.FINISHED )
c : State(name == "C", state == State.NOTRUN )
then
System.out.println(c.getName() + " finished" );
c.setState( State.FINISHED );
kcontext.getKnowledgeRuntime().getAgenda().getAgendaGroup( "B to D" ).setFocus();
end
The rule "B to C"
calls setFocus()
on the agenda group "B to D"
, enabling its active rules to fire, which then enables the rule "B to D"
to fire.
rule "B to D"
agenda-group "B to D"
when
State(name == "B", state == State.FINISHED )
d : State(name == "D", state == State.NOTRUN )
then
System.out.println(d.getName() + " finished" );
d.setState( State.FINISHED );
end
To execute the example, run the org.drools.examples.state.StateExampleUsingAgendaGroups
class as a Java application in your IDE.
After the execution, the following output appears in the IDE console window (same as the salience version of the State example):
A finished
B finished
C finished
D finished
Dynamic facts in the State example
Another notable concept in this State example is the use of dynamic facts, based on objects that implement a PropertyChangeListener
object. In order for the Drools engine to see and react to changes of fact properties, the application must notify the Drools engine that changes occurred. You can configure this communication explicitly in the rules by using the modify
statement, or implicitly by specifying that the facts implement the PropertyChangeSupport
interface as defined by the JavaBeans specification.
This example demonstrates how to use the PropertyChangeSupport
interface to avoid the need for explicit modify
statements in the rules. To make use of this interface, ensure that your facts implement PropertyChangeSupport
in the same way that the class org.drools.example.State
implements it, and then use the following code in the DRL rule file to configure the Drools engine to listen for property changes on those facts:
declare type State
@propertyChangeSupport
end
When you use PropertyChangeListener
objects, each setter must implement additional code for the notification. For example, the following setter for state
is in the class org.drools.examples
:
public void setState(final int newState) {
int oldState = this.state;
this.state = newState;
this.changes.firePropertyChange( "state",
oldState,
newState );
}
23.5. Fibonacci example decisions (recursion and conflict resolution)
The Fibonacci example decision set demonstrates how the Drools engine uses recursion to resolve execution conflicts for rules in a sequence. The example focuses on resolving conflicts through salience values that you can define in rules.
The following is an overview of the Fibonacci example:
-
Name:
fibonacci
-
Main class:
org.drools.examples.fibonacci.FibonacciExample
(insrc/main/java
) -
Module:
drools-examples
-
Type: Java application
-
Rule file:
org.drools.examples.fibonacci.Fibonacci.drl
(insrc/main/resources
) -
Objective: Demonstrates recursion and conflict resolution through rule salience
The Fibonacci Numbers form a sequence starting with 0 and 1. The next Fibonacci number is obtained by adding the two preceding Fibonacci numbers: 0, 1, 1, 2, 3, 5, 8, 13, 21, 34, 55, 89, 144, 233, 377, 610, 987, 1597, 2584, 4181, 6765, 10946, and so on.
The Fibonacci example uses the single fact class Fibonacci
with the following two fields:
-
sequence
-
value
The sequence
field indicates the position of the object in the Fibonacci number sequence. The value
field shows the value of that Fibonacci object for that sequence position, where -1
indicates a value that still needs to be computed.
public static class Fibonacci {
private int sequence;
private long value;
public Fibonacci( final int sequence ) {
this.sequence = sequence;
this.value = -1;
}
... setters and getters go here...
}
To execute the example, run the org.drools.examples.fibonacci.FibonacciExample
class as a Java application in your IDE.
After the execution, the following output appears in the IDE console window:
recurse for 50
recurse for 49
recurse for 48
recurse for 47
...
recurse for 5
recurse for 4
recurse for 3
recurse for 2
1 == 1
2 == 1
3 == 2
4 == 3
5 == 5
6 == 8
...
47 == 2971215073
48 == 4807526976
49 == 7778742049
50 == 12586269025
To achieve this behavior in Java, the example inserts a single Fibonacci
object with a sequence field of 50
. The example then uses a recursive rule to insert the other 49 Fibonacci
objects.
Instead of implementing the PropertyChangeSupport
interface to use dynamic facts, this example uses the MVEL dialect modify
keyword to enable a block setter action and notify the Drools engine of changes.
ksession.insert( new Fibonacci( 50 ) );
ksession.fireAllRules();
This example uses the following three rules:
-
"Recurse"
-
"Bootstrap"
-
"Calculate"
The rule "Recurse"
matches each asserted Fibonacci
object with a value of -1
, creating and asserting a new Fibonacci
object with a sequence of one less than the currently matched object. Each time a Fibonacci object is added while the one with a sequence field equal to 1
does not exist, the rule re-matches and fires again. The not
conditional element is used to stop the rule matching once you have all 50 Fibonacci objects in memory. The rule also has a salience
value because you need to have all 50 Fibonacci
objects asserted before you execute the "Bootstrap"
rule.
rule "Recurse"
salience 10
when
f : Fibonacci ( value == -1 )
not ( Fibonacci ( sequence == 1 ) )
then
insert( new Fibonacci( f.sequence - 1 ) );
System.out.println( "recurse for " + f.sequence );
end
To better understand the execution flow of this example, you can load the audit log file from target/fibonacci.log
into your IDE debug view or Audit View, if available (for example, in Window → Show View in some IDEs).
In this example, the Audit View shows the original assertion of the Fibonacci
object with a sequence
field of 50
, done from Java code. From there on, the Audit View shows the continual recursion of the rule, where each asserted Fibonacci
object causes the "Recurse"
rule to become activated and to fire again.
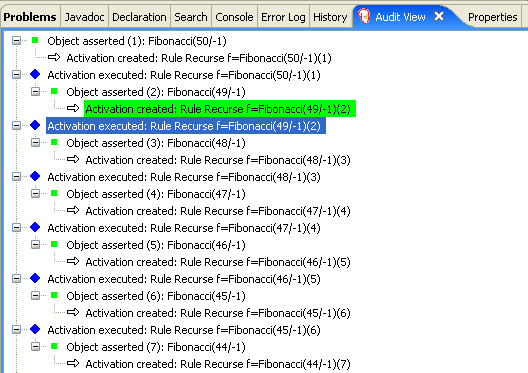
When a Fibonacci
object with a sequence
field of 2
is asserted, the "Bootstrap"
rule is matched and activated along with the "Recurse"
rule. Notice the multiple restrictions on field sequence
that test for equality with 1
or 2
:
rule "Bootstrap"
when
f : Fibonacci( sequence == 1 || == 2, value == -1 ) // multi-restriction
then
modify ( f ){ value = 1 };
System.out.println( f.sequence + " == " + f.value );
end
You can also use the Agenda View in your IDE to investigate the state of the Drools engine agenda. The "Bootstrap"
rule does not fire yet because the "Recurse"
rule has a higher salience value.
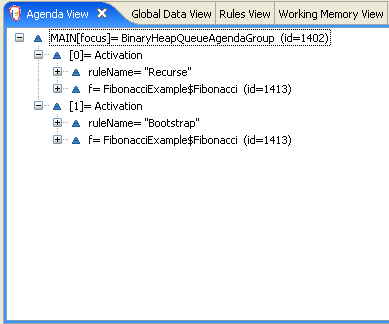
When a Fibonacci
object with a sequence
of 1
is asserted, the "Bootstrap"
rule is matched again, causing two activations for this rule. The "Recurse"
rule does not match and activate because the not
conditional element stops the rule matching as soon as a Fibonacci
object with a sequence
of 1
exists.
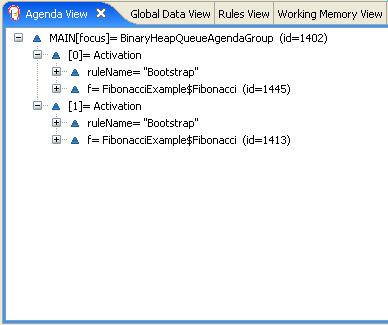
The "Bootstrap"
rule sets the objects with a sequence
of 1
and 2
to a value of 1
. Now that you have two Fibonacci
objects with values not equal to -1
, the "Calculate"
rule is able to match.
At this point in the example, nearly 50 Fibonacci
objects exist in the working memory. You need to select a suitable triple to calculate each of their values in turn. If you use three Fibonacci patterns in a rule without field constraints to confine the possible cross products, the result would be 50x49x48 possible combinations, leading to about 125,000 possible rule firings, most of them incorrect.
The "Calculate"
rule uses field constraints to evaluate the three Fibonacci patterns in the correct order. This technique is called cross-product matching.
The first pattern finds any Fibonacci
object with a value != -1
and binds both the pattern and the field. The second Fibonacci
object does the same thing, but adds an additional field constraint to ensure that its sequence is greater by one than the Fibonacci
object bound to f1
. When this rule fires for the first time, you know that only sequences 1
and 2
have values of 1
, and the two constraints ensure that f1
references sequence 1
and that f2
references sequence 2
.
The final pattern finds the Fibonacci
object with a value equal to -1
and with a sequence one greater than f2
.
At this point in the example, three Fibonacci
objects are correctly selected from the available cross products, and you can calculate the value for the third Fibonacci
object that is bound to f3
.
rule "Calculate"
when
// Bind f1 and s1.
f1 : Fibonacci( s1 : sequence, value != -1 )
// Bind f2 and v2, refer to bound variable s1.
f2 : Fibonacci( sequence == (s1 + 1), v2 : value != -1 )
// Bind f3 and s3, alternative reference of f2.sequence.
f3 : Fibonacci( s3 : sequence == (f2.sequence + 1 ), value == -1 )
then
// Note the various referencing techniques.
modify ( f3 ) { value = f1.value + v2 };
System.out.println( s3 + " == " + f3.value );
end
The modify
statement updates the value of the Fibonacci
object bound to f3
. This means that you now have another new Fibonacci
object with a value not equal to -1
, which allows the "Calculate"
rule to re-match and calculate the next Fibonacci number.
The debug view or Audit View of your IDE shows how the firing of the last "Bootstrap"
rule modifies the Fibonacci
object, enabling the "Calculate"
rule to match, which then modifies another Fibonacci
object that enables the "Calculate"
rule to match again. This process continues until the value is set for all Fibonacci
objects.
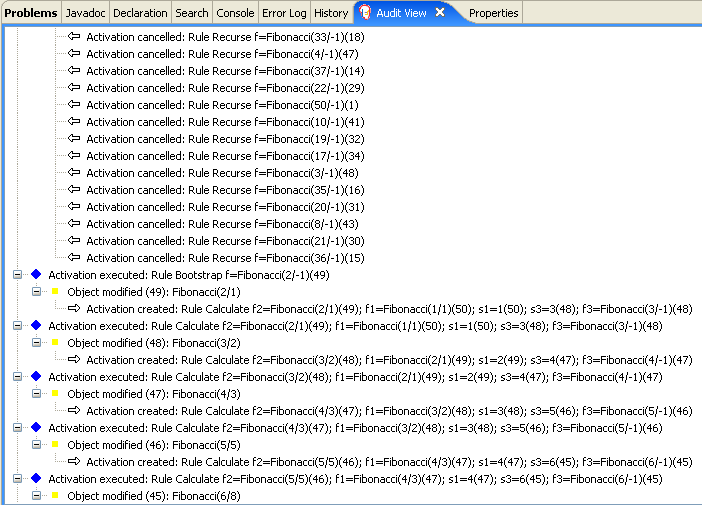
23.6. Pricing example decisions (decision tables)
The Pricing example decision set demonstrates how to use a spreadsheet decision table for calculating the retail cost of an insurance policy in tabular format instead of directly in a DRL file.
The following is an overview of the Pricing example:
-
Name:
decisiontable
-
Main class:
org.drools.examples.decisiontable.PricingRuleDTExample
(insrc/main/java
) -
Module:
drools-examples
-
Type: Java application
-
Rule file:
org.drools.examples.decisiontable.ExamplePolicyPricing.xls
(insrc/main/resources
) -
Objective: Demonstrates use of spreadsheet decision tables to define rules
Spreadsheet decision tables are XLS or XLSX spreadsheets that contain business rules defined in a tabular format. You can include spreadsheet decision tables with standalone Drools projects or upload them to projects in Business Central. Each row in a decision table is a rule, and each column is a condition, an action, or another rule attribute. After you create and upload your decision tables into your Drools project, the rules you defined are compiled into Drools Rule Language (DRL) rules as with all other rule assets.
The purpose of the Pricing example is to provide a set of business rules to calculate the base price and a discount for a car driver applying for a specific type of insurance policy. The driver’s age and history and the policy type all contribute to calculate the basic premium, and additional rules calculate potential discounts for which the driver might be eligible.
To execute the example, run the org.drools.examples.decisiontable.PricingRuleDTExample
class as a Java application in your IDE.
After the execution, the following output appears in the IDE console window:
Cheapest possible
BASE PRICE IS: 120
DISCOUNT IS: 20
The code to execute the example follows the typical execution pattern: the rules are loaded, the facts are inserted, and a stateless KIE session is created. The difference in this example is that the rules are defined in an ExamplePolicyPricing.xls
file instead of a DRL file or other source. The spreadsheet file is loaded into the Drools engine using templates and DRL rules.
Spreadsheet decision table setup
The ExamplePolicyPricing.xls
spreadsheet contains two decision tables in the first tab:
-
Base pricing rules
-
Promotional discount rules
As the example spreadsheet demonstrates, you can use only the first tab of a spreadsheet to create decision tables, but multiple tables can be within a single tab. Decision tables do not necessarily follow top-down logic, but are more of a means to capture data resulting in rules. The evaluation of the rules is not necessarily in the given order, because all of the normal mechanics of the Drools engine still apply. This is why you can have multiple decision tables in the same tab of a spreadsheet.
The decision tables are executed through the corresponding rule template files BasePricing.drt
and PromotionalPricing.drt
. These template files reference the decision tables through their template parameter and directly reference the various headers for the conditions and actions in the decision tables.
template header
age[]
profile
priorClaims
policyType
base
reason
package org.drools.examples.decisiontable;
template "Pricing bracket"
age
policyType
base
rule "Pricing bracket_@{row.rowNumber}"
when
Driver(age >= @{age0}, age <= @{age1}
, priorClaims == "@{priorClaims}"
, locationRiskProfile == "@{profile}"
)
policy: Policy(type == "@{policyType}")
then
policy.setBasePrice(@{base});
System.out.println("@{reason}");
end
end template
template header
age[]
priorClaims
policyType
discount
package org.drools.examples.decisiontable;
template "discounts"
age
priorClaims
policyType
discount
rule "Discounts_@{row.rowNumber}"
when
Driver(age >= @{age0}, age <= @{age1}, priorClaims == "@{priorClaims}")
policy: Policy(type == "@{policyType}")
then
policy.applyDiscount(@{discount});
end
end template
The rules are executed through the kmodule.xml
reference of the KIE Session DTableWithTemplateKB
, which specifically mentions the ExamplePolicyPricing.xls
spreadsheet and is required for successful execution of the rules. This execution method enables you to execute the rules as a standalone unit (as in this example) or to include the rules in a packaged knowledge JAR (KJAR) file, so that the spreadsheet is packaged along with the rules for execution.
The following section of the kmodule.xml
file is required for the execution of the rules and spreadsheet to work successfully:
<kbase name="DecisionTableKB" packages="org.drools.examples.decisiontable">
<ksession name="DecisionTableKS" type="stateless"/>
</kbase>
<kbase name="DTableWithTemplateKB" packages="org.drools.examples.decisiontable-template">
<ruleTemplate dtable="org/drools/examples/decisiontable-template/ExamplePolicyPricingTemplateData.xls"
template="org/drools/examples/decisiontable-template/BasePricing.drt"
row="3" col="3"/>
<ruleTemplate dtable="org/drools/examples/decisiontable-template/ExamplePolicyPricingTemplateData.xls"
template="org/drools/examples/decisiontable-template/PromotionalPricing.drt"
row="18" col="3"/>
<ksession name="DTableWithTemplateKS"/>
</kbase>
As an alternative to executing the decision tables using rule template files, you can use the DecisionTableConfiguration
object and specify an input spreadsheet as the input type, such as DecisionTableInputType.xls
:
DecisionTableConfiguration dtableconfiguration =
KnowledgeBuilderFactory.newDecisionTableConfiguration();
dtableconfiguration.setInputType( DecisionTableInputType.XLS );
KnowledgeBuilder kbuilder = KnowledgeBuilderFactory.newKnowledgeBuilder();
Resource xlsRes = ResourceFactory.newClassPathResource( "ExamplePolicyPricing.xls",
getClass() );
kbuilder.add( xlsRes,
ResourceType.DTABLE,
dtableconfiguration );
The Pricing example uses two fact types:
-
Driver
-
Policy
.
The example sets the default values for both facts in their respective Java classes Driver.java
and Policy.java
. The Driver
is 30 years old, has had no prior claims, and currently has a risk profile of LOW
. The Policy
that the driver is applying for is COMPREHENSIVE
.
In any decision table, each row is considered a different rule and each column is a condition or an action. Each row is evaluated in a decision table unless the agenda is cleared upon execution.
Decision table spreadsheets (XLS or XLSX) require two key areas that define rule data:
-
A
RuleSet
area -
A
RuleTable
area
The RuleSet
area of the spreadsheet defines elements that you want to apply globally to all rules in the same package (not only the spreadsheet), such as a rule set name or universal rule attributes. The RuleTable
area defines the actual rules (rows) and the conditions, actions, and other rule attributes (columns) that constitute that rule table within the specified rule set. A decision table spreadsheet can contain multiple RuleTable
areas, but only one RuleSet
area.
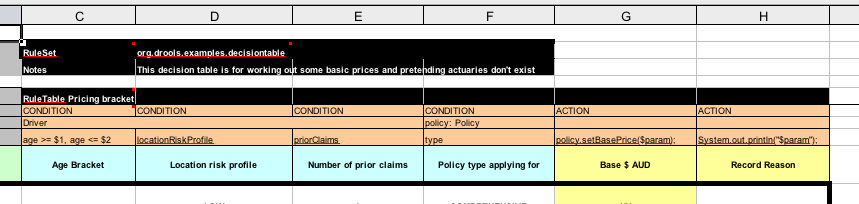
The RuleTable
area also defines the objects to which the rule attributes apply, in this case Driver
and Policy
, followed by constraints on the objects. For example, the Driver
object constraint that defines the Age Bracket
column is age >= $1, age <= $2
, where the comma-separated range is defined in the table column values, such as 18,24
.
Base pricing rules
The Base pricing rules
decision table in the Pricing example evaluates the age, risk profile, number of claims, and policy type of the driver and produces the base price of the policy based on these conditions.
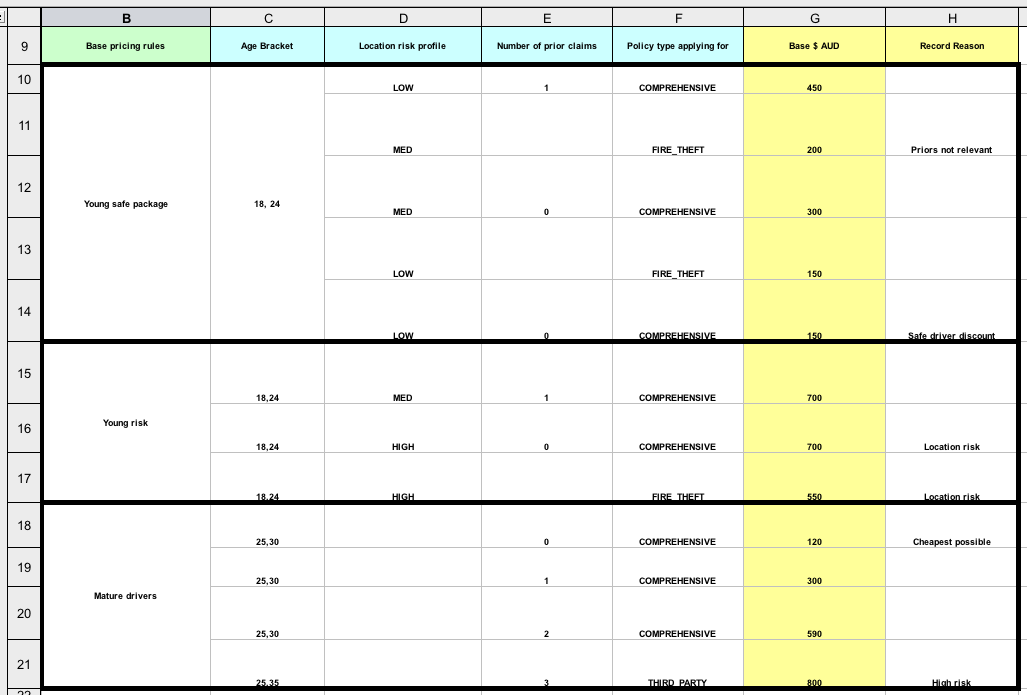
The Driver
attributes are defined in the following table columns:
-
Age Bracket
: The age bracket has a definition for the conditionage >=$1, age <=$2
, which defines the condition boundaries for the driver’s age. This condition column highlights the use of$1 and $2
, which is comma delimited in the spreadsheet. You can write these values as18,24
or18, 24
and both formats work in the execution of the business rules. -
Location risk profile
: The risk profile is a string that the example program passes always asLOW
but can be changed to reflectMED
orHIGH
. -
Number of prior claims
: The number of claims is defined as an integer that the condition column must exactly equal to trigger the action. The value is not a range, only exact matches.
The Policy
of the decision table is used in both the conditions and the actions of the rule and has attributes defined in the following table columns:
-
Policy type applying for
: The policy type is a condition that is passed as a string that defines the type of coverage:COMPREHENSIVE
,FIRE_THEFT
, orTHIRD_PARTY
. -
Base $ AUD
: ThebasePrice
is defined as anACTION
that sets the price through the constraintpolicy.setBasePrice($param);
based on the spreadsheet cells corresponding to this value. When you execute the corresponding DRL rule for this decision table, thethen
portion of the rule executes this action statement on the true conditions matching the facts and sets the base price to the corresponding value. -
Record Reason
: When the rule successfully executes, this action generates an output message to theSystem.out
console reflecting which rule fired. This is later captured in the application and printed.
The example also uses the first column on the left to categorize rules. This column is for annotation only and has no affect on rule execution.
Promotional discount rules
The Promotional discount rules
decision table in the Pricing example evaluates the age, number of prior claims, and policy type of the driver to generate a potential discount on the price of the insurance policy.
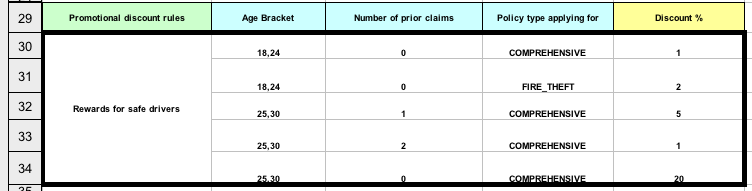
This decision table contains the conditions for the discount for which the driver might be eligible. Similar to the base price calculation, this table evaluates the Age
, Number of prior claims
of the driver, and the Policy type applying for
to determine a Discount %
rate to be applied. For example, if the driver is 30 years old, has no prior claims, and is applying for a COMPREHENSIVE
policy, the driver is given a discount of 20
percent.
23.7. Pet Store example decisions (agenda groups, global variables, callbacks, and GUI integration)
The Pet Store example decision set demonstrates how to use agenda groups and global variables in rules and how to integrate Drools rules with a graphical user interface (GUI), in this case a Swing-based desktop application. The example also demonstrates how to use callbacks to interact with a running Drools engine to update the GUI based on changes in the working memory at run time.
The following is an overview of the Pet Store example:
-
Name:
petstore
-
Main class:
org.drools.examples.petstore.PetStoreExample
(insrc/main/java
) -
Module:
drools-examples
-
Type: Java application
-
Rule file:
org.drools.examples.petstore.PetStore.drl
(insrc/main/resources
) -
Objective: Demonstrates rule agenda groups, global variables, callbacks, and GUI integration
In the Pet Store example, the sample PetStoreExample.java
class defines the following principal classes (in addition to several classes to handle Swing events):
-
Petstore
contains themain()
method. -
PetStoreUI
is responsible for creating and displaying the Swing-based GUI. This class contains several smaller classes, mainly for responding to various GUI events, such as user mouse clicks. -
TableModel
holds the table data. This class is essentially a JavaBean that extends the Swing classAbstractTableModel
. -
CheckoutCallback
enables the GUI to interact with the rules. -
Ordershow
keeps the items that you want to buy. -
Purchase
stores details of the order and the products that you are buying. -
Product
is a JavaBean containing details of the product available for purchase and its price.
Much of the Java code in this example is either plain JavaBean or Swing based. For more information about Swing components, see the Java tutorial on Creating a GUI with JFC/Swing.
Rule execution behavior in the Pet Store example
Unlike other example decision sets where the facts are asserted and fired immediately, the Pet Store example does not execute the rules until more facts are gathered based on user interaction. The example executes rules through a PetStoreUI
object, created by a constructor, that accepts the Vector
object stock
for collecting the products. The example then uses an instance of the CheckoutCallback
class containing the rule base that was previously loaded.
// KieServices is the factory for all KIE services.
KieServices ks = KieServices.Factory.get();
// Create a KIE container on the class path.
KieContainer kc = ks.getKieClasspathContainer();
// Create the stock.
Vector<Product> stock = new Vector<Product>();
stock.add( new Product( "Gold Fish", 5 ) );
stock.add( new Product( "Fish Tank", 25 ) );
stock.add( new Product( "Fish Food", 2 ) );
// A callback is responsible for populating the working memory and for firing all rules.
PetStoreUI ui = new PetStoreUI( stock,
new CheckoutCallback( kc ) );
ui.createAndShowGUI();
The Java code that fires the rules is in the CheckoutCallBack.checkout()
method. This method is triggered when the user clicks Checkout in the UI.
public String checkout(JFrame frame, List<Product> items) {
Order order = new Order();
// Iterate through list and add to cart.
for ( Product p: items ) {
order.addItem( new Purchase( order, p ) );
}
// Add the JFrame to the ApplicationData to allow for user interaction.
// From the KIE container, a KIE session is created based on
// its definition and configuration in the META-INF/kmodule.xml file.
KieSession ksession = kcontainer.newKieSession("PetStoreKS");
ksession.setGlobal( "frame", frame );
ksession.setGlobal( "textArea", this.output );
ksession.insert( new Product( "Gold Fish", 5 ) );
ksession.insert( new Product( "Fish Tank", 25 ) );
ksession.insert( new Product( "Fish Food", 2 ) );
ksession.insert( new Product( "Fish Food Sample", 0 ) );
ksession.insert( order );
// Execute rules.
ksession.fireAllRules();
// Return the state of the cart
return order.toString();
}
The example code passes two elements into the CheckoutCallBack.checkout()
method. One element is the handle for the JFrame
Swing component surrounding the output text frame, found at the bottom of the GUI. The second element is a list of order items, which comes from the TableModel
that stores the information from the Table
area at the upper-right section of the GUI.
The for
loop transforms the list of order items coming from the GUI into the Order
JavaBean, also contained in the file PetStoreExample.java
.
In this case, the rule is firing in a stateless KIE session because all of the data is stored in Swing components and is not executed until the user clicks Checkout in the UI. Each time the user clicks Checkout, the content of the list is moved from the Swing TableModel
into the KIE session working memory and is then executed with the ksession.fireAllRules()
method.
Within this code, there are nine calls to KieSession
. The first of these creates a new KieSession
from the KieContainer
(the example passed in this KieContainer
from the CheckoutCallBack
class in the main()
method). The next two calls pass in the two objects that hold the global variables in the rules: the Swing text area and the Swing frame used for writing messages. More inserts put information on products into the KieSession
, as well as the order list. The final call is the standard fireAllRules()
.
Pet Store rule file imports, global variables, and Java functions
The PetStore.drl
file contains the standard package and import statements to make various Java classes available to the rules. The rule file also includes global variables to be used within the rules, defined as frame
and textArea
. The global variables hold references to the Swing components JFrame
and JTextArea
components that were previously passed on by the Java code that called the setGlobal()
method. Unlike standard variables in rules, which expire as soon as the rule has fired, global variables retain their value for the lifetime of the KIE session. This means the contents of these global variables are available for evaluation on all subsequent rules.
package org.drools.examples;
import org.kie.api.runtime.KieRuntime;
import org.drools.examples.petstore.PetStoreExample.Order;
import org.drools.examples.petstore.PetStoreExample.Purchase;
import org.drools.examples.petstore.PetStoreExample.Product;
import java.util.ArrayList;
import javax.swing.JOptionPane;
import javax.swing.JFrame;
global JFrame frame
global javax.swing.JTextArea textArea
The PetStore.drl
file also contains two functions that the rules in the file use:
function void doCheckout(JFrame frame, KieRuntime krt) {
Object[] options = {"Yes",
"No"};
int n = JOptionPane.showOptionDialog(frame,
"Would you like to checkout?",
"",
JOptionPane.YES_NO_OPTION,
JOptionPane.QUESTION_MESSAGE,
null,
options,
options[0]);
if (n == 0) {
krt.getAgenda().getAgendaGroup( "checkout" ).setFocus();
}
}
function boolean requireTank(JFrame frame, KieRuntime krt, Order order, Product fishTank, int total) {
Object[] options = {"Yes",
"No"};
int n = JOptionPane.showOptionDialog(frame,
"Would you like to buy a tank for your " + total + " fish?",
"Purchase Suggestion",
JOptionPane.YES_NO_OPTION,
JOptionPane.QUESTION_MESSAGE,
null,
options,
options[0]);
System.out.print( "SUGGESTION: Would you like to buy a tank for your "
+ total + " fish? - " );
if (n == 0) {
Purchase purchase = new Purchase( order, fishTank );
krt.insert( purchase );
order.addItem( purchase );
System.out.println( "Yes" );
} else {
System.out.println( "No" );
}
return true;
}
The two functions perform the following actions:
-
doCheckout()
displays a dialog that asks the user if she or he wants to check out. If the user does, the focus is set to thecheckout
agenda group, enabling rules in that group to (potentially) fire. -
requireTank()
displays a dialog that asks the user if she or he wants to buy a fish tank. If the user does, a new fish tankProduct
is added to the order list in the working memory.
For this example, all rules and functions are within the same rule file for efficiency. In a production environment, you typically separate the rules and functions in different files or build a static Java method and import the files using the import function, such as import function my.package.name.hello .
|
Pet Store rules with agenda groups
Most of the rules in the Pet Store example use agenda groups to control rule execution. Agenda groups allow you to partition the Drools engine agenda to provide more execution control over groups of rules. By default, all rules are in the agenda group MAIN
. You can use the agenda-group
attribute to specify a different agenda group for the rule.
Initially, a working memory has its focus on the agenda group MAIN
. Rules in an agenda group only fire when the group receives the focus. You can set the focus either by using the method setFocus()
or the rule attribute auto-focus
. The auto-focus
attribute enables the rule to be given a focus automatically for its agenda group when the rule is matched and activated.
The Pet Store example uses the following agenda groups for rules:
-
"init"
-
"evaluate"
-
"show items"
-
"checkout"
For example, the sample rule "Explode Cart"
uses the "init"
agenda group to ensure that it has the option to fire and insert shopping cart items into the KIE session working memory:
// Insert each item in the shopping cart into the working memory.
rule "Explode Cart"
agenda-group "init"
auto-focus true
salience 10
dialect "java"
when
$order : Order( grossTotal == -1 )
$item : Purchase() from $order.items
then
insert( $item );
kcontext.getKnowledgeRuntime().getAgenda().getAgendaGroup( "show items" ).setFocus();
kcontext.getKnowledgeRuntime().getAgenda().getAgendaGroup( "evaluate" ).setFocus();
end
This rule matches against all orders that do not yet have their grossTotal
calculated. The execution loops for each purchase item in that order.
The rule uses the following features related to its agenda group:
-
agenda-group "init"
defines the name of the agenda group. In this case, only one rule is in the group. However, neither the Java code nor a rule consequence sets the focus to this group, and therefore it relies on theauto-focus
attribute for its chance to fire. -
auto-focus true
ensures that this rule, while being the only rule in the agenda group, gets a chance to fire whenfireAllRules()
is called from the Java code. -
kcontext….setFocus()
sets the focus to the"show items"
and"evaluate"
agenda groups, enabling their rules to fire. In practice, you loop through all items in the order, insert them into memory, and then fire the other rules after each insertion.
The "show items"
agenda group contains only one rule, "Show Items"
. For each purchase in the order currently in the KIE session working memory, the rule logs details to the text area at the bottom of the GUI, based on the textArea
variable defined in the rule file.
rule "Show Items"
agenda-group "show items"
dialect "mvel"
when
$order : Order( )
$p : Purchase( order == $order )
then
textArea.append( $p.product + "\n");
end
The "evaluate"
agenda group also gains focus from the "Explode Cart"
rule. This agenda group contains two rules, "Free Fish Food Sample"
and "Suggest Tank"
, which are executed in that order.
// Free fish food sample when users buy a goldfish if they did not already buy
// fish food and do not already have a fish food sample.
rule "Free Fish Food Sample"
agenda-group "evaluate" (1)
dialect "mvel"
when
$order : Order()
not ( $p : Product( name == "Fish Food") && Purchase( product == $p ) ) (2)
not ( $p : Product( name == "Fish Food Sample") && Purchase( product == $p ) ) (3)
exists ( $p : Product( name == "Gold Fish") && Purchase( product == $p ) ) (4)
$fishFoodSample : Product( name == "Fish Food Sample" );
then
System.out.println( "Adding free Fish Food Sample to cart" );
purchase = new Purchase($order, $fishFoodSample);
insert( purchase );
$order.addItem( purchase );
end
The rule "Free Fish Food Sample"
fires only if all of the following conditions are true:
1 | The agenda group "evaluate" is being evaluated in the rules execution. |
2 | User does not already have fish food. |
3 | User does not already have a free fish food sample. |
4 | User has a goldfish in the order. |
If the order facts meet all of these requirements, then a new product is created (Fish Food Sample) and is added to the order in working memory.
// Suggest a fish tank if users buy more than five goldfish and
// do not already have a tank.
rule "Suggest Tank"
agenda-group "evaluate"
dialect "java"
when
$order : Order()
not ( $p : Product( name == "Fish Tank") && Purchase( product == $p ) ) (1)
ArrayList( $total : size > 5 ) from collect( Purchase( product.name == "Gold Fish" ) ) (2)
$fishTank : Product( name == "Fish Tank" )
then
requireTank(frame, kcontext.getKieRuntime(), $order, $fishTank, $total);
end
The rule "Suggest Tank"
fires only if the following conditions are true:
1 | User does not have a fish tank in the order. |
2 | User has more than five fish in the order. |
When the rule fires, it calls the requireTank()
function defined in the rule file. This function displays a dialog that asks the user if she or he wants to buy a fish tank. If the user does, a new fish tank Product
is added to the order list in the working memory. When the rule calls the requireTank()
function, the rule passes the frame
global variable so that the function has a handle for the Swing GUI.
The "do checkout"
rule in the Pet Store example has no agenda group and no when
conditions, so the rule is always executed and considered part of the default MAIN
agenda group.
rule "do checkout"
dialect "java"
when
then
doCheckout(frame, kcontext.getKieRuntime());
end
When the rule fires, it calls the doCheckout()
function defined in the rule file. This function displays a dialog that asks the user if she or he wants to check out. If the user does, the focus is set to the checkout
agenda group, enabling rules in that group to (potentially) fire. When the rule calls the doCheckout()
function, the rule passes the frame
global variable so that the function has a handle for the Swing GUI.
This example also demonstrates a troubleshooting technique if results are not executing as you expect: You can remove the conditions from the when statement of a rule and test the action in the then statement to verify that the action is performed correctly.
|
The "checkout"
agenda group contains three rules for processing the order checkout and applying any discounts: "Gross Total"
, "Apply 5% Discount"
, and "Apply 10% Discount"
.
rule "Gross Total"
agenda-group "checkout"
dialect "mvel"
when
$order : Order( grossTotal == -1)
Number( total : doubleValue ) from accumulate( Purchase( $price : product.price ),
sum( $price ) )
then
modify( $order ) { grossTotal = total }
textArea.append( "\ngross total=" + total + "\n" );
end
rule "Apply 5% Discount"
agenda-group "checkout"
dialect "mvel"
when
$order : Order( grossTotal >= 10 && < 20 )
then
$order.discountedTotal = $order.grossTotal * 0.95;
textArea.append( "discountedTotal total=" + $order.discountedTotal + "\n" );
end
rule "Apply 10% Discount"
agenda-group "checkout"
dialect "mvel"
when
$order : Order( grossTotal >= 20 )
then
$order.discountedTotal = $order.grossTotal * 0.90;
textArea.append( "discountedTotal total=" + $order.discountedTotal + "\n" );
end
If the user has not already calculated the gross total, the Gross Total
accumulates the product prices into a total, puts this total into the KIE session, and displays it through the Swing JTextArea
using the textArea
global variable.
If the gross total is between 10
and 20
(currency units), the "Apply 5% Discount"
rule calculates the discounted total, adds it to the KIE session, and displays it in the text area.
If the gross total is not less than 20
, the "Apply 10% Discount"
rule calculates the discounted total, adds it to the KIE session, and displays it in the text area.
Pet Store example execution
Similar to other Drools decision examples, you execute the Pet Store example by running the org.drools.examples.petstore.PetStoreExample
class as a Java application in your IDE.
When you execute the Pet Store example, the Pet Store Demo
GUI window appears. This window displays a list of available products (upper left), an empty list of selected products (upper right), Checkout and Reset buttons (middle), and an empty system messages area (bottom).
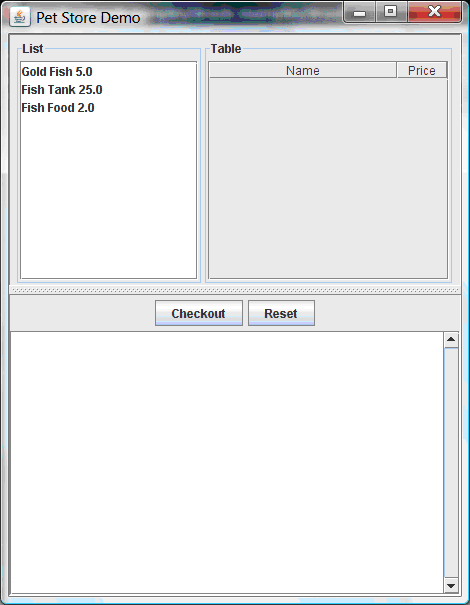
The following events occurred in this example to establish this execution behavior:
-
The
main()
method has run and loaded the rule base but has not yet fired the rules. So far, this is the only code in connection with rules that has been run. -
A new
PetStoreUI
object has been created and given a handle for the rule base, for later use. -
Various Swing components have performed their functions, and the initial UI screen is displayed and waits for user input.
You can click on various products from the list to explore the UI setup:
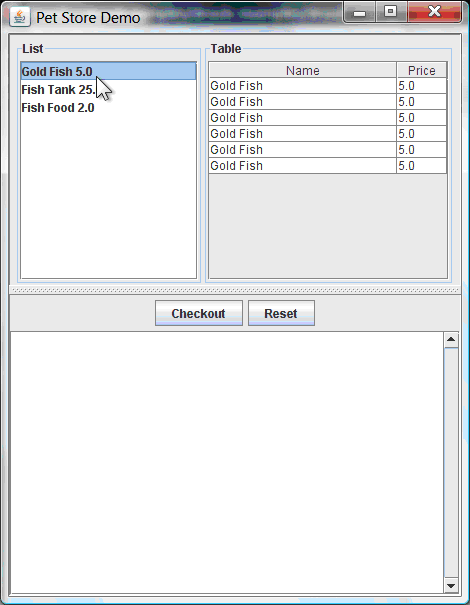
No rules code has been fired yet. The UI uses Swing code to detect user mouse clicks and add selected products to the TableModel
object for display in the upper-right corner of the UI. This example illustrates the Model-View-Controller design pattern.
When you click Checkout, the rules are then fired in the following way:
-
Method
CheckOutCallBack.checkout()
is called (eventually) by the Swing class waiting for the click on Checkout. This inserts the data from theTableModel
object (upper-right corner of the UI) into the KIE session working memory. The method then fires the rules. -
The
"Explode Cart"
rule is the first to fire, with theauto-focus
attribute set totrue
. The rule loops through all of the products in the cart, ensures that the products are in the working memory, and then gives the"show Items"
and"evaluate"
agenda groups the option to fire. The rules in these groups add the contents of the cart to the text area (bottom of the UI), evaluate if you are eligible for free fish food, and determine whether to ask if you want to buy a fish tank.Figure 359. Fish tank qualification -
The
"do checkout"
rule is the next to fire because no other agenda group currently has focus and because it is part of the defaultMAIN
agenda group. This rule always calls thedoCheckout()
function, which asks you if you want to check out. -
The
doCheckout()
function sets the focus to the"checkout"
agenda group, giving the rules in that group the option to fire. -
The rules in the
"checkout"
agenda group display the contents of the cart and apply the appropriate discount. -
Swing then waits for user input to either select more products (and cause the rules to fire again) or to close the UI.
Figure 360. Pet Store example GUI after all rules have fired
You can add more System.out
calls to demonstrate this flow of events in your IDE console:
Adding free Fish Food Sample to cart
SUGGESTION: Would you like to buy a tank for your 6 fish? - Yes
23.8. Honest Politician example decisions (truth maintenance and salience)
The Honest Politician example decision set demonstrates the concept of truth maintenance with logical insertions and the use of salience in rules.
The following is an overview of the Honest Politician example:
-
Name:
honestpolitician
-
Main class:
org.drools.examples.honestpolitician.HonestPoliticianExample
(insrc/main/java
) -
Module:
drools-examples
-
Type: Java application
-
Rule file:
org.drools.examples.honestpolitician.HonestPolitician.drl
(insrc/main/resources
) -
Objective: Demonstrates the concept of truth maintenance based on the logical insertion of facts and the use of salience in rules
The basic premise of the Honest Politician example is that an object can only exist while a statement is true. A rule consequence can logically insert an object with the insertLogical()
method. This means the object remains in the KIE session working memory as long as the rule that logically inserted it remains true. When the rule is no longer true, the object is automatically retracted.
In this example, rule execution causes a group of politicians to change from being honest to being dishonest as a result of a corrupt corporation. As each politician is evaluated, they start out with their honesty attribute being set to true
, but a rule fires that makes the politicians no longer honest. As they switch their state from being honest to dishonest, they are then removed from the working memory. The rule salience notifies the Drools engine how to prioritize any rules that have a salience defined for them, otherwise utilizing the default salience value of 0
. Rules with a higher salience value are given higher priority when ordered in the activation queue.
Politician and Hope classes
The sample class Politician
in the example is configured for an honest politician. The Politician
class is made up of a String item name
and a Boolean item honest
:
public class Politician {
private String name;
private boolean honest;
...
}
The Hope
class determines if a Hope
object exists. This class has no meaningful members, but is present in the working memory as long as society has hope.
public class Hope {
public Hope() {
}
}
Rule definitions for politician honesty
In the Honest Politician example, when at least one honest politician exists in the working memory, the "We have an honest Politician"
rule logically inserts a new Hope
object. As soon as all politicians become dishonest, the Hope
object is automatically retracted. This rule has a salience
attribute with a value of 10
to ensure that it fires before any other rule, because at that stage the "Hope is Dead"
rule is true.
rule "We have an honest Politician"
salience 10
when
exists( Politician( honest == true ) )
then
insertLogical( new Hope() );
end
As soon as a Hope
object exists, the "Hope Lives"
rule matches and fires. This rule also has a salience
value of 10
so that it takes priority over the "Corrupt the Honest"
rule.
rule "Hope Lives"
salience 10
when
exists( Hope() )
then
System.out.println("Hurrah!!! Democracy Lives");
end
Initially, four honest politicians exist so this rule has four activations, all in conflict. Each rule fires in turn, corrupting each politician so that they are no longer honest. When all four politicians have been corrupted, no politicians have the property honest == true
. The rule "We have an honest Politician"
is no longer true and the object it logically inserted (due to the last execution of new Hope()
) is automatically retracted.
rule "Corrupt the Honest"
when
politician : Politician( honest == true )
exists( Hope() )
then
System.out.println( "I'm an evil corporation and I have corrupted " + politician.getName() );
modify ( politician ) { honest = false };
end
With the Hope
object automatically retracted through the truth maintenance system, the conditional element not
applied to Hope
is no longer true so that the "Hope is Dead"
rule matches and fires.
rule "Hope is Dead"
when
not( Hope() )
then
System.out.println( "We are all Doomed!!! Democracy is Dead" );
end
Example execution and audit trail
In the HonestPoliticianExample.java
class, the four politicians with the honest state set to true
are inserted for evaluation against the defined business rules:
public static void execute( KieContainer kc ) {
KieSession ksession = kc.newKieSession("HonestPoliticianKS");
final Politician p1 = new Politician( "President of Umpa Lumpa", true );
final Politician p2 = new Politician( "Prime Minster of Cheeseland", true );
final Politician p3 = new Politician( "Tsar of Pringapopaloo", true );
final Politician p4 = new Politician( "Omnipotence Om", true );
ksession.insert( p1 );
ksession.insert( p2 );
ksession.insert( p3 );
ksession.insert( p4 );
ksession.fireAllRules();
ksession.dispose();
}
To execute the example, run the org.drools.examples.honestpolitician.HonestPoliticianExample
class as a Java application in your IDE.
After the execution, the following output appears in the IDE console window:
Hurrah!!! Democracy Lives
I'm an evil corporation and I have corrupted President of Umpa Lumpa
I'm an evil corporation and I have corrupted Prime Minster of Cheeseland
I'm an evil corporation and I have corrupted Tsar of Pringapopaloo
I'm an evil corporation and I have corrupted Omnipotence Om
We are all Doomed!!! Democracy is Dead
The output shows that, while there is at least one honest politician, democracy lives. However, as each politician is corrupted by some corporation, all politicians become dishonest, and democracy is dead.
To better understand the execution flow of this example, you can modify the HonestPoliticianExample.java
class to include a RuleRuntime
listener and an audit logger to view execution details:
package org.drools.examples.honestpolitician;
import org.kie.api.KieServices;
import org.kie.api.event.rule.DebugAgendaEventListener; (1)
import org.kie.api.event.rule.DebugRuleRuntimeEventListener;
import org.kie.api.runtime.KieContainer;
import org.kie.api.runtime.KieSession;
public class HonestPoliticianExample {
/**
* @param args
*/
public static void main(final String[] args) {
KieServices ks = KieServices.Factory.get(); (2)
//ks = KieServices.Factory.get();
KieContainer kc = KieServices.Factory.get().getKieClasspathContainer();
System.out.println(kc.verify().getMessages().toString());
//execute( kc );
execute( ks, kc); (3)
}
public static void execute( KieServices ks, KieContainer kc ) { (4)
KieSession ksession = kc.newKieSession("HonestPoliticianKS");
final Politician p1 = new Politician( "President of Umpa Lumpa", true );
final Politician p2 = new Politician( "Prime Minster of Cheeseland", true );
final Politician p3 = new Politician( "Tsar of Pringapopaloo", true );
final Politician p4 = new Politician( "Omnipotence Om", true );
ksession.insert( p1 );
ksession.insert( p2 );
ksession.insert( p3 );
ksession.insert( p4 );
// The application can also setup listeners (5)
ksession.addEventListener( new DebugAgendaEventListener() );
ksession.addEventListener( new DebugRuleRuntimeEventListener() );
// Set up a file-based audit logger.
ks.getLoggers().newFileLogger( ksession, "./target/honestpolitician" ); (6)
ksession.fireAllRules();
ksession.dispose();
}
}
1 | Adds to your imports the packages that handle the DebugAgendaEventListener and DebugRuleRuntimeEventListener |
2 | Creates a KieServices Factory and a ks element to produce the logs because this audit log is not available at the KieContainer level |
3 | Modifies the execute method to use both KieServices and KieContainer |
4 | Modifies the execute method to pass in KieServices in addition to the KieContainer |
5 | Creates the listeners |
6 | Builds the log that can be passed into the debug view or Audit View or your IDE after executing of the rules |
When you run the Honest Politician with this modified logging capability, you can load the audit log file from target/honestpolitician.log
into your IDE debug view or Audit View, if available (for example, in Window → Show View in some IDEs).
In this example, the Audit View shows the flow of executions, insertions, and retractions as defined in the example classes and rules:
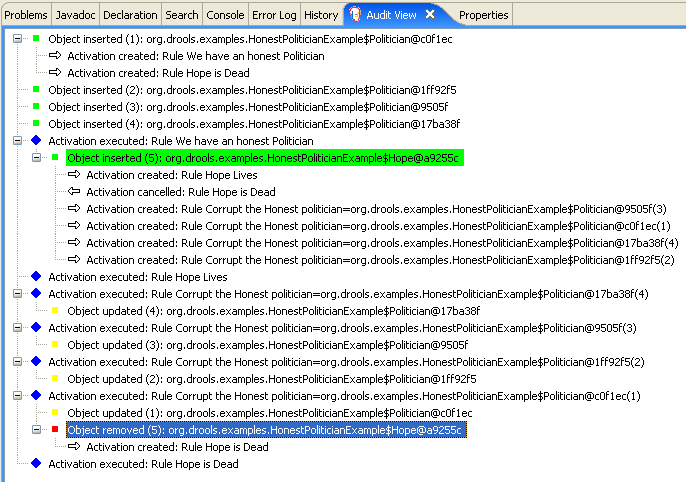
When the first politician is inserted, two activations occur. The rule "We have an honest Politician"
is activated only one time for the first inserted politician because it uses an exists
conditional element, which matches when at least one politician is inserted. The rule "Hope is Dead"
is also activated at this stage because the Hope
object is not yet inserted. The rule "We have an honest Politician"
fires first because it has a higher salience
value than the rule "Hope is Dead"
, and inserts the Hope
object (highlighted in green). The insertion of the Hope
object activates the rule "Hope Lives"
and deactivates the rule "Hope is Dead"
. The insertion also activates the rule "Corrupt the Honest"
for each inserted honest politician. The rule "Hope Lives"
is executed and prints "Hurrah!!! Democracy Lives"
.
Next, for each politician, the rule "Corrupt the Honest"
fires, printing "I’m an evil corporation and I have corrupted X"
, where X
is the name of the politician, and modifies the politician honesty value to false
. When the last honest politician is corrupted, Hope
is automatically retracted by the truth maintenance system (highlighted in blue). The green highlighted area shows the origin of the currently selected blue highlighted area. After the Hope
fact is retracted, the rule "Hope is dead"
fires, printing "We are all Doomed!!! Democracy is Dead"
.
23.9. Sudoku example decisions (complex pattern matching, callbacks, and GUI integration)
The Sudoku example decision set, based on the popular number puzzle Sudoku, demonstrates how to use rules in Drools to find a solution in a large potential solution space based on various constraints. This example also shows how to integrate Drools rules into a graphical user interface (GUI), in this case a Swing-based desktop application, and how to use callbacks to interact with a running Drools engine to update the GUI based on changes in the working memory at run time.
The following is an overview of the Sudoku example:
-
Name:
sudoku
-
Main class:
org.drools.examples.sudoku.SudokuExample
(insrc/main/java
) -
Module:
drools-examples
-
Type: Java application
-
Rule files:
org.drools.examples.sudoku.*.drl
(insrc/main/resources
) -
Objective: Demonstrates complex pattern matching, problem solving, callbacks, and GUI integration
Sudoku is a logic-based number placement puzzle. The objective is to fill a 9x9 grid so that each column, each row, and each of the nine 3x3 zones contains the digits from 1 to 9 only one time. The puzzle setter provides a partially completed grid and the puzzle solver’s task is to complete the grid with these constraints.
The general strategy to solve the problem is to ensure that when you insert a new number, it must be unique in its particular 3x3 zone, row, and column. This Sudoku example decision set uses Drools rules to solve Sudoku puzzles from a range of difficulty levels, and to attempt to resolve flawed puzzles that contain invalid entries.
Sudoku example execution and interaction
Similar to other Drools decision examples, you execute the Sudoku example by running the org.drools.examples.sudoku.SudokuExample
class as a Java application in your IDE.
When you execute the Sudoku example, the Drools Sudoku Example
GUI window appears. This window contains an empty grid, but the program comes with various grids stored internally that you can load and solve.
Click File → Samples → Simple to load one of the examples. Notice that all buttons are disabled until a grid is loaded.
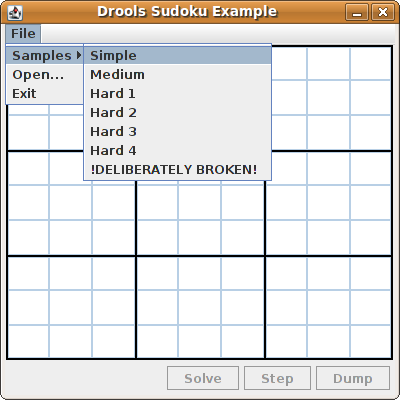
When you load the Simple example, the grid is filled according to the puzzle’s initial state.
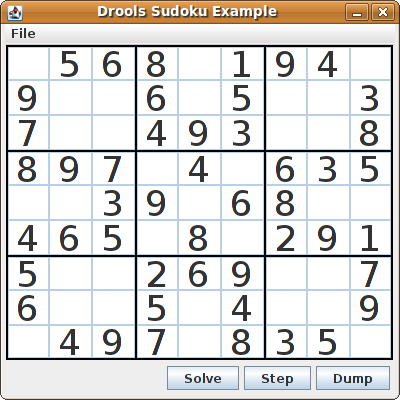
Choose from the following options:
-
Click Solve to fire the rules defined in the Sudoku example that fill out the remaining values and that make the buttons inactive again.
Figure 364. Simple sample solved -
Click Step to see the next digit found by the rule set. The console window in your IDE displays detailed information about the rules that are executing to solve the step.
Step execution output in the IDE consolesingle 8 at [0,1] column elimination due to [1,2]: remove 9 from [4,2] hidden single 9 at [1,2] row elimination due to [2,8]: remove 7 from [2,4] remove 6 from [3,8] due to naked pair at [3,2] and [3,7] hidden pair in row at [4,6] and [4,4]
-
Click Dump to see the state of the grid, with cells showing either the established value or the remaining possibilities.
Dump execution output in the IDE consoleCol: 0 Col: 1 Col: 2 Col: 3 Col: 4 Col: 5 Col: 6 Col: 7 Col: 8 Row 0: 123456789 --- 5 --- --- 6 --- --- 8 --- 123456789 --- 1 --- --- 9 --- --- 4 --- 123456789 Row 1: --- 9 --- 123456789 123456789 --- 6 --- 123456789 --- 5 --- 123456789 123456789 --- 3 --- Row 2: --- 7 --- 123456789 123456789 --- 4 --- --- 9 --- --- 3 --- 123456789 123456789 --- 8 --- Row 3: --- 8 --- --- 9 --- --- 7 --- 123456789 --- 4 --- 123456789 --- 6 --- --- 3 --- --- 5 --- Row 4: 123456789 123456789 --- 3 --- --- 9 --- 123456789 --- 6 --- --- 8 --- 123456789 123456789 Row 5: --- 4 --- --- 6 --- --- 5 --- 123456789 --- 8 --- 123456789 --- 2 --- --- 9 --- --- 1 --- Row 6: --- 5 --- 123456789 123456789 --- 2 --- --- 6 --- --- 9 --- 123456789 123456789 --- 7 --- Row 7: --- 6 --- 123456789 123456789 --- 5 --- 123456789 --- 4 --- 123456789 123456789 --- 9 --- Row 8: 123456789 --- 4 --- --- 9 --- --- 7 --- 123456789 --- 8 --- --- 3 --- --- 5 --- 123456789
The Sudoku example includes a deliberately broken sample file that the rules defined in the example can resolve.
Click File → Samples → !DELIBERATELY BROKEN! to load the broken sample. The grid starts with some issues, for example, the value 5
appears two times in the first row, which is not allowed.
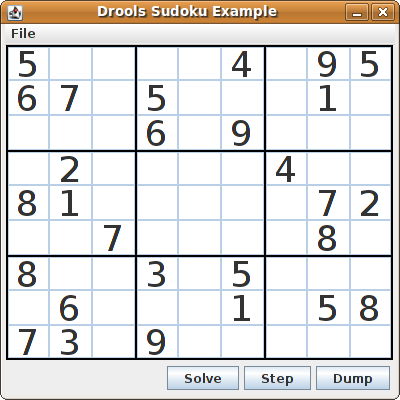
Click Solve to apply the solving rules to this invalid grid. The associated solving rules in the Sudoku example detect the issues in the sample and attempts to solve the puzzle as far as possible. This process does not complete and leaves some cells empty.
The solving rule activity is displayed in the IDE console window:
cell [0,8]: 5 has a duplicate in row 0 cell [0,0]: 5 has a duplicate in row 0 cell [6,0]: 8 has a duplicate in col 0 cell [4,0]: 8 has a duplicate in col 0 Validation complete.
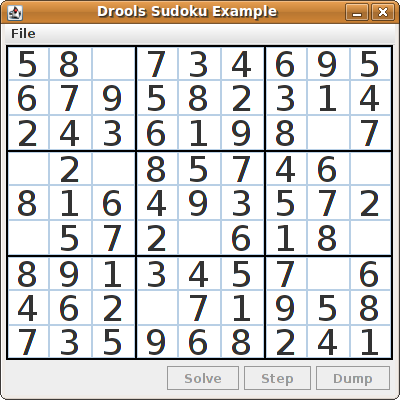
The sample Sudoku files labeled Hard are more complex and the solving rules might not be able to solve them. The unsuccessful solution attempt is displayed in the IDE console window:
Validation complete. ... Sorry - can't solve this grid.
The rules that work to solve the broken sample implement standard solving techniques based on the sets of values that are still candidates for a cell. For example, if a set contains a single value, then this is the value for the cell. For a single occurrence of a value in one of the groups of nine cells, the rules insert a fact of type Setting
with the solution value for some specific cell. This fact causes the elimination of this value from all other cells in any of the groups the cell belongs to and the value is retracted.
Other rules in the example reduce the permissible values for some cells. The rules "naked pair"
, "hidden pair in row"
, "hidden pair in column"
, and "hidden pair in square"
eliminate possibilities but do not establish solutions. The rules "X-wings in rows"
, "`X-wings in columns"`, "intersection removal row"
, and "intersection removal column"
perform more sophisticated eliminations.
Sudoku example classes
The package org.drools.examples.sudoku.swing
contains the following core set of classes that implement a framework for Sudoku puzzles:
-
The
SudokuGridModel
class defines an interface that is implemented to store a Sudoku puzzle as a 9x9 grid ofCell
objects. -
The
SudokuGridView
class is a Swing component that can visualize any implementation of theSudokuGridModel
class. -
The
SudokuGridEvent
andSudokuGridListener
classes communicate state changes between the model and the view. Events are fired when a cell value is resolved or changed. -
The
SudokuGridSamples
class provides partially filled Sudoku puzzles for demonstration purposes.
This package does not have any dependencies on Drools libraries. |
The package org.drools.examples.sudoku
contains the following core set of classes that implement the elementary Cell
object and its various aggregations:
-
The
CellFile
class, with subtypesCellRow
,CellCol
, andCellSqr
, all of which are subtypes of theCellGroup
class. -
The
Cell
andCellGroup
subclasses ofSetOfNine
, which provides a propertyfree
with the typeSet<Integer>
. For aCell
class, the set represents the individual candidate set. For aCellGroup
class, the set is the union of all candidate sets of its cells (the set of digits that still need to be allocated).In the Sudoku example are 81
Cell
and 27CellGroup
objects and a linkage provided by theCell
propertiescellRow
,cellCol
, andcellSqr
, and by theCellGroup
propertycells
(a list ofCell
objects). With these components, you can write rules that detect the specific situations that permit the allocation of a value to a cell or the elimination of a value from some candidate set. -
The
Setting
class is used to trigger the operations that accompany the allocation of a value. The presence of aSetting
fact is used in all rules that detect a new situation in order to avoid reactions to inconsistent intermediary states. -
The
Stepping
class is used in a low priority rule to execute an emergency halt when a"Step"
does not terminate regularly. This behavior indicates that the program cannot solve the puzzle. -
The main class
org.drools.examples.sudoku.SudokuExample
implements a Java application combining all of these components.
Sudoku validation rules (validate.drl)
The validate.drl
file in the Sudoku example contains validation rules that detect duplicate numbers in cell groups. They are combined in a "validate"
agenda group that enables the rules to be explicitly activated after a user loads the puzzle.
The when
conditions of the three rules "duplicate in cell …"
all function in the following ways:
-
The first condition in the rule locates a cell with an allocated value.
-
The second condition in the rule pulls in any of the three cell groups to which the cell belongs.
-
The final condition finds a cell (other than the first one) with the same value as the first cell and in the same row, column, or square, depending on the rule.
rule "duplicate in cell row"
when
$c: Cell( $v: value != null )
$cr: CellRow( cells contains $c )
exists Cell( this != $c, value == $v, cellRow == $cr )
then
System.out.println( "cell " + $c.toString() + " has a duplicate in row " + $cr.getNumber() );
end
rule "duplicate in cell col"
when
$c: Cell( $v: value != null )
$cc: CellCol( cells contains $c )
exists Cell( this != $c, value == $v, cellCol == $cc )
then
System.out.println( "cell " + $c.toString() + " has a duplicate in col " + $cc.getNumber() );
end
rule "duplicate in cell sqr"
when
$c: Cell( $v: value != null )
$cs: CellSqr( cells contains $c )
exists Cell( this != $c, value == $v, cellSqr == $cs )
then
System.out.println( "cell " + $c.toString() + " has duplicate in its square of nine." );
end
The rule "terminate group"
is the last to fire. This rule prints a message and stops the sequence.
rule "terminate group"
salience -100
when
then
System.out.println( "Validation complete." );
drools.halt();
end
Sudoku solving rules (sudoku.drl)
The sudoku.drl
file in the Sudoku example contains three types of rules: one group handles the allocation of a number to a cell, another group detects feasible allocations, and the third group eliminates values from candidate sets.
The rules "set a value"
, "eliminate a value from Cell"
, and "retract setting"
depend on the presence of a Setting
object. The first rule handles the assignment to the cell and the operations for removing the value from the free
sets of the three groups of the cell. This group also reduces a counter that, when zero, returns control to the Java application that has called fireUntilHalt()
.
The purpose of the rule "eliminate a value from Cell"
is to reduce the candidate lists of all cells that are related to the newly assigned cell. Finally, when all eliminations have been made, the rule "retract setting"
retracts the triggering Setting
fact.
// A Setting object is inserted to define the value of a Cell.
// Rule for updating the cell and all cell groups that contain it
rule "set a value"
when
// A Setting with row and column number, and a value
$s: Setting( $rn: rowNo, $cn: colNo, $v: value )
// A matching Cell, with no value set
$c: Cell( rowNo == $rn, colNo == $cn, value == null,
$cr: cellRow, $cc: cellCol, $cs: cellSqr )
// Count down
$ctr: Counter( $count: count )
then
// Modify the Cell by setting its value.
modify( $c ){ setValue( $v ) }
// System.out.println( "set cell " + $c.toString() );
modify( $cr ){ blockValue( $v ) }
modify( $cc ){ blockValue( $v ) }
modify( $cs ){ blockValue( $v ) }
modify( $ctr ){ setCount( $count - 1 ) }
end
// Rule for removing a value from all cells that are siblings
// in one of the three cell groups
rule "eliminate a value from Cell"
when
// A Setting with row and column number, and a value
$s: Setting( $rn: rowNo, $cn: colNo, $v: value )
// The matching Cell, with the value already set
Cell( rowNo == $rn, colNo == $cn, value == $v, $exCells: exCells )
// For all Cells that are associated with the updated cell
$c: Cell( free contains $v ) from $exCells
then
// System.out.println( "clear " + $v + " from cell " + $c.posAsString() );
// Modify a related Cell by blocking the assigned value.
modify( $c ){ blockValue( $v ) }
end
// Rule for eliminating the Setting fact
rule "retract setting"
when
// A Setting with row and column number, and a value
$s: Setting( $rn: rowNo, $cn: colNo, $v: value )
// The matching Cell, with the value already set
$c: Cell( rowNo == $rn, colNo == $cn, value == $v )
// This is the negation of the last pattern in the previous rule.
// Now the Setting fact can be safely retracted.
not( $x: Cell( free contains $v )
and
Cell( this == $c, exCells contains $x ) )
then
// System.out.println( "done setting cell " + $c.toString() );
// Discard the Setter fact.
delete( $s );
// Sudoku.sudoku.consistencyCheck();
end
Two solving rules detect a situation where an allocation of a number to a cell is possible. The rule "single"
fires for a Cell
with a candidate set containing a single number. The rule "hidden single"
fires when no cell exists with a single candidate, but when a cell exists containing a candidate, this candidate is absent from all other cells in one of the three groups to which the cell belongs. Both rules create and insert a Setting
fact.
// Detect a set of candidate values with cardinality 1 for some Cell.
// This is the value to be set.
rule "single"
when
// Currently no setting underway
not Setting()
// One element in the "free" set
$c: Cell( $rn: rowNo, $cn: colNo, freeCount == 1 )
then
Integer i = $c.getFreeValue();
if (explain) System.out.println( "single " + i + " at " + $c.posAsString() );
// Insert another Setter fact.
insert( new Setting( $rn, $cn, i ) );
end
// Detect a set of candidate values with a value that is the only one
// in one of its groups. This is the value to be set.
rule "hidden single"
when
// Currently no setting underway
not Setting()
not Cell( freeCount == 1 )
// Some integer
$i: Integer()
// The "free" set contains this number
$c: Cell( $rn: rowNo, $cn: colNo, freeCount > 1, free contains $i )
// A cell group contains this cell $c.
$cg: CellGroup( cells contains $c )
// No other cell from that group contains $i.
not ( Cell( this != $c, free contains $i ) from $cg.getCells() )
then
if (explain) System.out.println( "hidden single " + $i + " at " + $c.posAsString() );
// Insert another Setter fact.
insert( new Setting( $rn, $cn, $i ) );
end
Rules from the largest group, either individually or in groups of two or three, implement various solving techniques used for solving Sudoku puzzles manually.
The rule "naked pair"
detects identical candidate sets of size 2
in two cells of a group. These two values may be removed from all other candidate sets of that group.
// A "naked pair" is two cells in some cell group with their sets of
// permissible values being equal with cardinality 2. These two values
// can be removed from all other candidate lists in the group.
rule "naked pair"
when
// Currently no setting underway
not Setting()
not Cell( freeCount == 1 )
// One cell with two candidates
$c1: Cell( freeCount == 2, $f1: free, $r1: cellRow, $rn1: rowNo, $cn1: colNo, $b1: cellSqr )
// The containing cell group
$cg: CellGroup( freeCount > 2, cells contains $c1 )
// Another cell with two candidates, not the one we already have
$c2: Cell( this != $c1, free == $f1 /*** , rowNo >= $rn1, colNo >= $cn1 ***/ ) from $cg.cells
// Get one of the "naked pair".
Integer( $v: intValue ) from $c1.getFree()
// Get some other cell with a candidate equal to one from the pair.
$c3: Cell( this != $c1 && != $c2, freeCount > 1, free contains $v ) from $cg.cells
then
if (explain) System.out.println( "remove " + $v + " from " + $c3.posAsString() + " due to naked pair at " + $c1.posAsString() + " and " + $c2.posAsString() );
// Remove the value.
modify( $c3 ){ blockValue( $v ) }
end
The three rules "hidden pair in …"
functions similarly to the rule "naked pair"
. These rules detect a subset of two numbers in exactly two cells of a group, with neither value occurring in any of the other cells of the group. This means that all other candidates can be eliminated from the two cells harboring the hidden pair.
// If two cells within the same cell group contain candidate sets with more than
// two values, with two values being in both of them but in none of the other
// cells, then we have a "hidden pair". We can remove all other candidates from
// these two cells.
rule "hidden pair in row"
when
// Currently no setting underway
not Setting()
not Cell( freeCount == 1 )
// Establish a pair of Integer facts.
$i1: Integer()
$i2: Integer( this > $i1 )
// Look for a Cell with these two among its candidates. (The upper bound on
// the number of candidates avoids a lot of useless work during startup.)
$c1: Cell( $rn1: rowNo, $cn1: colNo, freeCount > 2 && < 9, free contains $i1 && contains $i2, $cellRow: cellRow )
// Get another one from the same row, with the same pair among its candidates.
$c2: Cell( this != $c1, cellRow == $cellRow, freeCount > 2, free contains $i1 && contains $i2 )
// Ascertain that no other cell in the group has one of these two values.
not( Cell( this != $c1 && != $c2, free contains $i1 || contains $i2 ) from $cellRow.getCells() )
then
if( explain) System.out.println( "hidden pair in row at " + $c1.posAsString() + " and " + $c2.posAsString() );
// Set the candidate lists of these two Cells to the "hidden pair".
modify( $c1 ){ blockExcept( $i1, $i2 ) }
modify( $c2 ){ blockExcept( $i1, $i2 ) }
end
rule "hidden pair in column"
when
not Setting()
not Cell( freeCount == 1 )
$i1: Integer()
$i2: Integer( this > $i1 )
$c1: Cell( $rn1: rowNo, $cn1: colNo, freeCount > 2 && < 9, free contains $i1 && contains $i2, $cellCol: cellCol )
$c2: Cell( this != $c1, cellCol == $cellCol, freeCount > 2, free contains $i1 && contains $i2 )
not( Cell( this != $c1 && != $c2, free contains $i1 || contains $i2 ) from $cellCol.getCells() )
then
if (explain) System.out.println( "hidden pair in column at " + $c1.posAsString() + " and " + $c2.posAsString() );
modify( $c1 ){ blockExcept( $i1, $i2 ) }
modify( $c2 ){ blockExcept( $i1, $i2 ) }
end
rule "hidden pair in square"
when
not Setting()
not Cell( freeCount == 1 )
$i1: Integer()
$i2: Integer( this > $i1 )
$c1: Cell( $rn1: rowNo, $cn1: colNo, freeCount > 2 && < 9, free contains $i1 && contains $i2,
$cellSqr: cellSqr )
$c2: Cell( this != $c1, cellSqr == $cellSqr, freeCount > 2, free contains $i1 && contains $i2 )
not( Cell( this != $c1 && != $c2, free contains $i1 || contains $i2 ) from $cellSqr.getCells() )
then
if (explain) System.out.println( "hidden pair in square " + $c1.posAsString() + " and " + $c2.posAsString() );
modify( $c1 ){ blockExcept( $i1, $i2 ) }
modify( $c2 ){ blockExcept( $i1, $i2 ) }
end
Two rules deal with "X-wings"
in rows and columns. When only two possible cells for a value exist in each of two different rows (or columns) and these candidates lie also in the same columns (or rows), then all other candidates for this value in the columns (or rows) can be eliminated. When you follow the pattern sequence in one of these rules, notice how the conditions that are conveniently expressed by words such as same
or only
result in patterns with suitable constraints or that are prefixed with not
.
rule "X-wings in rows"
when
not Setting()
not Cell( freeCount == 1 )
$i: Integer()
$ca1: Cell( freeCount > 1, free contains $i,
$ra: cellRow, $rano: rowNo, $c1: cellCol, $c1no: colNo )
$cb1: Cell( freeCount > 1, free contains $i,
$rb: cellRow, $rbno: rowNo > $rano, cellCol == $c1 )
not( Cell( this != $ca1 && != $cb1, free contains $i ) from $c1.getCells() )
$ca2: Cell( freeCount > 1, free contains $i,
cellRow == $ra, $c2: cellCol, $c2no: colNo > $c1no )
$cb2: Cell( freeCount > 1, free contains $i,
cellRow == $rb, cellCol == $c2 )
not( Cell( this != $ca2 && != $cb2, free contains $i ) from $c2.getCells() )
$cx: Cell( rowNo == $rano || == $rbno, colNo != $c1no && != $c2no,
freeCount > 1, free contains $i )
then
if (explain) {
System.out.println( "X-wing with " + $i + " in rows " +
$ca1.posAsString() + " - " + $cb1.posAsString() +
$ca2.posAsString() + " - " + $cb2.posAsString() + ", remove from " + $cx.posAsString() );
}
modify( $cx ){ blockValue( $i ) }
end
rule "X-wings in columns"
when
not Setting()
not Cell( freeCount == 1 )
$i: Integer()
$ca1: Cell( freeCount > 1, free contains $i,
$c1: cellCol, $c1no: colNo, $ra: cellRow, $rano: rowNo )
$ca2: Cell( freeCount > 1, free contains $i,
$c2: cellCol, $c2no: colNo > $c1no, cellRow == $ra )
not( Cell( this != $ca1 && != $ca2, free contains $i ) from $ra.getCells() )
$cb1: Cell( freeCount > 1, free contains $i,
cellCol == $c1, $rb: cellRow, $rbno: rowNo > $rano )
$cb2: Cell( freeCount > 1, free contains $i,
cellCol == $c2, cellRow == $rb )
not( Cell( this != $cb1 && != $cb2, free contains $i ) from $rb.getCells() )
$cx: Cell( colNo == $c1no || == $c2no, rowNo != $rano && != $rbno,
freeCount > 1, free contains $i )
then
if (explain) {
System.out.println( "X-wing with " + $i + " in columns " +
$ca1.posAsString() + " - " + $ca2.posAsString() +
$cb1.posAsString() + " - " + $cb2.posAsString() + ", remove from " + $cx.posAsString() );
}
modify( $cx ){ blockValue( $i ) }
end
The two rules "intersection removal …"
are based on the restricted occurrence of some number within one square, either in a single row or in a single column. This means that this number must be in one of those two or three cells of the row or column and can be removed from the candidate sets of all other cells of the group. The pattern establishes the restricted occurrence and then fires for each cell outside of the square and within the same cell file.
rule "intersection removal column"
when
not Setting()
not Cell( freeCount == 1 )
$i: Integer()
// Occurs in a Cell
$c: Cell( free contains $i, $cs: cellSqr, $cc: cellCol )
// Does not occur in another cell of the same square and a different column
not Cell( this != $c, free contains $i, cellSqr == $cs, cellCol != $cc )
// A cell exists in the same column and another square containing this value.
$cx: Cell( freeCount > 1, free contains $i, cellCol == $cc, cellSqr != $cs )
then
// Remove the value from that other cell.
if (explain) {
System.out.println( "column elimination due to " + $c.posAsString() +
": remove " + $i + " from " + $cx.posAsString() );
}
modify( $cx ){ blockValue( $i ) }
end
rule "intersection removal row"
when
not Setting()
not Cell( freeCount == 1 )
$i: Integer()
// Occurs in a Cell
$c: Cell( free contains $i, $cs: cellSqr, $cr: cellRow )
// Does not occur in another cell of the same square and a different row.
not Cell( this != $c, free contains $i, cellSqr == $cs, cellRow != $cr )
// A cell exists in the same row and another square containing this value.
$cx: Cell( freeCount > 1, free contains $i, cellRow == $cr, cellSqr != $cs )
then
// Remove the value from that other cell.
if (explain) {
System.out.println( "row elimination due to " + $c.posAsString() +
": remove " + $i + " from " + $cx.posAsString() );
}
modify( $cx ){ blockValue( $i ) }
end
These rules are sufficient for many but not all Sudoku puzzles. To solve very difficult grids, the rule set requires more complex rules. (Ultimately, some puzzles can be solved only by trial and error.)
23.10. Conway’s Game of Life example decisions (ruleflow groups and GUI integration)
The Conway’s Game of Life example decision set, based on the famous cellular automaton by John Conway, demonstrates how to use ruleflow groups in rules to control rule execution. The example also demonstrates how to integrate Drools rules with a graphical user interface (GUI), in this case a Swing-based implementation of Conway’s Game of Life.
The following is an overview of the Conway’s Game of Life (Conway) example:
-
Name:
conway
-
Main classes:
org.drools.examples.conway.ConwayRuleFlowGroupRun
,org.drools.examples.conway.ConwayAgendaGroupRun
(insrc/main/java
) -
Module:
droolsjbpm-integration-examples
-
Type: Java application
-
Rule files:
org.drools.examples.conway.*.drl
(insrc/main/resources
) -
Objective: Demonstrates ruleflow groups and GUI integration
The Conway’s Game of Life example is separate from most of the other example decision sets in Drools and is located in the Drools and jBPM integration repository in GitHub. |
In Conway’s Game of Life, a user interacts with the game by creating an initial configuration or an advanced pattern with defined properties and then observing how the initial state evolves. The objective of the game is to show the development of a population, generation by generation. Each generation results from the preceding one, based on the simultaneous evaluation of all cells.
The following basic rules govern what the next generation looks like:
-
If a live cell has fewer than two live neighbors, it dies of loneliness.
-
If a live cell has more than three live neighbors, it dies from overcrowding.
-
If a dead cell has exactly three live neighbors, it comes to life.
Any cell that does not meet any of those criteria is left as is for the next generation.
The Conway’s Game of Life example uses Drools rules with ruleflow-group
attributes to define the pattern implemented in the game. The example also contains a version of the decision set that achieves the same behavior using agenda groups. Agenda groups enable you to partition the Drools engine agenda to provide execution control over groups of rules. By default, all rules are in the agenda group MAIN
. You can use the agenda-group
attribute to specify a different agenda group for the rule.
This overview does not explore the version of the Conway example using agenda groups. For more information about agenda groups, see the Drools example decision sets that specifically address agenda groups.
Conway example execution and interaction
Similar to other Drools decision examples, you execute the Conway ruleflow example by running the org.drools.examples.conway.ConwayRuleFlowGroupRun
class as a Java application in your IDE.
When you execute the Conway example, the Conway’s Game of Life
GUI window appears. This window contains an empty grid, or "arena" where the life simulation takes place. Initially the grid is empty because no live cells are in the system yet.
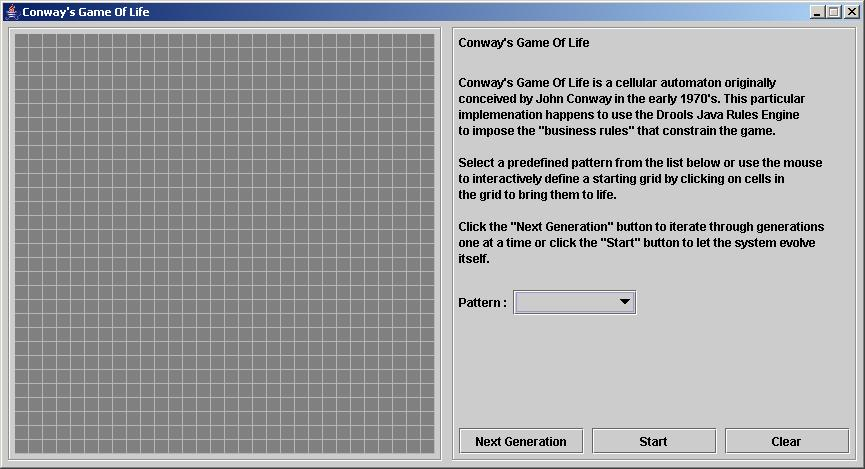
Select a predefined pattern from the Pattern drop-down menu and click Next Generation to click through each population generation. Each cell is either alive or dead, where live cells contain a green ball. As the population evolves from the initial pattern, cells live or die relative to neighboring cells, according to the rules of the game.
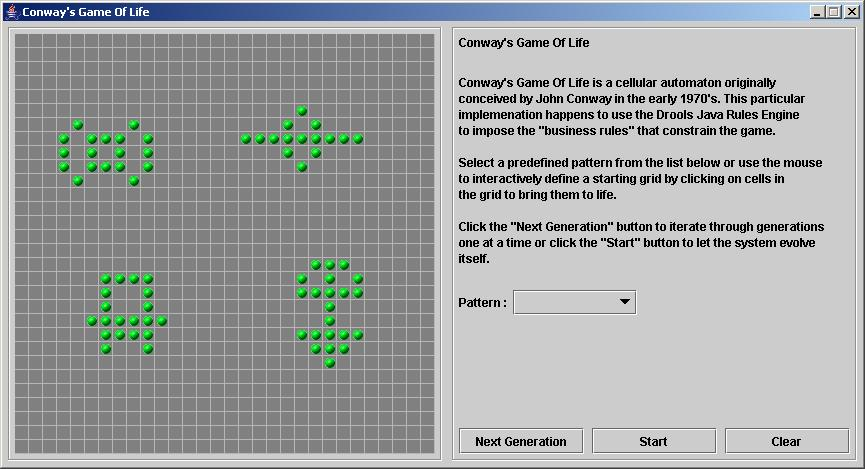
Neighbors include not only cells to the left, right, top, and bottom but also cells that are connected diagonally, so that each cell has a total of eight neighbors. Exceptions are the corner cells, which have only three neighbors, and the cells along the four borders, with five neighbors each.
You can manually intervene to create or kill cells by clicking the cell.
To run through an evolution automatically from the initial pattern, click Start.
Conway example rules with ruleflow groups
The rules in the ConwayRuleFlowGroupRun
example use ruleflow groups to control rule execution. A ruleflow group is a group of rules associated by the ruleflow-group
rule attribute. These rules can only fire when the group is activated. The group itself can only become active when the elaboration of the ruleflow diagram reaches the node representing the group.
The Conway example uses the following ruleflow groups for rules:
-
"register neighbor"
-
"evaluate"
-
"calculate"
-
"reset calculate"
-
"birth"
-
"kill"
-
"kill all"
All of the Cell
objects are inserted into the KIE session and the "register …"
rules in the ruleflow group "register neighbor"
are allowed to execute by the ruleflow process. This group of four rules creates Neighbor
relations between some cell and its northeastern, northern, northwestern, and western neighbors.
This relation is bidirectional and handles the other four directions. Border cells do not require any special treatment. These cells are not paired with neighboring cells where there is not any.
By the time all activations have fired for these rules, all cells are related to all their neighboring cells.
rule "register north east"
ruleflow-group "register neighbor"
when
$cell: Cell( $row : row, $col : col )
$northEast : Cell( row == ($row - 1), col == ( $col + 1 ) )
then
insert( new Neighbor( $cell, $northEast ) );
insert( new Neighbor( $northEast, $cell ) );
end
rule "register north"
ruleflow-group "register neighbor"
when
$cell: Cell( $row : row, $col : col )
$north : Cell( row == ($row - 1), col == $col )
then
insert( new Neighbor( $cell, $north ) );
insert( new Neighbor( $north, $cell ) );
end
rule "register north west"
ruleflow-group "register neighbor"
when
$cell: Cell( $row : row, $col : col )
$northWest : Cell( row == ($row - 1), col == ( $col - 1 ) )
then
insert( new Neighbor( $cell, $northWest ) );
insert( new Neighbor( $northWest, $cell ) );
end
rule "register west"
ruleflow-group "register neighbor"
when
$cell: Cell( $row : row, $col : col )
$west : Cell( row == $row, col == ( $col - 1 ) )
then
insert( new Neighbor( $cell, $west ) );
insert( new Neighbor( $west, $cell ) );
end
After all the cells are inserted, some Java code applies the pattern to the grid, setting certain cells to Live
. Then, when the user clicks Start or Next Generation, the example executes the Generation
ruleflow. This ruleflow manages all changes of cells in each generation cycle.
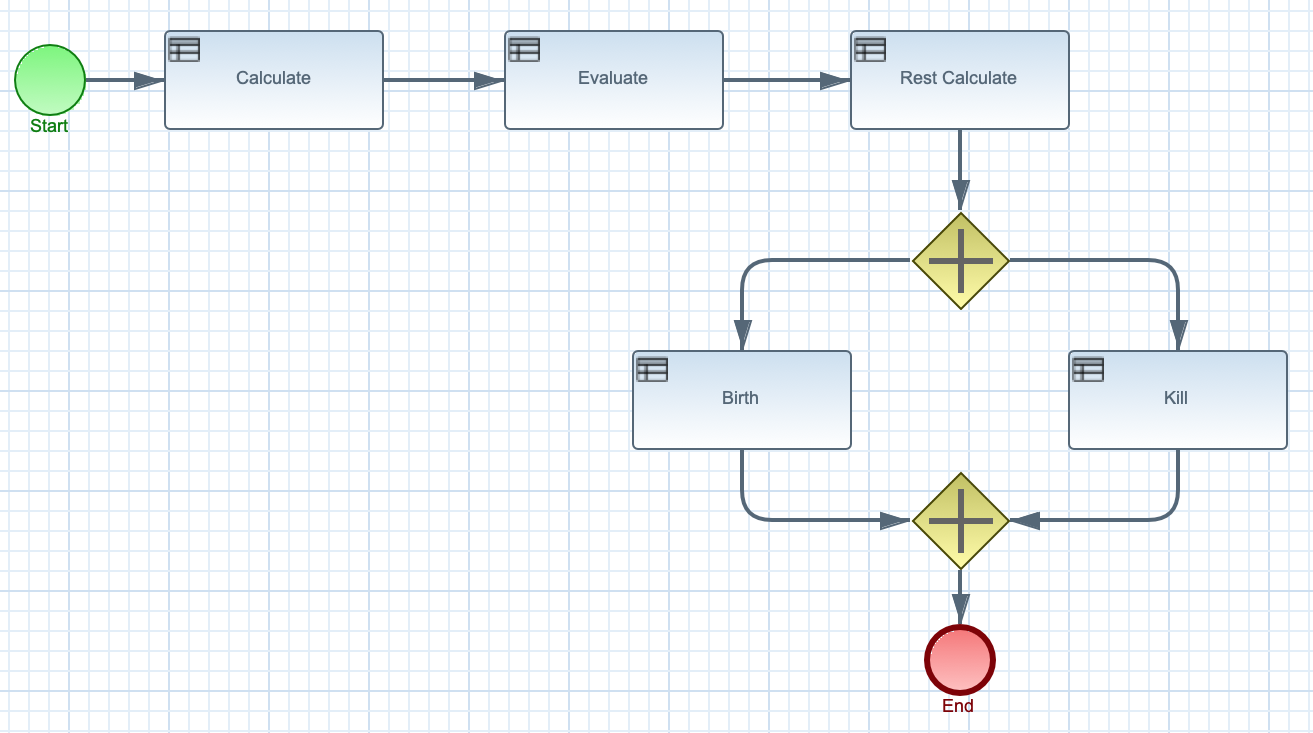
The ruleflow process enters the "evaluate"
ruleflow group and any active rules in the group can fire. The rules "Kill the …"
and "Give Birth"
in this group apply the game rules to birth or kill cells. The example uses the phase
attribute to drive the reasoning of the Cell
object by specific groups of rules. Typically, the phase is tied to a ruleflow group in the ruleflow process definition.
Notice that the example does not change the state of any Cell
objects at this point because it must complete the full evaluation before those changes can be applied. The example sets the cell to a phase
that is either Phase.KILL
or Phase.BIRTH
, which is used later to control actions applied to the Cell
object.
rule "Kill The Lonely"
ruleflow-group "evaluate"
no-loop
when
// A live cell has fewer than 2 live neighbors.
theCell: Cell( liveNeighbors < 2, cellState == CellState.LIVE,
phase == Phase.EVALUATE )
then
modify( theCell ){
setPhase( Phase.KILL );
}
end
rule "Kill The Overcrowded"
ruleflow-group "evaluate"
no-loop
when
// A live cell has more than 3 live neighbors.
theCell: Cell( liveNeighbors > 3, cellState == CellState.LIVE,
phase == Phase.EVALUATE )
then
modify( theCell ){
setPhase( Phase.KILL );
}
end
rule "Give Birth"
ruleflow-group "evaluate"
no-loop
when
// A dead cell has 3 live neighbors.
theCell: Cell( liveNeighbors == 3, cellState == CellState.DEAD,
phase == Phase.EVALUATE )
then
modify( theCell ){
theCell.setPhase( Phase.BIRTH );
}
end
After all Cell
objects in the grid have been evaluated, the example uses the "reset calculate"
rule to clear any activations in the "calculate"
ruleflow group. The example then enters a split in the ruleflow that enables the rules "kill"
and "birth"
to fire, if the ruleflow group is activated. These rules apply the state change.
rule "reset calculate"
ruleflow-group "reset calculate"
when
then
WorkingMemory wm = drools.getWorkingMemory();
wm.clearRuleFlowGroup( "calculate" );
end
rule "kill"
ruleflow-group "kill"
no-loop
when
theCell: Cell( phase == Phase.KILL )
then
modify( theCell ){
setCellState( CellState.DEAD ),
setPhase( Phase.DONE );
}
end
rule "birth"
ruleflow-group "birth"
no-loop
when
theCell: Cell( phase == Phase.BIRTH )
then
modify( theCell ){
setCellState( CellState.LIVE ),
setPhase( Phase.DONE );
}
end
At this stage, several Cell
objects have been modified with the state changed to either LIVE
or DEAD
. When a cell becomes live or dead, the example uses the Neighbor
relation in the rules "Calculate …"
to iterate over all surrounding cells, increasing or decreasing the liveNeighbor
count. Any cell that has its count changed is also set to to the EVALUATE
phase to make sure it is included in the reasoning during the evaluation stage of the ruleflow process.
After the live count has been determined and set for all cells, the ruleflow process ends. If the user initially clicked Start, the Drools engine restarts the ruleflow at that point. If the user initially clicked Next Generation, the user can request another generation.
rule "Calculate Live"
ruleflow-group "calculate"
lock-on-active
when
theCell: Cell( cellState == CellState.LIVE )
Neighbor( cell == theCell, $neighbor : neighbor )
then
modify( $neighbor ){
setLiveNeighbors( $neighbor.getLiveNeighbors() + 1 ),
setPhase( Phase.EVALUATE );
}
end
rule "Calculate Dead"
ruleflow-group "calculate"
lock-on-active
when
theCell: Cell( cellState == CellState.DEAD )
Neighbor( cell == theCell, $neighbor : neighbor )
then
modify( $neighbor ){
setLiveNeighbors( $neighbor.getLiveNeighbors() - 1 ),
setPhase( Phase.EVALUATE );
}
end
23.11. House of Doom example decisions (backward chaining and recursion)
The House of Doom example decision set demonstrates how the Drools engine uses backward chaining and recursion to reach defined goals or subgoals in a hierarchical system.
The following is an overview of the House of Doom example:
-
Name:
backwardchaining
-
Main class:
org.drools.examples.backwardchaining.HouseOfDoomMain
(insrc/main/java
) -
Module:
drools-examples
-
Type: Java application
-
Rule file:
org.drools.examples.backwardchaining.BC-Example.drl
(insrc/main/resources
) -
Objective: Demonstrates backward chaining and recursion
A backward-chaining rule system is a goal-driven system that starts with a conclusion that the Drools engine attempts to satisfy, often using recursion. If the system cannot reach the conclusion or goal, it searches for subgoals, which are conclusions that complete part of the current goal. The system continues this process until either the initial conclusion is satisfied or all subgoals are satisfied.
In contrast, a forward-chaining rule system is a data-driven system that starts with a fact in the working memory of the Drools engine and reacts to changes to that fact. When objects are inserted into working memory, any rule conditions that become true as a result of the change are scheduled for execution by the agenda.
The Drools engine in Drools uses both forward and backward chaining to evaluate rules.
The following diagram illustrates how the Drools engine evaluates rules using forward chaining overall with a backward-chaining segment in the logic flow:
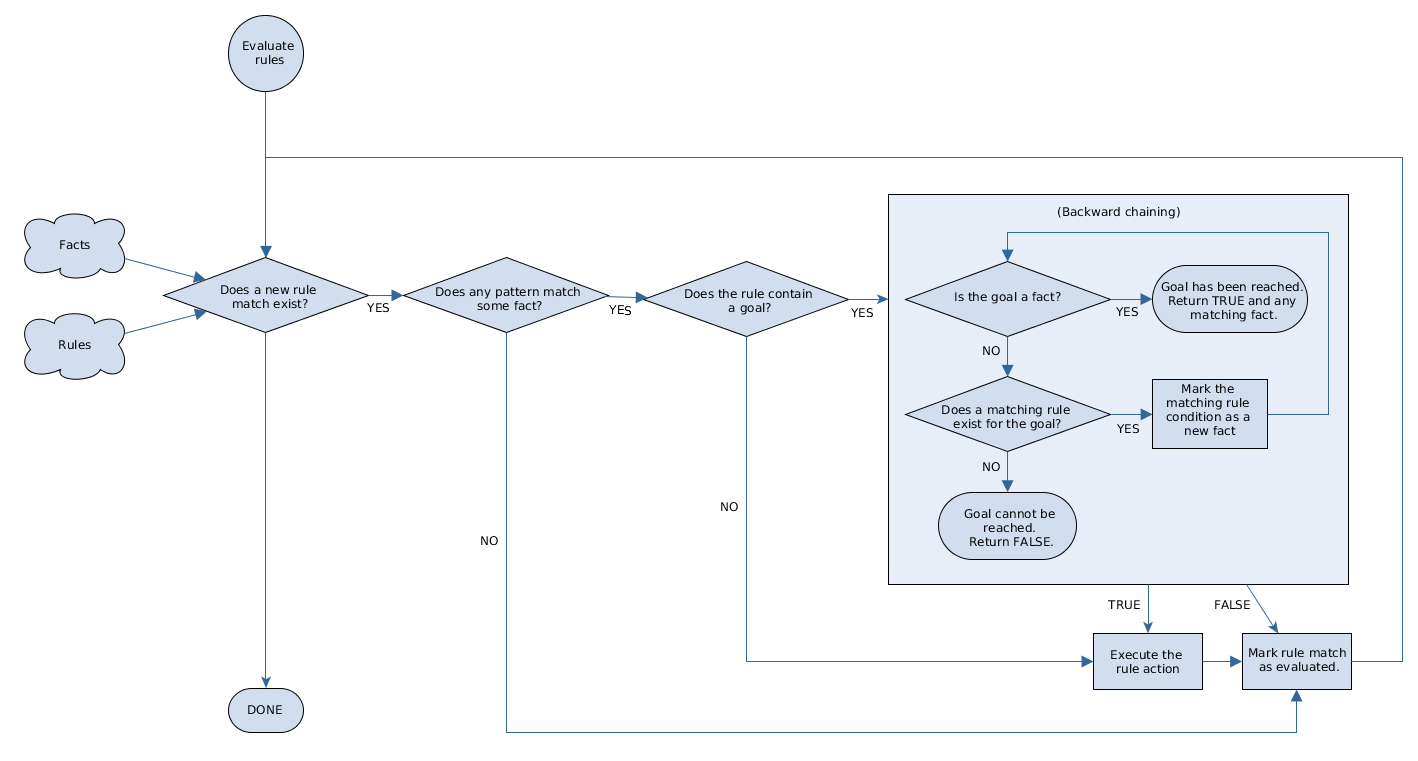
The House of Doom example uses rules with various types of queries to find the location of rooms and items within the house. The sample class Location.java
contains the item
and location
elements used in the example. The sample class HouseOfDoomMain.java
inserts the items or rooms in their respective locations in the house and executes the rules.
ksession.insert( new Location("Office", "House") );
ksession.insert( new Location("Kitchen", "House") );
ksession.insert( new Location("Knife", "Kitchen") );
ksession.insert( new Location("Cheese", "Kitchen") );
ksession.insert( new Location("Desk", "Office") );
ksession.insert( new Location("Chair", "Office") );
ksession.insert( new Location("Computer", "Desk") );
ksession.insert( new Location("Drawer", "Desk") );
The example rules rely on backward chaining and recursion to determine the location of all items and rooms in the house structure.
The following diagram illustrates the structure of the House of Doom and the items and rooms within it:
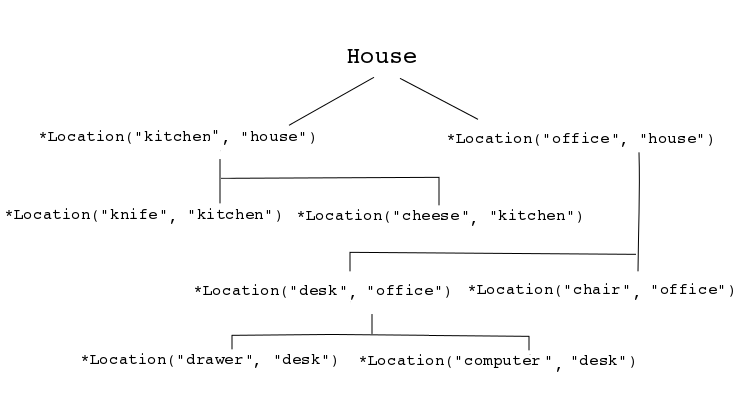
To execute the example, run the org.drools.examples.backwardchaining.HouseOfDoomMain
class as a Java application in your IDE.
After the execution, the following output appears in the IDE console window:
go1
Office is in the House
---
go2
Drawer is in the House
---
go3
---
Key is in the Office
---
go4
Chair is in the Office
Desk is in the Office
Key is in the Office
Computer is in the Office
Drawer is in the Office
---
go5
Chair is in Office
Desk is in Office
Drawer is in Desk
Key is in Drawer
Kitchen is in House
Cheese is in Kitchen
Knife is in Kitchen
Computer is in Desk
Office is in House
Key is in Office
Drawer is in House
Computer is in House
Key is in House
Desk is in House
Chair is in House
Knife is in House
Cheese is in House
Computer is in Office
Drawer is in Office
Key is in Desk
All rules in the example have fired to detect the location of all items in the house and to print the location of each in the output.
Recursive query and related rules
A recursive query repeatedly searches through the hierarchy of a data structure for relationships between elements.
In the House of Doom example, the BC-Example.drl
file contains an isContainedIn
query that most of the rules in the example use to recursively evaluate the house data structure for data inserted into the Drools engine:
query isContainedIn( String x, String y )
Location( x, y; )
or
( Location( z, y; ) and isContainedIn( x, z; ) )
end
The rule "go"
prints every string inserted into the system to determine how items are implemented, and the rule "go1"
calls the query isContainedIn
:
rule "go" salience 10
when
$s : String( )
then
System.out.println( $s );
end
rule "go1"
when
String( this == "go1" )
isContainedIn("Office", "House"; )
then
System.out.println( "Office is in the House" );
end
The example inserts the "go1"
string into the Drools engine and activates the "go1"
rule to detect that item Office
is in the location House
:
ksession.insert( "go1" );
ksession.fireAllRules();
go1
Office is in the House
Transitive closure rule
Transitive closure is a relationship between an element contained in a parent element that is multiple levels higher in a hierarchical structure.
The rule "go2"
identifies the transitive closure relationship of the Drawer
and the House
: The Drawer
is in the Desk
in the Office
in the House
.
rule "go2"
when
String( this == "go2" )
isContainedIn("Drawer", "House"; )
then
System.out.println( "Drawer is in the House" );
end
The example inserts the "go2"
string into the Drools engine and activates the "go2"
rule to detect that item Drawer
is ultimately within the location House
:
ksession.insert( "go2" );
ksession.fireAllRules();
go2
Drawer is in the House
The Drools engine determines this outcome based on the following logic:
-
The query recursively searches through several levels in the house to detect the transitive closure between
Drawer
andHouse
. -
Instead of using
Location( x, y; )
, the query uses the value of(z, y; )
becauseDrawer
is not directly inHouse
. -
The
z
argument is currently unbound, which means it has no value and returns everything that is in the argument. -
The
y
argument is currently bound toHouse
, soz
returnsOffice
andKitchen
. -
The query gathers information from the
Office
and checks recursively if theDrawer
is in theOffice
. The query lineisContainedIn( x, z; )
is called for these parameters. -
No instance of
Drawer
exists directly inOffice
, so no match is found. -
With
z
unbound, the query returns data within theOffice
and determines that z == Desk.isContainedIn(x==drawer, z==desk)
-
The
isContainedIn
query recursively searches three times, and on the third time, the query detects an instance ofDrawer
inDesk
.Location(x==drawer, y==desk)
-
After this match on the first location, the query recursively searches back up the structure to determine that the
Drawer
is in theDesk
, theDesk
is in theOffice
, and theOffice
is in theHouse
. Therefore, theDrawer
is in theHouse
and the rule is satisfied.
Reactive query rule
A reactive query searches through the hierarchy of a data structure for relationships between elements and is dynamically updated when elements in the structure are modified.
The rule "go3"
functions as a reactive query that detects if a new item Key
ever becomes present in the Office
by transitive closure: A Key
in the Drawer
in the Office
.
rule "go3"
when
String( this == "go3" )
isContainedIn("Key", "Office"; )
then
System.out.println( "Key is in the Office" );
end
The example inserts the "go3"
string into the Drools engine and activates the "go3"
rule. Initially, this rule is not satisfied because no item Key
exists in the house structure, so the rule produces no output.
ksession.insert( "go3" );
ksession.fireAllRules();
go3
The example then inserts a new item Key
in the location Drawer
, which is in Office
. This change satisfies the transitive closure in the "go3"
rule and the output is populated accordingly.
ksession.insert( new Location("Key", "Drawer") );
ksession.fireAllRules();
Key is in the Office
This change also adds another level in the structure that the query includes in subsequent recursive searches.
Queries with unbound arguments in rules
A query with one or more unbound arguments returns all undefined (unbound) items within a defined (bound) argument of the query. If all arguments in a query are unbound, then the query returns all items within the scope of the query.
The rule "go4"
uses an unbound argument thing
to search for all items within the bound argument Office
, instead of using a bound argument to search for a specific item in the Office
:
rule "go4"
when
String( this == "go4" )
isContainedIn(thing, "Office"; )
then
System.out.println( thing + "is in the Office" );
end
The example inserts the "go4"
string into the Drools engine and activates the "go4"
rule to return all items in the Office
:
ksession.insert( "go4" );
ksession.fireAllRules();
go4
Chair is in the Office
Desk is in the Office
Key is in the Office
Computer is in the Office
Drawer is in the Office
The rule "go5"
uses both unbound arguments thing
and location
to search for all items and their locations in the entire House
data structure:
rule "go5"
when
String( this == "go5" )
isContainedIn(thing, location; )
then
System.out.println(thing + " is in " + location );
end
The example inserts the "go5"
string into the Drools engine and activates the "go5"
rule to return all items and their locations in the House
data structure:
ksession.insert( "go5" );
ksession.fireAllRules();
go5
Chair is in Office
Desk is in Office
Drawer is in Desk
Key is in Drawer
Kitchen is in House
Cheese is in Kitchen
Knife is in Kitchen
Computer is in Desk
Office is in House
Key is in Office
Drawer is in House
Computer is in House
Key is in House
Desk is in House
Chair is in House
Knife is in House
Cheese is in House
Computer is in Office
Drawer is in Office
Key is in Desk
Drools Release Notes
24. Release Notes
24.1. What is New and Noteworthy in KIE Workbench 7.23.0
24.1.1. Enhanced BC collaboration features
The following enhancements were added to Business Central to provide additional options for managing access to spaces and projects.
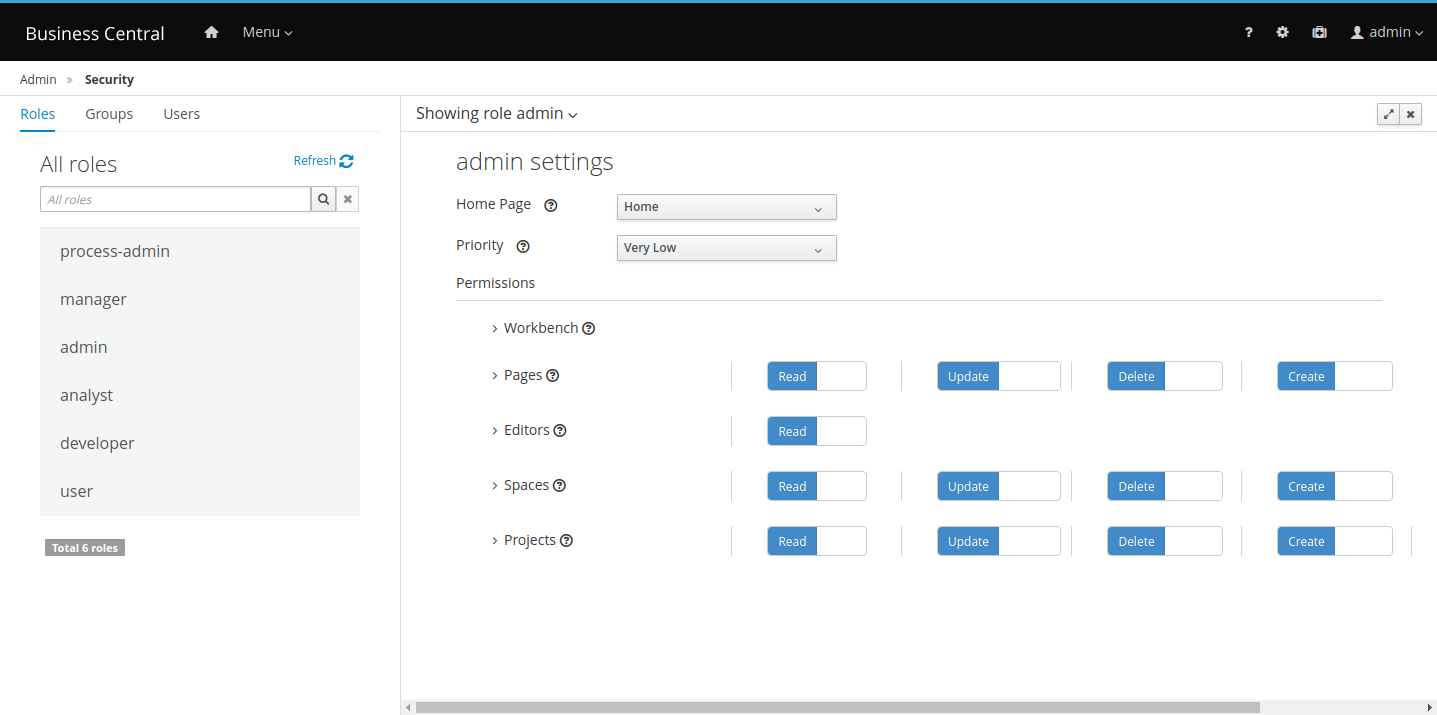
With the introduction of this new feature, it is now possible to manage spaces and projects permissions directly in their respective screens, using the Contributors tab. When contributors are added to a space, they are able to open it and see its projects and other information available. Based on their contributor role, they also have the following permissions granted:
-
Owner: Update contributors, delete spaces, create and delete projects
-
Admin: Update contributors (except owners) and create projects
-
Contributor: Create projects
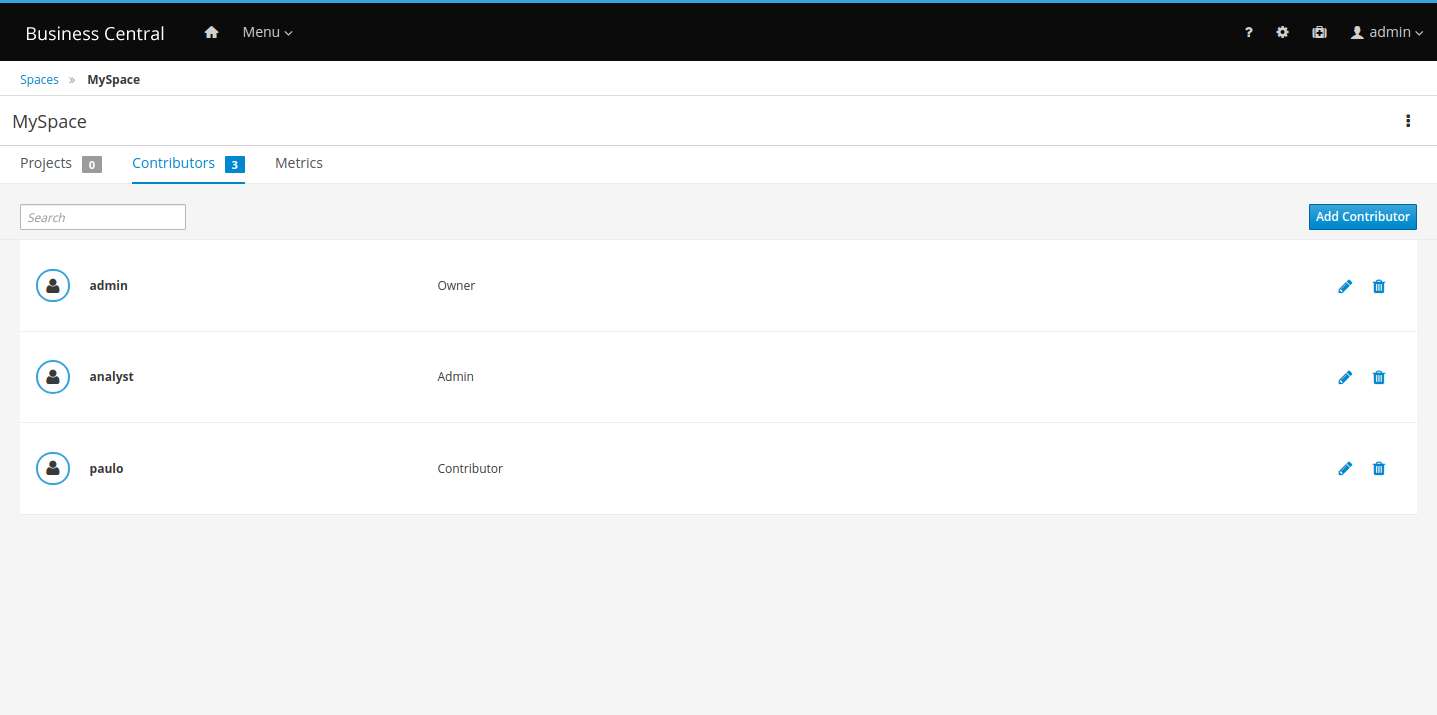
When a project is created inside a space, its contributors are copied from the space and the project creator becomes the owner of the new project. It is also possible to add new contributors to the project if they are also contributors to the project’s space. Contributors can view the project, and depending on their role, they may also have the following permissions:
-
Owner: View, update, build, deploy, and delete projects
-
Admin: View, update, build and deploy projects
-
Contributor: View, update and build projects
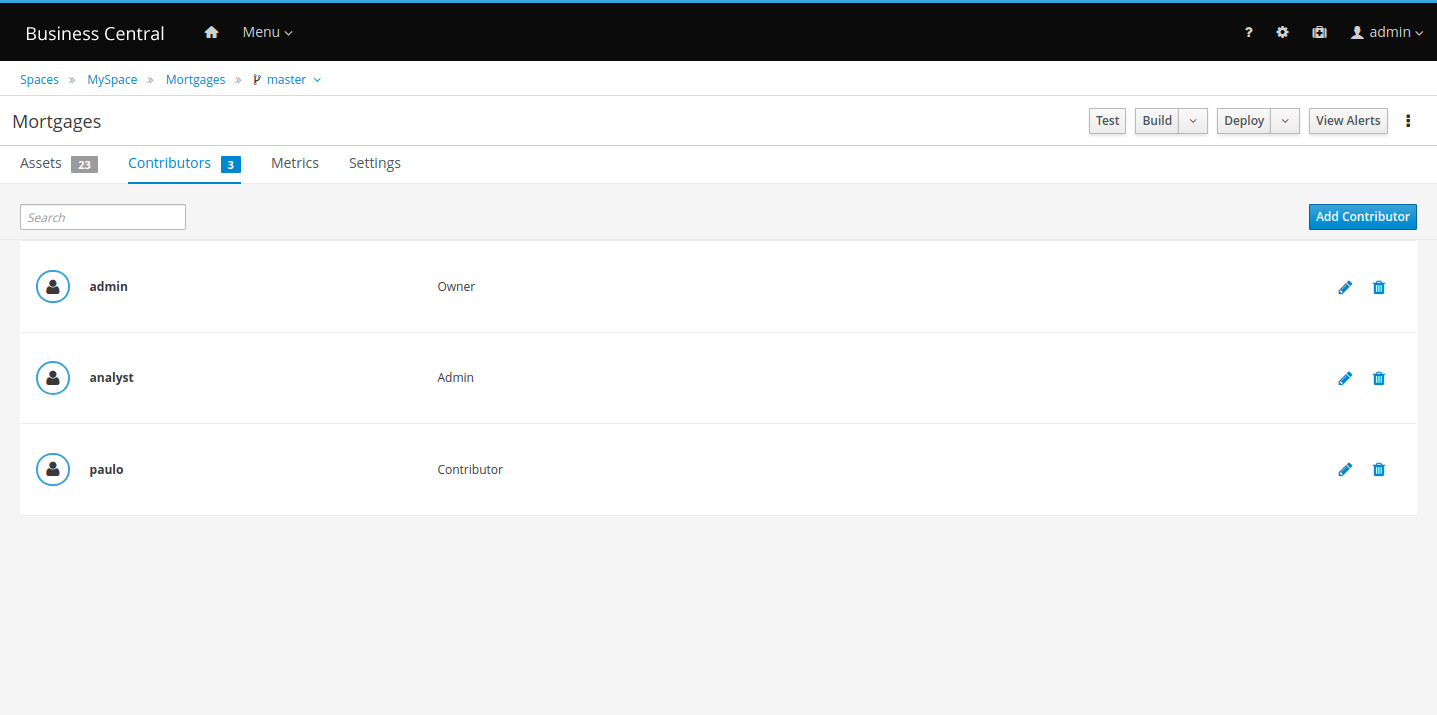
The security check uses both the Security Management user interface and Contributors tab to assign permissions to spaces and projects. For example, users can delete a space if they are assigned to a role with the required permissions or is a owner of that space.
24.1.2. Role based access control for branches
In addition to the new collaboration features, you can customize contributor role permissions for each branch of a project.
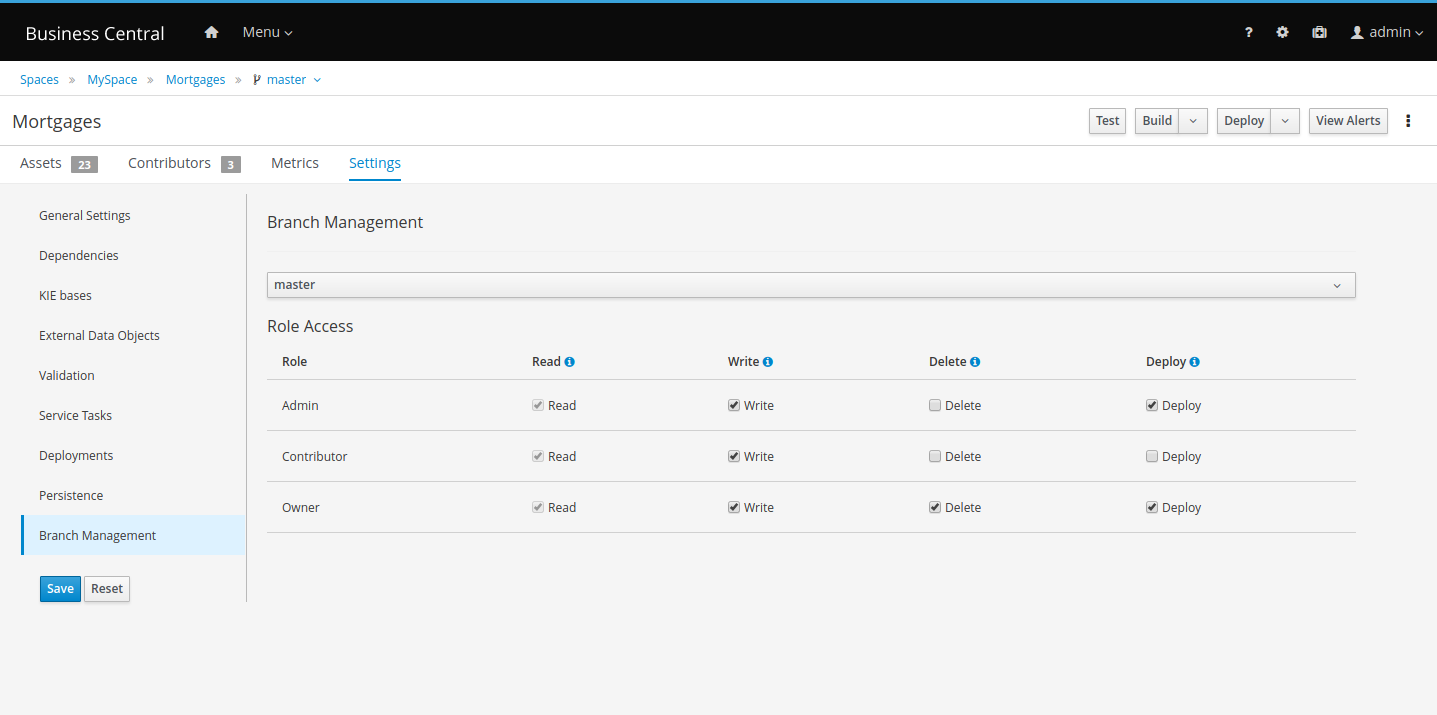
Select which permissions each contributor role has for the selected branch.
24.1.3. Importing a subset of branches
When importing projects from a repository, you can select only the branches that you want to persist in Business Central.
-
In Business Central, click Menu → Design → Projects.
-
Select or create the space into which you want to import the project. The default space is MySpace.
-
Click the three dots on the right side of the screen and select Import Project.
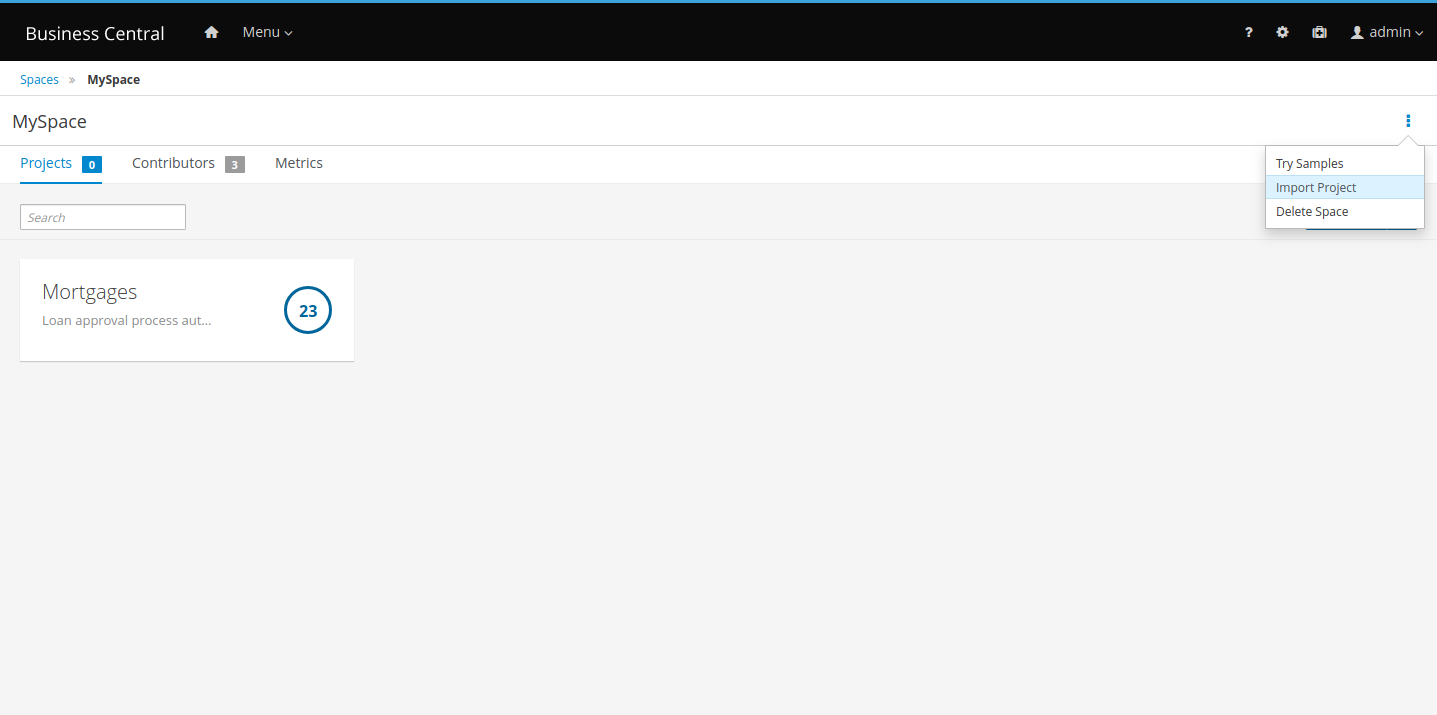
In the Import Project window, enter the URL and credentials for the Git repository that contains the project that you want to import and click Import.
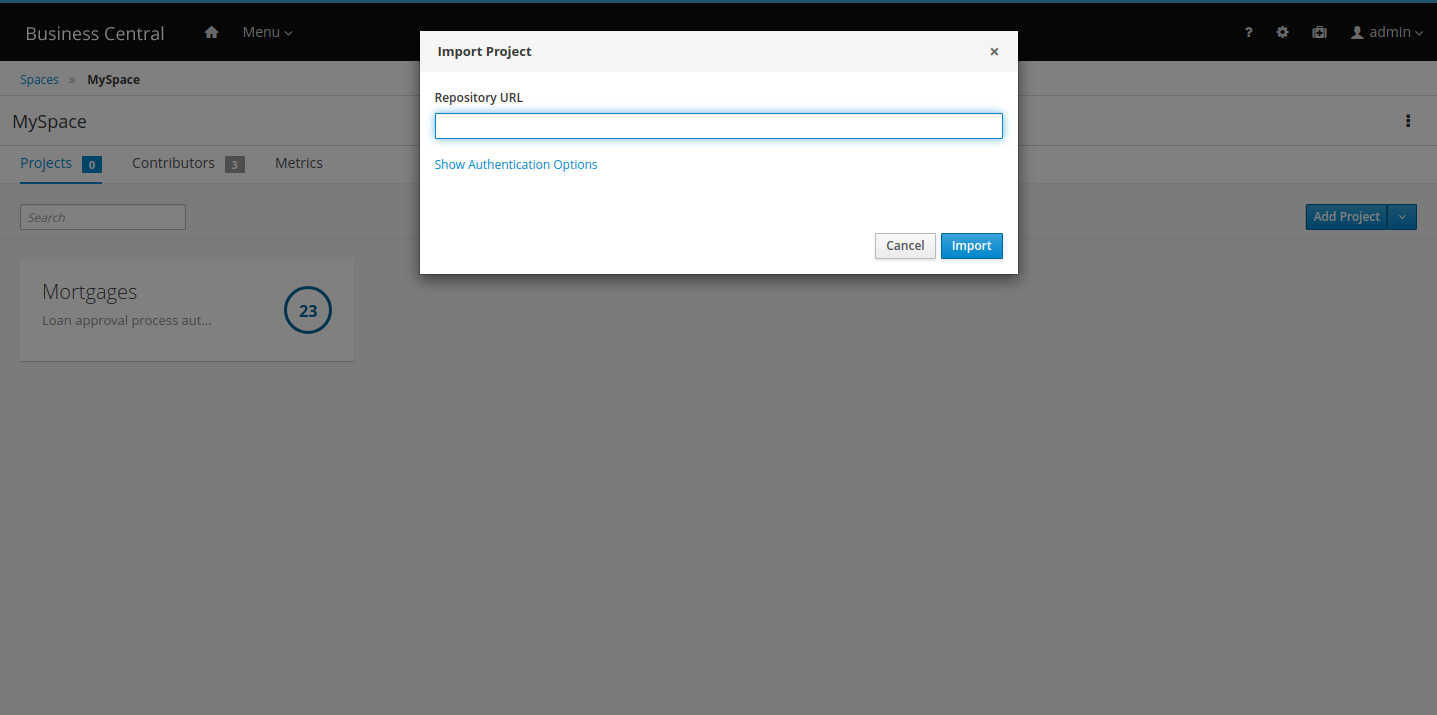
After clicking on Import, all projects found in that repository will be listed:
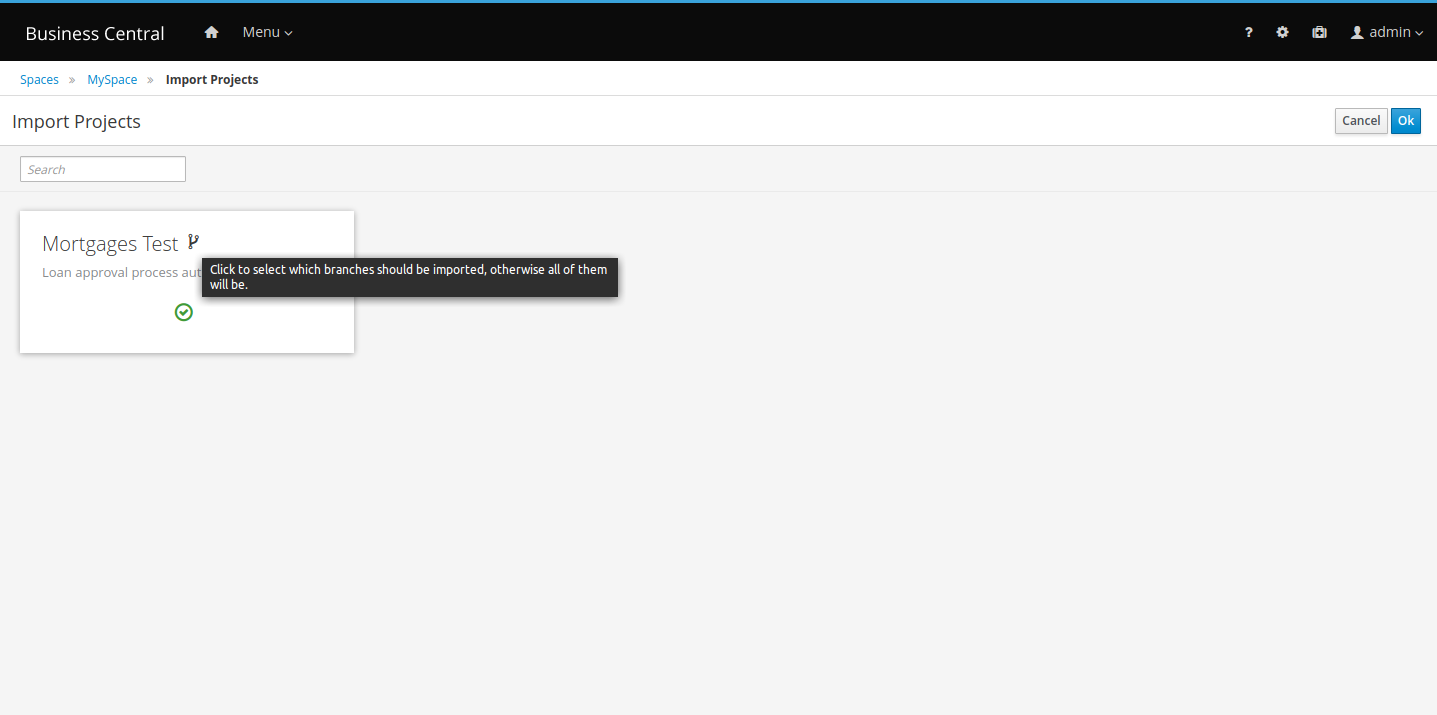
On the right side of each project name, click on the branch icon. Select the branches that you want to import.
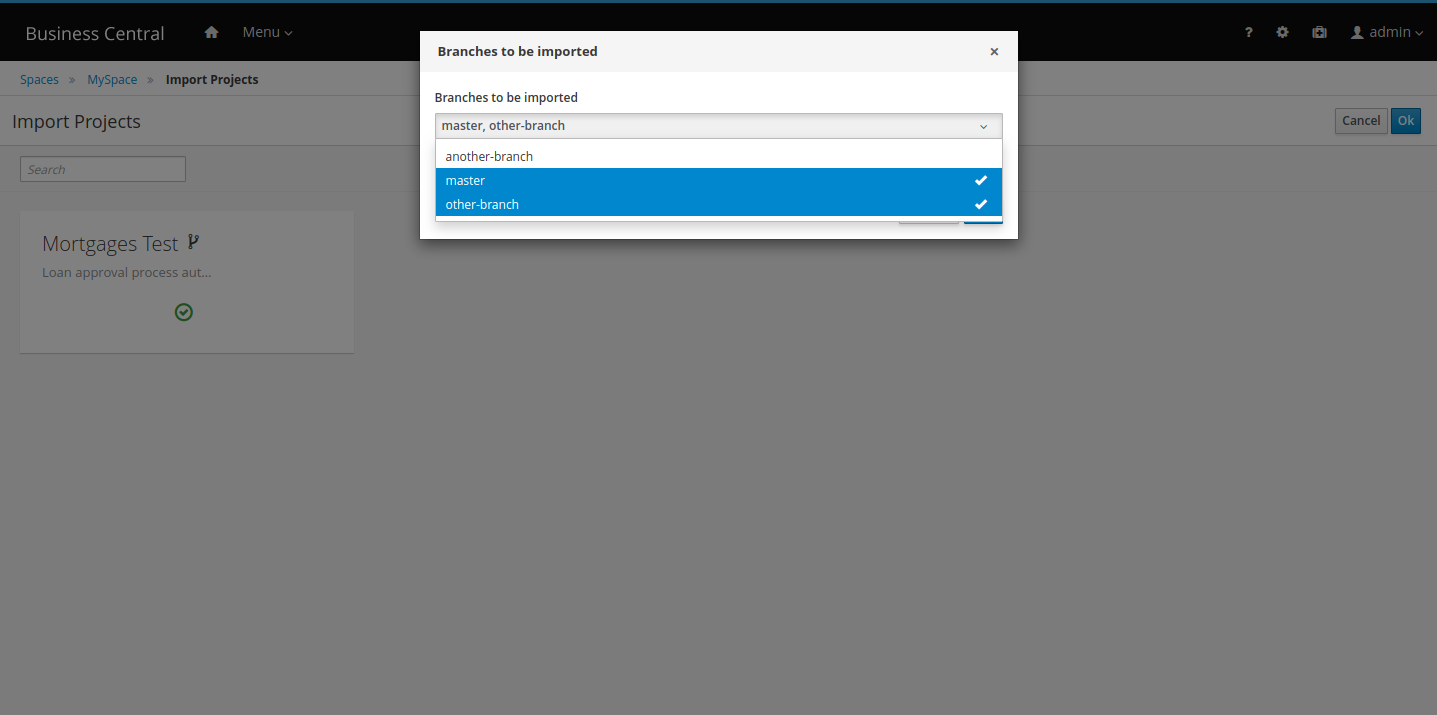
Only the selected branches are persisted:
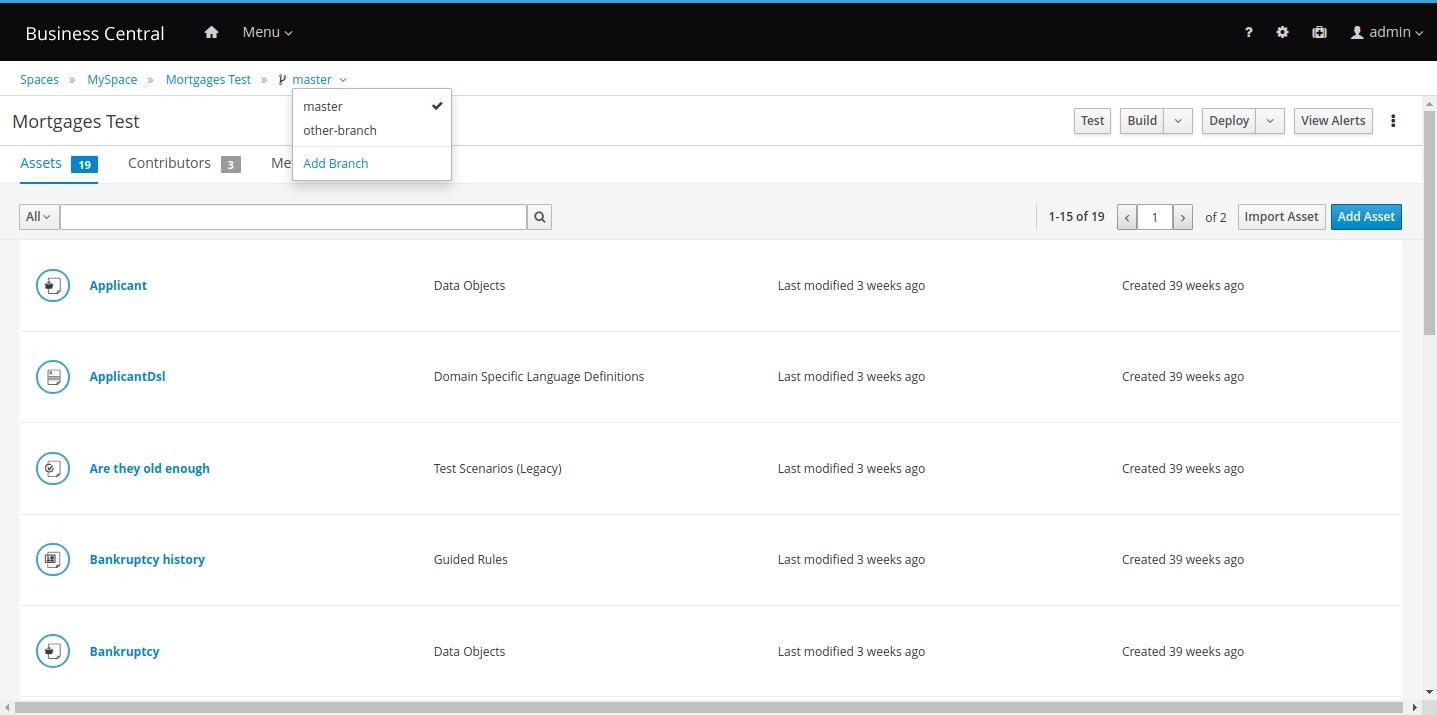
24.2. What is New and Noteworthy in KIE Workbench 7.19.0
24.2.1. DMN Automatic Layout
Nodes in DMN decision requirements diagrams (DRDs) can be positioned automatically with the new Perform automatic layout toolbar button. This automatic layout feature orients DRD nodes vertically, from the bottom to the top of the DRD. However, automatic layout currently does not support Decision Service nodes.
24.2.2. DMN and Test Scenario context menu keyboard control
In both DMN and Test Scenario tables, keyboard support for the context menu invocation was added. You can now invoke a context menu by typing Ctrl+Space.
24.3. What is New and Noteworthy in Drools 7.18
24.3.1. New Builder Options
24.3.1.1. TrimCellsInDTableOption
By default all the value of the cells in decision tables are trimmed before being processed. There could be situations where this automatic trimming is not required or even detrimental. In this cases it is now possible to disable the trimming using the new TrimCellsInDTableOption or the correspondent "drools.trimCellsInDTable" System property.
24.3.2. GroupDRLsInKieBasesByFolderOption
By default all the Drools artifacts under the resources folder, at any level, are included into the KieBase. From now on, the packages attribute of the kmodule.xml allows to limit the artifacts that will be compiled in this KieBase to only the ones belonging to the list of packages. However older versions of Drools actually checked the folder name instead of the package one. The new GroupDRLsInKieBasesByFolderOption or the correspondent "drools.groupDRLsInKieBasesByFolder" System property allows to re-enable that old folder-based behaviour.
24.4. What is New and Noteworthy in KIE Workbench 7.18.0
24.4.1. Guided rules designer filtering
The guided rules designer now supports filtering of DSL (domain specific language) files and Fact Types when you add new Condition or Action elements.
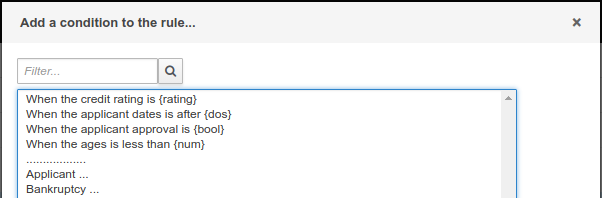
24.4.2. Test Scenario (Preview) renamed to Test Scenario and List/Map support
Old Test Scenario has been renamed as Test Scenario (Legacy) and Test Scenario (Preview) now is Test Scenario. The editor now supports also List and Map as supported data types for testing.
24.4.3. Development streamline lifecycle
Along with the new development mode on KIE Server, Business Central also adds a simplified deployment mechanism for SNAPSHOT modules to improve the user experience during the development and testing phases of a module. Some of the changes introduced:
-
More flexible deployment policy, allowing you to run module updates with no need of undeploying previous deployments.
-
Once a SNAPSHOT module is deployed, Business Central will store the user deployment preferences making subsequent deployments update the previously deployed container. This mechanism also keeps the active process instances.
-
Added abilitiy to redeploy a module, updating the container with latest changes but aborting the active process instances.
Other changes introduced:
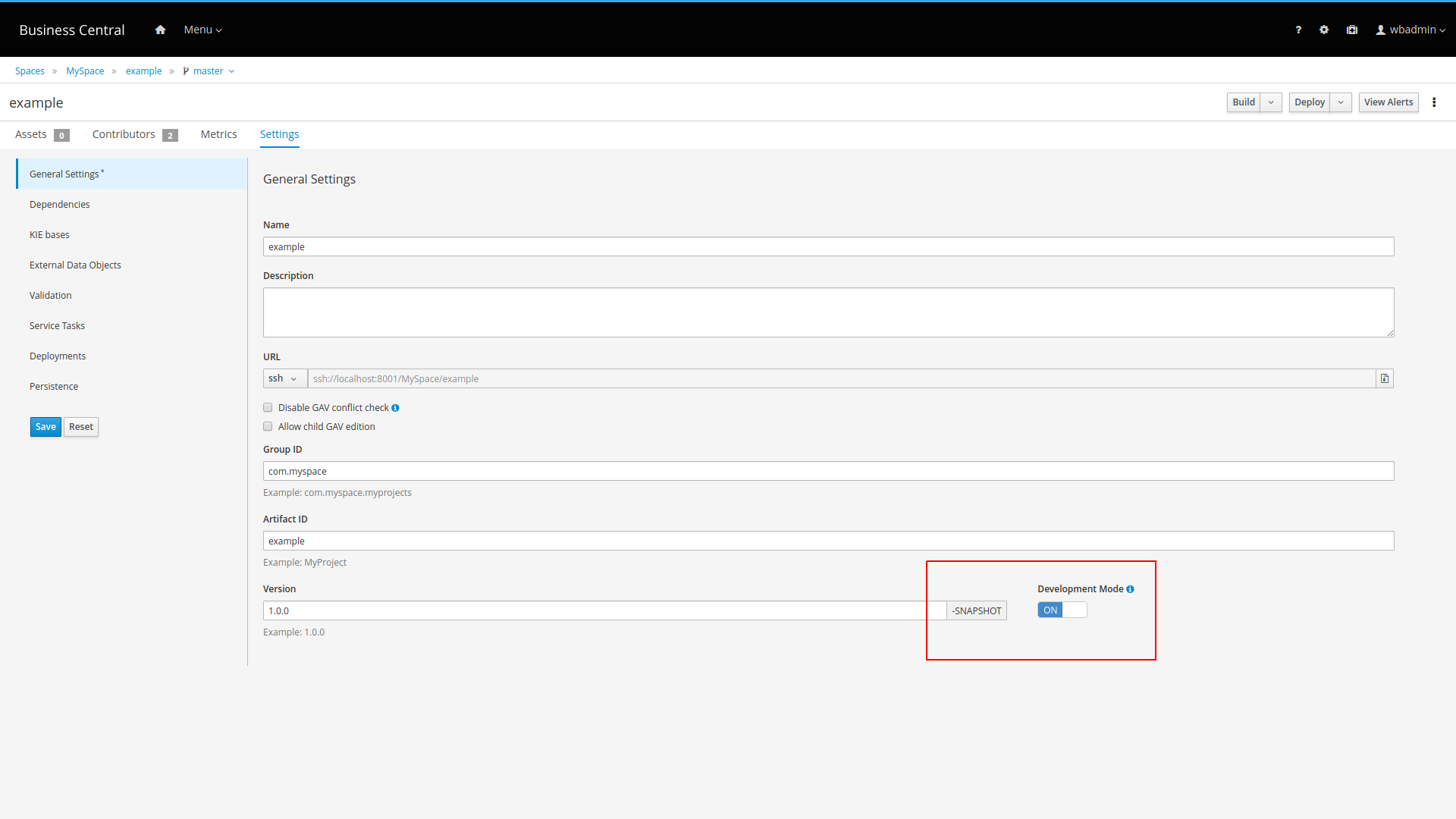
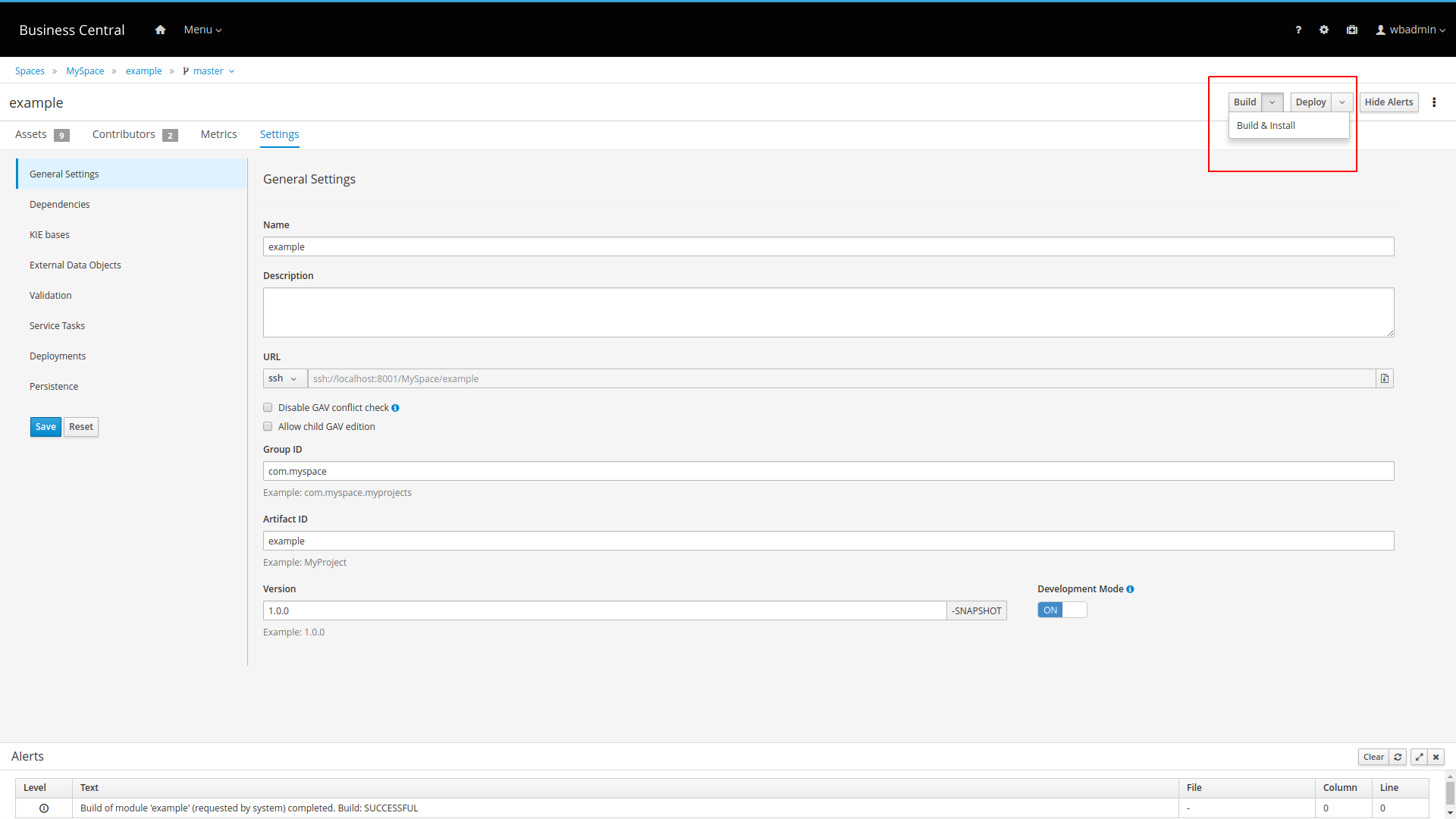
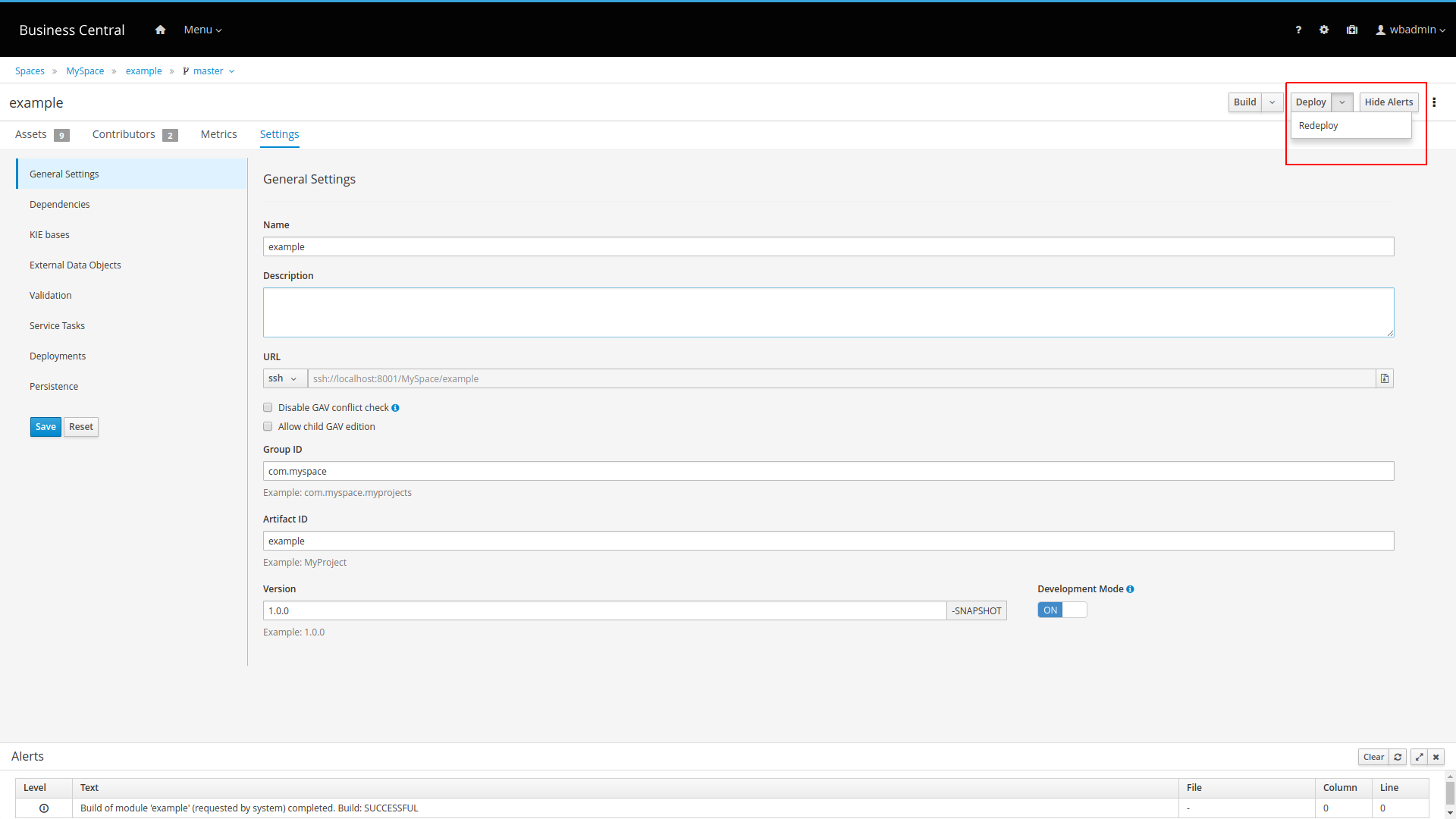
24.5. What is New and Noteworthy in Drools 7.17
24.5.1. Minor changes in DMN FEEL built-in functions' parameter names
Previous releases of Drools offered (optionally) a FEEL built-in function split()
as an extended function, beyond the DMN v1.1 specification.
In order to align with version v1.2 of the DMN specification, which now includes split()
as part of the standardised FEEL built-in functions set, the function parameter names have been aligned to the ones mandated by the DMN specification itself, with the signature split( string, delimiter )
as specified in Table 68 of the DMN v1.2 specification document.
24.6. What is New and Noteworthy in KIE Workbench 7.17.0
24.6.1. Test Scenario (Preview) enabled by default
Test Scenario (Preview) is now enabled by default so there is no additional configuration needed.
24.6.2. Test Scenario DMN support
Test Scenario (Preview) now support DMN model testing
24.6.3. DMN Decision Service support
A DMN decision service node is now available in the DMN designer palette in Business Central.
24.7. What is New and Noteworthy in KIE Workbench 7.16.0
24.7.1. DMN and Test Scenario keyboard control
For both DMN and Test Scenario tables, the following keyboard control support was added:
-
Table navigation: After you select a cell in a DMN or Test Scenario table, you can use the arrow keys to navigate between other cells. The way you select the first cell differs in DMN and Test Scenario tables:
-
In a DMN table, the top-left cell is selected by default. Next to standard navigation, press Enter to select a nested expression and Esc to return to the parent expression.
-
In a Test Scenario table, no cell is selected by default. Press Shift+Home to select the first available cell.
-
-
Table editing: After you select a cell in a DMN or Test Scenario table, you can input a new value into the cell or change the already added value. To edit a cell, press Enter. To stop the editing, press Shift+Tab.
24.8. What is New and Noteworthy in Drools 7.15
24.8.1. Broken DMN resources detected in Drools project builds
When a Drools project (KJAR file) contains an invalid or a broken resource, the project fails to build and reports the failure in one or more error messages. Previously, a Drools project with an invalid DMN resource could build successfully, with no error messages. With this release, this issue has been resolved so that an invalid DMN resource now causes a project build failure with error messaging, as expected (ref: DROOLS-3335).
For more information about project packaging and deployment, see Build, Deploy, Utilize and Run.
For a custom programmatic build, you can use the following code snippet to retrieve a list of build errors:
KieServices ks = KieServices.Factory.get();
KieFileSystem kfs = ks.newKieFileSystem()
.generateAndWritePomXML(releaseId)
.write(dmnResource);
KieBuilder kieBuilder = ks.newKieBuilder(kfs)
.buildAll();
Results results = kieBuilder.getResults();
if (results.hasMessages(Message.Level.ERROR)) {
throw new IllegalStateException(results.getMessages(Message.Level.ERROR).toString());
}
24.8.2. New method for creating a knowledge run time
The preferred way to create a knowledge run time (for example, DMNRuntime
) is no longer through a KieSession
instance, but rather through the new KieRuntimeFactory
API:
DMNRuntime rt = KieRuntimeFactory.of(kieContainer.getKieBase()).get(DMNRuntime.class);
24.9. New and Noteworthy in KIE Workbench 7.15.0
24.9.1. Git hooks notifications
Improved git hooks integration to provide feedback notifications to the user with customized messages.
24.9.2. KIE Workbench Consolidation
KIE Workbench is now called Business Central and it is available on business-central
web context.
Profiles provide a set of Business Central features and you can choose a profile based on your requirements.
The FULL
profile is a default profile that includes all features. The PLANNER_AND_RULES
profile includes only drools-kie-wb features. You can select a profile either by using the org.kie.workbench.profile
system property (possible values are FULL or PLANNER_AND_RULES) or from the Profile option in the Administration screen.
24.10. What is New and Noteworthy in Drools 7.14
24.10.1. File-system based KieScanner
The KieScanner
allows continuous monitoring of your Maven repository to check whether a new release of a Kie project has been installed
and if so it live updates the running KieContainer
with the newer version of that project. However in many cases installing a maven
repository is impractical especially in production environment. For this reason it is now possible to have a KieScanner
that works
by simply fetching update from a folder of a plain file system. You can create and start such a KieScanner
as simply as
KieServices kieServices = KieServices.Factory.get();
KieScanner kScanner = kieServices.newKieScanner( kContainer, "/myrepo/kjars" );
// Start the KieScanner polling the Maven repository every 10 seconds
kScanner.start( 10000L );
where "/myrepo/kjars" will be the folder where the KieScanner
will look for kjar updates. The jar files placed in this folder
have to follow the maven convention and then have to be a name in the form {artifactId}-{versionId}.jar
24.10.2. Support for executable models in DMN projects
You can now use the kie-maven-plugin
build component to generate DMN executable model classes and compile them in a Drools project (kjar
). This support enables DMN decision table logic in DMN projects to be evaluated more efficiently.
To enable executable models in DMN projects, add the required kie-dmn-core
dependency in the pom.xml
file:
<dependency>
<groupId>org.kie</groupId>
<artifactId>kie-dmn-core</artifactId>
<scope>provided</scope>
</dependency>
To build a DMN project enabling executable model compilation, navigate to your Maven project directory in a command terminal and run the following command:
mvn clean install -DgenerateDMNModel=yes
Alternatively, you can define the property directly in the pom.xml
file:
<project>
...
<properties>
<generateDMNModel>yes</generateDMNModel>
</properties>
...
</project>
For more information about configuring executable models for your Maven or Java project, see Executable rule models.
24.11. New and Noteworthy in KIE Workbench 7.14.0
24.11.1. SSH and Git Daemon Port Assignment Changes
If the SSH or Git daemon default or assigned ports are already in use, a new port is automatically selected. Ensure that the ports are available and check the log for more information.
Before this change, the application used to fail to start.
24.12. New and Noteworthy in KIE Workbench 7.13.0
24.12.1. Test Scenarios (Preview) editor
This version contains the preview of the new Test Scenarios editor that tests a rule with a completely new user experience.
See section Test scenarios for details on enabling and using the editor.
24.12.2. Experimental Features support
New Experimental Features Framework added to the Workbench. It provides an easy mechanism for users to preview features which are not part of the product but might be interesting for them (for example, ongoing developments, tech previews, POCs…).
See section Experimental Features Framework for more details.
24.12.3. SSH keystore
In order to provide the Workbench VFS with proper SSH authentication, a new keystore must added. This keystore enables users to register their SSH public keys.
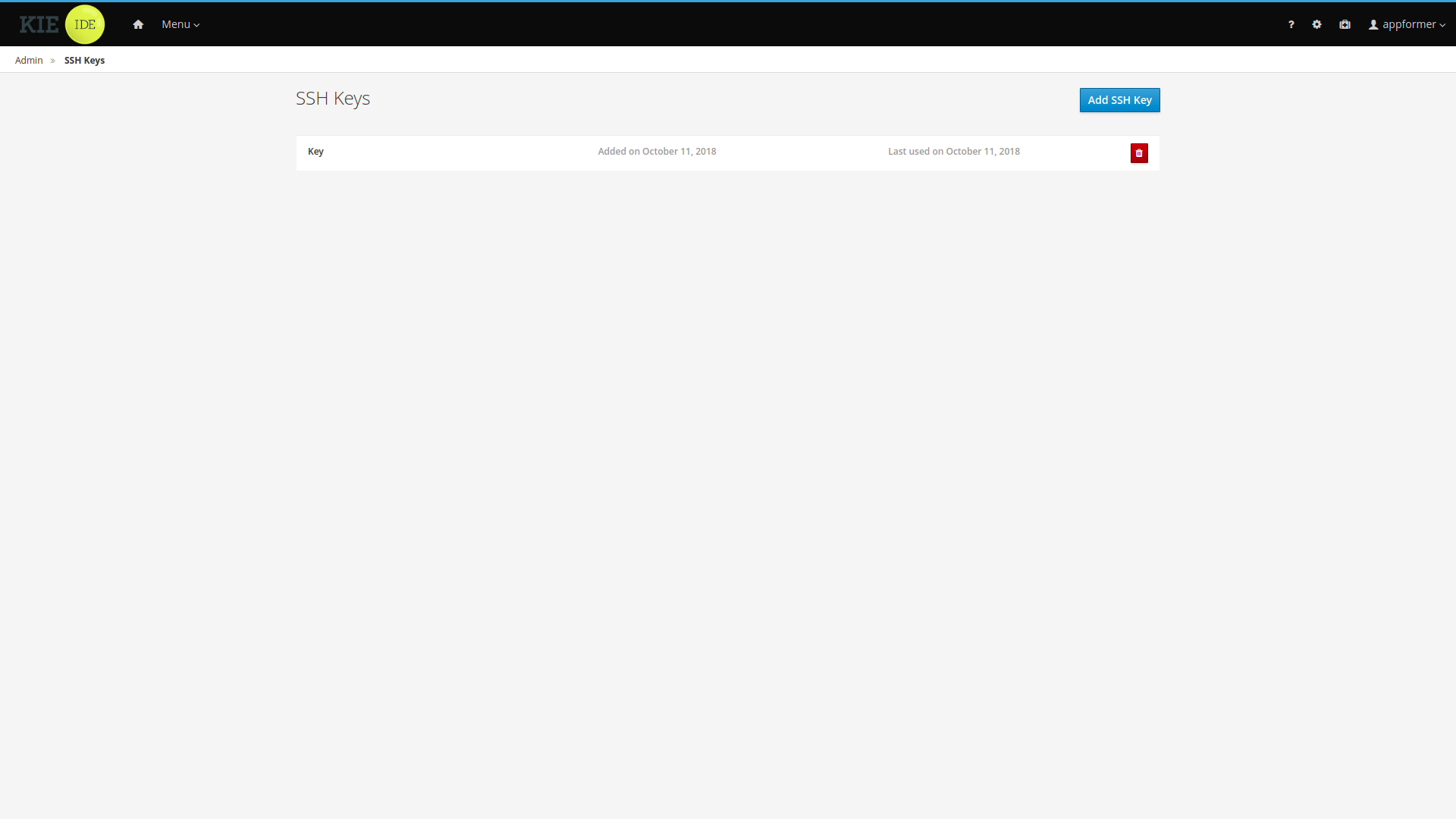
You can access it from the Admin page using the new SSH Keys menu option.
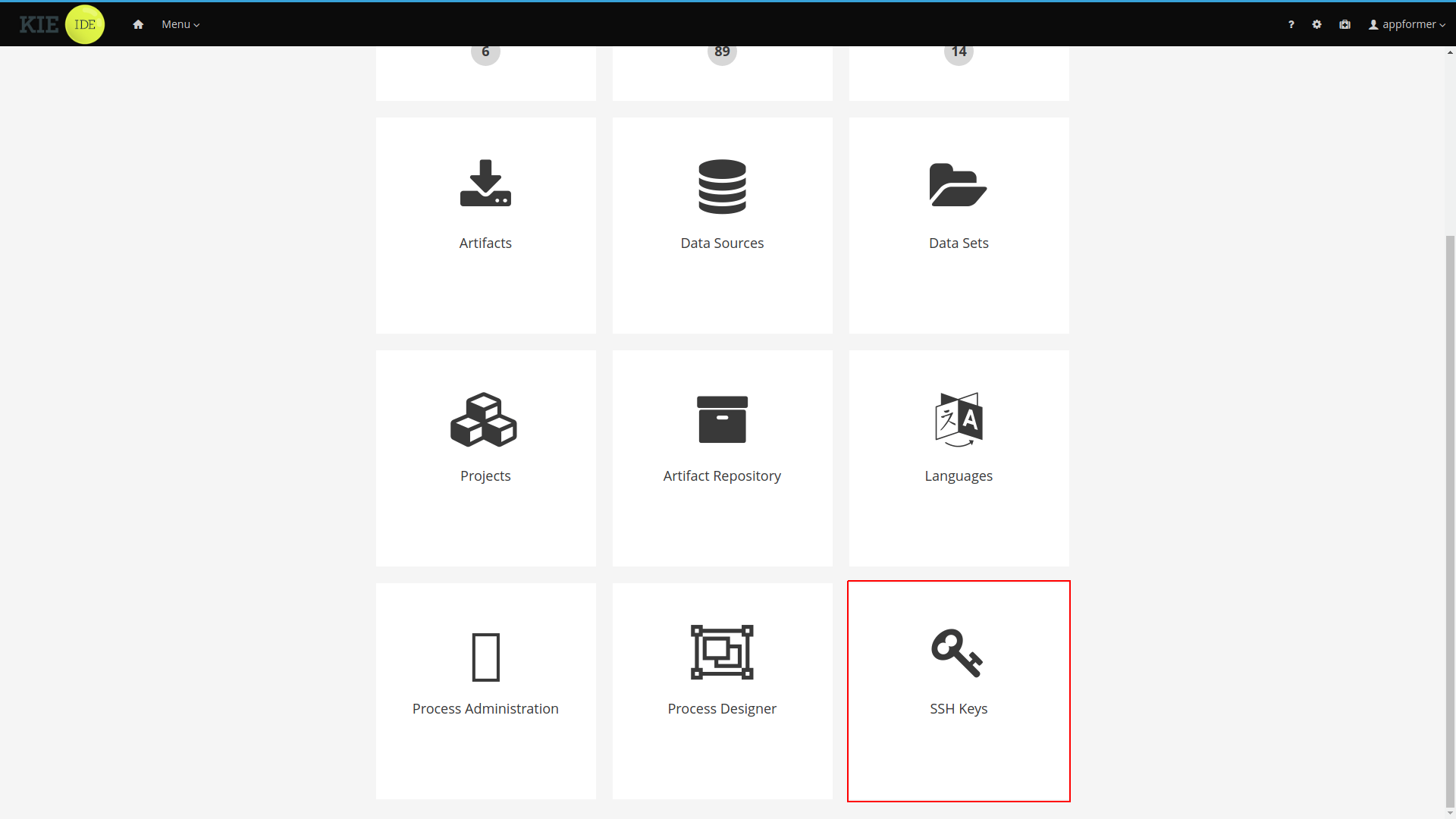
See the SSH keystore section for details.
24.13. What is New and Noteworthy in Drools 7.13
24.13.1. Allow to declare serialVersionUID on classes generated from declared types
To improve the compatibility of serialized KieSession, it has been introduced the possibility to specify the serialVersionUID on the classes generated from the declared types through an annotation like the following:
declare MyClass
@serialVersionUID( 42 )
name : String
end
24.13.2. Declaratively set Calendars on KieSession via kmodule.xml
It is possible to declaratively set one or more Calendars on a KieSession through the kmodule.xml configuration file as in the following example:
<ksession name="KSession1">
<calendars>
<calendar name="monday" type="org.domain.Monday"/>
</calendars>
</ksession>
where the type is the name of class implementing the org.kie.api.time.Calendar
interface.
24.13.3. KieSessions pool
In high volume use cases `KieSession`s get created and disposed with a very high frequency. In general this operation is not extremely time consuming, but when repeated millions of times can become a bottleneck and also requires a huge GC effort. Some users tried to alleviate this problem with self-made pools and for this reason it has been decided to provide this solution out-of-the-box.
To obtain a pool of KieSession
from a KieContainer
is enough to invoke on it the method
KieContainerSessionsPool KieContainer.newKieSessionsPool(int initialSize)
where initialSize
is the number of the KieSession`s that will be initially created in the pool. However, if required by the running
application, the number of `KieSession`s in the pool will dynamically grow beyond that value. At this point you can create
`KieSession`s from that pool as you would normally do from a `KieContainer
:
KieContainerSessionsPool pool = kContainer.newKieSessionsPool(10);
KieSession kSession = pool.newKieSession();
Now you can use the KieSession as per normal, and when you call dispose()
on it, instead of being destroyed, it just
gets resetted and pushed back into the pool. Note that using this pool will also affect the case when you have one or more
StatelessKieSession`s and you keep reusing them with multiple call to the `execute()
method. In fact a StatelessKieSession
created directly from a KieContainer
will keep to internally create a new KieSession
for each execute()
invocation.
Conversely if you create the StatelessKieSession
from the pool it will internally uses the KieSession`s provided by the
pool itself. In other words even if you asked a `StatelessKieSession
to the pool, what is actually pooled are the `KieSession`s
that are wrapped by it.
Once you’re done with the pool it is required that you call the shutdown()
method on it to avoid memory leaks. Alternatively
calling dispose()
on the whole KieContainer
will also automatically shutdown all the pools eventually created from it.
24.13.4. New and Noteworthy in KIE Workbench 7.13.0
24.13.4.1. Test Scenarios (Preview) editor
This version contains the preview of the new Test Scenarios editor that tests a rule with a completely new user experience.
See section Test scenarios for details on enabling and using the editor.
24.13.4.2. Experimental Features support
New Experimental Features Framework added to the Workbench. It provides an easy mechanism for users to preview features which are not part of the product but might be interesting for them (for example, ongoing developments, tech previews, POCs…).
See section Experimental Features Framework for more details.
24.13.4.3. SSH keystore
In order to provide the Workbench VFS with proper SSH authentication, a new keystore must added. This keystore enables users to register their SSH public keys.
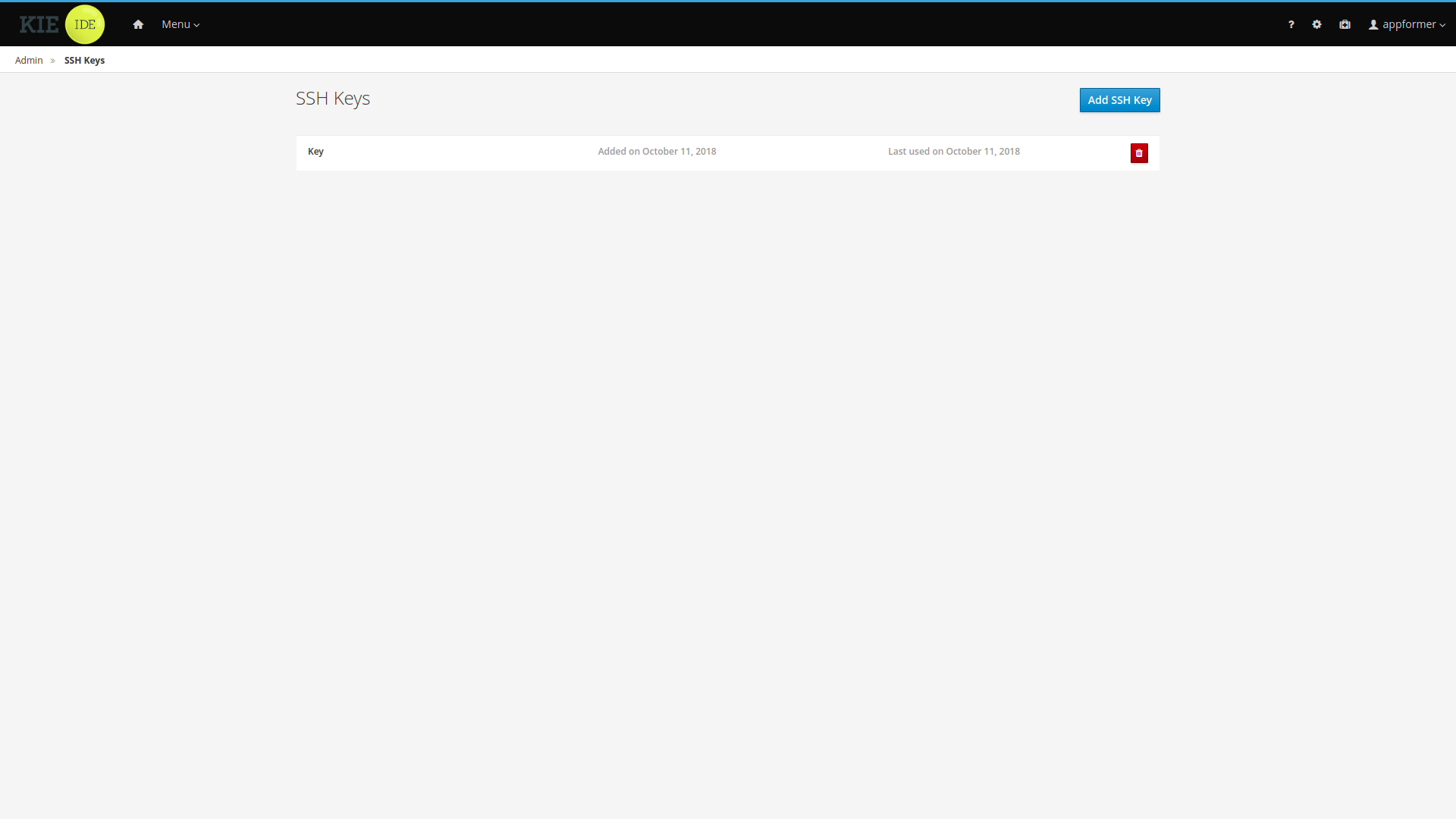
You can access it from the Admin page using the new SSH Keys menu option.
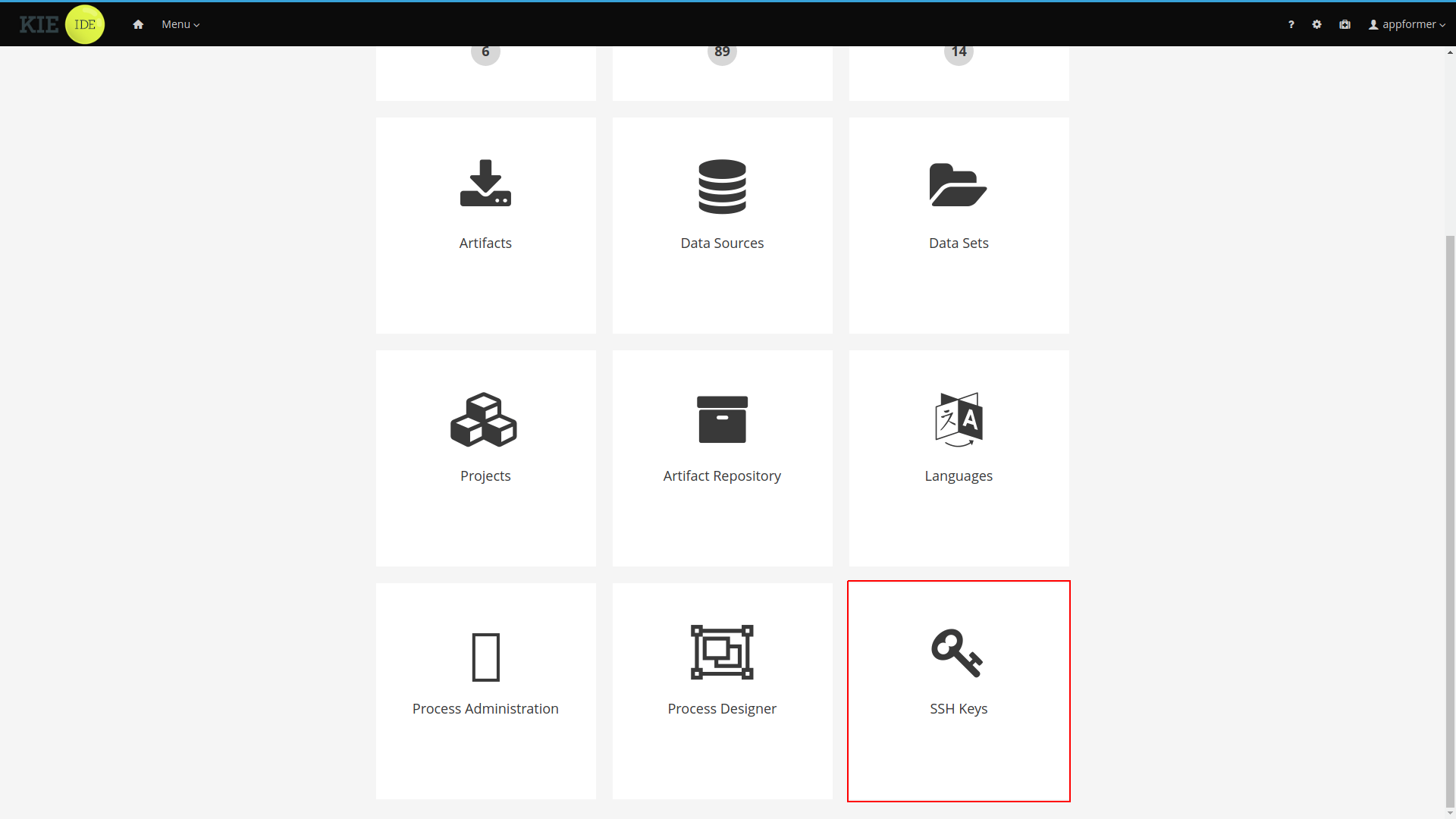
See the SSH keystore section for details.
24.14. What is New and Noteworthy in Drools 7.12
24.14.1. End of support for drools-rhq-plugin
component
The drools-rhq-plugin
component for monitoring Drools using JBoss Operations Network is no longer supported because it is underutilized and has become outdated.
24.15. What is New and Noteworthy in Drools 7.11
24.15.1. Minor API changes of Kie DMN open source engine
The API of Kie DMN open source engine introduces some minor changes, in order to support the new DMN v1.2 format.
A new package org.kie.dmn.model.api
inside Maven module kie-dmn-model
provides a generic model to support for both DMN versions v1.1 and v1.2.
This requires users of previous versions to update references from previous package org.kie.dmn.model.v1_1
to this new package org.kie.dmn.model.api
.
Users of the Kie DMN API must update references of import packages from import org.kie.dmn.model.v1_1.; to import org.kie.dmn.model.api.; .
|
Package org.kie.dmn.api.marshalling.v1_1
has been deprecated in favour of org.kie.dmn.api.marshalling
; normally this shouldn’t impact end-users of the Kie DMN API as the DMNRuntime
will automatically provide support for both DMN v1.1 and v1.2. This change impacts users who manage the marshaling of DMN resources manually, who must upgrade to the new package in order to support the new DMN v1.2 version.
24.16. New and Noteworthy in KIE Workbench 7.11.0
24.16.1. Multiple Git branches support
You can now work on multiple Git source branches interchangeably in Business Central to improve the Git workflow of your projects.
After you have created and opened a project, you can see all the assets of your project:
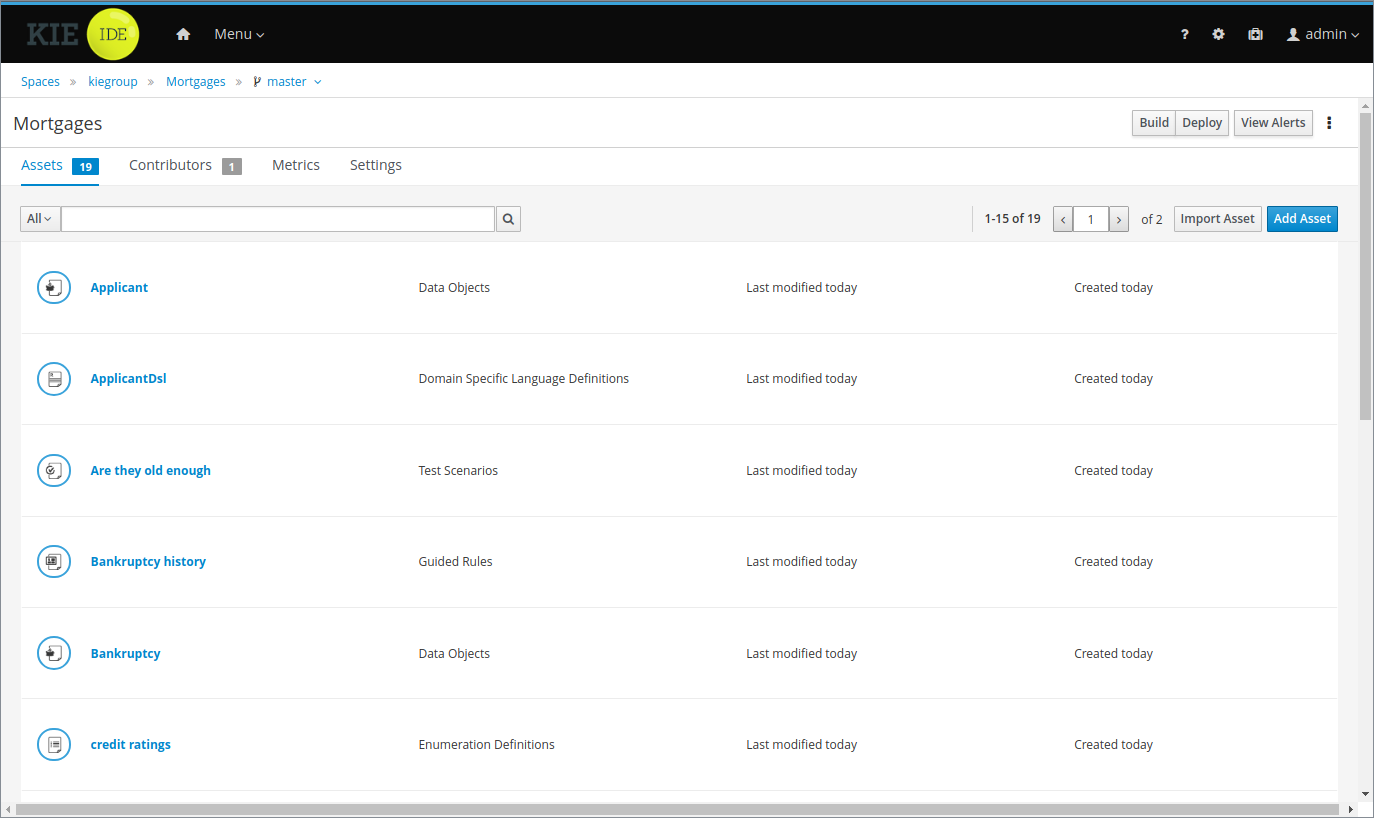
In the breadcrumbs navigation, you can now see a drop-down menu that, when clicked, displays all the Git branches available:
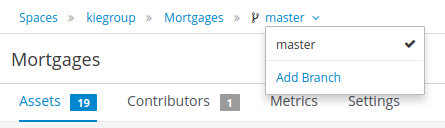
You can click Add Branch to add more branches to your project:
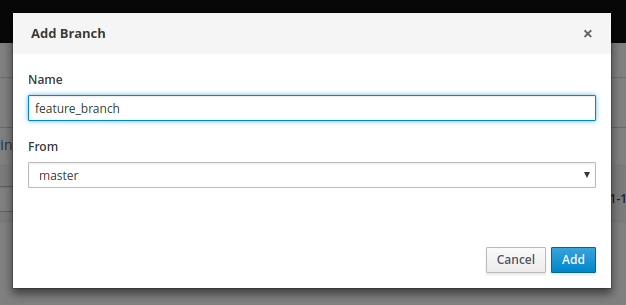
After adding the new branch, you are redirected to the new branch with all the assets that you had in the base branch:
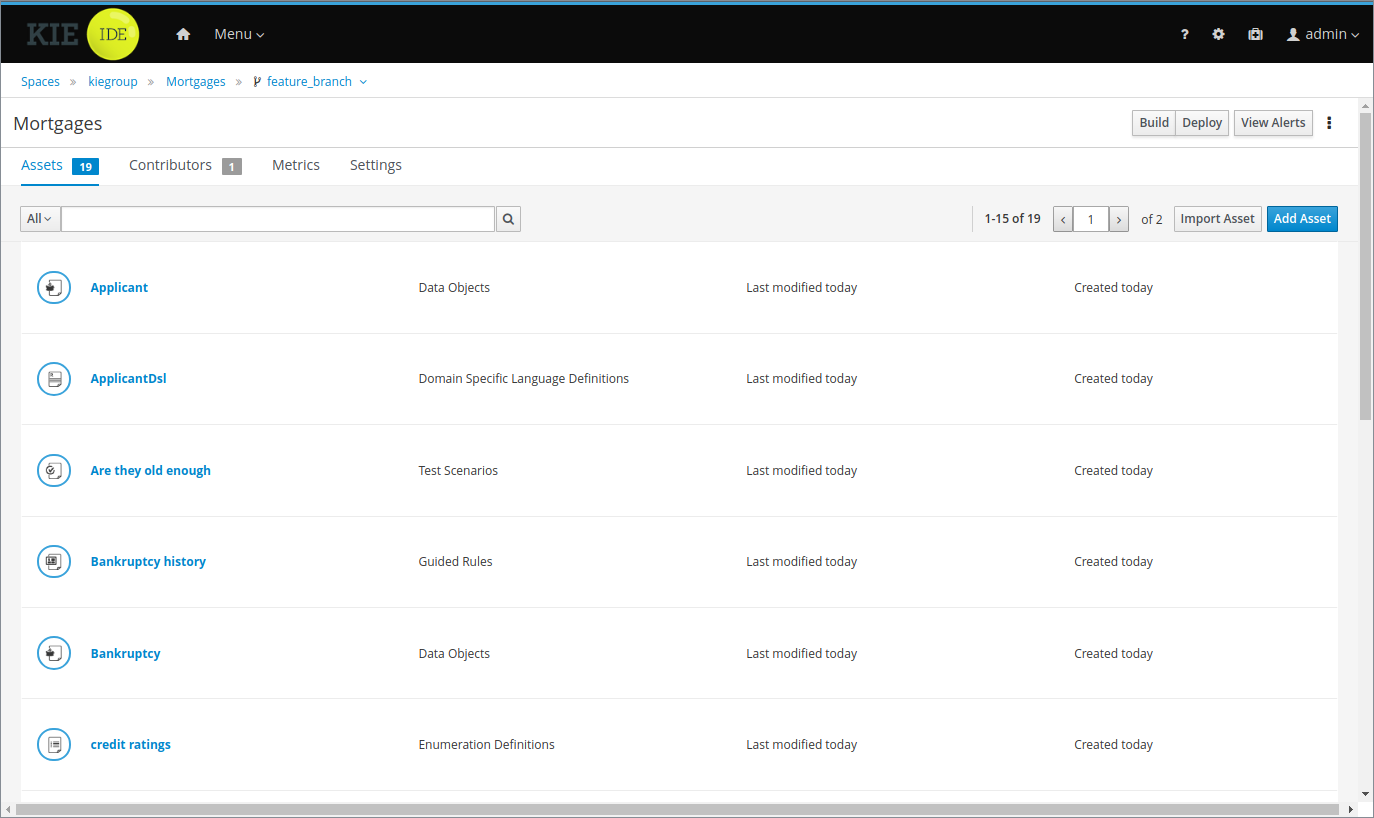
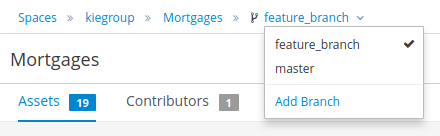
You can also click Delete Branch in the top-right corner of the screen to delete any branch except for the master branch:
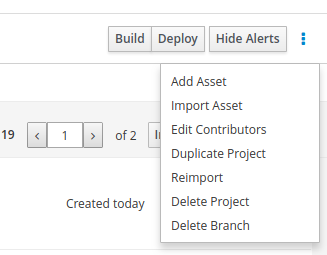
24.17. What is New and Noteworthy in Drools 7.9
24.17.1. Moved ExecutableCommand to KIE public API
Drools internal ExecutableCommand
interface has been moved to KIE public API. It’s now possible to create custom
executable commands without rely on internal interface.
A deprecated internal ExecutableCommand
interface still exists for backward compatibility and will be removed in a
future release.
Existing custom commands based on old internal interface that override canRunInTransaction() method will no
longer compile due to this change.
|
24.17.2. Alpha Network Compiler
Drools now supports an optimization on evaluating alpha nodes which involves generating an intermediate class that gets compiled to evaluate the constraint faster.
It’s highly experimental and it’s supposed to work only with the new executable model system. To enable it use drools.alphaNetworkCompiler
configuration key in the KieModuleModel
configuration.
<kmodule xmlns:xsi="http://www.w3.org/2001/XMLSchema-instance" xmlns="http://www.drools.org/xsd/kmodule"> <configuration> <property key="drools.alphaNetworkCompiler" value="true"/> </configuration> </kmodule>
The current implementation has problems with incremental compilation so don’t use it together. |
24.18. New and Noteworthy in KIE Workbench 7.8.0
24.18.1. New System Property for setting the Default Maven Repository in Project pom.xml files
To make building Workbench projects outside of the Workbench easier, it is now possible to set the URL for the default Maven Repository that is added into each new Project pom.xml. It is recommended that you set this before starting you Workbench for the first time.
24.19. What is New and Noteworthy in Drools 7.7
24.19.1. Executable rule models
Executable rule models are embeddable models that provide a Java-based representation of a rule set for execution at build time. The executable model is a more efficient alternative to the standard asset packaging in Drools and enables KIE containers and KIE bases to be created more quickly, especially when you have large lists of DRL (Drools Rule Language) files and other Drools assets. The model is low level and enables you to provide all necessary execution information, such as the lambda expressions for the index evaluation.
Executable rule models provide the following specific advantages for your projects:
-
Compile time: Traditionally, a packaged Drools project (KJAR) contains a list of DRL files and other Drools artifacts that define the rule base together with some pre-generated classes implementing the constraints and the consequences. Those DRL files must be parsed and compiled when the KJAR is downloaded from the Maven repository and installed in a KIE container. This process can be slow, especially for large rule sets. With an executable model, you can package within the project KJAR the Java classes that implement the executable model of the project rule base and re-create the KIE container and its KIE bases out of it in a much faster way. In Maven projects, you use the
kie-maven-plugin
to automatically generate the executable model sources from the DRL files during the compilation process. -
Run time: In an executable model, all constraints are defined as Java lambda expressions. The same lambda expressions are also used for constraints evaluation, so you no longer need to use
mvel
expressions for interpreted evaluation nor the just-in-time (JIT) process to transform themvel
-based constraints into bytecode. This creates a quicker and more efficient run time. -
Development time: An executable model enables you to develop and experiment with new features of the Drools engine without needing to encode elements directly in the DRL format or modify the DRL parser to support them.
24.19.1.1. Executable model domain-specific languages (DSLs)
One goal while designing the first iteration of the domain-specific language (DSL) for the executable model was to get rid of the notion of pattern and to consider a rule as a flow of expressions (constraints) and actions (consequences). For this reason we called it Flow DSL. Some examples of this DSL are available here.
However after having implemented the Flow DSL it became clear that the decision of avoiding the explicit use of patterns obliged us to implement some extra logic that had both a complexity and a performance cost, since in order to properly re-create the data structures expected by the Drools compiler it is necessary to put together the patterns out of those apparently unrelated expressions.
For this reason it has been decided to reintroduce the patterns in a second DSL that we called Pattern DSL. This allowed to bypass that algorithm grouping expressions that has to fill an artificial semantic gap and that is also time consuming at run time. We believe that both DSLs are valid for different use cases and so we decided to keep and support both. In particular the Pattern DSL is safer and faster (even if more verbose) so this will be the DSL that will be automatically generated when creating a KJAR through the kie-maven-plugin. Conversely the Flow DSL is more succinct and closer to the way a user may want to programmatically define a rule in Java and we planned to make it even less verbose by generating in an automatic way through a post processor the parts of the model defining the indexing and property reactivity. In other words, we expect that the Pattern DSL will be written by machines and the Flow DSL eventually by humans.
24.19.1.2. Embedding an executable rule model in a Maven project
You can embed an executable rule model in your Maven project to compile your rule assets more efficiently at build time.
-
You have a Mavenized project that contains Drools business assets.
-
In the
pom.xml
file of your Maven project, ensure that the packaging type is set tokjar
and add thekie-maven-plugin
build component:<packaging>kjar</packaging> ... <build> <plugins> <plugin> <groupId>org.kie</groupId> <artifactId>kie-maven-plugin</artifactId> <version>${drools.version}</version> <extensions>true</extensions> </plugin> </plugins> </build>
The
kjar
packaging type activates thekie-maven-plugin
component to validate and pre-compile artifact resources. The<version>
is the Maven artifact version for Drools currently used in your project (for example, 7.23.0.Final-redhat-00002). These settings are required to properly package the Maven project. -
Add the following dependencies to the
pom.xml
file to enable rule assets to be built from an executable model:-
drools-canonical-model
: Enables an executable canonical representation of a rule set model that is independent from Drools -
drools-model-compiler
: Compiles the executable model into Drools internal data structures so that it can be executed by the Drools engine
<dependency> <groupId>org.drools</groupId> <artifactId>drools-canonical-model</artifactId> <version>${drools.version}</version> </dependency> <dependency> <groupId>org.drools</groupId> <artifactId>drools-model-compiler</artifactId> <version>${drools.version}</version> </dependency>
-
-
In a command terminal, navigate to your Maven project directory and run the following command to build the project from an executable model:
mvn clean install -DgenerateModel=<VALUE>
The
-DgenerateModel=<VALUE>
property enables the project to be built as a model-based KJAR instead of a DRL-based KJAR.Replace
<VALUE>
with one of three values:-
YES
: Generates the executable model corresponding to the DRL files in the original project and excludes the DRL files from the generated KJAR. -
WITHDRL
: Generates the executable model corresponding to the DRL files in the original project and also adds the DRL files to the generated KJAR for documentation purposes (the KIE base is built from the executable model regardless). -
NO
: Does not generate the executable model.
Example build command:
mvn clean install -DgenerateModel=YES
-
24.19.1.3. Embedding an executable rule model in a Java application
You can embed an executable rule model programmatically within your Java application to compile your rule assets more efficiently at build time.
-
You have a Java application that contains Drools business assets.
-
Add the following dependencies to the relevant classpath for your Java project:
-
drools-canonical-model
: Enables an executable canonical representation of a rule set model that is independent from Drools -
drools-model-compiler
: Compiles the executable model into Drools internal data structures so that it can be executed by the Drools engine
<dependency> <groupId>org.drools</groupId> <artifactId>drools-canonical-model</artifactId> <version>${drools.version}</version> </dependency> <dependency> <groupId>org.drools</groupId> <artifactId>drools-model-compiler</artifactId> <version>${drools.version}</version> </dependency>
The
<version>
is the Maven artifact version for Drools currently used in your project (for example, 7.23.0.Final-redhat-00002). -
-
Add rule assets to the KIE virtual file system
KieFileSystem
and useKieBuilder
withbuildAll( ExecutableModelProject.class )
specified to build the assets from an executable model:import org.kie.api.KieServices; import org.kie.api.builder.KieFileSystem; import org.kie.api.builder.KieBuilder; KieServices ks = KieServices.Factory.get(); KieFileSystem kfs = ks.newKieFileSystem() kfs.write("src/main/resources/KBase1/ruleSet1.drl", stringContainingAValidDRL) .write("src/main/resources/dtable.xls", kieServices.getResources().newInputStreamResource(dtableFileStream)); KieBuilder kieBuilder = ks.newKieBuilder( kfs ); // Build from an executable model kieBuilder.buildAll( ExecutableModelProject.class ) assertEquals(0, kieBuilder.getResults().getMessages(Message.Level.ERROR).size());
After
KieFileSystem
is built from the executable model, the resultingKieSession
uses constraints based on lambda expressions instead of less-efficientmvel
expressions. IfbuildAll()
contains no arguments, the project is built in the standard method without an executable model.As a more manual alternative to using
KieFileSystem
for creating executable models, you can define aModel
with a fluent API and create aKieBase
from it:Model model = new ModelImpl().addRule( rule ); KieBase kieBase = KieBaseBuilder.createKieBaseFromModel( model );
24.20. New and Noteworthy in KIE Workbench 7.7.0
24.20.1. Project Oriented Workbench
Workbench becomes Project Oriented. Meaning each project is now in a dedicated repository. Previously each project was in a folder and one repository could hold several projects. These changes merge the concepts of Project and Repository.
One project one repository approach makes the UI simpler and improves releasing or freezing a single project. Previously if a project was frozen, branched or tagged the repository was still shared with other projects, making the release control harder and more complicated.
Projects from older Workbench versions need to be migrated to the new setup. For this we offer a command line migration tool.
Project Orientated does not, at the moment, offer support for multimodule setup. This feature is planned, but not in this release. The now deprecated Asset Management features depended on multimodule support and can not be migrated to this Workbench version.
24.20.2. Connecting to a headless Drools controller
When running a Workbench instance, there is now greater flexibility to decide how Kie Server instances will be managed. Previously, whenever a Workbench is started, it would always start an embedded Drools controller. This setup is still available but now there is also an option to not start this service and instead connect to a headless Drools controller. This allows a more fine grained deployment model where it’s possible to decide the best approach for a specific scenario.
To switch between these modes, a key system property is used: org.kie.workbench.controller. By default, the Workbench will continue to start the embedded service if this system property is missing. Otherwise, it will try to connect to the remote service and also ensure that none of the embedded services are started.
It is important to note that only Web Socket connection protocol is available to use when connecting to headless Drools controller.
For more details regarding all possible system configs regarding user name, password, token and secured password via key store, please refer to Workbench system properties.
24.20.3. Content management enhancements
24.20.3.1. Properties panel
It is possible to edit the properties of the different page elements including, the page itself or any of its rows and components. Once an element is selected, either by hovering on the element and clicking on the editor’s area or selecting the element in the Properties panel dropdown, its properties are displayed in the left docked panel. See screenshot:
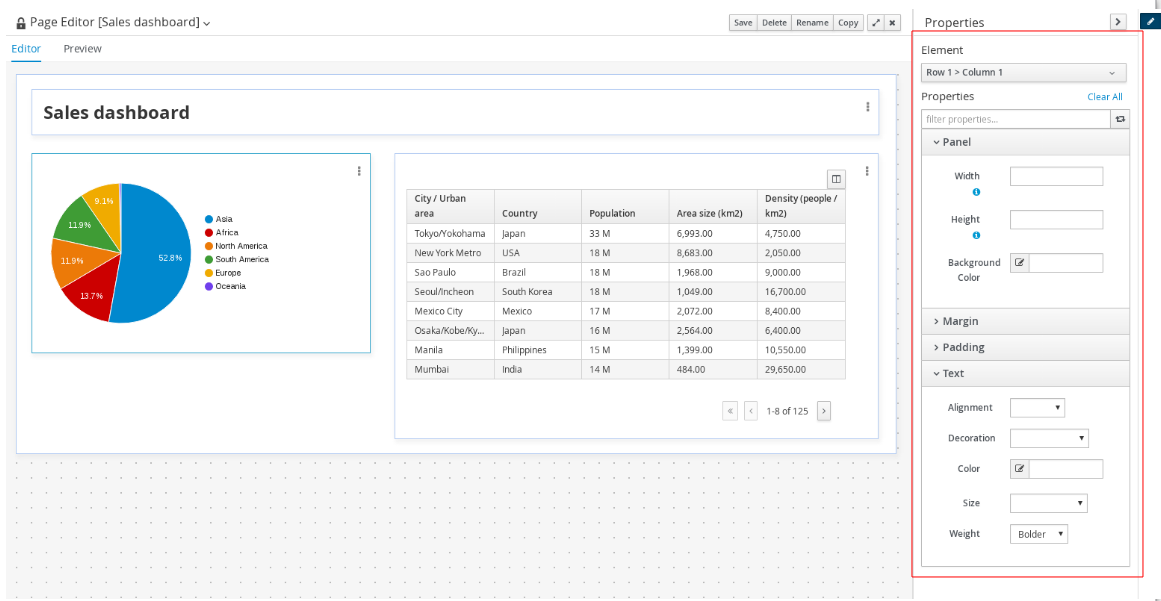
The properties available in this version are basically those related with the element style such as width, height or margins, amongst others. The properties available might differ for each type. Notice for example, the HTML component provides an extra set of properties all related with the text style.
Once a property is changed, its value is reflected in the editor’s area, both in design and preview modes.
24.20.3.2. Screen component removed
The Screen component, which was placed under the Core group in the right sidebar’s Components panel, has been removed. The reason is, this component was not suitable for production environments.
In future versions though, domain related components, such as a BPM’s task list, will be available for easy consumption by end users.
24.20.4. New Migration Tool
A new command line Migration tool with support for Linux and Windows has been provided to move different resources to it’s latest version. It makes possible to perform different migrations:
-
Project Migration: migrates KIE projects from the old project layout (7.4.x and previous) to the new project-oriented structure.
-
Forms Migration: migrates old jBPM Form Modeler forms into the new Forms format.

You can find more info here.
24.21. New and Noteworthy in KIE Workbench 7.6.0
24.21.1. Content management enhancements
A few extra changes have been introduced in the Content Management tooling (aka Page Authoring) in order to improve the user experience. The following screenshot reveals the changes introduced since the latest version.
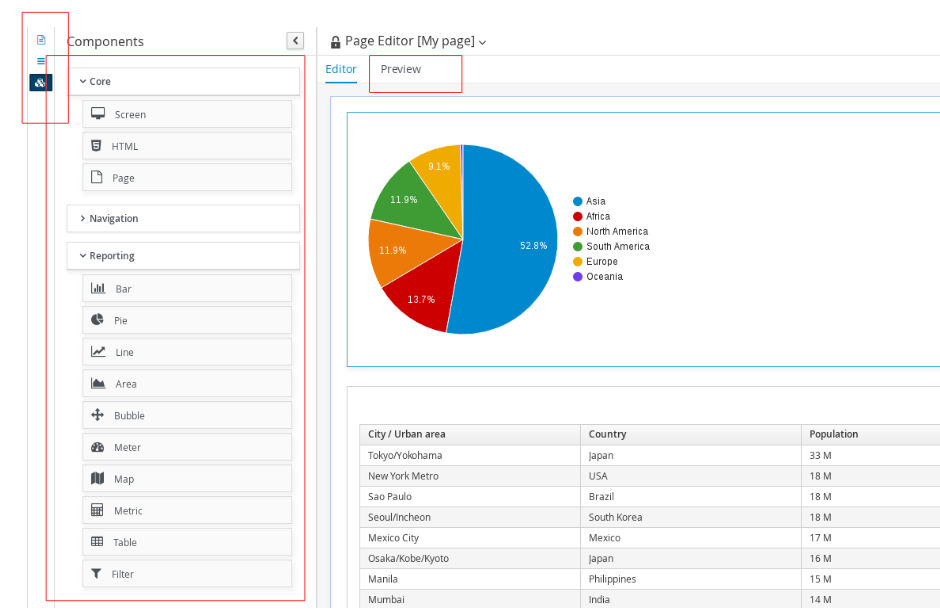
24.21.1.1. Fluid/Page editor mode selection
The new page pop up allows for the selection of two edition modes:
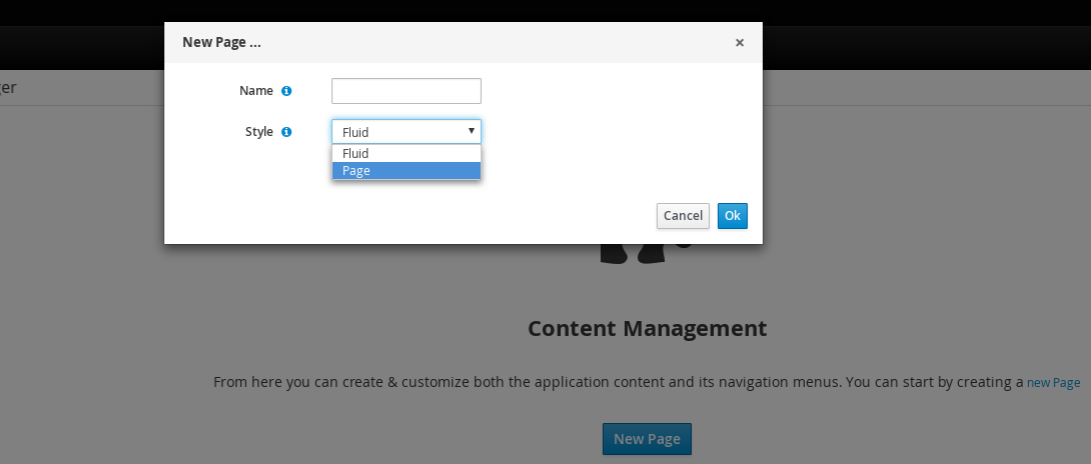
-
Fluid: it acts more as a classical web page, showing a vertical scrollbar when the page exceeds the available height.
-
Page: it was the default in previous versions. When selected, it forces the page to always fit the window’s 100% height.
24.21.1.2. Preview feature
Page authors can go back and forth from the Editor to the Preview mode as many times as needed. In the Preview mode all the editor’s controls are removed, allowing for the display of the page as it would be seen by end users once the page is published.
24.21.1.3. Use dock panels to increase the editor content area
In order to increase the available space in the editor’s central area, the page listing, the navigation configuration and the component palette panels have all been moved to the left sidebar as docked panels.
24.21.1.4. Component palette reorganization
In previous versions, there only existed one single category of components in the palette. As of version 7.6, the components are now grouped into three main categories:
-
Core: HTML and Page components
-
Navigation: Menu Bar, Tree, Tiles, Tab List, Carousel and Target Div
-
Reporting: Bar, Pie, Line, Area, Map, Bubble, Metric, Meter, Table and Filter
The goal of these changes is to make the palette more appealing as well as to ease the selection of the target component to drag.
24.22. New Drools controller client API
In order to facilitate the management of Drools controller related tasks such as creating server templates, starting and stopping containers, etc, we developed a new Java client API available under the kie-server-controller-client Maven module. With this API, you can connect to a Drools controllerusing either REST or Web Socket protocols. For more details, see Drools controller Client API chapter.
24.23. Breaking changes in Kie Server 7.5.1 from 7.0
24.23.1. Drools controller API changes
Changes to SpecManagementService
interface:
-
Included new method
getContainerInfo
that allows to retrieve a singleContainerSpec
defined in aServerTemplate
. -
Changed methods
listContainerSpec
,listServerTemplateKeys
, andlistServerTemplates
return types from generic collection to specific domain list types (ContainerSpecList
,ServerTemplateKeyList
, andServerTemplateList
) in order to properly serialize and deserialize the returned values using JAXB and JSON.
Changes to RuleCapabilitiesService
interface:
-
startScanner
method now uses ajava.lang.Long
time instead of a primitivelong
for theinterval
parameter in order to avoid JSON and JAXB serialization issues.
Changes to RuntimeManagementService
interface:
-
Changed methods
getContainers
andgetServerInstances
return types from generic collection to specific domain list types (ContainerList
andServerInstanceKeyList
) in order to properly serialize and deserialize the returned values using JAXB and JSON.
For more details, see JBPM-6243.
24.23.2. Kie Server API changes
Changes to ServiceResponse
wrapper:
-
Moved
ResponseType
enum and common methods to a new interface calledKieServiceResponse
, allowing it to be extended to multiple implementations.
24.24. New and Noteworthy in KIE Workbench 7.5.0
24.24.1. Content management enhancements
Remarkable changes have been introduced in the Content Management (aka Dashboards) tooling in order to improve the user experience.
24.24.1.1. Perspective to page renaming
The "page" term is far more familiar to users. Notice that, "perspective" is a concept that was borrowed from the Eclipse development tool, thus it is a concept mostly used in developer circles. Page is more related to web content, easier to understand by regular people. For this reason, perspective has been renamed to page all over the tooling.
24.24.1.2. Left sidebar new look and feel
This is the most noticeable change introduced. The left sidebar has been completely rewritten in order to provide an enhanced look and feel.
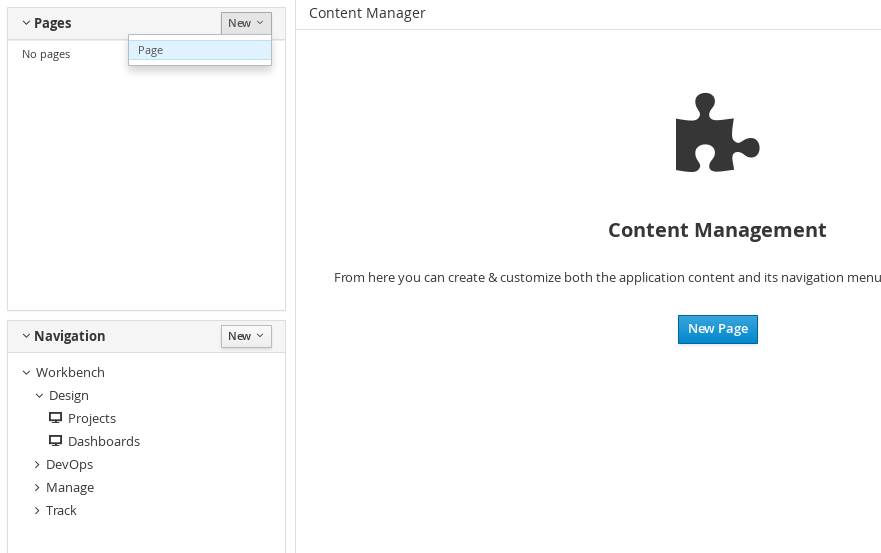
The two sections Pages and Navigation have been merged into a single view. From this view users can either create new pages or change the navigation configuration.
The Navigation section lists the navigation trees. Specifically, the tree named Workbench is available by default, it can not be deleted and it contains the entries displayed in the workbench’s top mega menu. Any change applied to it will cause the mega menu to modify its entries. This is actually the mechanism users must use to extend the workbench with new pages.
24.24.1.3. Tags button disabled
The tags feature or the ability to attach a set of labels to a page during edition time has been removed. This feature in combination with the former "Apps" perspective was used to allow users to publish their dynamic pages in a categorized way. This feature is no longer needed. During the edition of a page, the Tags button, that used to appear at the editor’s top right button bar, has been removed.
As a replacement, users can leverage the existing navigation features to create new pages and attach them to the workbench’s mega menu.
24.24.2. Extra navigation components
The existing navigation components available in the Content Management tooling have been extended with some extra types. To date there exist the following components:
-
Tile navigator
-
Tab list
-
Carousel
The following have recently been added to the release:
24.24.2.1. Menu bar
As its name states, this component displays the entries of a navigation tree in a manu bar shape. There is no limit to the number of levels supported. When a page item is clicked, the page content is displayed in the Target div (see details below) component specified in the menu bar’s configuration.
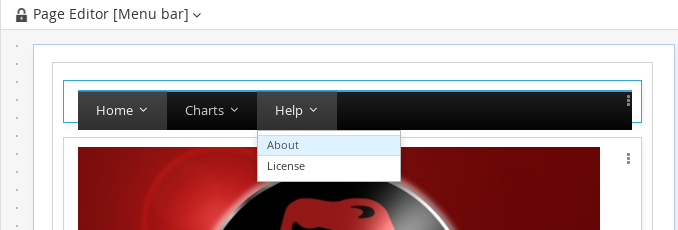
24.24.2.2. Tree navigator
Same as the Menu bar, but the entries are displayed as a vertical tree structure.
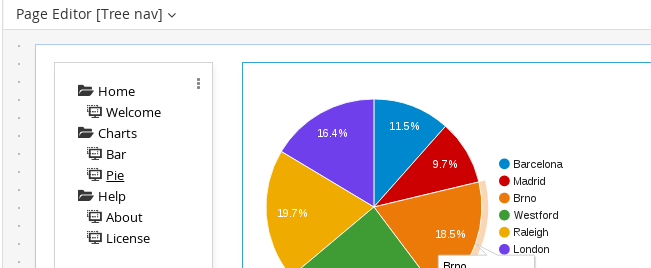
24.24.2.3. Target div
Both the Carousel and the Tile navigator components can handle by themselves the display of the items the user clicks on. Others like Tab list, Menu bar and Tree navigator require a Target div component as its display output since they have a clear separation between the display of its entries and the content of the last item clicked.
So, every time, a Target div based navigation component is dropped into a page, a Target div component must have been dropped as well, so that the first one can link to it. The following screen shows the configuration panel that is displayed every time a target div based component is dropped into a page.
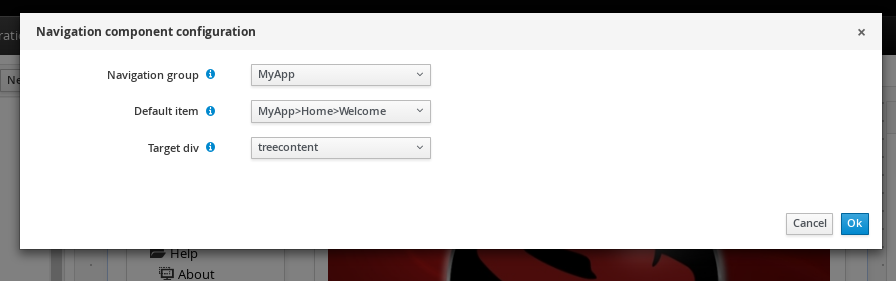
The navigation group is mandatory for all the navigation components as it indicates the navigation structure to display whereas the Target div setting is not available for non target div components like Carousel or Tile navigator.
24.25. New and Noteworthy in KIE Workbench 7.4.0
24.25.1. Guided Decision Table improvements
In addition to fixing numerous bugs the Wizard used to create and edit columns has been improved to show descriptions of the different steps required for the different column types.
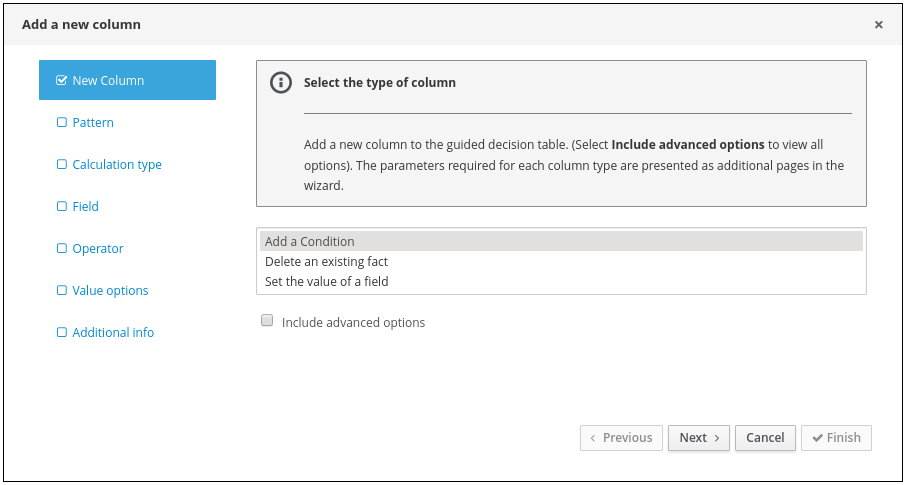
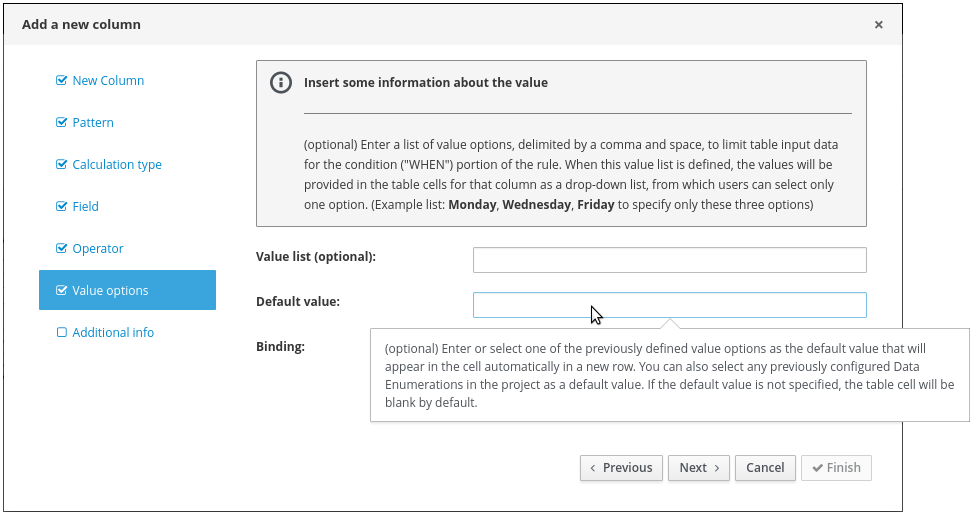
24.25.2. Disable experimental editors
The following features are considered experimental and can be disabled using the Security/User Management administration screen:-
-
Guided Decision Tree Editor
-
Guided Score Card Editor
-
XLS Score Card Editor
-
(New) BPMN2 Process Editor
-
Deployments/Server Provisioning Perspective
These features are enabled by default.
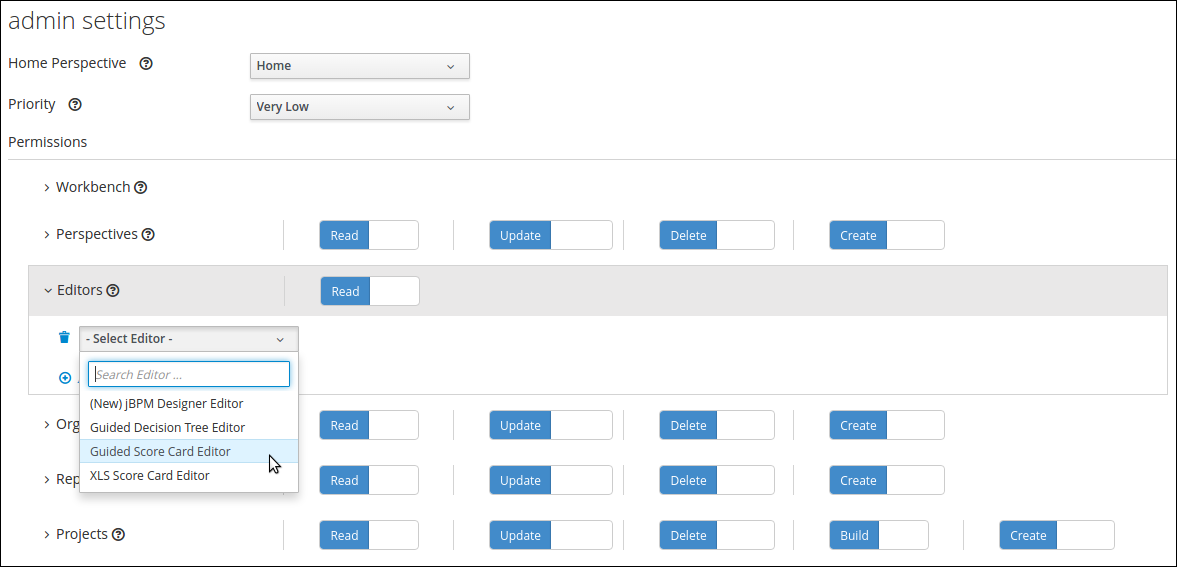
24.26. New and Noteworthy in KIE Workbench 7.3.0
24.26.1. New Home and Menu Bar
The Home page, Menu bar and About popup now have a new design. Menu items are split into groups representing major functional areas.
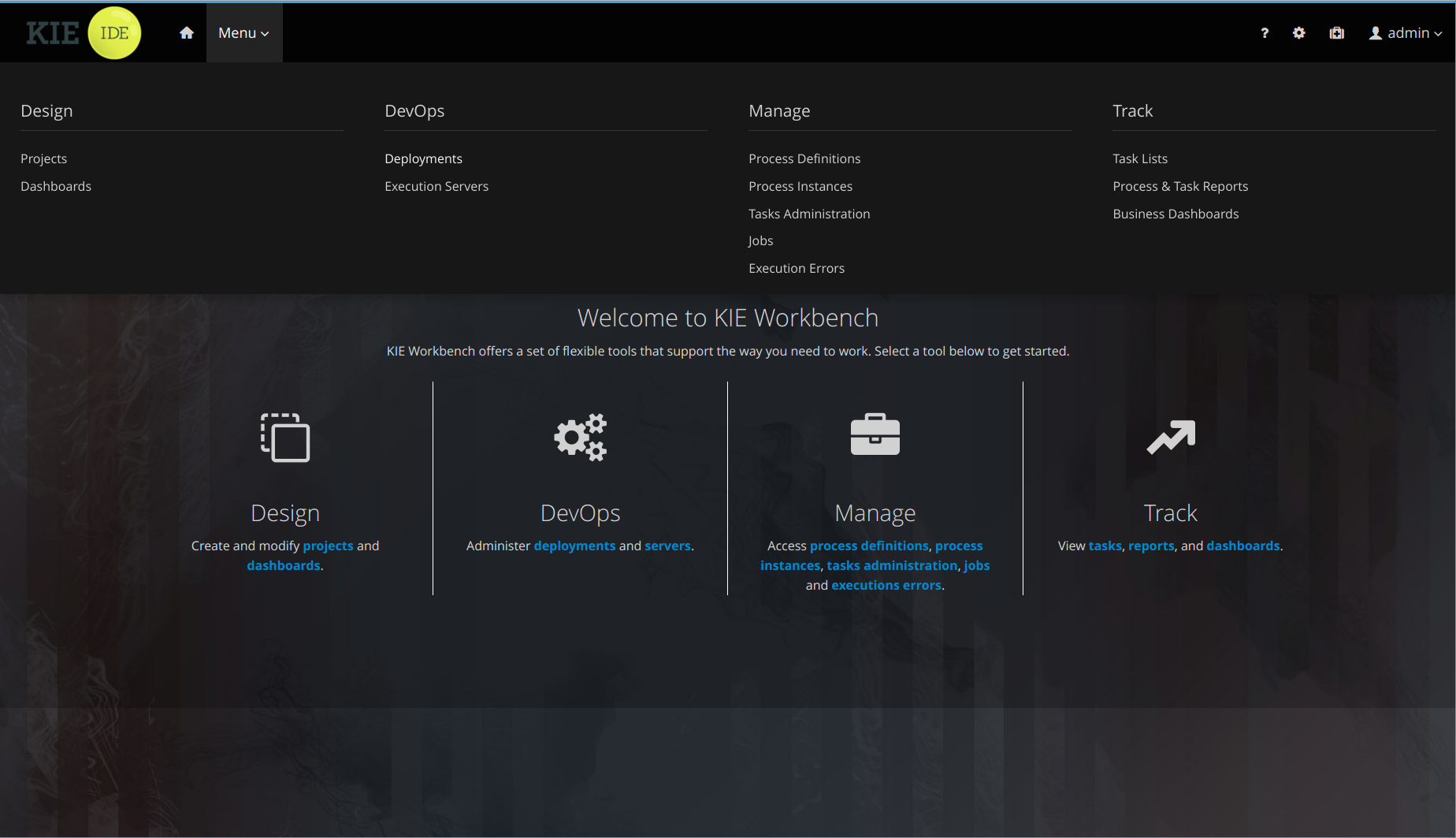
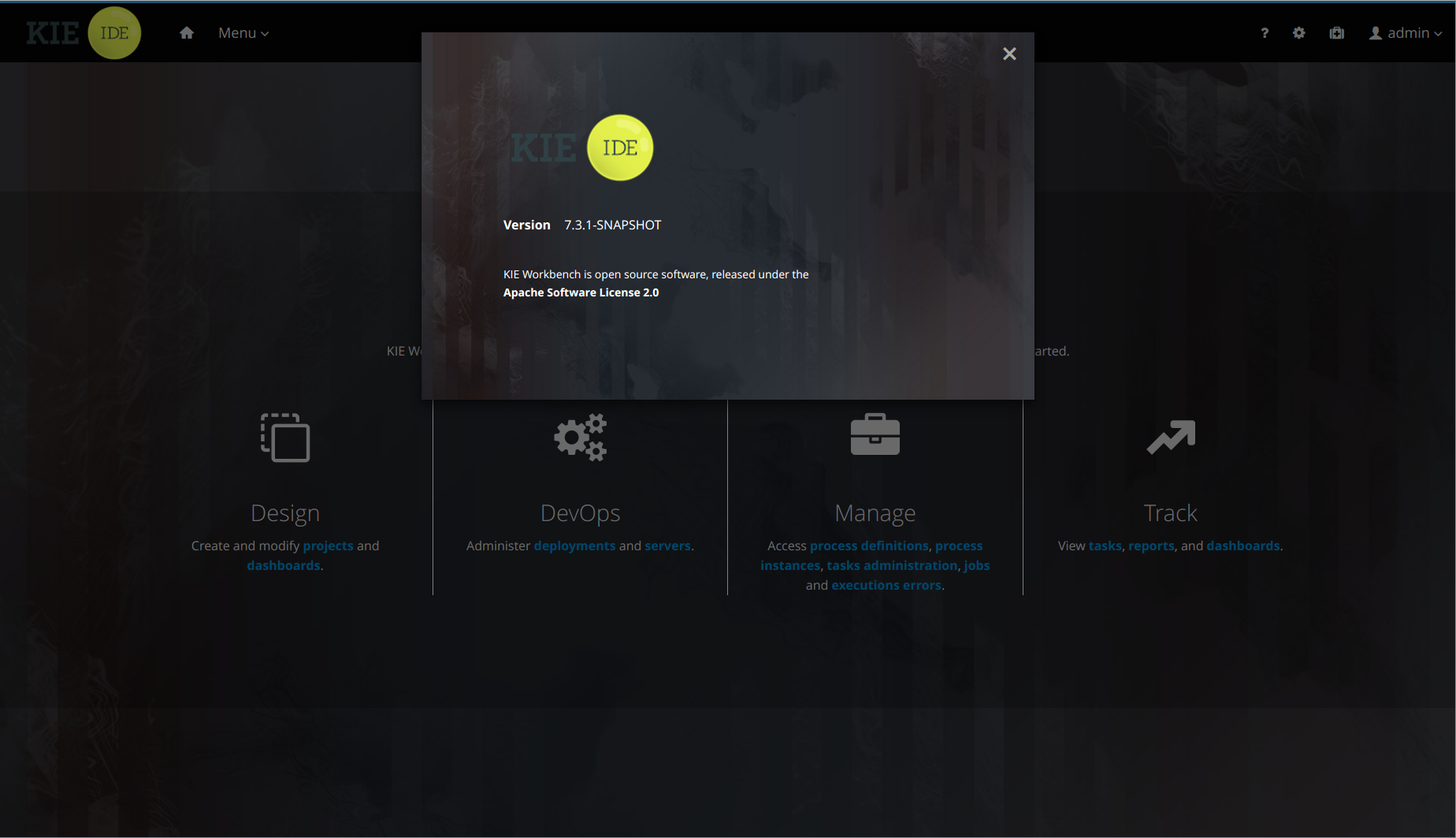
24.26.2. Admin page changes
The Admin page is now accessible with the cog icon on the Menu bar and has more items. Access to the perspective artifacts, Data Sets, Data Sources and Language options have been moved there.
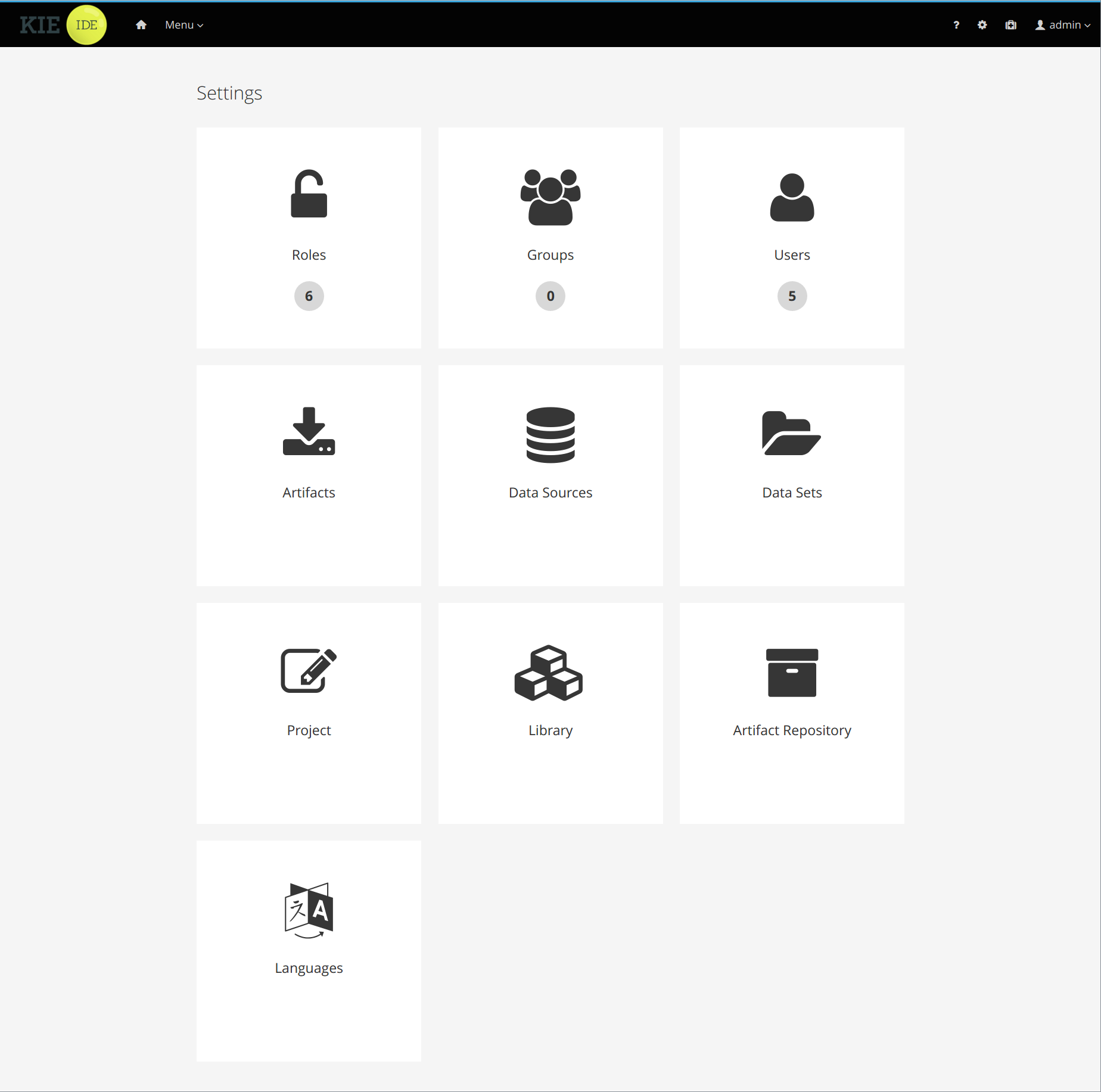
24.28. New and Noteworthy in KIE Workbench 7.1.0
24.28.1. Project Metrics Dashboard
A brand new dashboard is now available for every project listed in the authoring library. After opening the project details page, a metrics card shows up on the right side of the screen.
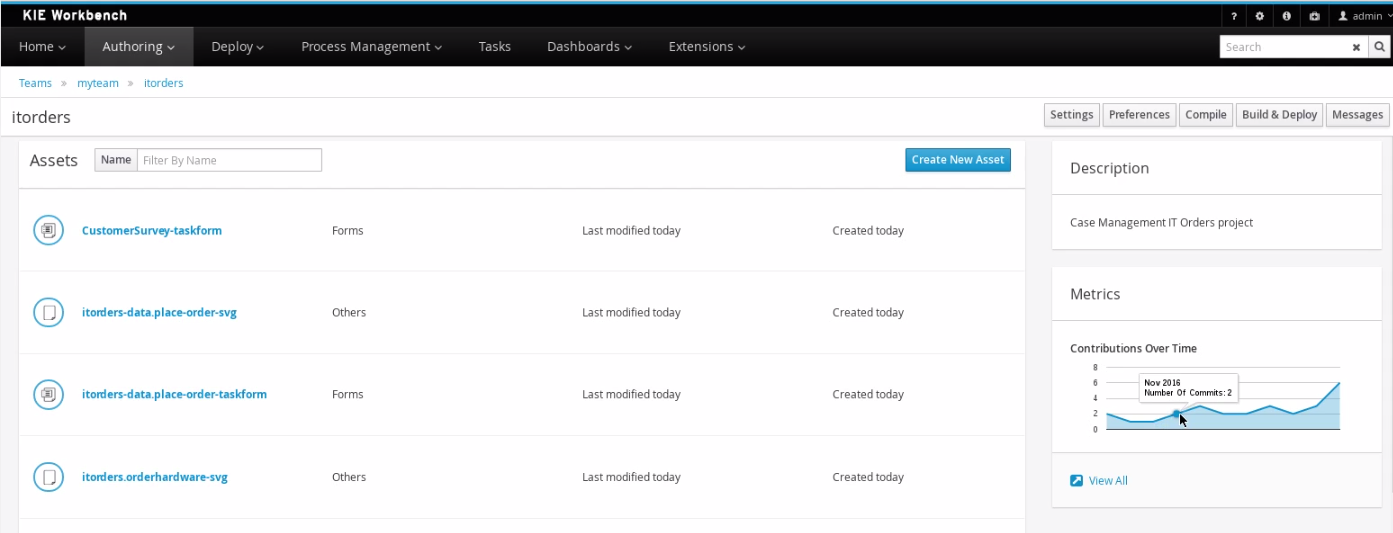
The card shows the history of contributions (commits) made to that specific project over time. Click the View All link to access the full dashboard that shows several metrics all about the project’s contributions.
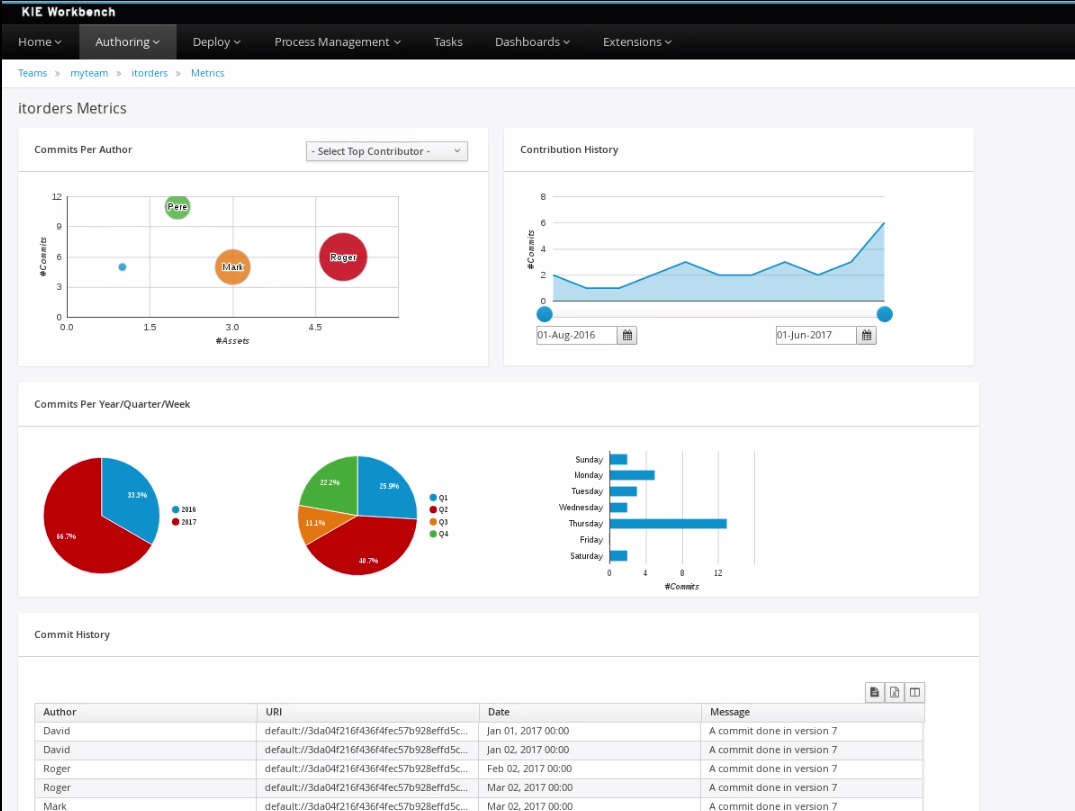
Notice that different filter controls are available for selecting the contributions made either by a concrete user or in a specific time frame.
24.28.2. Teams Metrics Dashboard
A brand new dashboard has also been added to the Teams page. A metrics card on the right side shows the history of all contributions (commits).
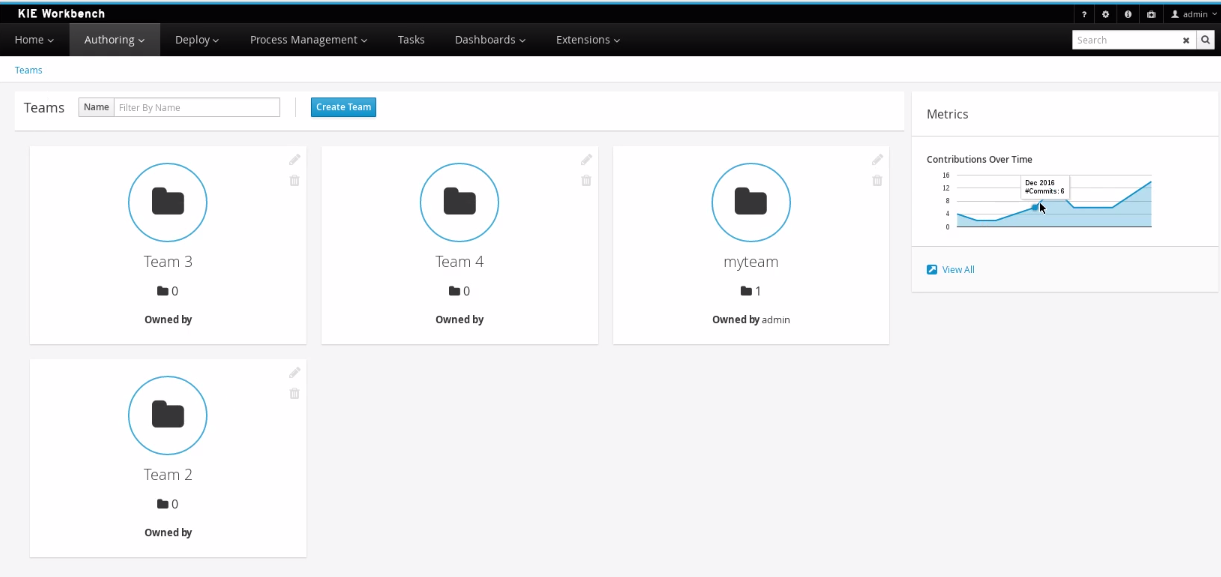
Click the View All link to access the full dashboard showing overall contributions metrics.
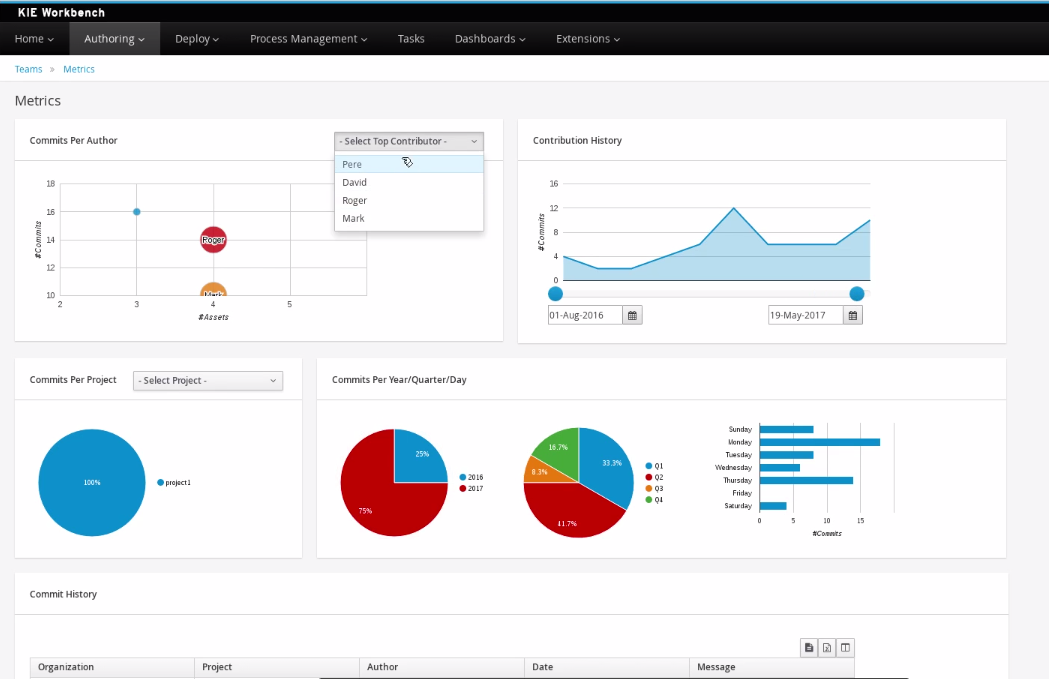
Note that different filter controls are available for selecting the contributions by different criteria:
-
by a concrete user,
-
within a specific time frame,
-
by team or,
-
by project.
This dashboard replaces the former Authoring>Contributors dashboard, which is no longer available at the top menu bar of the workbench.
24.29. What is New and Noteworthy in Drools 7.0
24.29.1. Core Engine
24.29.1.1. DMN Runtime Support
Drools now has complete runtime support for DMN (Decision Model and Notation). DMN files are now an asset that can be added to any kjar for execution. Please refer to the DMN section for details on how to build and execute DMN models.
At this time, no DMN authoring is supported, but it will be supported in future versions.
24.29.1.2. Multithreaded evaluation in the Drools engine
This feature is experimental in Drools 7.0. |
In Drools 7.0, the Drools engine can now evaluate more business rules in parallel by dividing the RETE and PHREAK pattern-matching algorithms in independent partitions and evaluating them in parallel.
Multithreaded evaluation is disabled by default in Drools. To enable multithreaded evaluation for a parallel KIE base, use one of the following options:
-
Enable multithreaded evaluation with
KieBaseConfiguration
:KieServices ks = KieServices.Factory.get(); KieBaseConfiguration kieBaseConf = ks.newKieBaseConfiguration(); kieBaseConf.setOption(MultithreadEvaluationOption.YES); KieBase kieBase = kieContainer.newKieBase(kieBaseConf);
-
Enable the multithreaded evaluation system property:
drools.multithreadEvaluation = true
Rules using queries, salience, or agenda groups are currently not supported by the parallel Drools engine. If these rule elements are present in the KIE base, the compiler emits a warning and automatically switches back to single-threaded evaluation. However, in some cases, the Drools engine might not detect the unsupported rule elements and rules might be evaluated incorrectly. For example, the Drools engine might not detect when rules rely on implicit salience given by rule ordering inside the DRL file, resulting in incorrect evaluation due to the unsupported salience attribute. |
24.29.1.3. OOPath improvements
This feature is experimental |
OOPath has been introduced with Drools 6.3.0 but in Drools 7.0.0 the syntax has been slightly changed to make it closer to standard xpath one. This means that constraints have to be put between square brackets instead of curly ones and inline cast has to be expressed out of constraints. For instance the following oopath expressed with 6.x syntax:
/list{#SubClass, prop == 0}
in Drools 7.x becomes:
/list#SubClass[prop == 0]
Also Drools 7.0.0 improves the support for standard Java Collection, with a dedicated implementation for List
and Set
,
as specialized ReactiveList
and ReactiveSet
; a ReactiveCollection
is also available.
This also includes out of the box reactive support when performing mutable operations through their Iterator
and ListIterator
.
public class School extends AbstractReactiveObject {
private String name;
private final List<Child> children = new ReactiveList<Child>(); (1)
public void setName(String name) {
this.name = name;
notifyModification(); (2)
}
public void addChild(Child child) {
children.add(child); (3)
// no need to call notifyModification() here.
}
1 | Using specialized ReactiveList for reactive support over standard Java List . |
2 | Usually notifyModification() is required to be called when a field is changed for reactive support |
3 | but in the case of ReactiveList this is handled automatically, like every other mutating operations performed over the field children . |
As a best-practice, it is recommended to declare reactive collection fields final as per the example shown.
|
OOPath Maven plug-in
The Kie Maven plug-in offers a new goal injectreactive
to instrument bytecode and automatically inject reactivity support for standard cases.
The injectreactive
goal is disabled by default, and can be enabled via Maven plug-in configuration instrument-enabled
settings.
<groupId>org.kie</groupId>
<artifactId>kie-maven-plugin</artifactId>
<extensions>true</extensions>
<configuration>
<instrument-enabled>true</instrument-enabled> (1)
</configuration>
1 | Enable the injectreactive goal. |
The injectreactive
goal will instrument bytecode pertaining to the Maven project build’s output directory ${project.build.outputDirectory}
.
It is possible to limit the scope of the goal to a specific package or hierarchy of packages via Maven plug-in
configuration instrument-packages
settings list.
.Example:
<groupId>org.kie</groupId>
<artifactId>kie-maven-plugin</artifactId>
<extensions>true</extensions>
<configuration>
<instrument-enabled>true</instrument-enabled>
<instrument-packages>
<instrumentPackage>to.instrument</instrumentPackage> (1)
<instrumentPackage>org.drools.compiler.xpath.tobeinstrumented.*</instrumentPackage> (2)
</instrument-packages>
</configuration>
1 | Limit scope of instrumentation specifically to to.instrument package only. |
2 | Limit scope of instrumentation to org.drools.compiler.xpath.tobeinstrumented and its children packages. |
The plug-in will instrument bytecode for every field assignment under the following standard cases:
-
a field assignment will also trigger
notifyModification()
-
wrap any field defined as List with a
ReactiveList
-
wrap any field defined as Set with a
ReactiveSet
-
wrap any field defined as Collection with a
ReactiveCollection
In order for a field of type List/Set to be wrapped correctly, the field member of the java class must be declared specifically using either
java.util.Collection , java.util.List or java.util.Set
(declaring for instance a field as java.util.ArrayList will not be instrumented with the specialized reactive collections).
|
It is not recommended to mix manual support for reactivity (implemented manually) and the bytecode instrumentation Maven plug-in; it is better envisaged to keep the two scopes distinct, for instance by making use of the plug-in configuration to instrument only specific packages as documented above. |
The following section present detailed examples of the plug-in instrumentation.
A field assignment like in the following example:
public class Toy {
private String owner;
...
public void setOwner(String owner) {
this.owner = owner;
}
}
will be instrumented by intercepting the field assignment and triggering the notifyModification()
:
public class Toy implements ReactiveObject {
private String owner;
...
public void setOwner(final String owner) {
this.$$_drools_write_owner(owner);
}
public void $$_drools_write_owner(final String owner) {
this.owner = owner;
ReactiveObjectUtil.notifyModification((ReactiveObject) this);
}
}
Please notice this instrumentation applies only if the field is not a Collection
.
In the case the field assignment is referring a List
or a Set
, the instrumentation will wrap the assignment with a ReactiveList
or `ReactiveSet
accordingly; for example:
public class School {
private final String name;
private final List<Child> children = new ArrayList<Child>();
...
public School(String name) {
this.name = name;
}
public List<Child> getChildren() {
return children;
}
}
will be instrumented by intercepting and wrapping with ReactiveList
:
public class School implements ReactiveObject {
private final String name;
private final List<Child> children;
public School(final String name) {
this.$$_drools_write_children(new ArrayList());
this.name = name;
}
public List<Child> getChildren() {
return this.children;
}
public void $$_drools_write_children(final List list) {
this.children = (List<Child>) new ReactiveList(list);
}
24.29.1.4. PMML Support
This feature is experimental |
This feature makes use of Rule Units |
Drools now support assets that conform to a subset of the Predictive Modeling Markup Language (PMML). The following predictive model types are now supported:
-
Regression
-
Scorecard
-
Tree
Additionally, the Mining model type has partial support; with the following modes currently available:
-
Model Chain
-
Select All
-
Select First
Further modes of operation will be supported as they become available.
24.29.1.5. Soft expiration for events
When explicitly defining an event expiration in Drools 6.x, it is always considered an hard expiration, meaning that it always takes precedence on any other expiration implicitly calculated on temporal windows and constraints where the event is involved. Drools 7 also allows to specify a soft expiration for events that can be used if the inferred expiration offset is infinite. In this way it is possible to have a guaranteed expiration that is either the inferred one or the specified one if the other is missing. Moreover this implies that rule authors are not required to include a temporal constraint in all rules and then event classes can be designed even if the rules are not yet known.
By default event expiration is considered to be hard, but it is possible to change the expiration policy and define a soft expiration either annotating the event’s class as it follows:
@Role(Role.Type.EVENT)
@Expires( value = "30s", policy = TIME_SOFT )
public class MyEvent { ... }
or using a type declaration:
declare MyEvent
@role( event )
@expires(value = 30s, policy = TIME_SOFT)
end
24.29.1.6. Rule Units
This feature is experimental |
Rule units represent a purely declarative approach to partition a rules set into smaller units, binding different data sources to those units and orchestrate the execution of the individual unit. A rule unit is an aggregate of data sources, global variables and rules.
24.29.2. Business Central
Apart from the generic improvements to Business Central (listed below in a separate section), there are also some Drools-specific enhancements in Business Central.
24.29.2.1. DMN style hit policies for Decision Tables
With each Hit Policy, by default a row has priority over each row below it.
-
Unique Hit With unique hit policy each row has to be unique meaning there can be no overlap. There can never be a situation where two rows can fire, if there is the Verification feature warns about this on development time.
-
First Hit First hit fires only one row, the one that is satisfied first from top to bottom.
-
Resolved Hit Similar to First Hit, but you can for example give row 10 priority over row 5. This means you can keep the order of the rows you want for visual readability, but specify priority exceptions.
-
Rule Order Multiple rows can fire and Verification does not report about conflicts between the rows since they are expected to happen.
-
None This is the normal hit mode. Old decision tables will use this by default, but since 7.0 uses PHREAK the row order now matters. There is no migration tooling needed for the old tables. Multiple rows can fire. Verification warns about rows that conflict.
24.29.2.2. Guided Rule Editor : Support formulae in composite field constraints
Composite field constraints now support use of formulae.
When adding constraints to a Pattern the "Multiple Field Constraint" selection ("All of (and)" and "Any of (or)") supports use of formulae in addition to expressions.
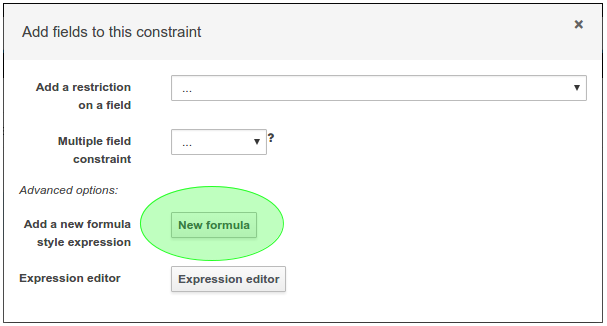
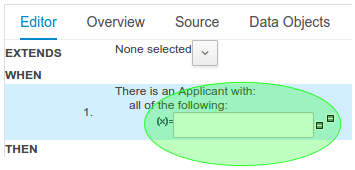
24.29.2.3. Guided Decision Table Editor : New editor
The Guided Decision Table Editor has been extensively rewritten to support editing of multiple tables in the same editor. Tables that share an association are visibly linked making it easier to visualise relationships. Associations are infered from Actions that create or update a Fact consumed by the Conditions of another table.
Highlights include:-
-
A new look and feel
-
Resizable columns
-
Reordering of columns by dragging and dropping "in table"
-
Reordering of rows by dragging and dropping "in table"
-
Repositioning of tables with drag and drop
-
Panning of view to scroll content
-
Zoomable view, so you can zoom "out" to see more content at once
-
File locks and Version History per Decision Table

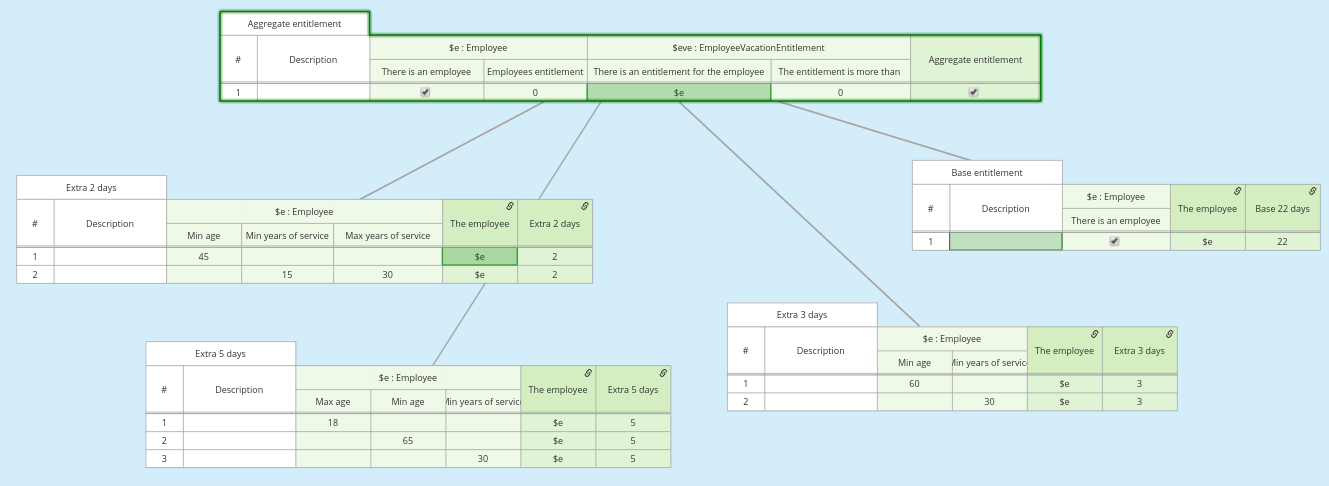
24.29.2.4. Guided Decision Table Editor : Caching of enumeration lookups
The Guided Decision Table Editor has long been capable of using enumeration definitions. However since a table can contain many cells performance of enumerations could sometimes be less than ideal if the definition required a server round-trip to retrieve the lookups from a helper class.
Results from server round-trips are now cached in the client hence removing the need for successive network calls when cells are modified. The cache is initialised when the editor is opened and populated on demand.
24.29.2.5. Guided Decision Table Editor : Verification and Validation
System Property
It is possible to disable the Verification & Validation with the system property org.kie.verification.disable-dtable-realtime-verification. This can be useful for large decision tables or if the users want to ignore V&V.
Range Checks
The verification takes the first steps towards helping you to make complete decision tables. In the next release we add the support for checking if all the ranges are covered for boolean, numeric and date values. This means if your table has a check for if an Application is approved the verification report will remind you to make sure you also handle situations where the Application was not approved.
Unique Single Hit
In the past verification and validation has raised an issue if rows subsume each other. If a row subsumes another, then the conditions can be satisfied with the same set of facts. Meaning two rows from the same table can fire at the same time. In some cases subsumption does not matter, but in other cases you want to have a table where only one rule fires at the time. The table is then a single hit decision table. To help the making of single hit tables where only one row can fire, the verification keeps an eye on the conditions. Reporting situations when single hit is broken.
24.30. Breaking changes in Drools 7.0 from 6.x
24.30.1. Property reactivity enabled by default
Property reactivity has been introduced in Drools 5.4 but users had to explicitly enable it on a class by class basis through
the @PropertyReactive
annotation or on the whole KIE base using the PropertySpecificOption.ALWAYS
builder option.
However, since using this feature is considered a good practice both under correctness and performance points of view, it has
been enabled by default in Drools 7.0. If required it is possible to disable property reactivity and reconfigure Drools 7.0
to work exactly as it did in version 6.x by adding the following configuration to the kmodule.xml file.
<configuration>
<property key="drools.propertySpecific" value="ALLOWED"/>
</configuration>
24.30.2. Type preserving accumulate functions
In Drools 6 when using the sum
function inside an accumulate pattern the result was always a Double regardless of the
field type on which the sum was performed. This caused the following 3 problems:
-
Loss of precision: the sum of a long
1881617265586265321L
will incorrectly return1.88161726558626534E18
. The BigDecimal sum of0.09
and0.01
will also be incorrect. -
Loss of performance: summing with a Double total is significantly slower than summing with a Long or an Integer.
-
Leaked complexity: it enforced the user to pattern matching on Double, or more generically (suggested choice) on Number, while it may be expected that the result of summing on a field of type Integer would be an Integer as well.
Conversely Drools 7 preserves the type of the expression on which the sum is executed, so it will be possible to directly match on that type as in:
Long(...) from accumulate(..., sum($p.getLongWeight()))
24.30.3. Renaming TimedRuleExecutionOption
The KieSession option to control when timed rules have to be automatically executed has been renamed into TimedRuleExecutionOption
fixing a typing mistake in its name which affected previous releases; the property has been aligned into drools.timedRuleExecution
.
previous releases | version 7.0.0.Final |
|
---|---|---|
KieSession option |
|
|
property |
|
|
24.30.4. Renaming and unification of configuration files
In Drools 6.x, the default Drools configuration properties were configured in two distinct files:
* drools.default.rulebase.conf
located in the META-INF folder of drools-core
* drools.default.packagebuilder.conf
located in the META-INF folder of of drools-compiler
In Drools 7.0.0, these files are unified into a single one named kie.default.properties.conf
, located in the META-INF folder of drools-core.
If you want to override the default values of these properties or add your own, you can put them in a file called kie.properties.conf
located in the META-INF folder of your project.
24.31. New and Noteworthy in KIE Workbench 7.0.0
The workbench has been updated to support Wildfly 10 and EAP7. Minimum Java requirement is JDK8.
24.31.1. New Authoring (Library)
Authoring now has a new design, with a better information organization. It’s now possible to manage (create, delete and edit) Teams (Organizational Units), list Projects in a Repository and the Assets in a Project. When an Asset is selected, you can see the Asset Editor and the Project Explorer.
The Library uses the indexing of the Workbench. It is, therefore, imperative that existing index information is deleted so that the Workbench can rebuild them with the necessary information. Index information is stored in the |
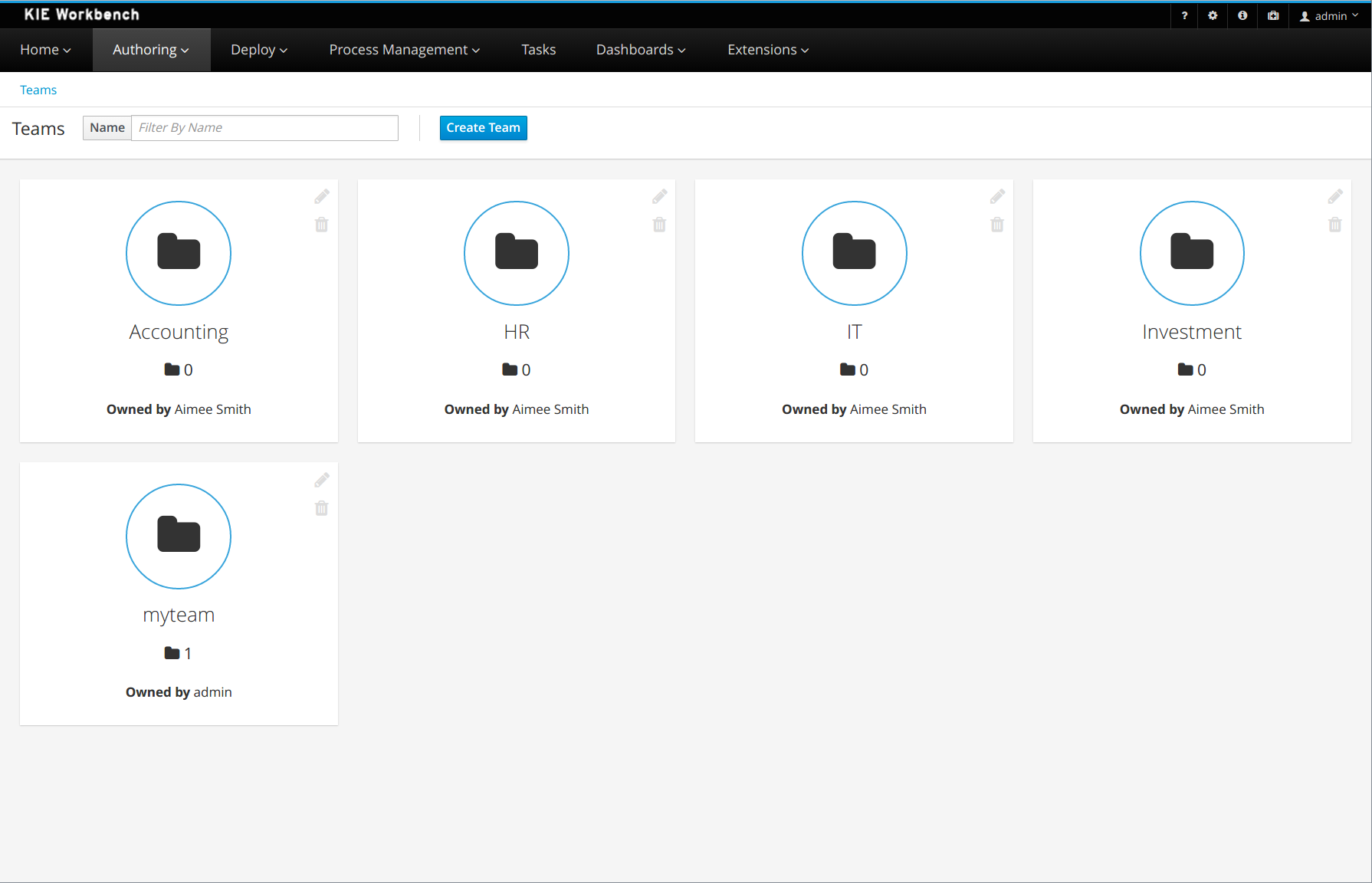
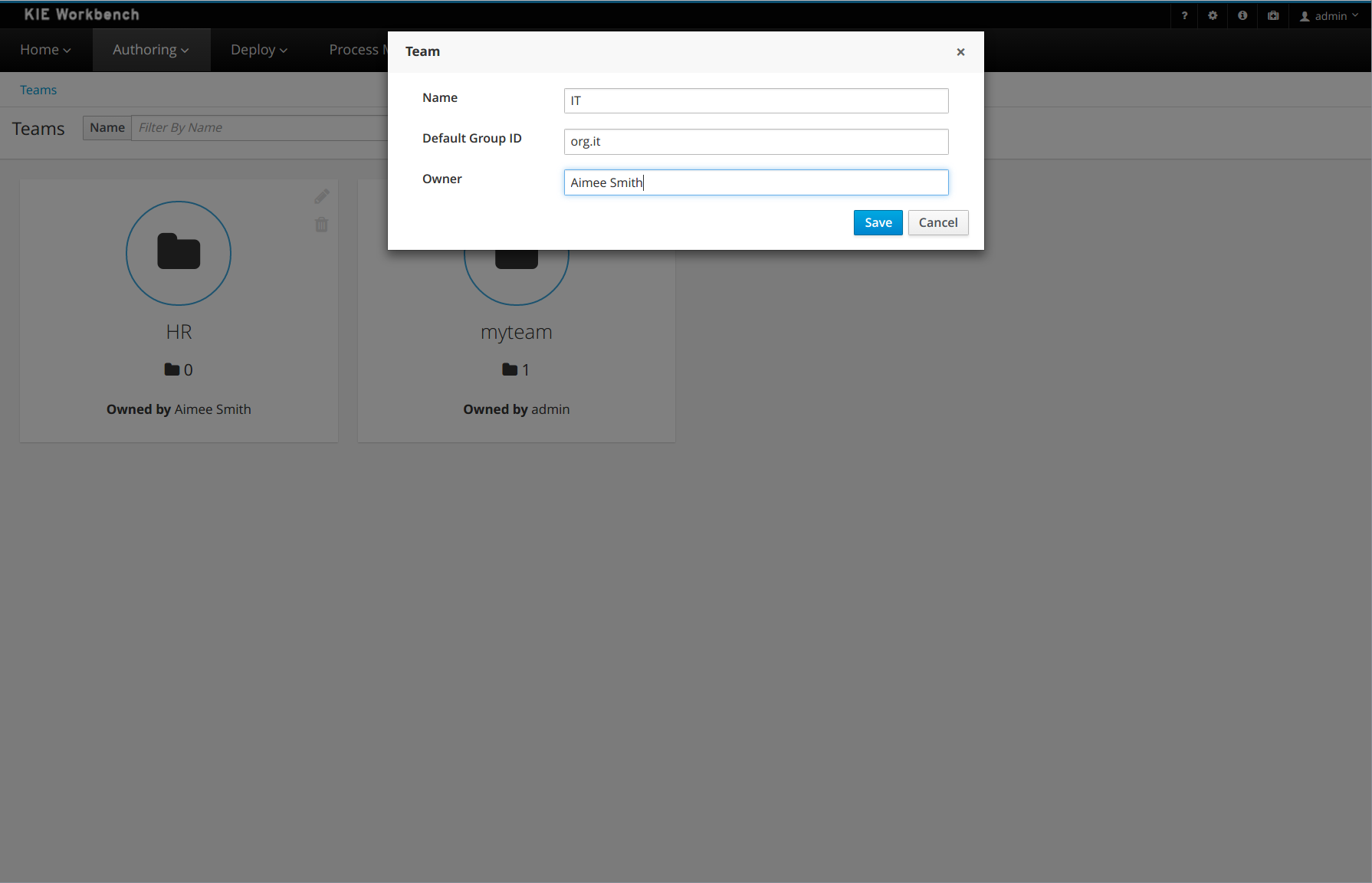
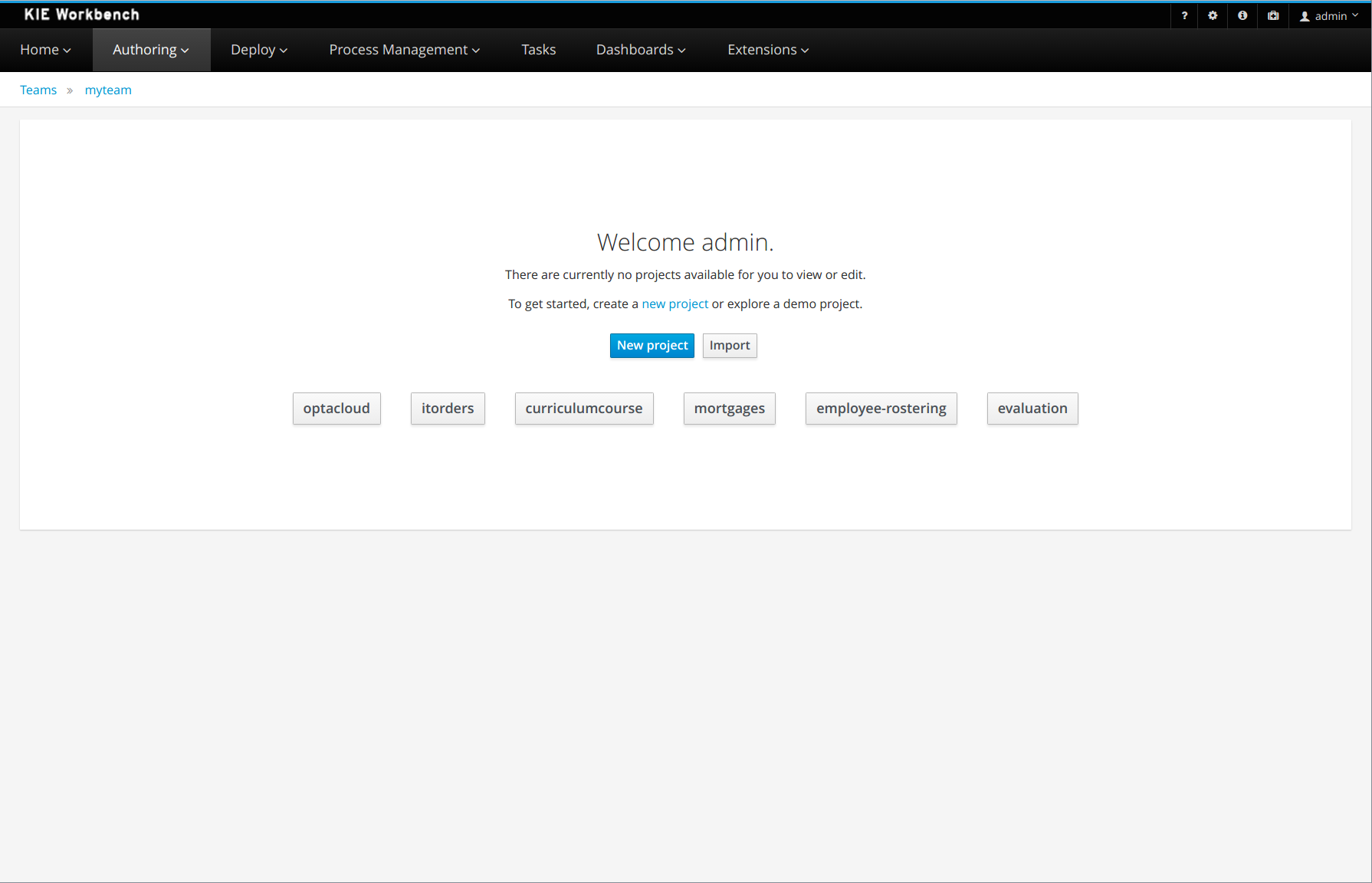
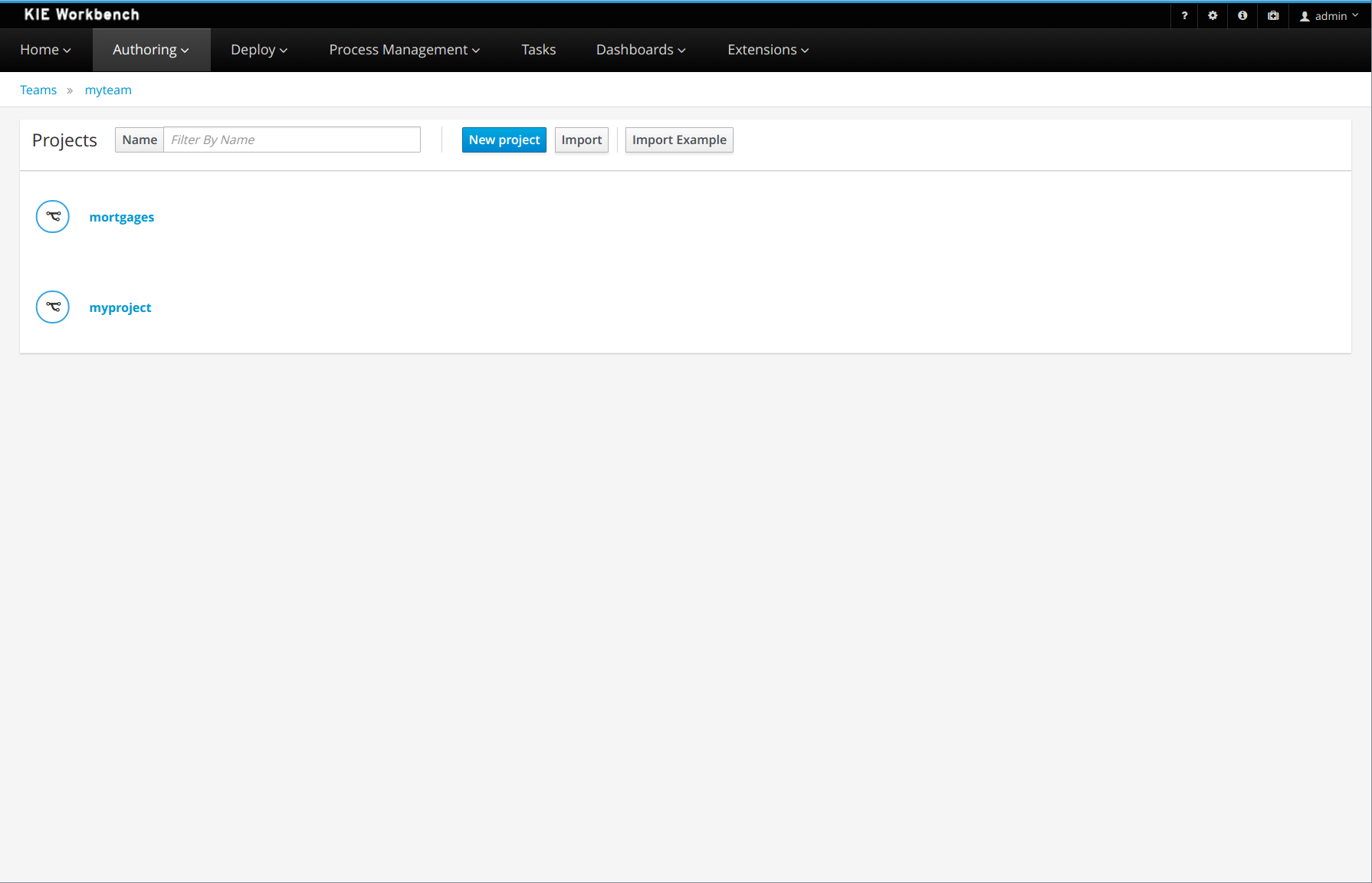
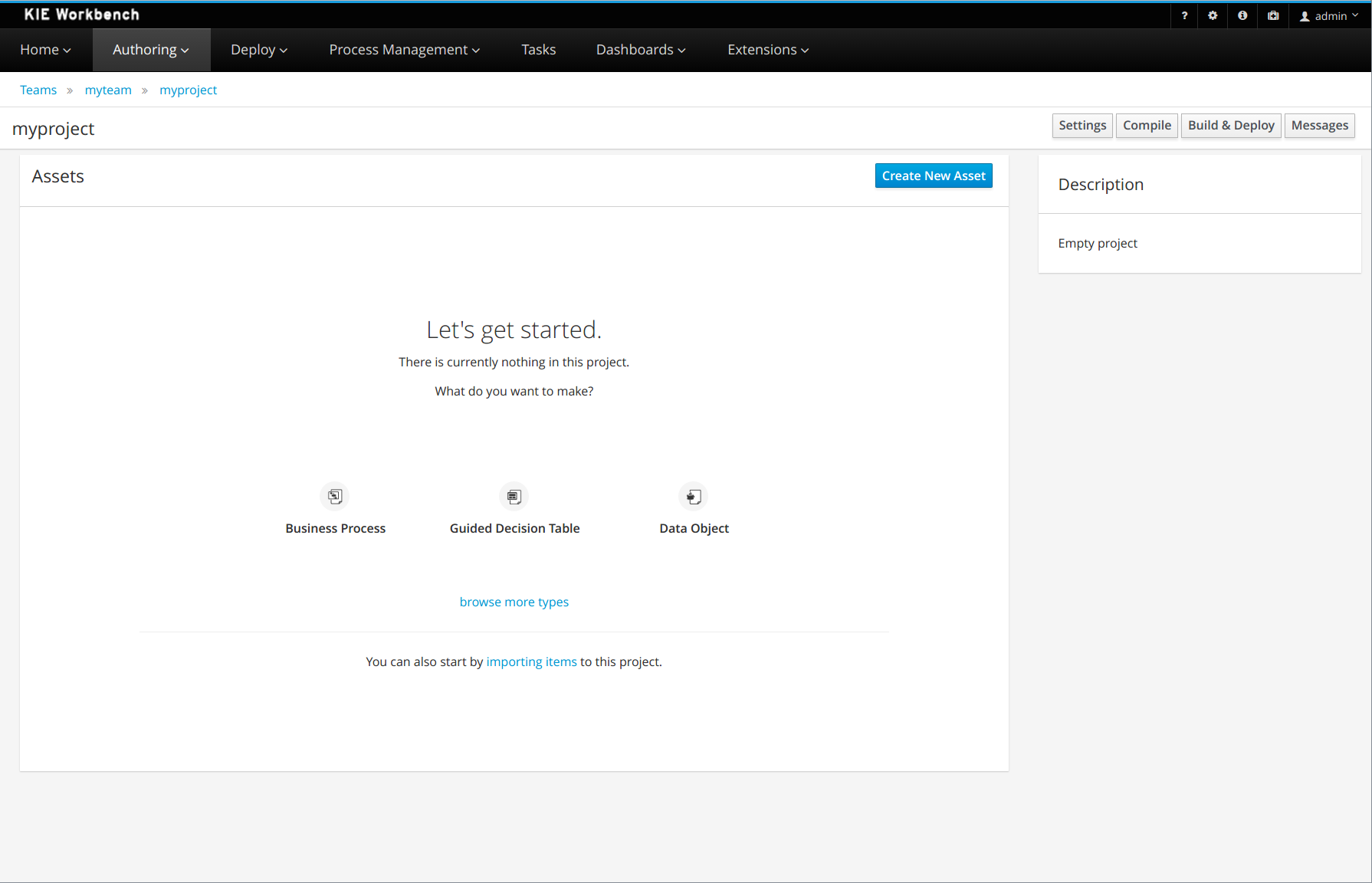
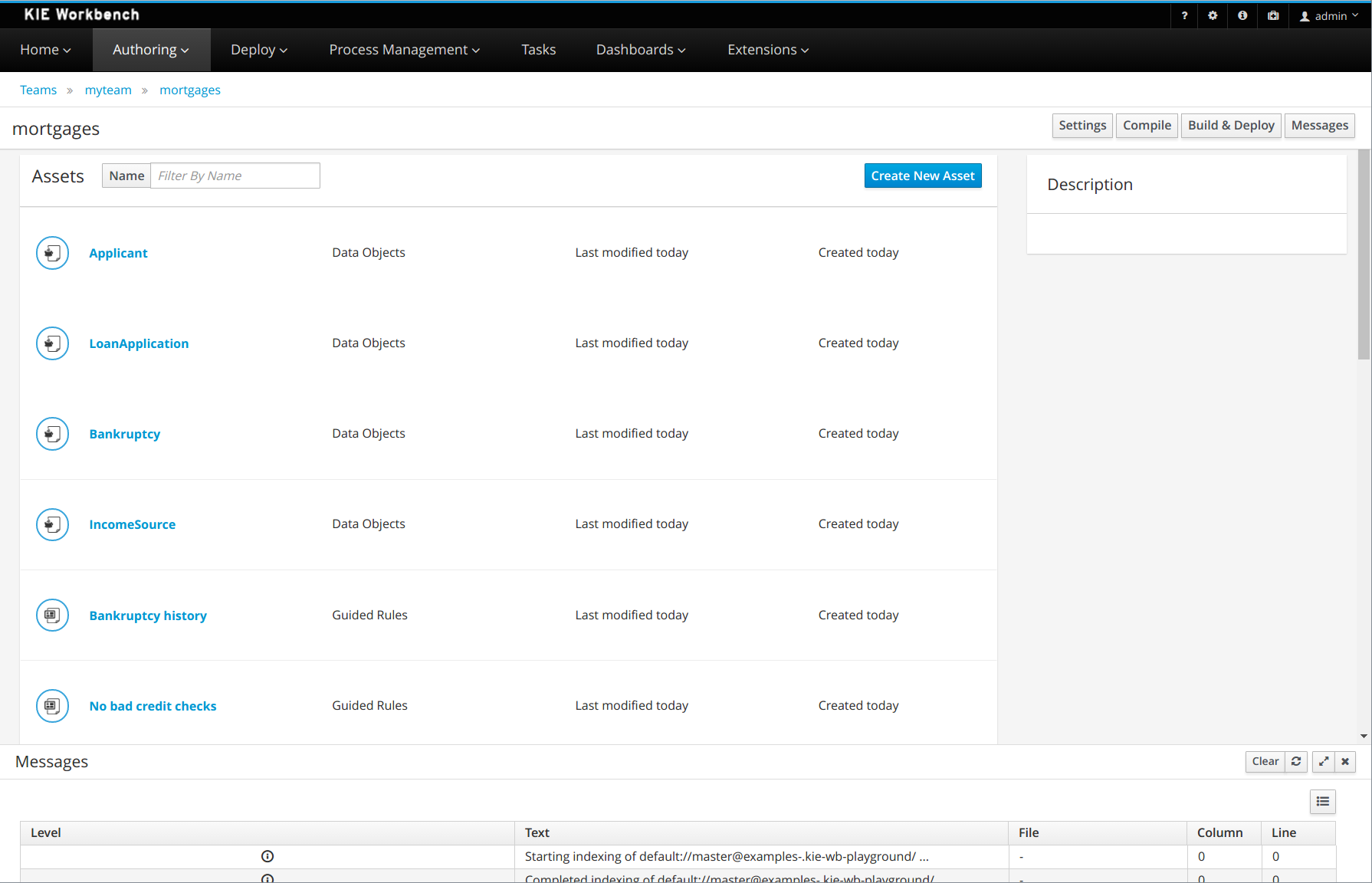
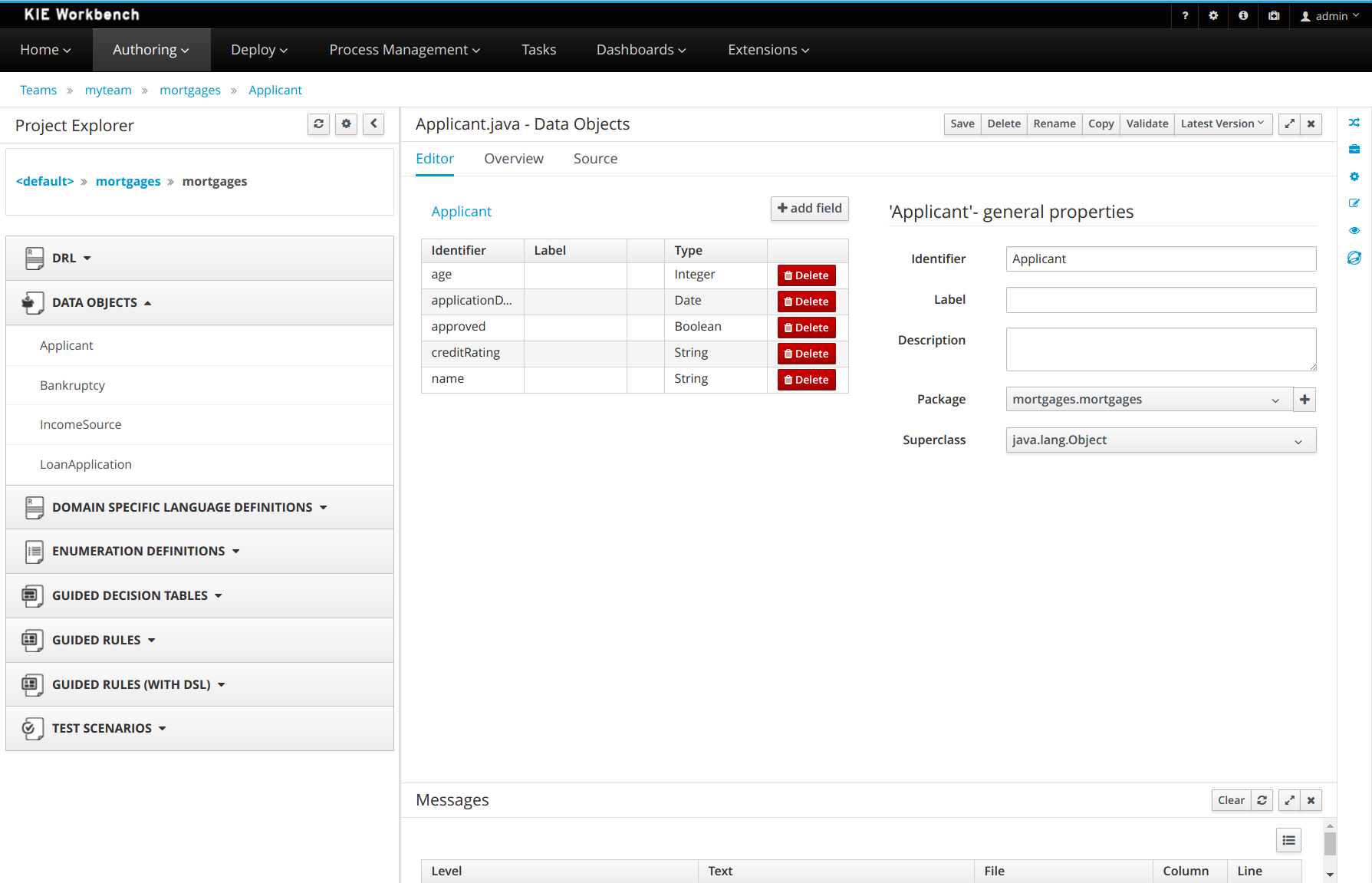
Also, you can set some preferences about your default workspace by accessing Home > Admin > Library.
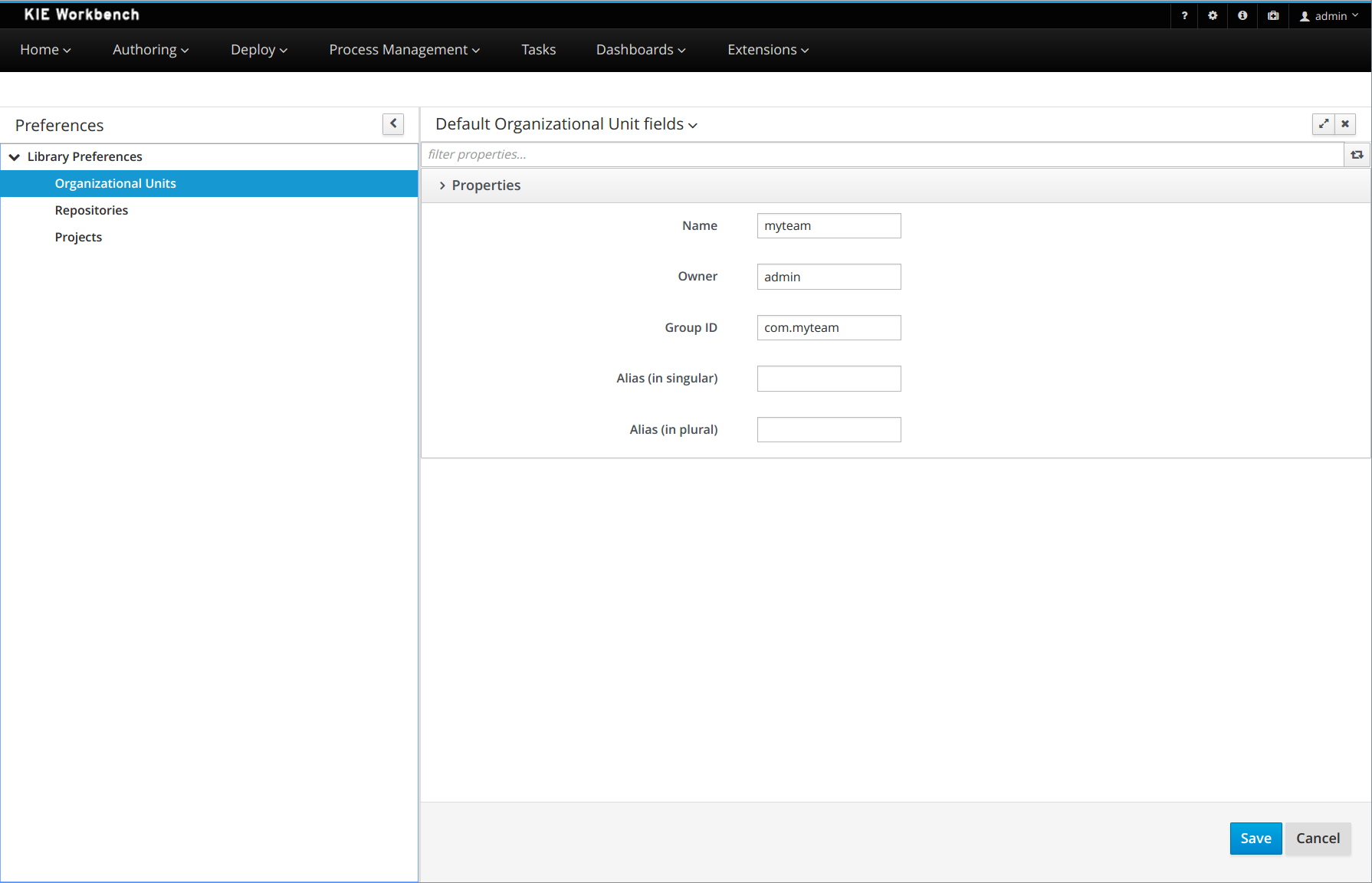
24.31.2. Authoring - Imports of Examples
Prior to 7.x the Workbench used to install pre-defined examples at startup.
Version 7.x brings the ability to import examples from git repositories. The Authoring Perspective contains a menu item for 'Examples' clicking this launches a Wizard to guide you through the import.
The Authoring Perspective contains a menu item for 'Examples'.
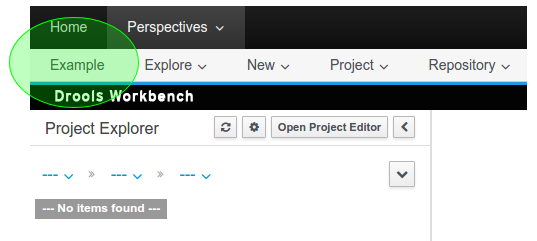
Page 1 of the Wizard allows the User to select a pre-defined examples repository, or enter their own URL.
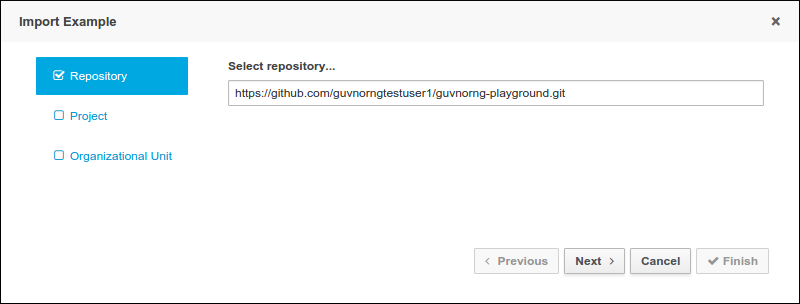
Page 2 of the Wizard lists Projects available in the source repository.
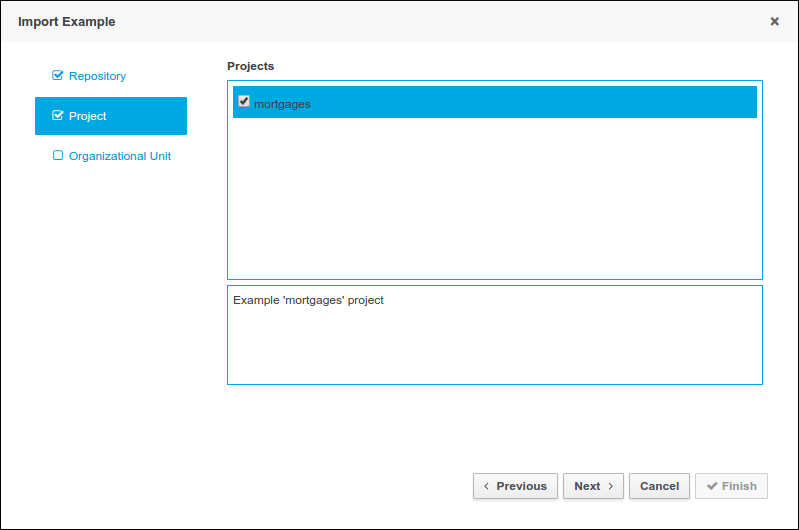
Page 3 of the Wizard allows the User to enter a target Repository name and associate it with an Organizational Unit.
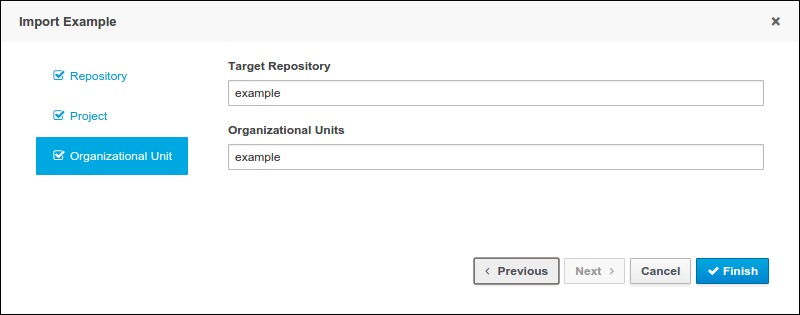
24.31.3. Authoring - Pop-ups improvements
All system pop-ups had their UX improved.
The "comment" field is hidden by default.
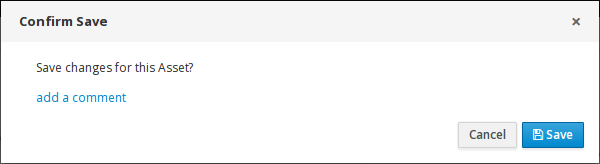
Now, the destination package can be selected when a project file is copied from any package.
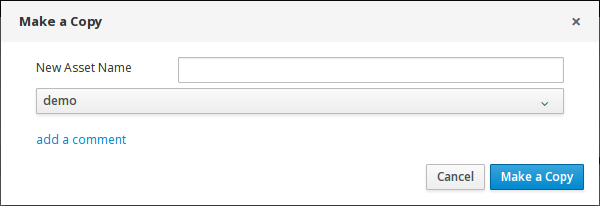
24.31.4. Authoring - Project Editor - Reimport button
The "Reimport" button invalidates all cached dependencies, in order to handle scenarios where a specific dependency was updated without having its version modified.
24.31.5. Security Management
The User and Group management perspectives released in version 6.4 have been unified into a single perspective which delivers a shared view for managing both users and groups as well as the permissions granted to any of the application roles.
This very new perspective is placed under the Home section in the top menu bar.
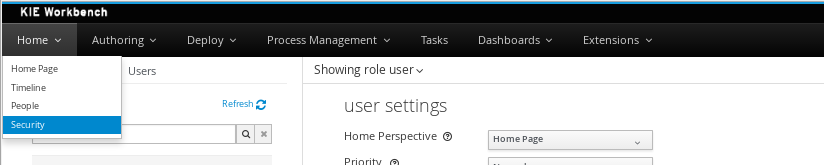
The next screenshot shows how this new perspective looks:
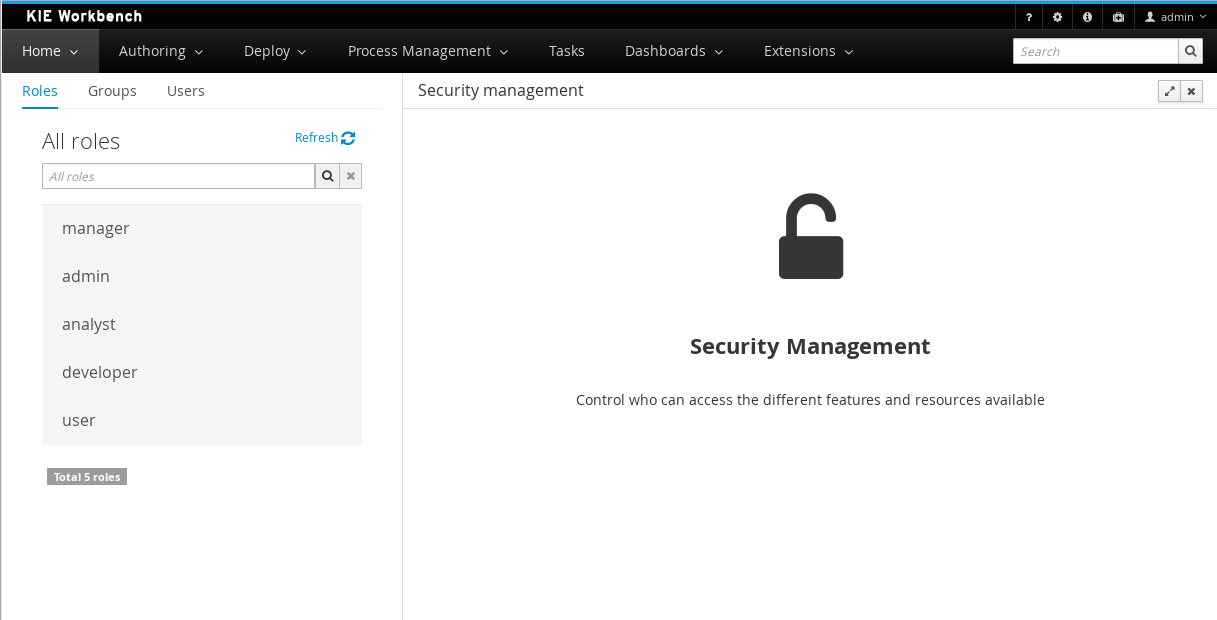
A tabbed pane is shown on the left, allowing the User to select the Roles, Groups or Users tab. After clicking on a Role (or Group) a detailed screen is displayed allowing the user to configure some security settings.
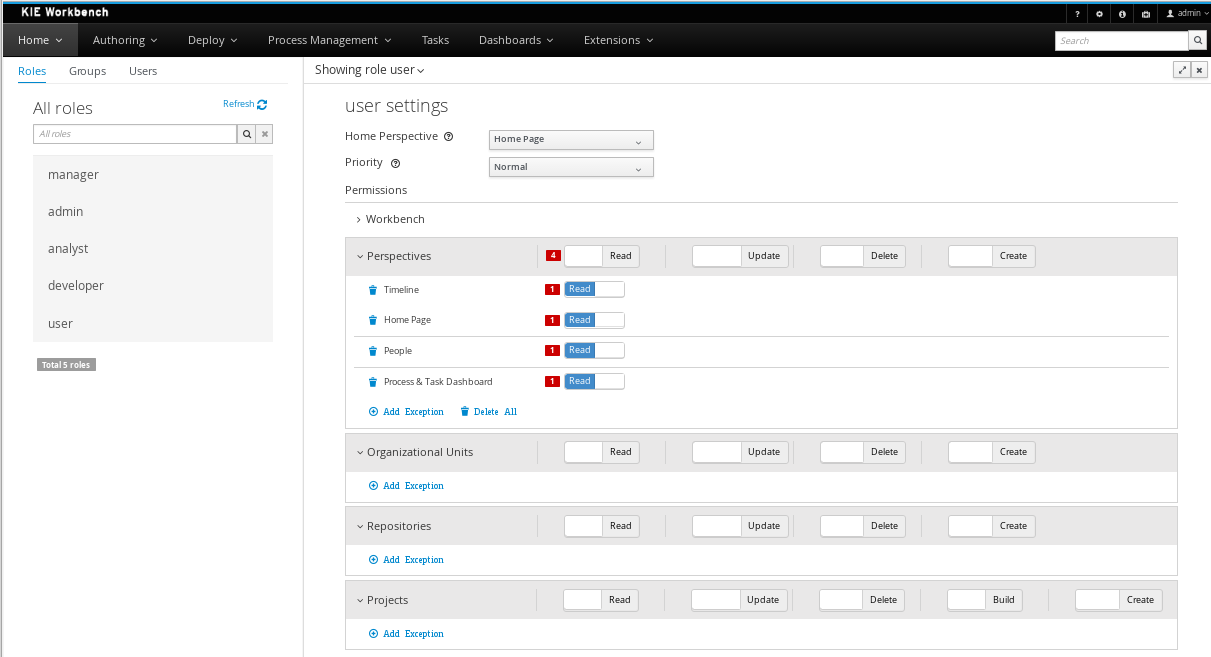
-
Home Perspective: The target perspective where the user is directed after login, which makes it possible to have different home pages per role/group.
-
Priority: Used to determine what settings (home perspective, permissions, …) have precedence for those users with more than one role or group assigned.
-
Permissions: A full ACL (Access Control List) editor for grant/deny permissions over the different resources available in the platform like Perspectives, Organizational Units, Repositories or Projects. Global permissions on top of any of those resource types can be ovewritten by means of adding individual exceptions which makes it possible to implement both the grant all deny a few or the deny all grant a few strategies.
24.31.6. kie-config-cli has been removed
The command-line tool kie-config-cli.[sh/bat]
for managing remote repositories that was present in 6.x has been removed for the following reasons:
-
The security-related operations it provided (
add-role-repo
,remove-role-repo
,add-role-org-unit
,remove-role-org-unit
,add-role-project
,remove-role-project
) have been replaced by more comprehensive Security management feature. -
The operations related to managing deployments (
list-deployment
,add-deployment
,remove-deployment
) no longer make sense, since jBPM Runtime has been removed from workbench. Deployments can still be managed programmatically using Kie Server REST API. -
The remaining operations (
create-org-unit
,remove-org-unit
,list-org-units
,create-repo
,remove-repo
,list-repo
,add-repo-org-unit
,remove-repo-org-unit
,list-project-details
) are available as a part of Knowledge Store REST API.
24.31.7. User and Project Admin Pages and Preferences
The workbench now has a new menu item: "Admin". In there, you can find some admin tools, like "Users", "Groups" and "Roles" management, and also general preferences. When a preference is changed there, it will affect all places that depend on it, but only for the logged user.
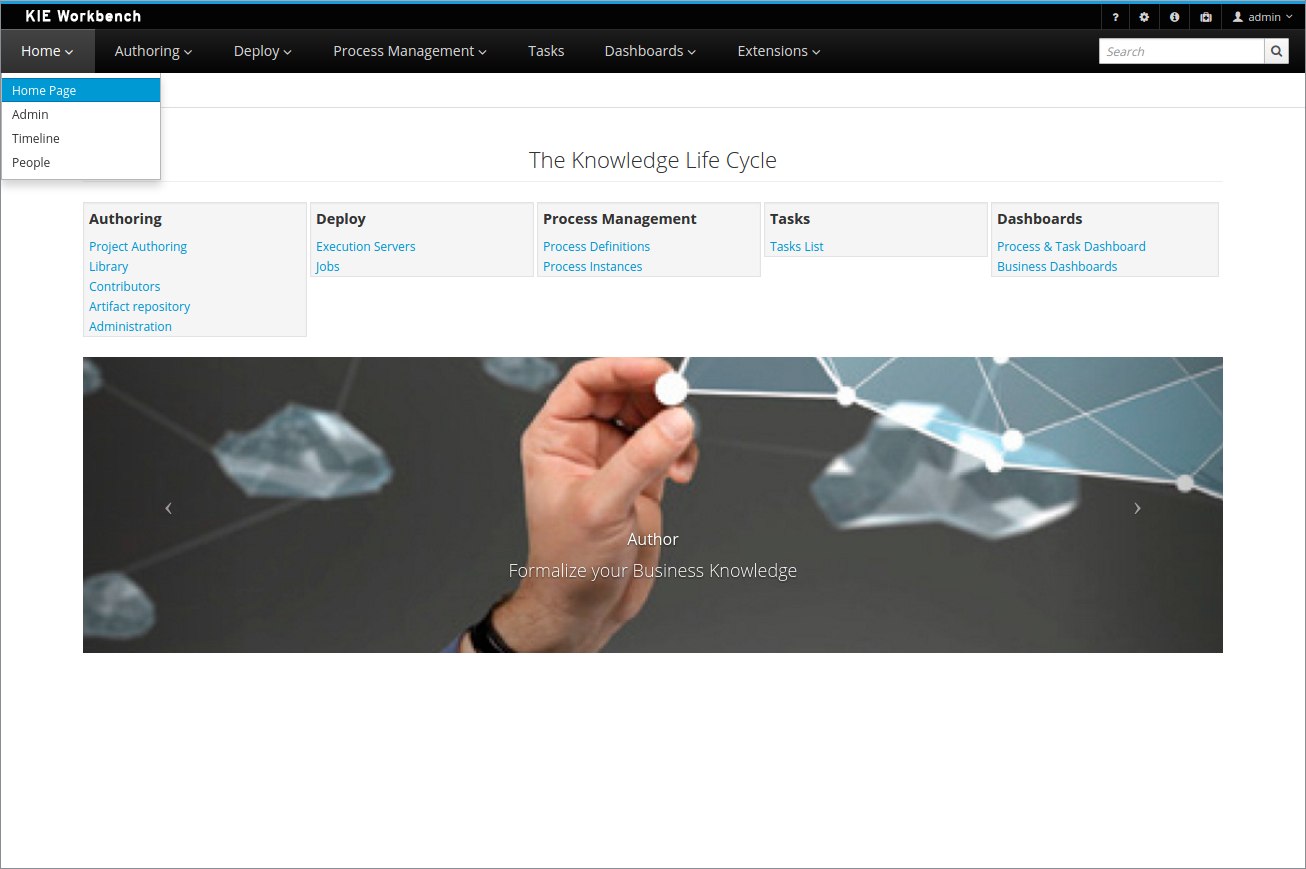
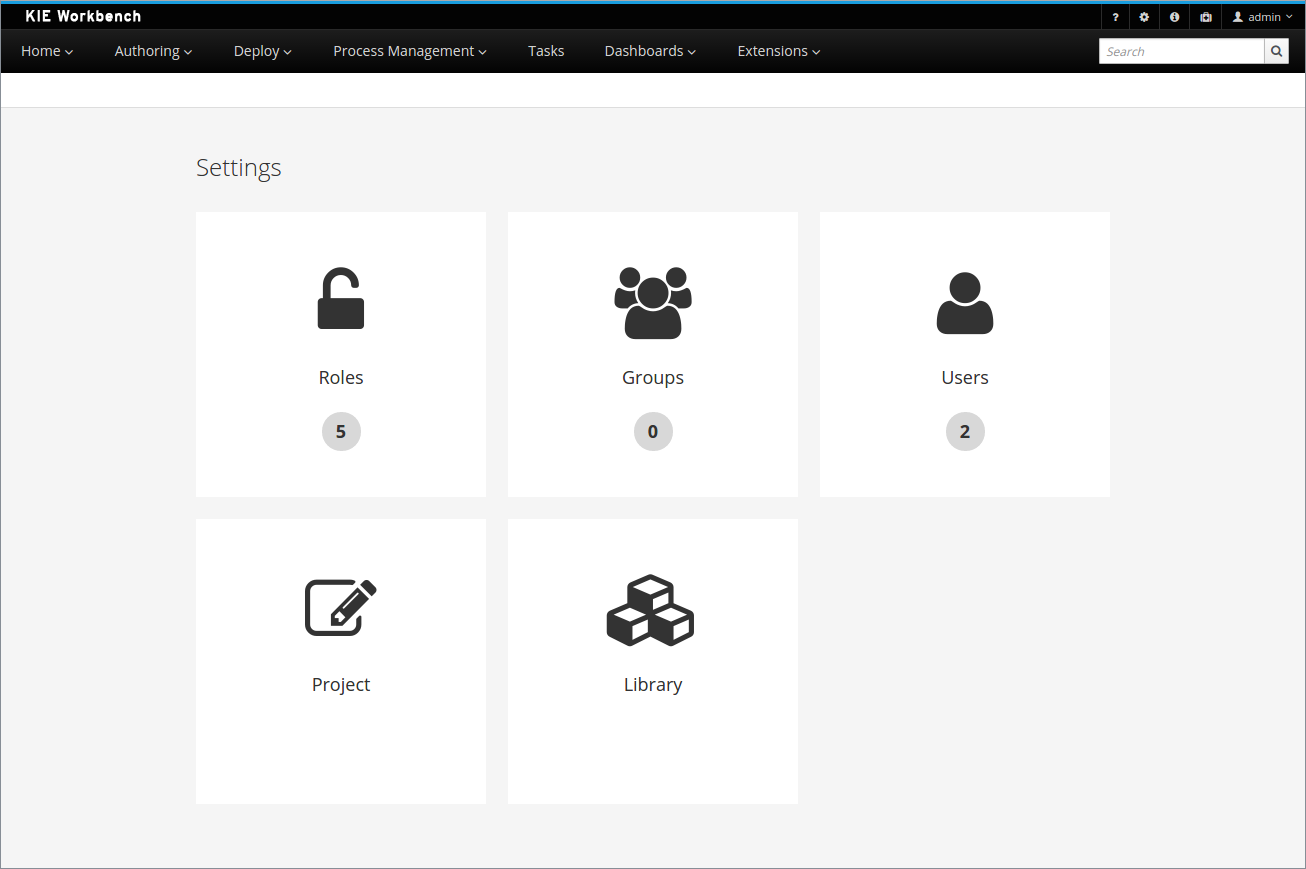
Each project also has its own admin page, with admin tools and preferences. When a preference is changed there, it will affect only that project, and only for the logged user.
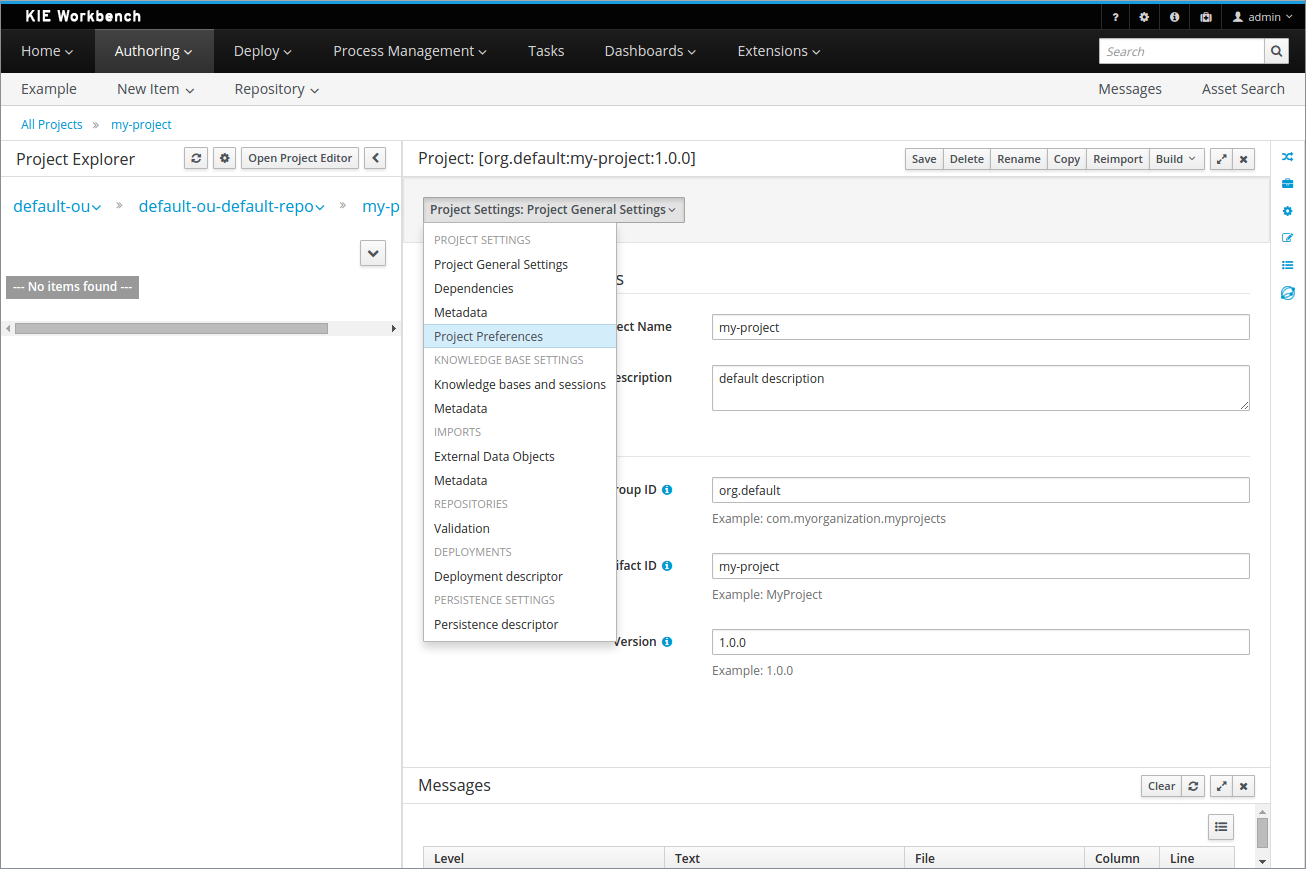
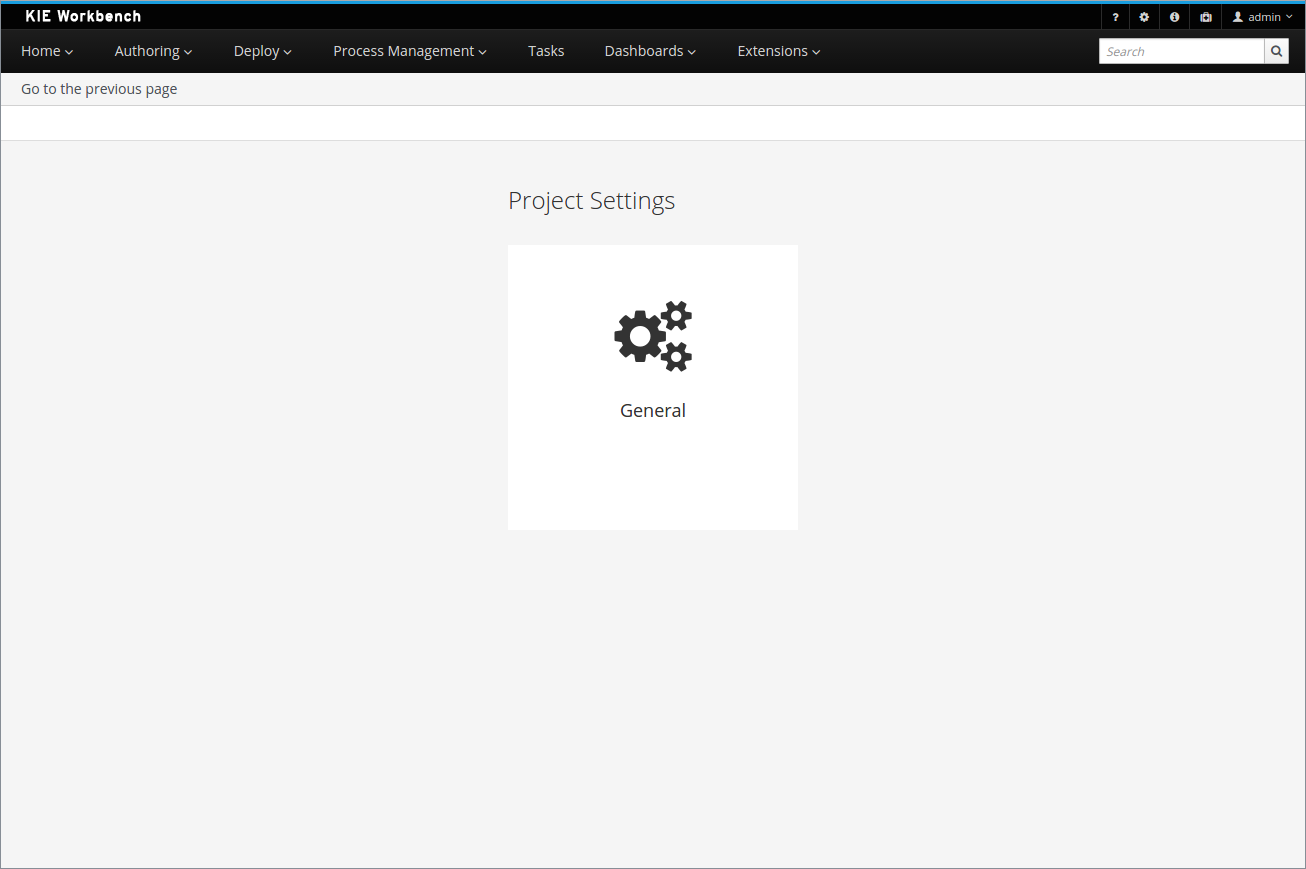
24.31.8. GAV conflict check and child GAV edition
It is now possible, for each user, to set the GAV conflict check flag, and also allow or block child GAV edition for all their projects, or specifically for each project.
The configuration can be found inside the admin tool "Project", in case the access is made through the "Admin" menu item. It can also be found by entering the admin tool "General", on the Project admin page.
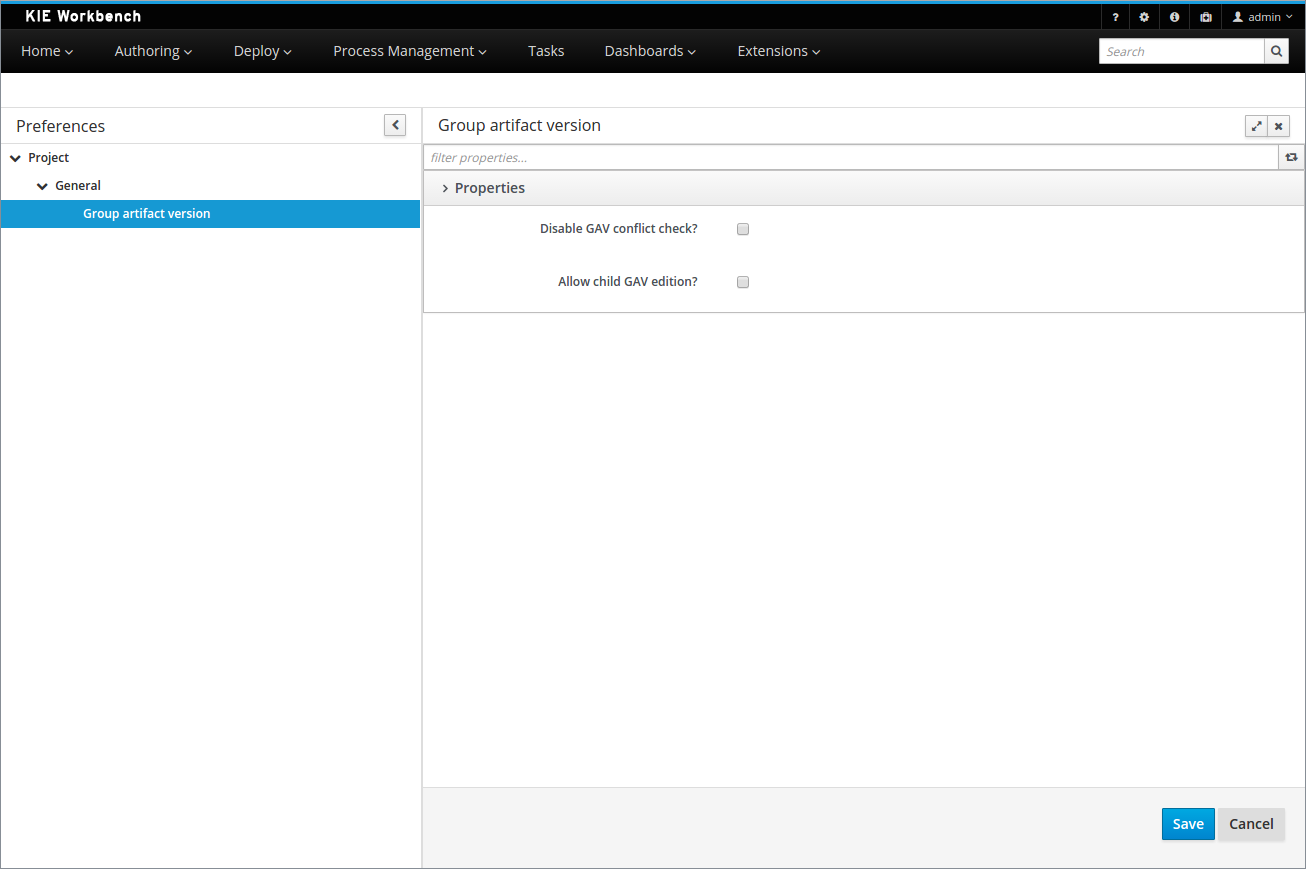
24.31.9. Data Source Management
The new data source management system empowers the workbench with the ability of defining data sources and drivers for accessing external databases.
Some of the included functionalities are:
-
A new perspective for managing the data sources:
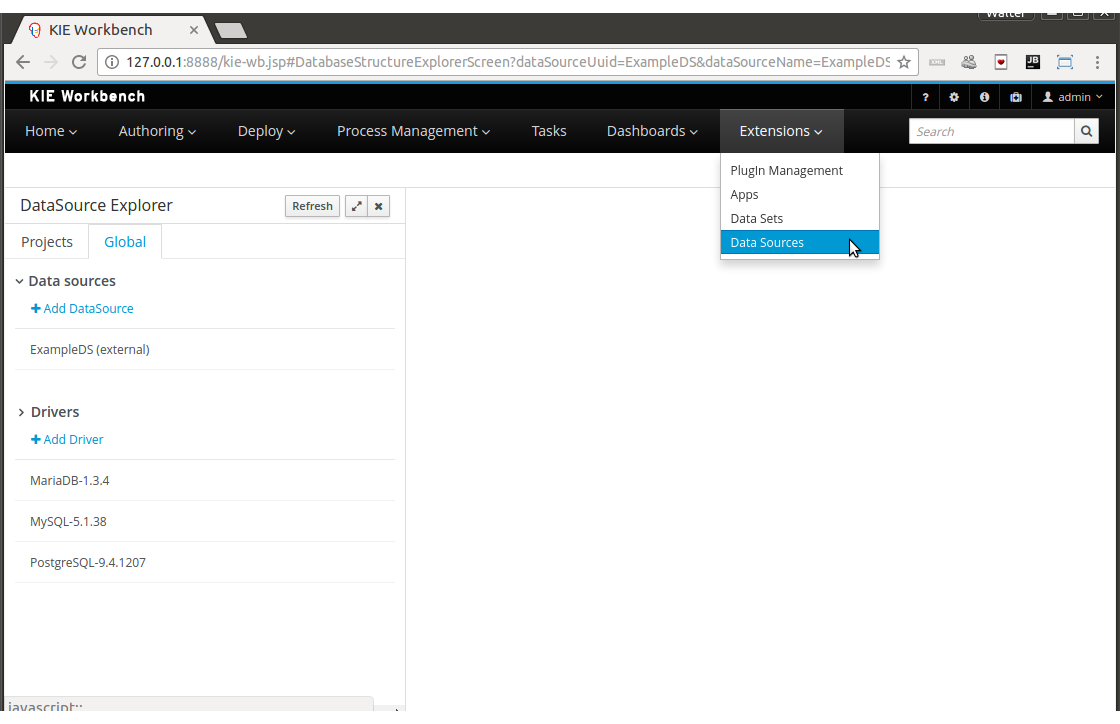
-
A new wizard for guiding the data source creation.
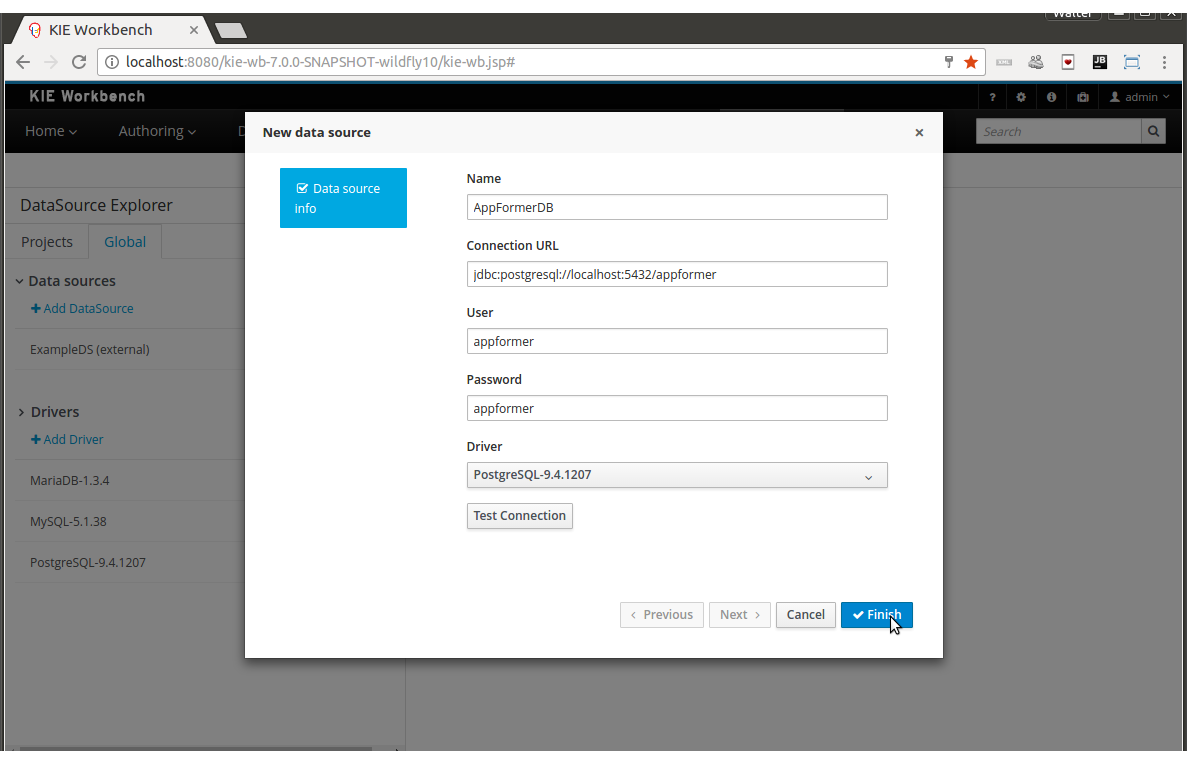
-
A new wizard for guiding the drivers creation.
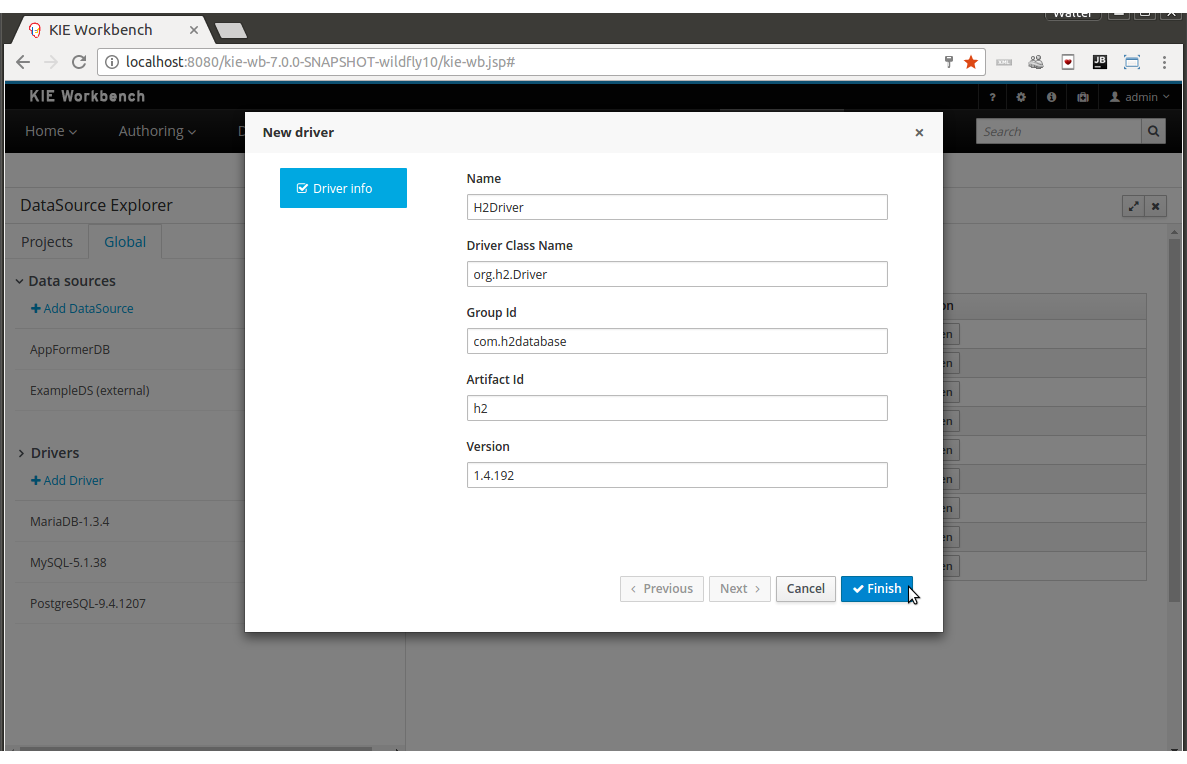
And the ability of browsing the database information for the databases pointed to by the data sources.
-
Available schemas browsing
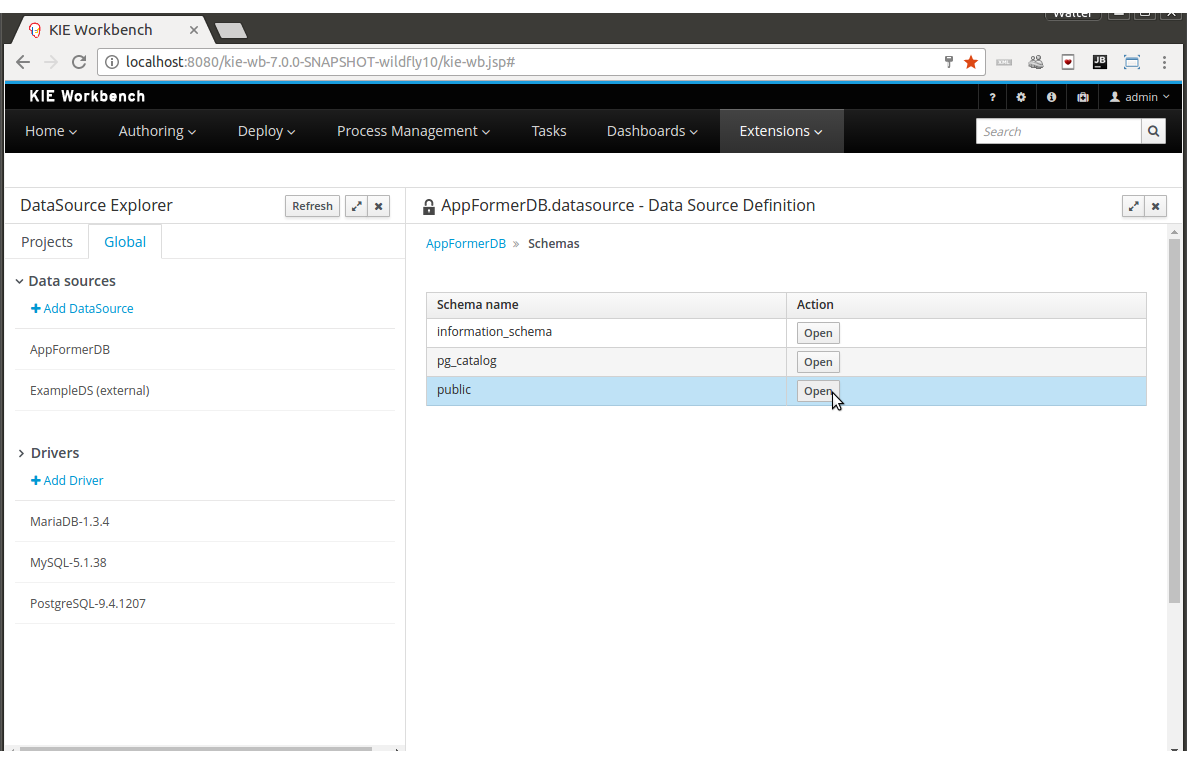
-
Available tables browsing
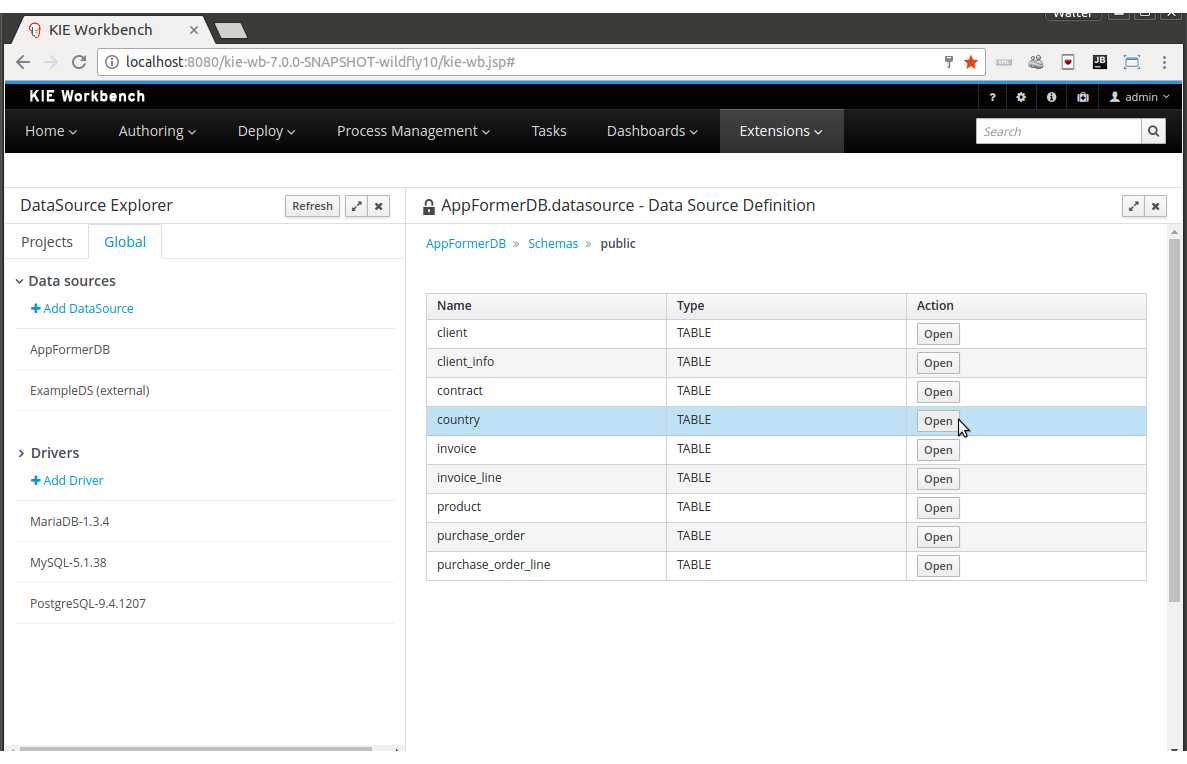
-
Table content browsing
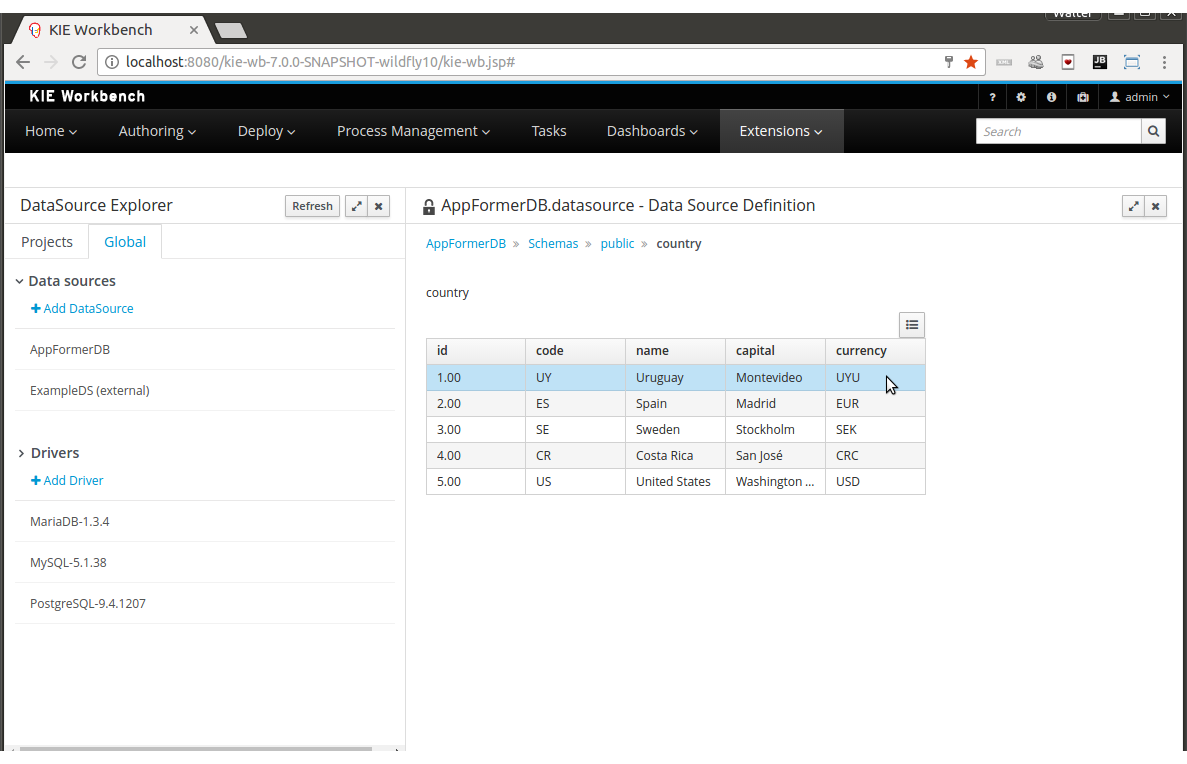
24.32. Breaking changes in Kie Server 7.0 from 6.x
24.32.1. ServiceResponse XStream marshalling changes
This release note applies only when directly interfacing with the Kie Server (kie-server) API, not when using the Kie Server Java Client (kie-server-client) API. |
In an effort to be more consistent with JAXB marshalling, XStream marshalling has undergone the following changes:
-
The XML ServiceResponse element’s
response
object no longer renders with the canonical name. -
XStream now uses
type
andmsg
as attributes, not child elements.
For more details, see DROOLS-1509.
24.32.2. Simplified Planner REST API
24.32.2.1. ServiceResponse wrapper removal
ServiceResponse
wrapper has been removed from Planner service responses returned by KIE Server.
This allows an easier processing of the responses on the client side.
<solver-instance>
...
<status>SOLVING</status>
<score scoreClass="org.optaplanner.core.api.score.buildin.hardsoft.HardSoftScore">0hard/-10soft</score>
<best-solution class="curriculumcourse.curriculumcourse.CourseSchedule">
...
</best-solution>
</solver-instance>
24.32.2.2. New Planner API overview
Register a solver:
Submit a solution:
Get the best solution:
Terminate a solver:
Dispose a solver:
24.33. What is New and Noteworthy in Drools 6.5.0
24.33.1. Configurable ThreadFactory
Some runtime environments (like for example Google App Engine) don’t allow to directly create new Threads.
For this reason it is now possible to plug your own ThreadFactory implementation by setting the system property drools.threadFactory with its class name.
For instance if you implemented your Google App Engine compatible ThreadFactory with the class com.user.project.GoogleAppEngineThreadFactory
you can make Drools to use it by setting:
drools.threadFactory = com.user.project.GoogleAppEngineThreadFactory
24.33.2. Use of any expressions as input for a query
It is now possible to use as input argument for a query both the field of a fact as in:
query contains(String $s, String $c)
$s := String( this.contains( $c ) )
end
rule PersonNamesWithA when
$p : Person()
contains( $p.name, "a"; )
then
end
and more in general any kind of valid expression like in:
query checkLength(String $s, int $l)
$s := String( length == $l )
end
rule CheckPersonNameLength when
$i : Integer()
$p : Person()
checkLength( $p.name, 1 + $i + $p.age; )
then
end
24.33.3. Update with modified properties
Property reactivity has been introduced to avoid unwanted and useless (re)evaluations and allow the Drools engine to react only to modification of properties actually constrained or bound inside of a given pattern. However this feature is automatically available only for modifications performed inside the consequence of a rule. Conversely a programmatic update is unaware of the object’s properties that have been changed, so it is unable of using this feature.
To overcome this limitation it is now possible to optionally specify in an update statement the names of the properties that have been changed in the modified object as in the following example:
Person me = new Person("me", 40);
FactHandle meHandle = ksession.insert( me );
me.setAge(41);
me.setAddress("California Avenue");
ksession.update( meHandle, me, "age", "address" );
24.33.4. Monitoring framework improvements
A new type of MBean has been introduced in order to provide monitoring of the KieContainers, and the JMX MBeans hierarchical structure have been revisited to reflect the relationship with the related MBeans of the KieBases. The JMX objectnaming has been normalized to reflect the terminology used in the Kie API. A new type of MBean has been introduced in order to provide monitoring for Stateless KieSession, which was not available in previous releases.
MBean | before 6.5.x |
from 6.5.0.Final |
---|---|---|
KieContainer |
n/a |
|
KieBase |
|
|
KieSession (stateful) |
|
|
Stateless KieSession |
n/a |
|
The KieSession MBeans consolidate the statistics data for all sessions instantiated under the same name.
KieSession created via JPAKnowledgeService
, will be monitored under a KieSession MBean having constant {ksessionName}
valorized to persistent
; this MBean is not managed by the KieContainer directly, hence it requires to be manually deregistered from JMX, when monitoring is no longer needed.
The new JMX objectnaming scheme now enforces proper JMX quoting for IDs, e.g.: org.kie:kcontainerId="2cb55f40-f220-432a-aba8-7940c18bf108",kbaseId="KBase1"
The old DroolsManagementAgent (which was registered on JMX under org.drools:type=DroolsManagementAgent
) is no longer necessary, hence no longer registered on JMX.
The KieSession MBeans now have proper JMX CompositeData
and TabularData
support, where applicable. The KieSession MBeans continue to support all process-related aggregated statistics monitoring, but no longer display start/end dates for each process instances: auditing and logging support is available in jBPM for this scope.
The Drools RHQ/JON plug-in have been changed to reflect all the above mentioned changes, in addition to specific bug-fixing aiming to display hierarchical nesting correctly.
24.34. What is New and Noteworthy in Drools 6.4.0
24.34.1. Better Java 8 compatibility
It is now possible to use Java 8 syntax (lambdas and method references) in the Right Hand Side (then) part of a rule.
24.34.2. More robust incremental compilation
The incremental compilation (dynamic rule-base update) had some relevant flaws when one or more rules with a subnetwork (rules with complex existential patterns) were involved, especially when the same subnetwork was shared among different rules. This issue required a partial rewriting of the existing incremental compilation algorithm, followed by a complete audit that has also been validated by brand new test suite made by more than 20,000 test cases only in this area.
24.34.3. Improved multi-threading behaviour
Engine’s code dealing with multi-threading has been partially rewritten in order to remove a large number of synchronisation points and improve stability and predictability. In particular this new implementation allows a clearer separation and better interaction between the User thread (performing the insert/update/delete actions on the session), the Drools engine thread (doing the proper rules evaluation) and the Timer one (performing time-based actions like events expiration).
This improvement has been made possible by the new phreak algorithm introduced with Drools 6. In fact with in the ReteOO algorithm the network evaluation is performed during the User insert/update/delete action, meaning that each user action locks the entire engine. Conversely with phreak the insert/update/delete is separated and the network evaluation happens when fireAllRules or fireUntilHalt is called.
More in detail this improvement has been made by 2 parts. First of all a new thread-safe queue has been added to store all user actions as commands. This queue is populated by the User thread while its entries are flushed and processed by the Drools engine thread during the rules evaluations phase. The second part introduced a state machine coordinating the User, Timer and Engine threads and then providing a clearer and self-documenting way to model their interactions.
24.34.4. OOPath improvements
This feature is experimental |
OOPath has been introduced with Drools 6.3.0. In Drools 6.4.0 it has been enhanced to support the following features:
-
A constraint can also have a beckreference to an object of the graph traversed before the currently iterated one. For example the following OOPath:
Student( $grade: /plan/exams/grades{ result > ../averageResult } )
will match only the grades having a result above the average for the passed exam.
-
A constraint can also recursively be another OOPath as it follows:
Student( $exam: /plan/exams{ /grades{ result > 20 } } )
-
It is also possible to use the
?/
separator instead of the/
one. As in the following example:Student( $grade: /plan/exams{ course == "Big Data" }?/grades )
By doing so the Drools engine will react to a change made to an exam, or if an exam is added to the plan, but not if a new grade is added to an existing exam. Of course if a OOPath chunk is not reactive, all remaining part of the OOPath from there till the end of the expression will be non-reactive as well. For instance the following OOPath
Student( $grade: ?/plan/exams{ course == "Big Data" }/grades )
will be completely non-reactive. For this reason it is not allowed to use the
?/
separator more than once in the same OOPath so an expression like:Student( $grade: /plan?/exams{ course == "Big Data" }?/grades )
will cause a compile time error.
24.35. New and Noteworthy in KIE Workbench 6.4.0
24.35.1. New look and feel
The general look and feel in the entire workbench has been updated to adopt PatternFly. The update brings a cleaner, lightweight and more consistent user experience throughout every screen. Allowing users focus on the data and the tasks by removing all uncessary visual elements. Interactions and behaviors remain mostly unchanged, limiting the scope of this change to visual updates.
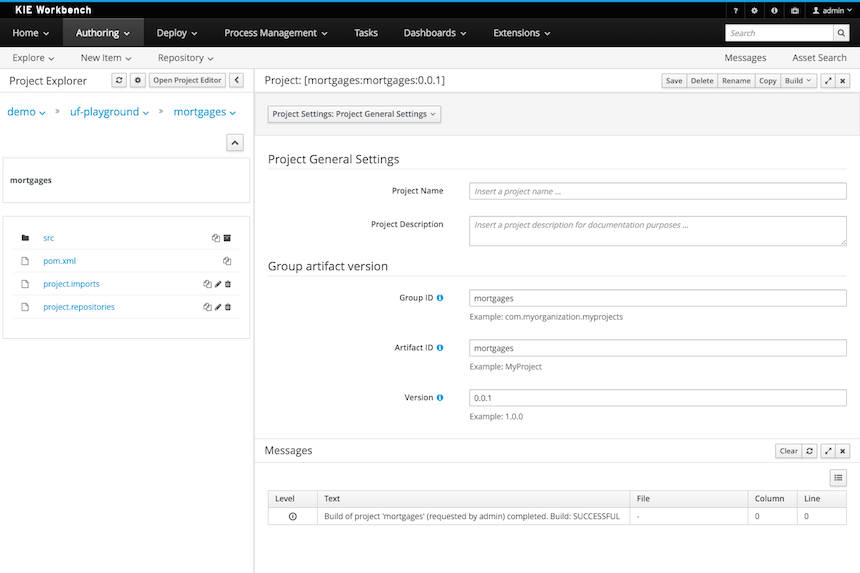
24.35.2. Various UI improvements
In addition to the PatternFly update described above which targeted the general look and feel, many individual components in the workbench have been improved to create a better user experience. This involved making sure the default size of modal popup windows is appropriate to fit the corresponding content, adjusting the size of text fields as well as aligning labels, and improving the resize behaviour of various components when used on smaller screens.
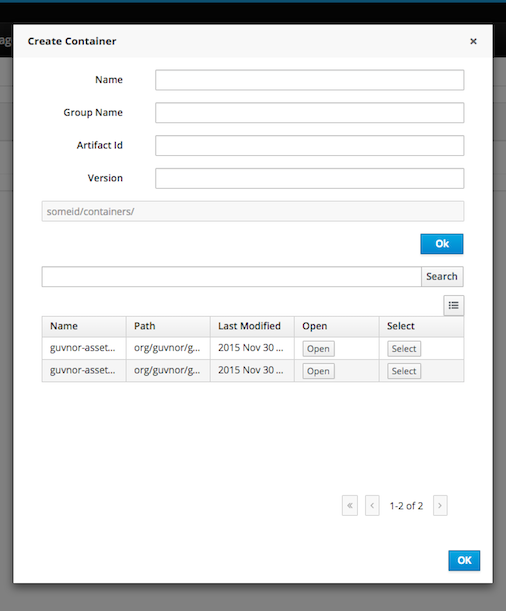
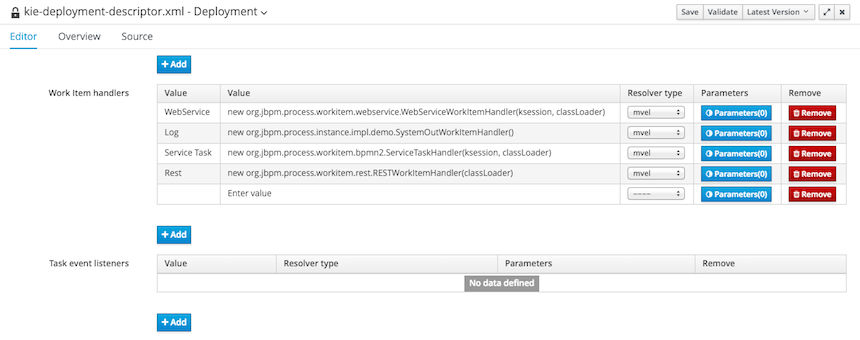
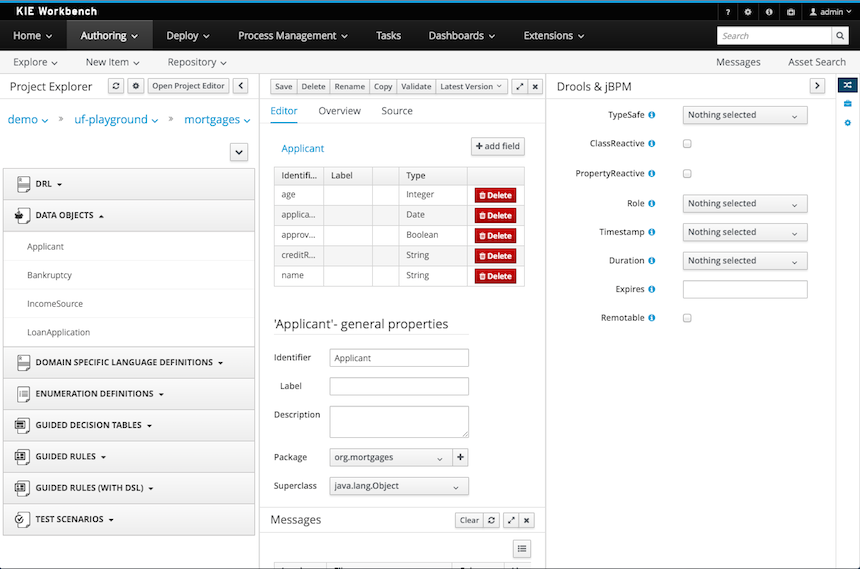
24.35.3. New locales
Locales ru
(Russian) and zh_TW
(Chineses Traditional) have now been added.
The locales now supported are:
-
Default English.
-
es
(Spanish) -
fr
(French) -
de
(German) -
ja
(Japanese) -
pt_BR
(Portuguese - Brazil) -
zh_CN
(Chinese - Simplified) -
zh_TW
(Chinese - Traditional) -
ru
(Russian)
24.35.4. Authoring - Imports - Consistent terminology
The Workbench used to have a section in the Project Editor for "Import Suggestions" which was really a way for Users to register classes provided by the Java Runtime environment to be available to Rule authoring. Furthermore Editors had a "Config" tab which was where Users were expected to import classes from other packages to that in which the rule is located.
Neither term was clear and both were inconsistent with each other and other aspects of the Workbench.
We have changed these terms to (hopefully) be clearer in their meaning and to be consistent with the "Data Object" term used in relation to authoring Java classes within the Workbench.
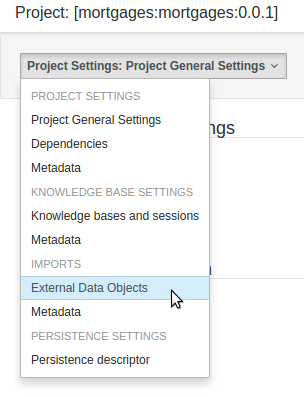
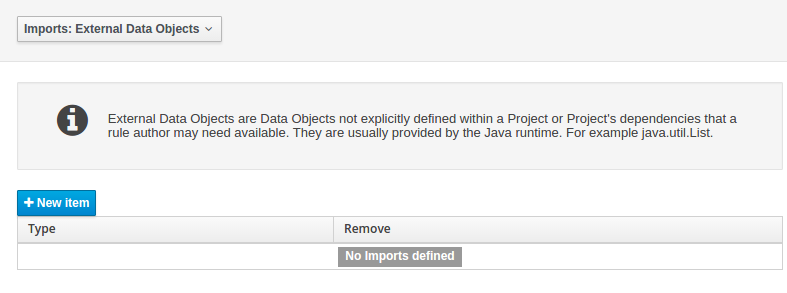
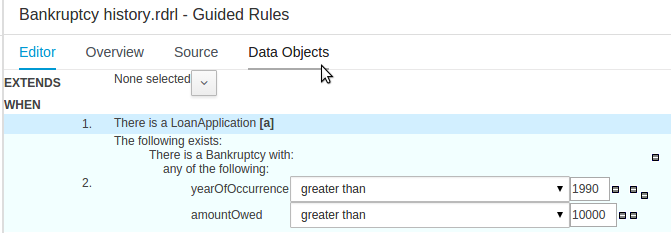
The Data Object screen lists all Data Objects in the same package as the asset and allows other Data Objects from other packages to be imported.
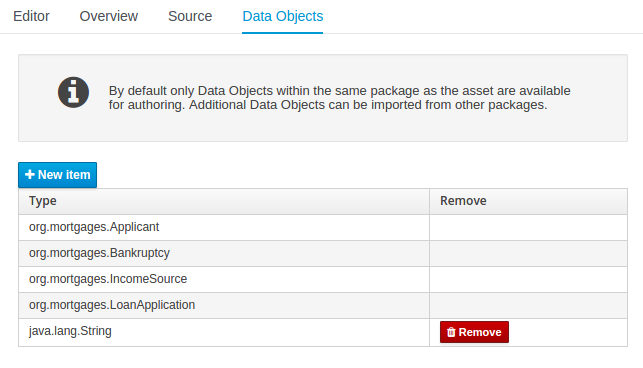
24.35.5. Disable automatic build
When navigating Projects with the Project Explorer the workbench automatically builds the selected project, displaying build messages in the Message Console.
Whilst this is beneficial it can have a detremental impact on performance of the workbench when authoring large projects.
The automatic build can now be disabled with the org.kie.build.disable-project-explorer
System Property.
Set the value to true
to disable.
The default value is false
.
24.35.6. Support for SCP
style git
Repository URLs
When cloning git
Repositories it is now possible to use SCP
style URLS, for example git@github.com:user/repository.git
.
If your Operating System’s public keystore is password protected the passphrase can be provided with the org.uberfire.nio.git.ssh.passphrase
System Property.
24.35.7. Authoring - Duplicate GAV detection
When performing any of the following operations a check is now made against all Maven Repositories, resolved for the Project, for whether the Project’s GroupId, ArtifactId and Version pre-exist.
If a clash is found the operation is prevented; although this can be overridden by Users with the admin
role.
The feature can be disabled by setting the System Property |
Resolved repositories are those discovered in:
-
The Project’s
POM
<repositories>
section (or any parentPOM
). -
The Project’s
POM
<distributionManagement>
section. -
Maven’s global
settings.xml
configuration file.
Affected operations:
-
Creation of new Managed Repositories.
-
Saving a Project defintion with the Project Editor.
-
Adding new Modules to a Managed Multi-Module Repository.
-
Saving the
pom.xml
file. -
Build & installing a Project with the Project Editor.
-
Build & deploying a Project with the Project Editor.
-
Asset Management operations building, installing or deloying Projects.
-
REST
operations creating, installing or deploying Projects.
Users with the Admin
role can override the list of Repositories checked using the "Repositories" settings in the Project Editor.
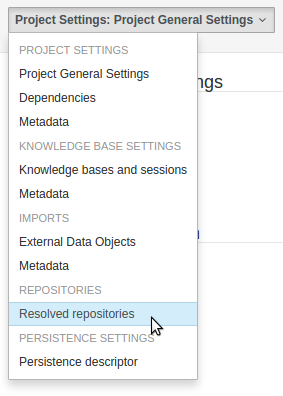
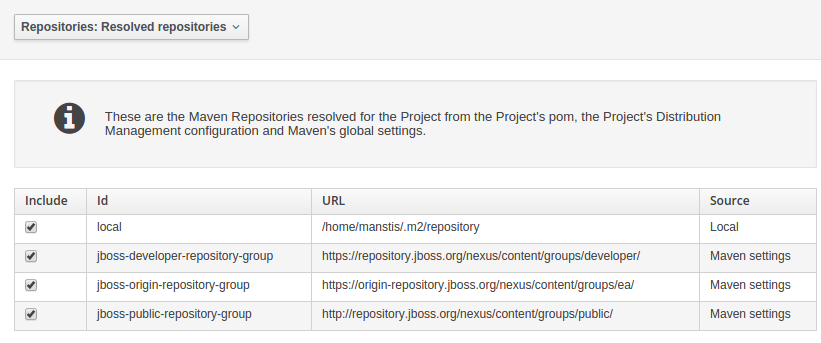
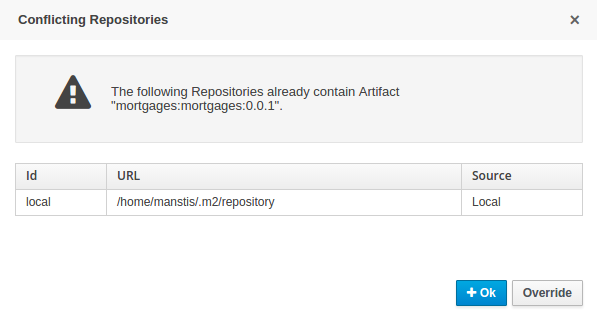
24.35.8. New Execution Server Management User Interface
The KIE Execution Server Management UI has been completely redesigned to adjust to major improvements introduced recently. Besides the fact that new UI has been built from scratch and following best practices provided by PatternFly, the new interface expands previous features giving users more control of their servers.
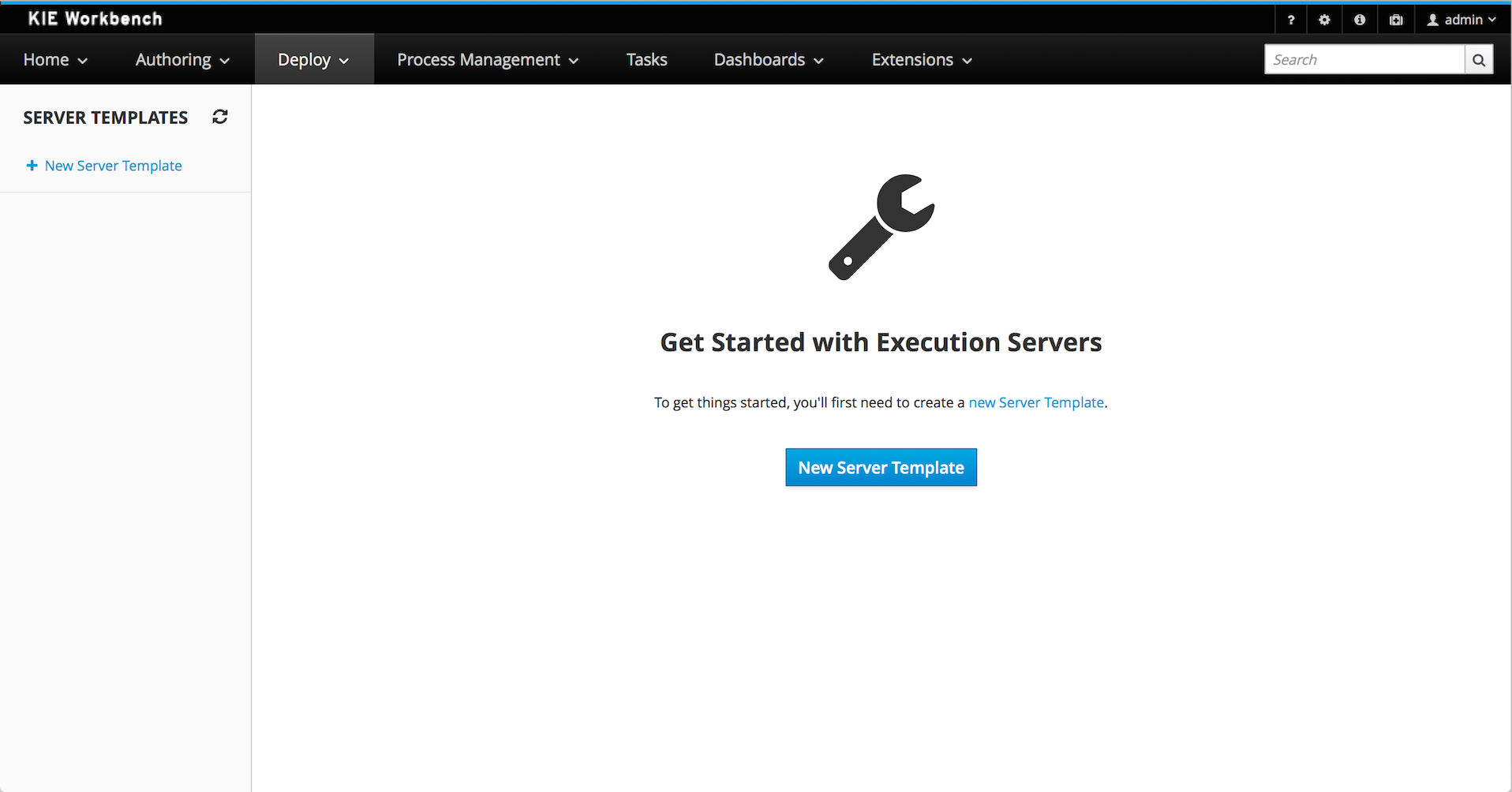
24.35.9. User and group management
Provides the backend services and an intuitive and friendly user interface that allows the workbench administrators to manage the application’s users and groups.
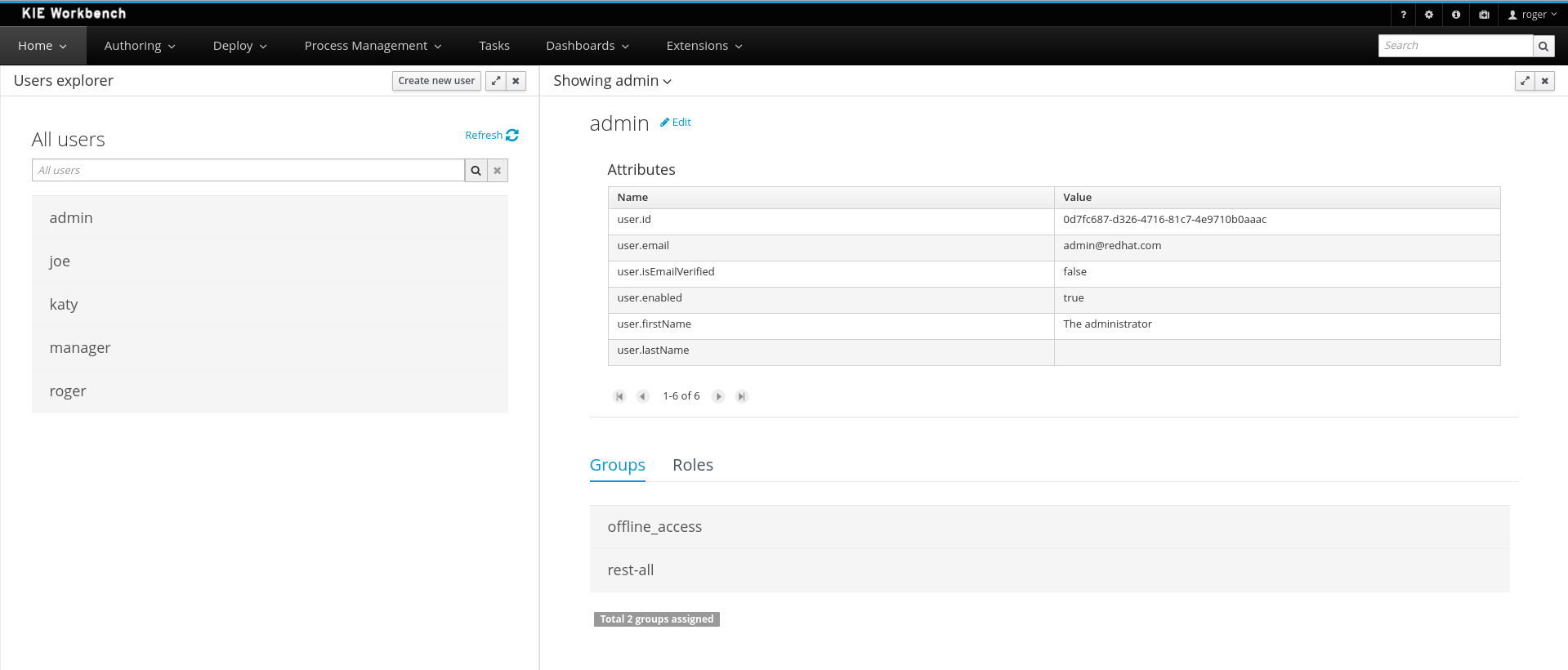
This interface provides to the workbench administrators the ability to perform realm related operations such as create users, create groups, assign groups or roles to a given user, etc.
It comes by default with built-in implementations for the administration of Wildfly, EAP and Tomcat default realms, and it’s designed to be extensible - any third party realm management system can be easily integrated into the workbench.
24.36. What is New and Noteworthy in Drools 6.3.0
24.36.1. Browsing graphs of objects with OOPath
This feature is experimental |
When the field of a fact is a collection it is possible to bind and reason over all the items in that collection on by one using the from
keyword.
Nevertheless, when it is required to browse a graph of object the extensive use of the from
conditional element may result in a verbose and cubersome syntax like in the following example:
rule "Find all grades for Big Data exam" when
$student: Student( $plan: plan )
$exam: Exam( course == "Big Data" ) from $plan.exams
$grade: Grade() from $exam.grades
then /* RHS */ end
In this example it has been assumed to use a domain model consisting of a Student
who has a Plan
of study: a Plan
can have zero or more Exam
s and an Exam
zero or more Grade
s.
Note that only the root object of the graph (the Student
in this case) needs to be in the working memory in order to make this works.
By borrowing ideas from XPath, this syntax can be made more succinct, as XPath has a compact notation for navigating through related elements while handling collections and filtering constraints.
This XPath-inspired notation has been called OOPath
since it is explictly intended to browse graph of objects.
Using this notation the former example can be rewritten as it follows:
rule "Find all grades for Big Data exam" when
Student( $grade: /plan/exams[course == "Big Data"]/grades )
then /* RHS */ end
Formally, the core grammar of an OOPath
expression can be defined in EBNF notation in this way.
OOPExpr = "/" OOPSegment { ( "/" | "." ) OOPSegment } ;
OOPSegment = [ID ( ":" | ":=" )] ID ["[" Number "]"] ["{" Constraints "}"];
In practice an OOPath
expression has the following features.
-
It has to start with
/
. -
It can dereference a single property of an object with the
.
operator -
It can dereference a multiple property of an object using the
/
operator. If a collection is returned, it will iterate over the values in the collection -
While traversing referenced objects it can filter away those not satisfying one or more constraints, written as predicate expressions between curly brackets like in:
Student( $grade: /plan/exams[course == "Big Data"]/grades )
-
Items can also be accessed by their index by putting it between square brackets like in:
Student( $grade: /plan/exams[0]/grades )
To adhere to Java convention OOPath indexes are 0-based, compared to XPath 1-based
24.36.1.1. Reactive OOPath
At the moment Drools is not able to react to updates involving a deeply nested traversed during the evaluation of an OOPath
expression.
To make these objects reactive to changes at the moment it is necessary to make them extend the class org.drools.core.phreak.ReactiveObject
.
It is planned to overcome this limitation by implementing a mechanism that automatically instruments the classes belonging to a specific domain model.
Having extendend that class, the domain objects can notify the Drools engine when one of its field has been updated by invoking the inherited method notifyModification
as in the following example:
public void setCourse(String course) {
this.course = course;
notifyModification(this);
}
In this way if an exam is moved to a different course, the rule is re-triggered and the list of grades matching the rule recomputed.
24.36.2. Kie Navigator View for Eclipse
A new viewer has been added to the Eclipse Tooling. This Kie Navigator View is used to manage Kie Server installations and projects.
24.37. New and Noteworthy in KIE Workbench 6.3.0
24.37.1. Real Time Validation and Verification for the Decision Tables
Decision tables used to have a Validation-button for validating the table. This is now removed and the table is validated after each cell value change. The validation and verification checks include:
-
Redundancy
-
Subsumption
-
Conflicts
-
Missing Columns
These checks are explained in detail in the workbench documentation.
24.37.2. Improved DRL Editor
The DRL Editor has undergone a face lift; moving from a plain TextArea to using ACE Editor and a custom DRL syntax highlighter.
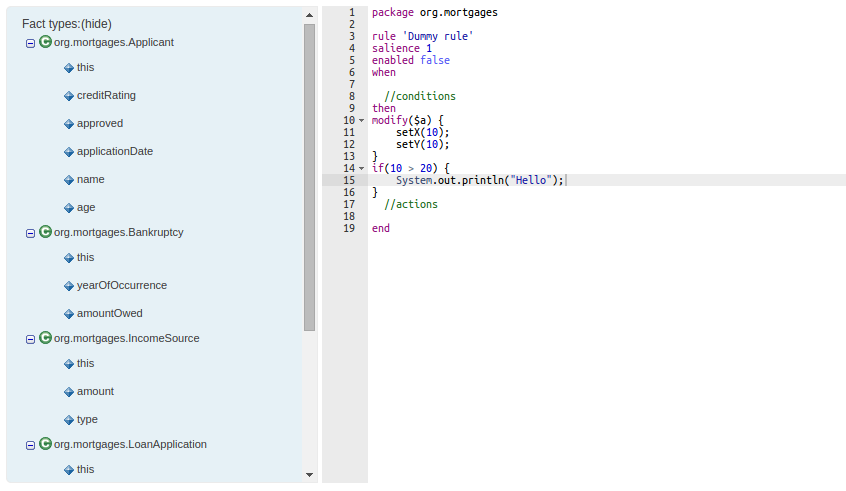
24.37.3. Asset locking
To avoid conflicts when editing assets, a new locking mechanism has been introduced that makes sure that only one user at a time can edit an asset. When a user begins to edit an asset, a lock will automatically be acquired. This is indicated by a lock symbol appearing on the asset title bar as well as in the project explorer view. If a user starts editing an already locked asset a pop-up notification will appear to inform the user that the asset can’t currently be edited, as it is being worked on by another user. As long as the editing user holds the lock, changes by other users will be prevented. Locks will automatically be released when the editing user saves or closes the asset, or logs out of the workbench. Every user further has the option to force a lock release in the metadata tab, if required.
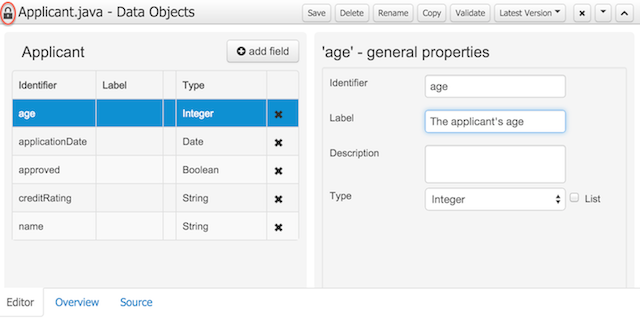
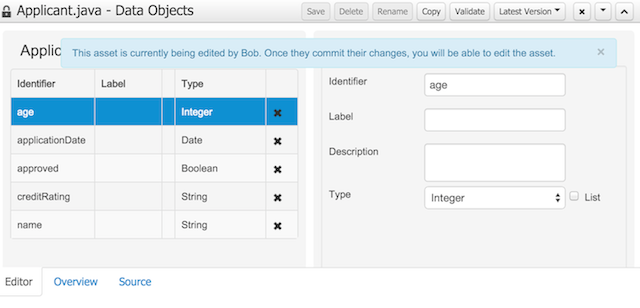
24.37.4. Data Modeller Tool Windows
Drools and jBPM configurations, Persistence (see Generation of JPA enabled Data Models) and Advanced configurations were moved into "Tool Windows". "Tool Windows" are a new concept introduced in latest Uberfire version that enables the development of context aware screens. Each "Tool Window" will contain a domain editor that will manage a set of related Data Object parameters.
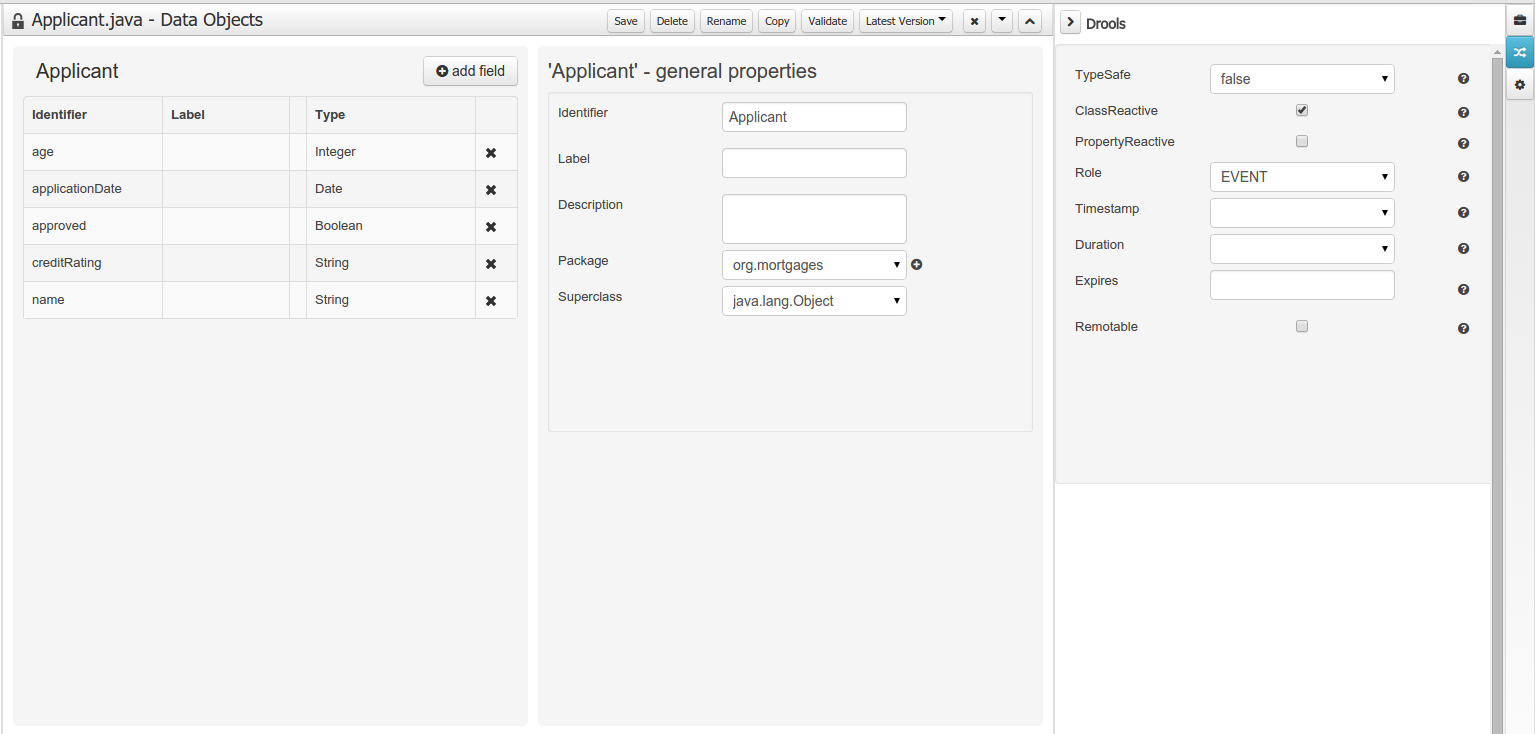
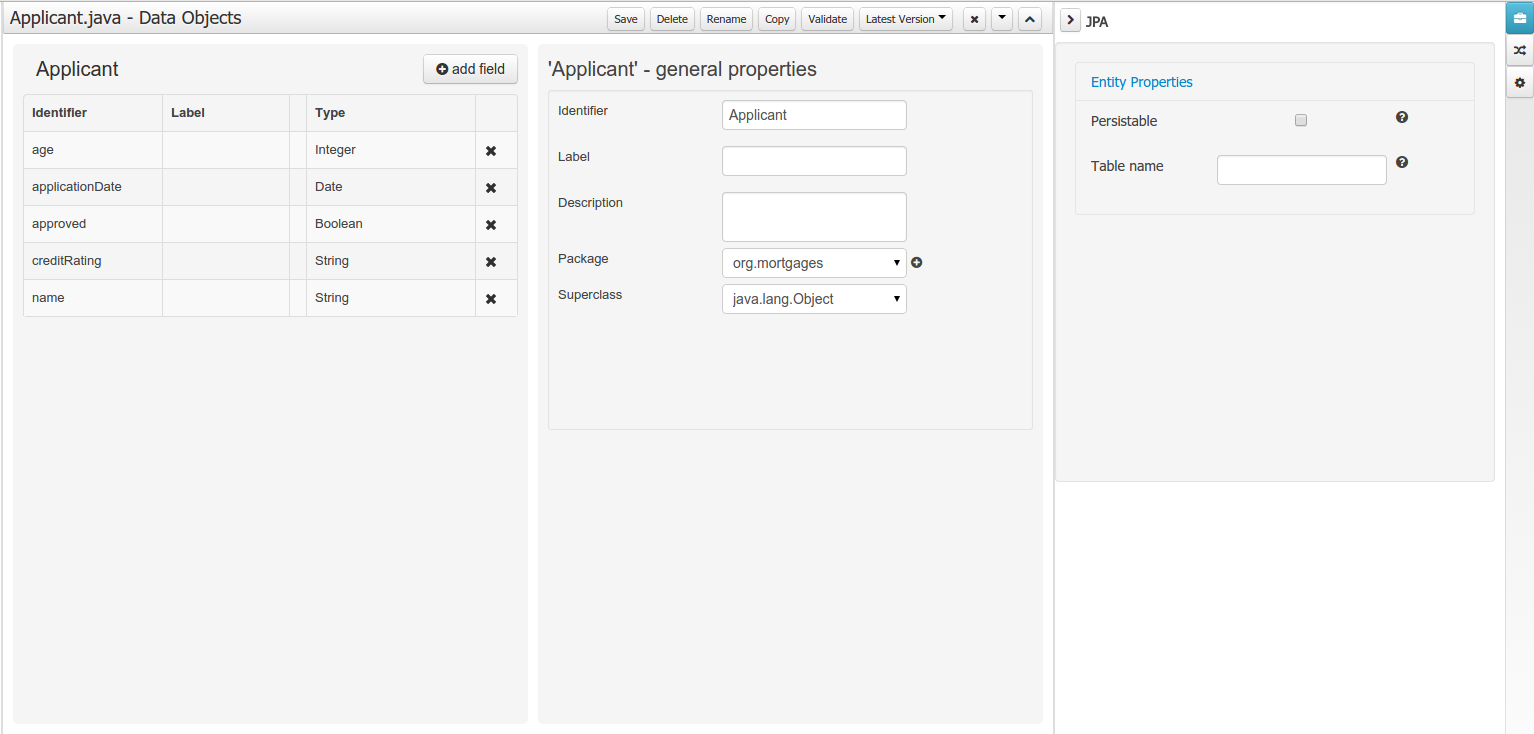
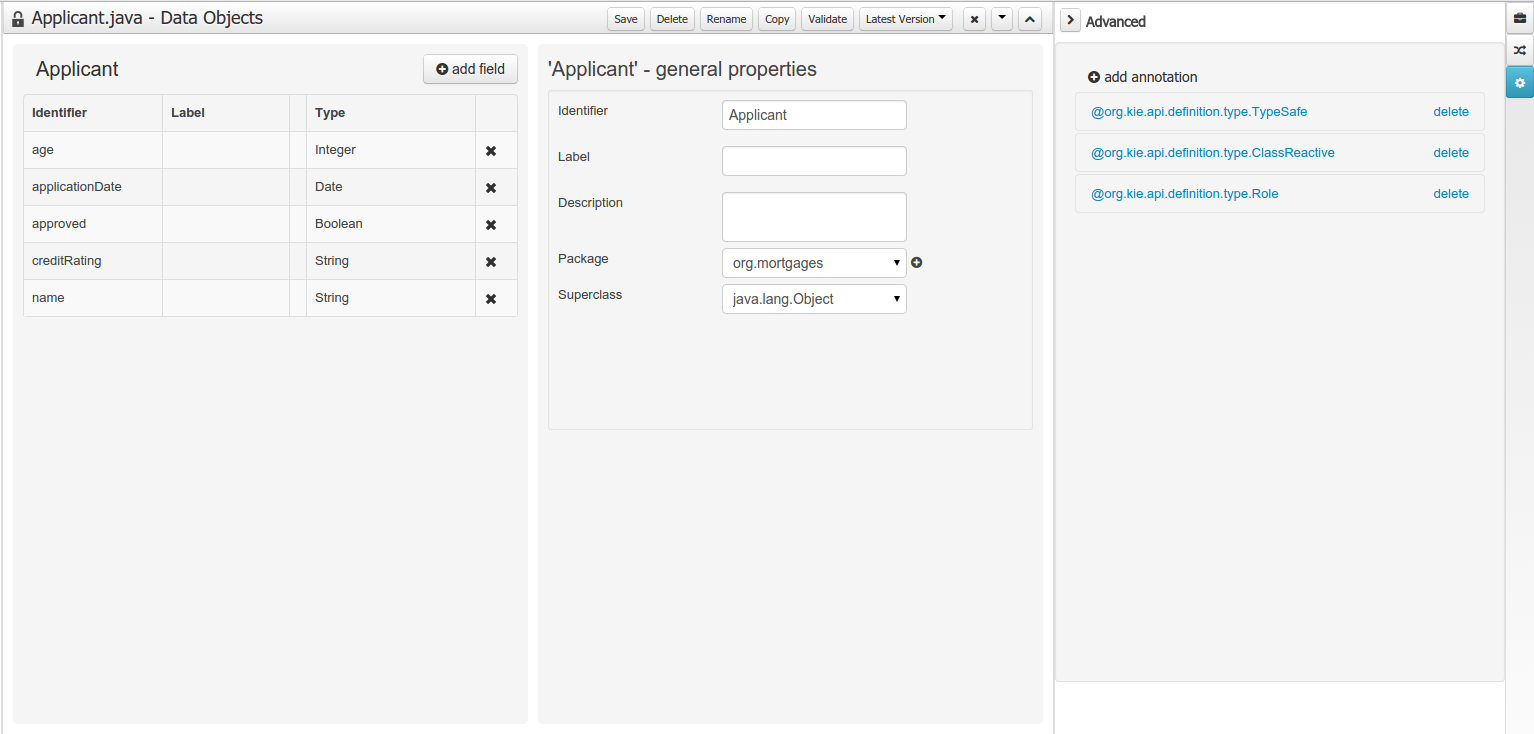
24.37.5. Generation of JPA enabled Data Models
Data modeller was extended to support the generation of persistable Data Objects. The persistable Data Objects are based on the JPA specification and all the underlying metadata are automatically generated.
-
"The New → Data Object" Data Objects can be marked as persistable at creation time.
Figure 451. New Data Object -
The Persistence tool window contains the JPA Domain editors for both Data Object and Field. Each editor will manage the by default generated JPA metadata
Figure 452. Data Object level JPA domain editorFigure 453. Field level JPA domain editor -
Persistence configuration screen was added to the project editor.
Figure 454. Persistence configuration
24.37.6. Data Set Authoring
A new perspective for authoring data set definitions has been added. Data set definitions make it possible to retrieve data from external systems like databases, CSV/Excel files or even use a Java class to generate the data. Once the data is available it can be used, for instance, to create charts and dashboards from the Perspective Editor just feeding the charts from any of the data sets available.
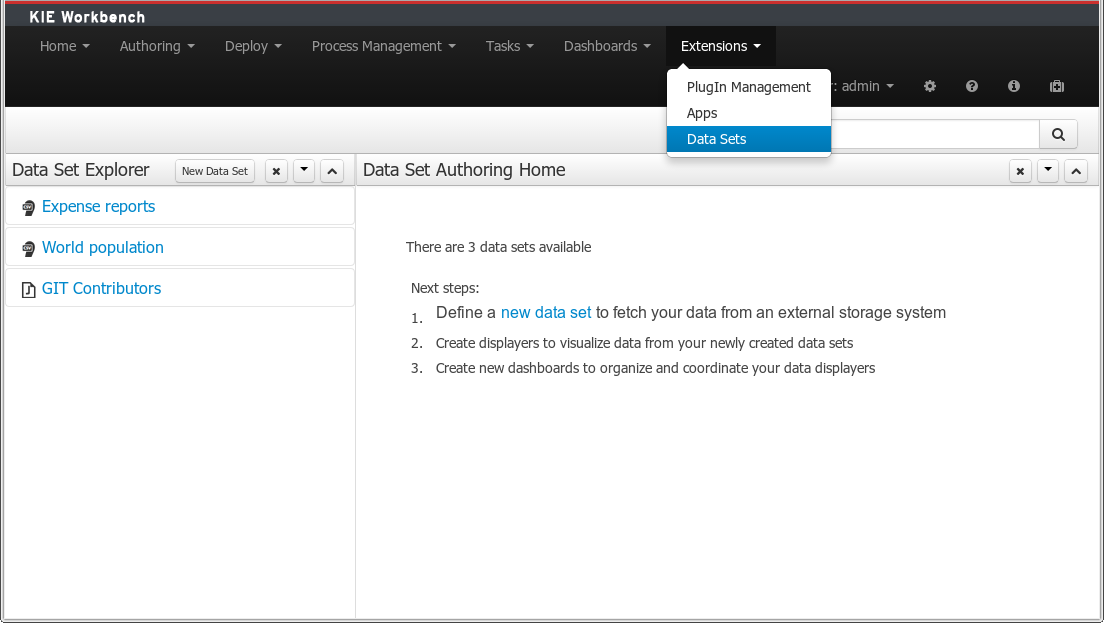
24.38. What is New and Noteworthy in Drools 6.2.0
24.38.1. Propagation modes
The introduction of PHREAK as default algorithm for the Drools engine made the rules' evaluation lazy. This new Drools lazy behavior allowed a relevant performance boost but, in some very specific cases, breaks the semantic of a few Drools features.
More precisely in some circumstances it is necessary to propagate the insertion of new fact into th session immediately. For instance Drools allows a query to be executed in pull only (or passive) mode by prepending a '?' symbol to its invocation as in the following example:
query Q (Integer i)
String( this == i.toString() )
end
rule R when
$i : Integer()
?Q( $i; )
then
System.out.println( $i );
end
In this case, since the query is passive, it shouldn’t react to the insertion of a String matching the join condition in the query itself. In other words this sequence of commands
KieSession ksession = ...
ksession.insert(1);
ksession.insert("1");
ksession.fireAllRules();
shouldn’t cause the rule R to fire because the String satisfying the query condition has been inserted after the Integer and the passive query shouldn’t react to this insertion. Conversely the rule should fire if the insertion sequence is inverted because the insertion of the Integer, when the passive query can be satisfied by the presence of an already existing String, will trigger it.
Unfortunately the lazy nature of PHREAK doesn’t allow the Drools engine to make any distinction regarding the insertion sequence of the two facts, so the rule will fire in both cases. In circumstances like this it is necessary to evaluate the rule eagerly as done by the old RETEOO-based engine.
In other cases it is required that the propagation is eager, meaning that it is not immedate, but anyway has to happen before the Drools engine/agenda starts scheduled evaluations. For instance this is necessary when a rule has the no-loop or the lock-on-active attribute and in fact when this happens this propagation mode is automatically enforced by the Drools engine.
To cover these use cases, and in all other situations where an immediate or eager rule evaluation is required, it is possible to declaratively specify so by annotating the rule itself with @Propagation(Propagation.Type), where Propagation.Type is an enumeration with 3 possible values:
-
IMMEDIATE means that the propagation is performed immediately.
-
EAGER means that the propagation is performed lazily but eagerly evaluated before scheduled evaluations.
-
LAZY means that the propagation is totally lazy and this is default PHREAK behaviour
This means that the following drl:
query Q (Integer i)
String( this == i.toString() )
end
rule R @Propagation(IMMEDIATE) when
$i : Integer()
?Q( $i; )
then
System.out.println( $i );
end
will make the rule R to fire if and only if the Integer is inserted after the String, thus behaving in accordance with the semantic of the passive query.
24.39. New and Noteworthy in KIE Workbench 6.2.0
24.39.1. Download Repository or Part of the Repository as a ZIP
This feature makes it possible to download a repository or a folder from the repository as a ZIP file.
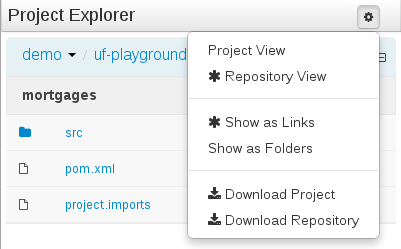
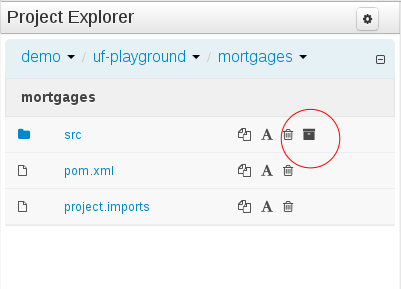
24.39.2. Project Editor permissions
The ability to configure role-based permissions for the Project Editor have been added.
Permissions can be configured using the WEB-INF/classes/workbench-policy.properties
file.
The following permissions are supported:
-
Save button
feature.wb_project_authoring_save
-
Delete button
feature.wb_project_authoring_delete
-
Copy button
feature.wb_project_authoring_copy
-
Rename button
feature.wb_project_authoring_rename
-
Build & Deploy button
feature.wb_project_authoring_buildAndDeploy
24.39.3. Unify validation style in Guided Decision Table Wizard.
All of our new screens use GWT-Bootstrap widgets and alert users to input errors in a consistent way.
One of the most noticable differences was the Guided Decision Table Wizard that alerted errors in a way inconsistent with our use of GWT-Bootstrap.
This Wizard has been updated to use the new look and feel.
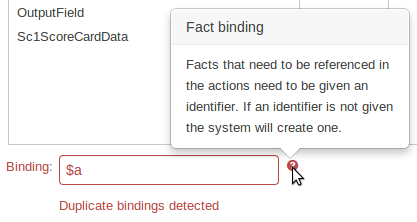
24.39.4. Improved Wizards
During the re-work of the Guided Decision Table’s Wizard to make it’s validation consistent with other areas of the application we took the opportunity to move the Wizard Framework to GWT-Bootstrap too.
The resulting appearance is much more pleasing. We hope to migrate more legacy editors to GWT-Bootstrap as time and priorities permit.
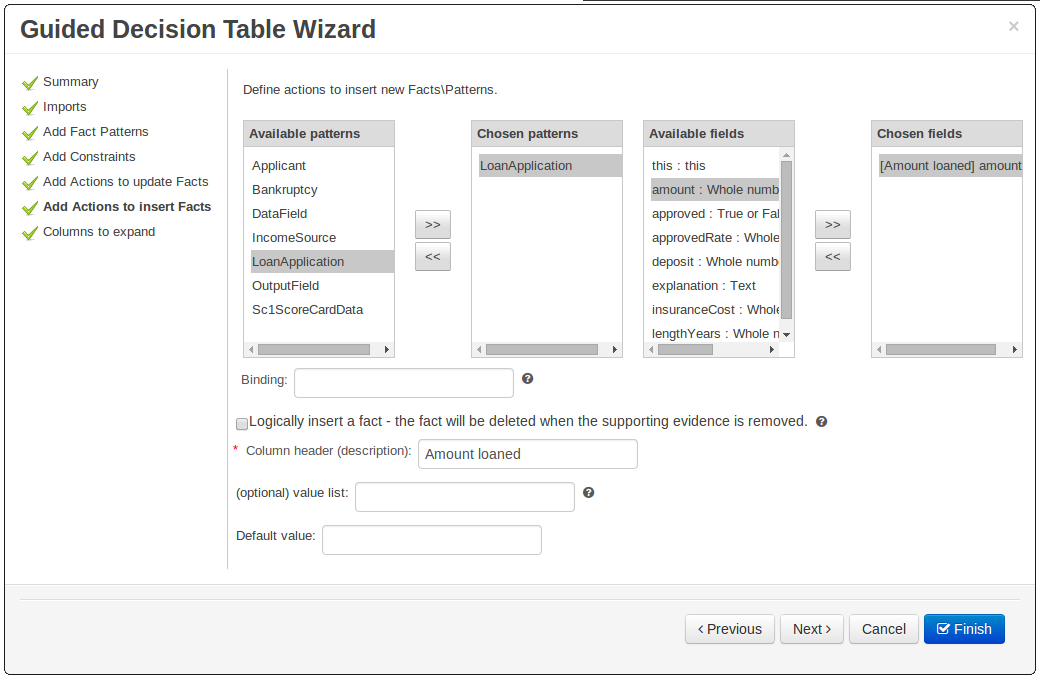
24.39.5. Consistent behaviour of XLS, Guided Decision Tables and Guided Templates
Consistency is a good thing for everybody. Users can expect different authoring metaphores to produce the same rule behaviour (and developers know when something is a bug!).
There were a few inconsistencies in the way XLS Decision Tables, Guidied Decision Tables and Guided Rule Templates generated the underlying rules for empty cells. These have been eliminated making their operation consistent.
-
If all constraints have null values (empty cells) the Pattern is not created.
Should you need the Pattern but no constraints; you will need to include the constraint
this != null
.This operation is consistent with how XLS and Guided Decision Tables have always worked.
-
You can define a constraint on a String field for an empty String or white-space by delimiting it with double-quotation marks. The enclosing quotation-marks are removed from the value when generating the rules.
The use of quotation marks for other String values is not required and they can be omitted. Their use is however essential to differentiate a constraint for an empty String from an empty cell - in which case the constraint is omitted.
24.39.6. Improved Metadata Tab
The Metadata tab provided in previous versions was redesigned to provide a better asset versioning information browsing and recovery. Now every workbench editor will provide an "Overview tab" that will enable the user to manage the following information.
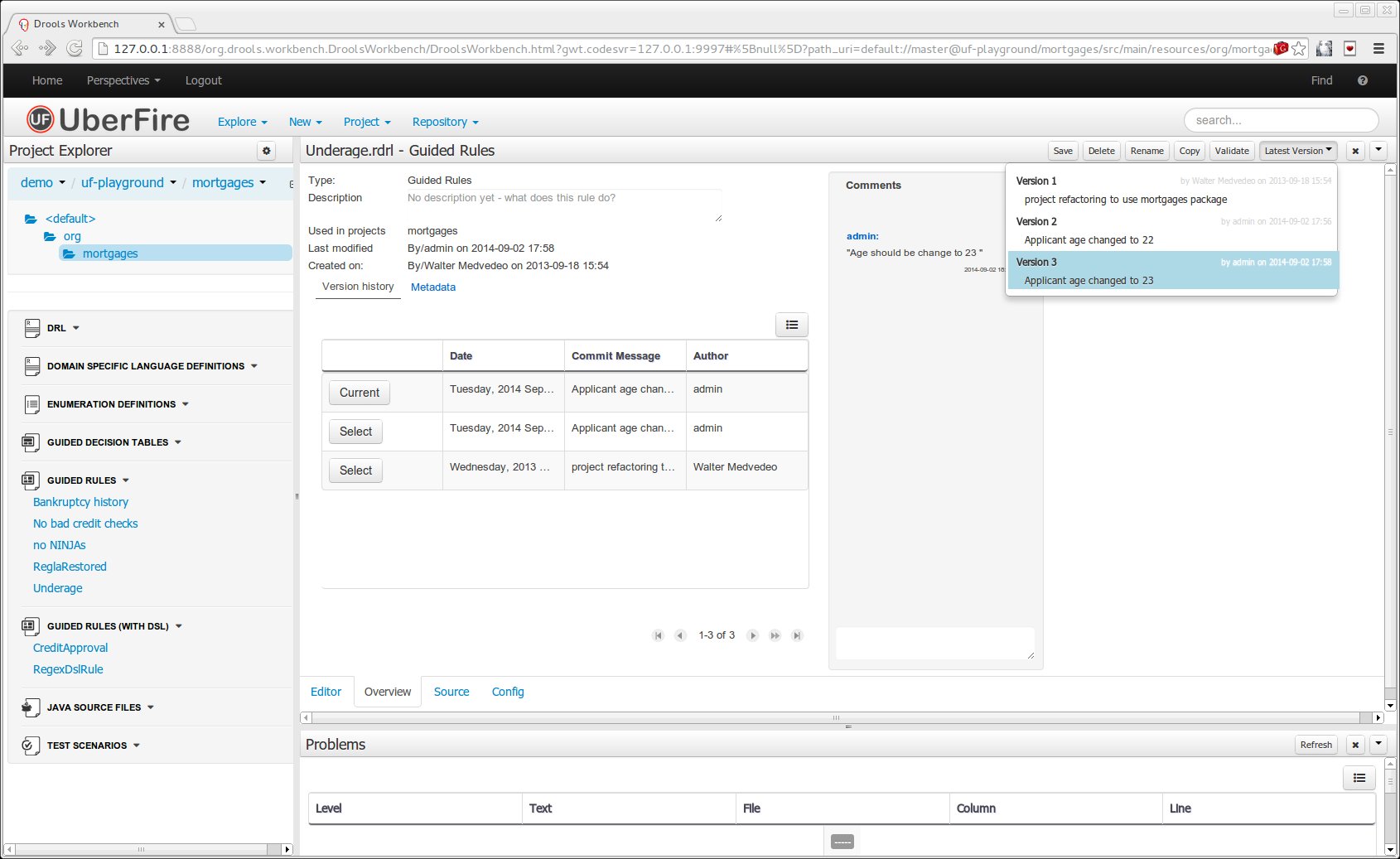
-
Versions history
The versions history shows a tabular view of the asset versions and provides a "Select" button that will enable the user to load a previously created version.
Figure 461. Versions history -
Metadata
The metadata section gets access to additional file attributes.
Figure 462. Metadata section -
Comments area
The redesigned comments area enables much clearer discussions on a file.
-
Version selection dropdown
The "Version selector dropdown" located at the menu bar provides the ability to load and restore previous versions from the "Editor tab", without having to open the "Overview tab" to load the "Version history".
Figure 463. Version selection dropdown
24.39.7. Improved Data Objects Editor
The Java editor was unified to the standard workbench editors functioning. It means that and now every data object is edited on his own editor window.
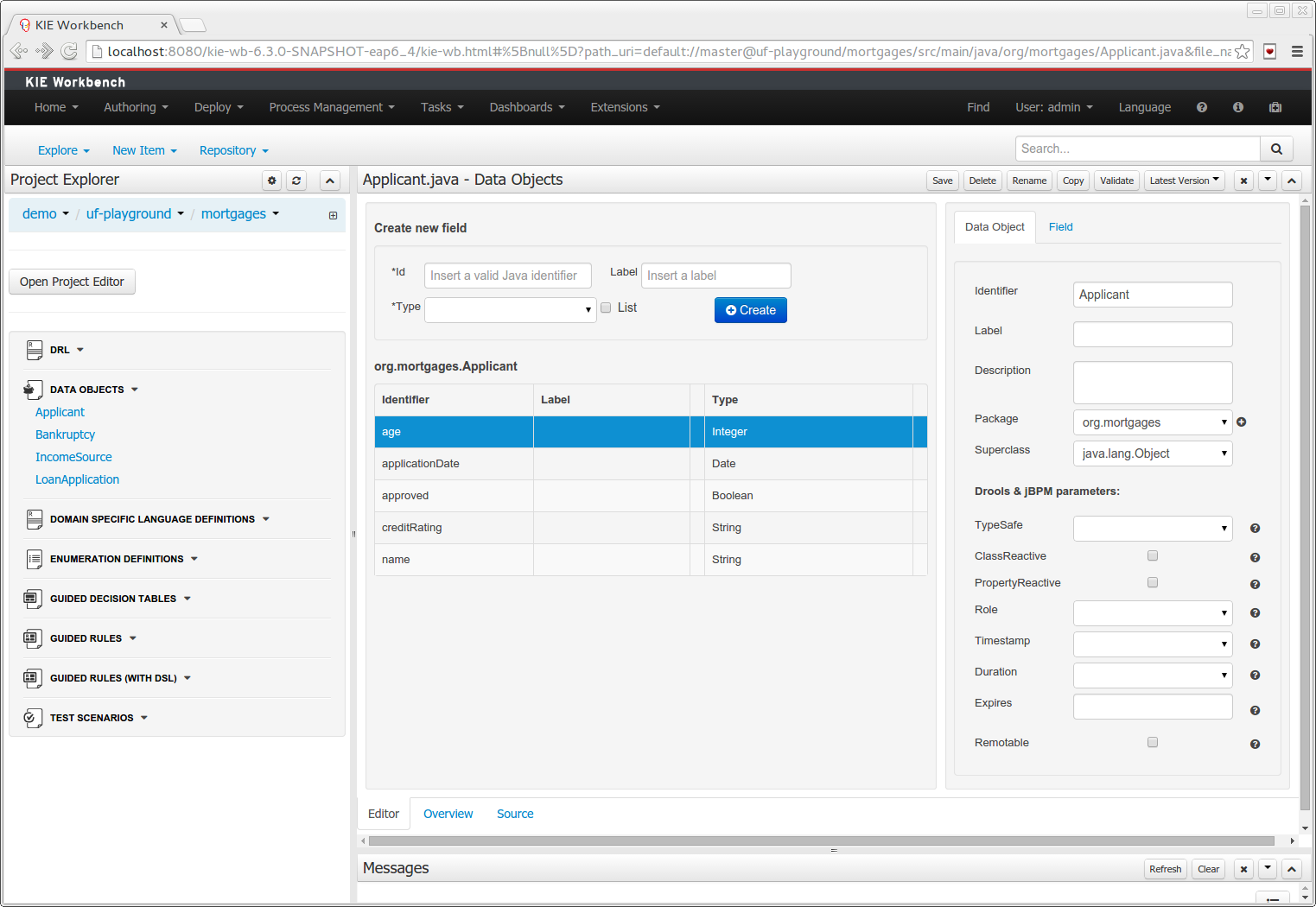
-
"New → Data Object" option was added to create the data objects.
-
Overview tab was added for every file to manage the file metadata and have access to the file versions history.
-
Editable "Source Tab" tab was added. Now the Java code can be modified by administrators using the workbench.
-
"Editor" - "Source Tab" round trip is provided. This will let administrators to do manual changes on the generated Java code and go back to the editor tab to continue working.
-
Class usages detection. Whenever a Data Object is about to be deleted or renamed, the project will be scanned for the class usages. If usages are found (e.g. in drl files, decision tables, etc.) the user will receive an alert. This will prevent the user from breaking the project build.
Figure 465. Usages detection
24.39.8. Execution Server Management UI
A new perspective called Management has been added under Servers top level menu. This perspective provides users the ability to manage multiple execution servers with multiple containers. Available features includes connect to already deployed execution servers; create new, start, stop, delete or upgrade containers.
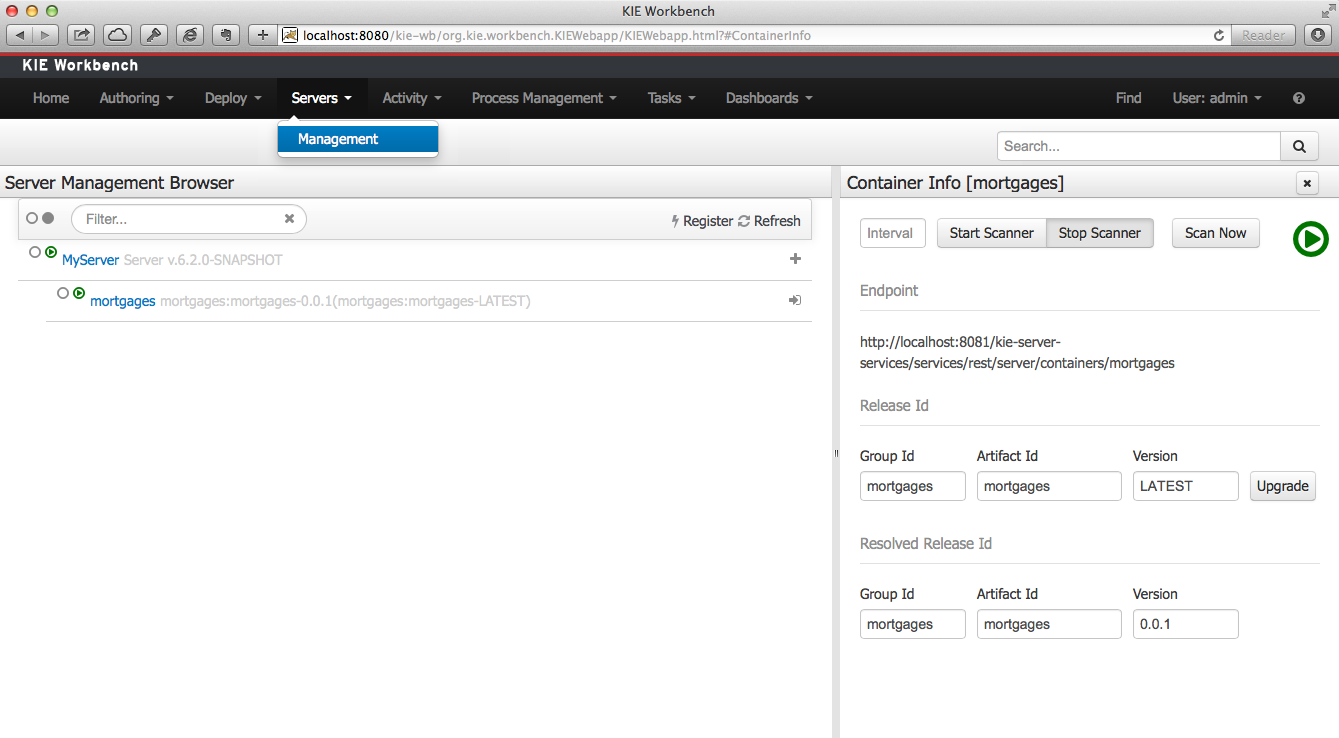
Current version of Execution Server just supports rule based execution. |
24.39.9. Social Activities
A brand new feature called Social Activities has been added under a new top level menu item group called Activity.
This new feature is divided in two different perspectives: Timeline Perspective and People Perspective.
The Timeline Perspective shows on left side the recent assets created or edited by the logged user. In the main window there is the "Latest Changes" screen, showing all the recent updated assets and an option to filter the recent updates by repository.
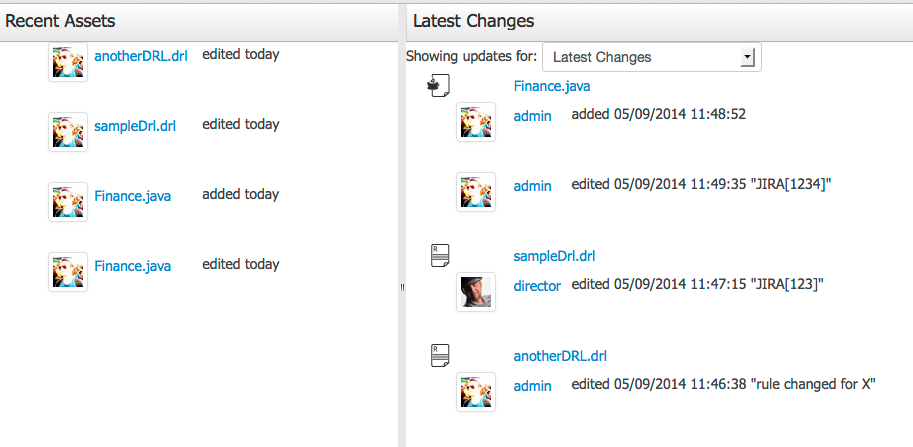
The People Perspective is the home page of an user. Showing his infos (including a gravatar picture from user e-mail), user connections (people that user follow) and user recent activities. There is also a way to edit an user info. The search suggestion can be used to navigate to a user profile, follow him and see his updates on your timeline.
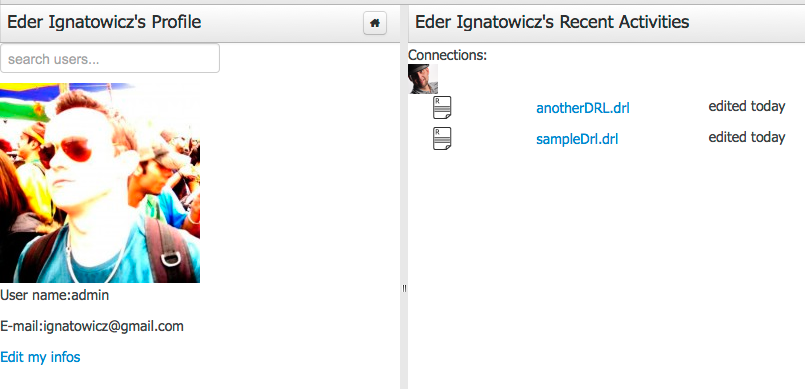
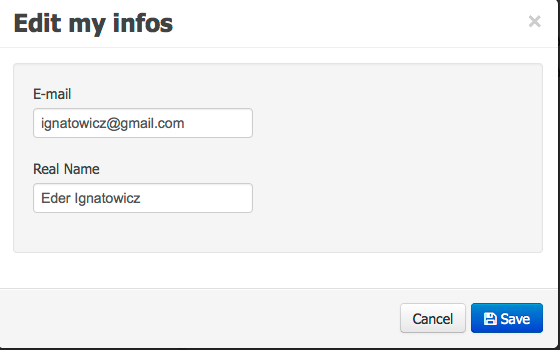
24.39.10. Contributors Dashboard
A brand new perspective called Contributors has been added under a new top level menu item group called Activity. The perspective itself is a dashboard which shows several indicators about the contributions made to the managed organizations / repositories within the workbench. Every time a organization/repository is added/removed from the workbench the dashboard itself is updated accordingly.
This new perspective allows for the monitoring of the underlying activity on the managed repositories.
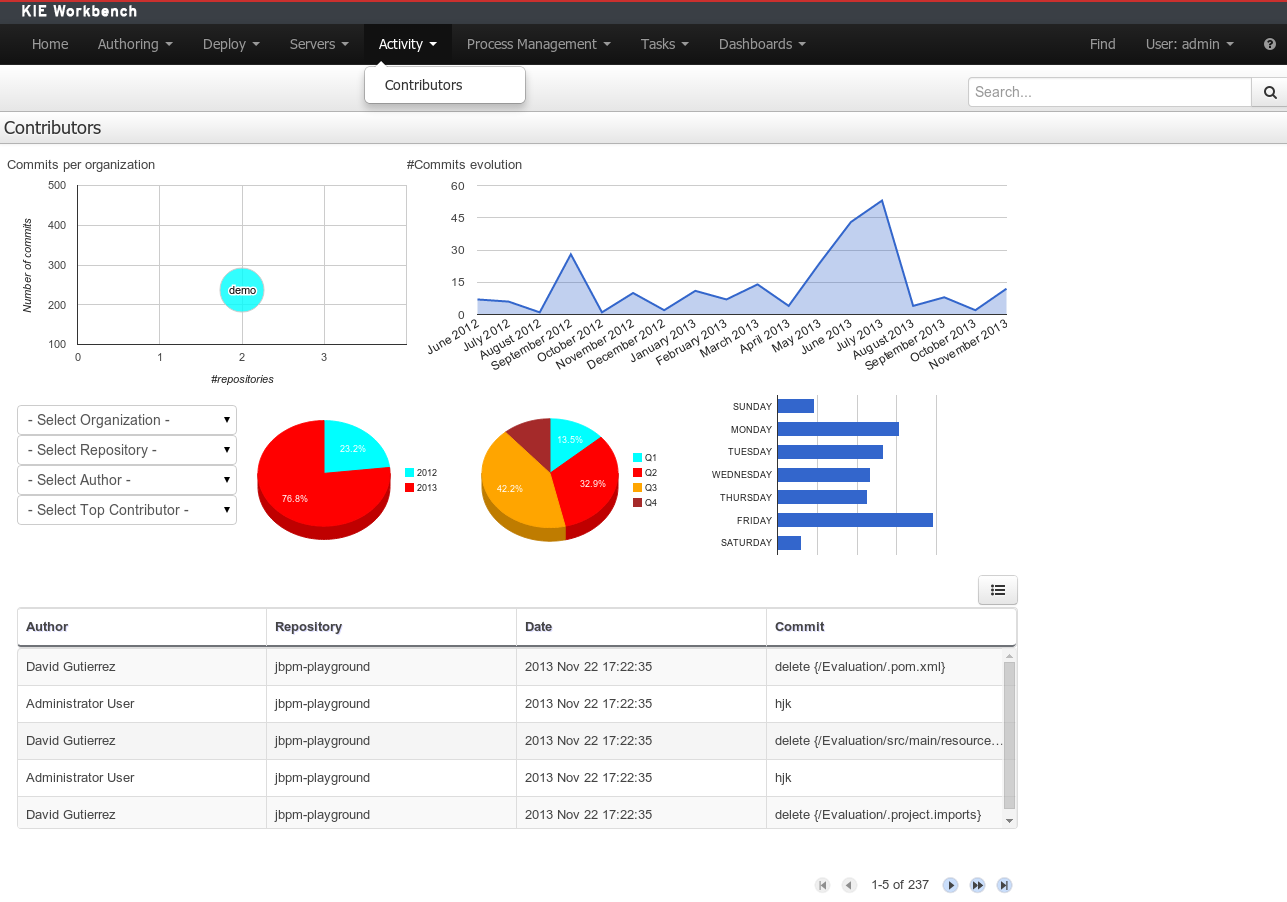
24.39.11. Package selector
The location of new assets whilst authoring was driven by the context of the Project Explorer.
This has been replaced with a Package Selector in the New Resource Popup.
The location defaults to the Project Explorer context but different packages can now be more easily chosen.
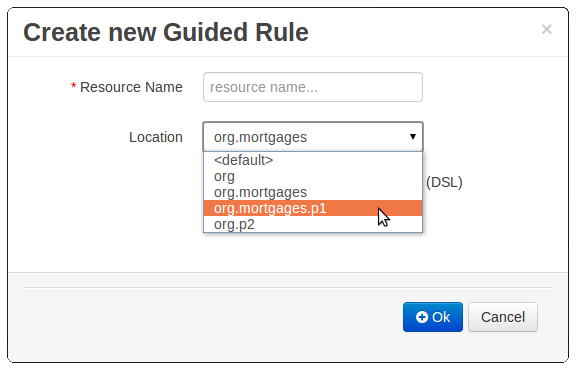
24.39.12. Improved visual consistency
All Popups have been refactored to use GWT-Bootstrap widgets.
Whilst a simple change it brings greater visual consistency to the application as a whole.
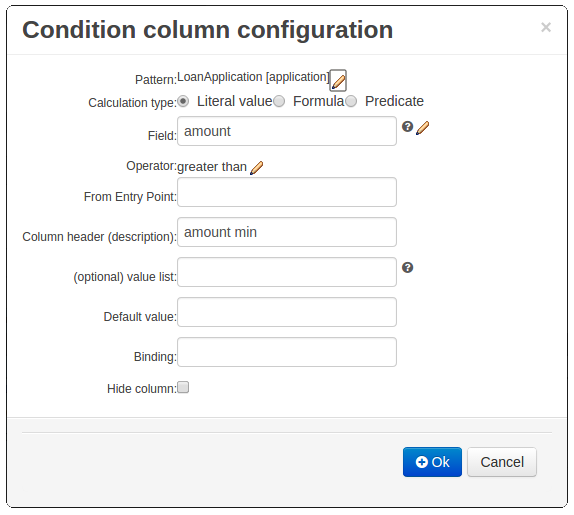
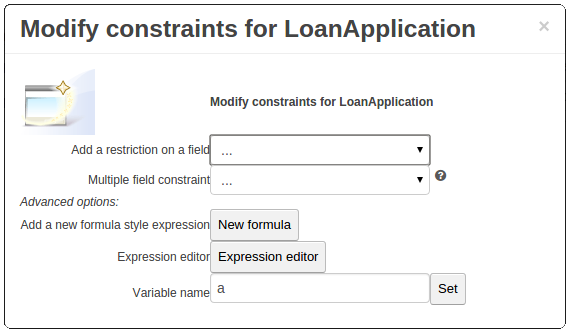
24.39.13. Guided Decision Tree Editor
A new editor has been added to support modelling of simple decision trees.
See the applicable section within the User Guide for more information about usage.
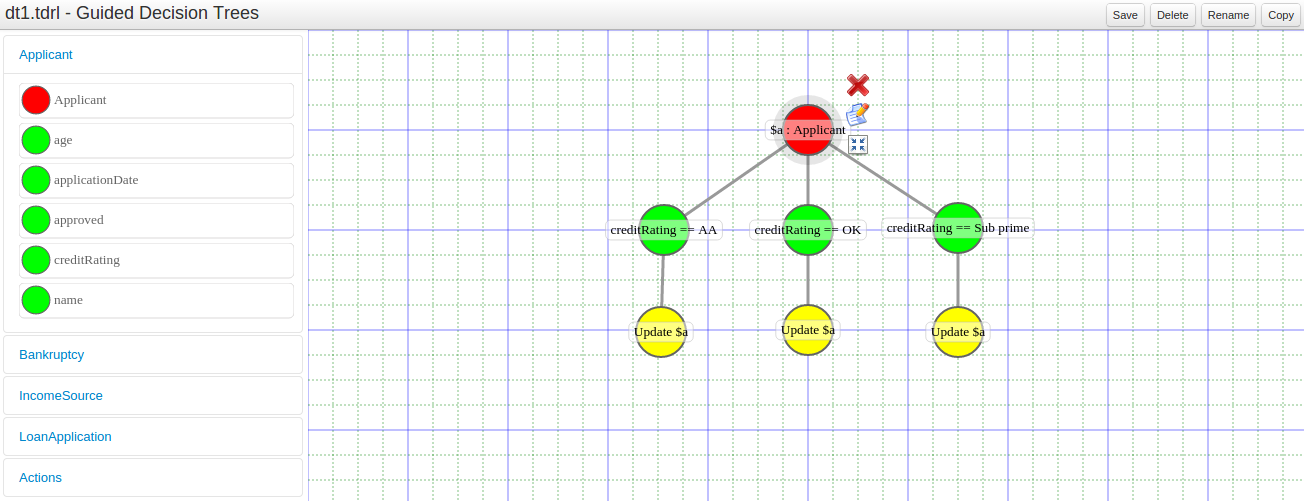
24.39.14. Create Repository Wizard
A wizard has been created to guide the repository creation process. Now the user can decide at repository creation time if it should be a managed or unmanaged repository and configure all related parameters.
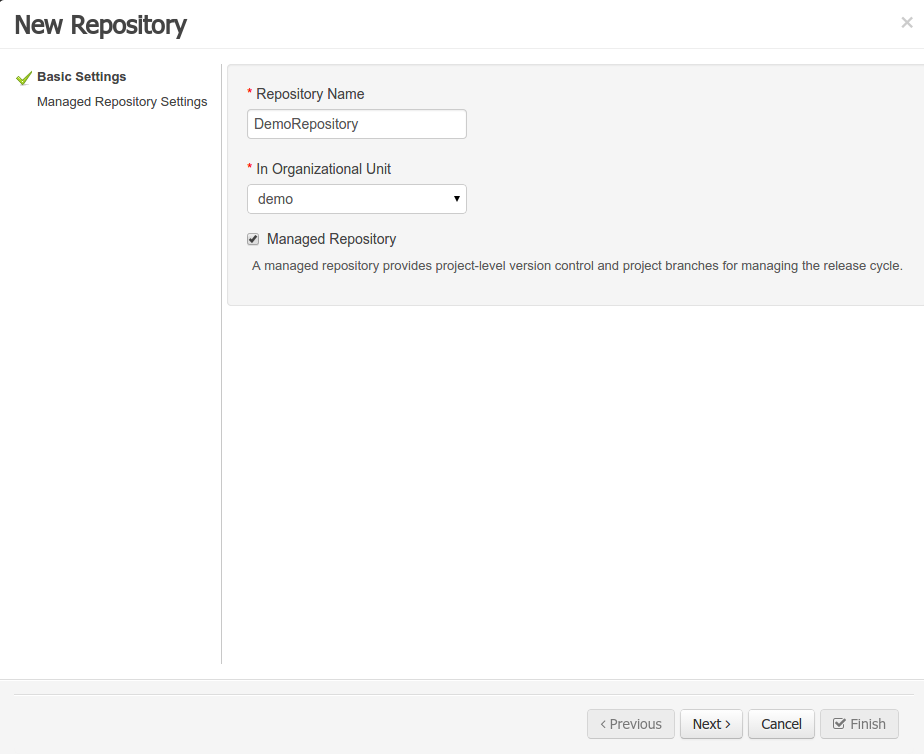
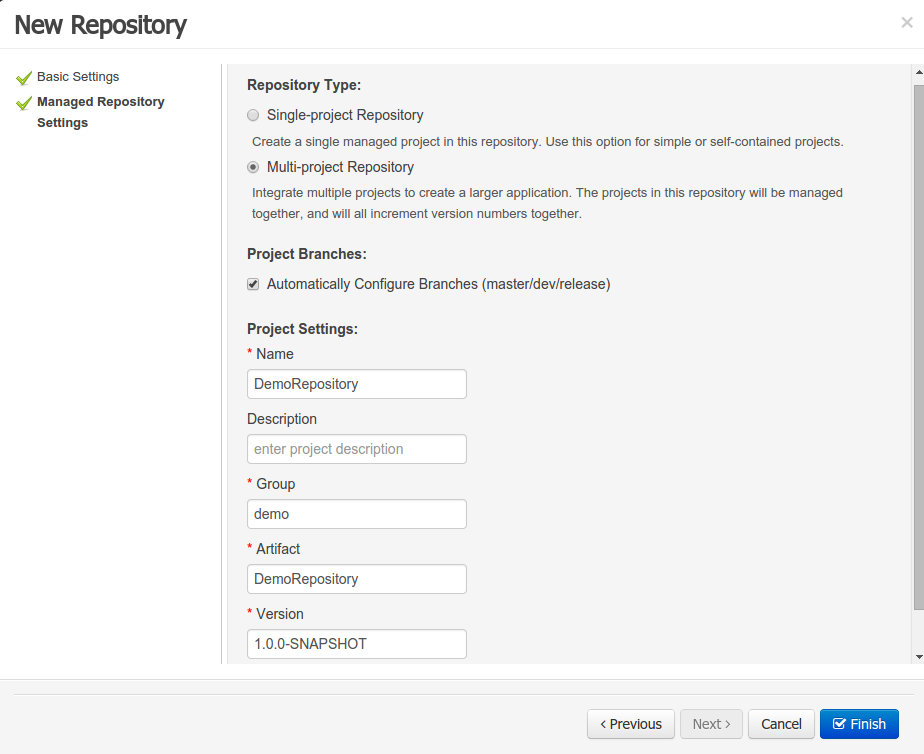
24.39.15. Repository Structure Screen
The new Repository Structure Screen will let users to manage the projects for a given repository, as well as other operations related to managed repositories like: branch creation, assets promotion and project release.
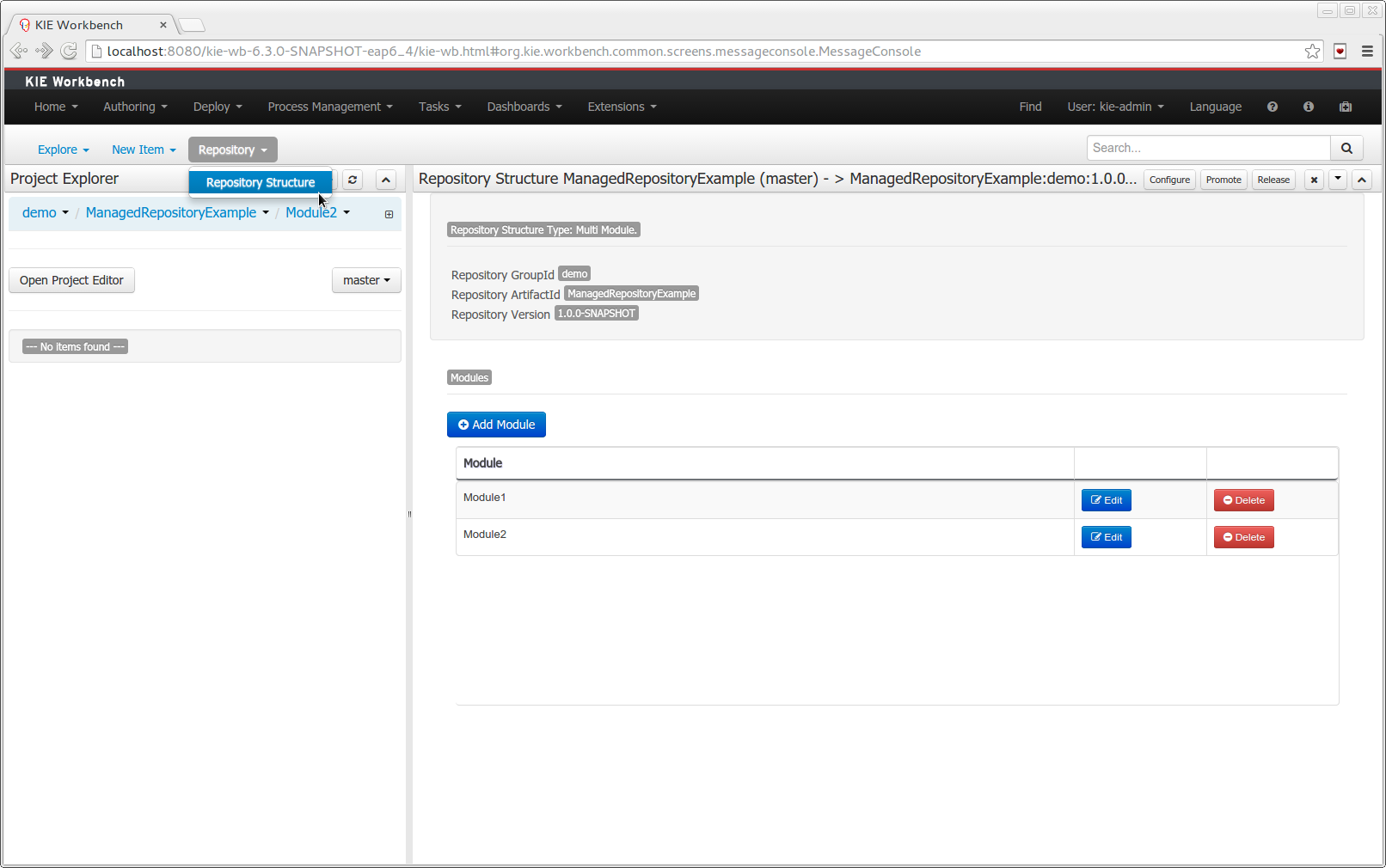
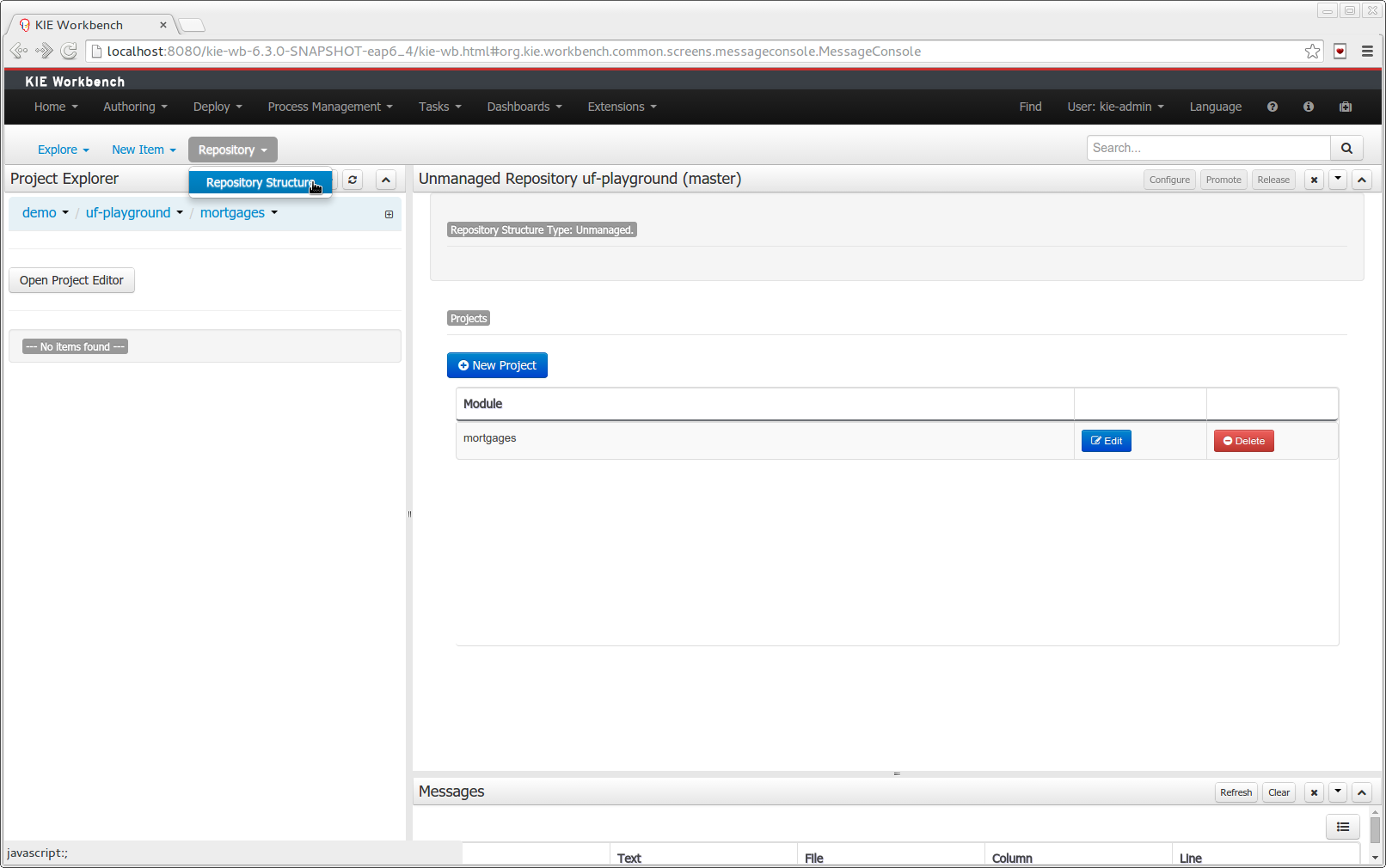
24.40. New and Noteworthy in Integration 6.2.0
24.40.1. KIE Execution Server
A new KIE Execution Server was created with the goal of supporting the deployment of kjars and the automatic creation of REST endpoints for remote rules execution. This initial implementation supports provisioning and execution of kjars via REST without any glue code.
A user interface was also integrated into the workbench for remote provisioning. See the workbench’s New&Noteworthy for details.
@Path("/server")
public interface KieServer {
@GET
@Produces({MediaType.APPLICATION_XML, MediaType.APPLICATION_JSON})
public Response getInfo();
@POST
@Consumes({MediaType.APPLICATION_XML, MediaType.APPLICATION_JSON})
@Produces({MediaType.APPLICATION_XML, MediaType.APPLICATION_JSON})
public Response execute( CommandScript command );
@GET
@Path("containers")
@Produces({MediaType.APPLICATION_XML, MediaType.APPLICATION_JSON})
public Response listContainers();
@GET
@Path("containers/{id}")
@Produces({MediaType.APPLICATION_XML, MediaType.APPLICATION_JSON})
public Response getContainerInfo( @PathParam("id") String id );
@PUT
@Path("containers/{id}")
@Consumes({MediaType.APPLICATION_XML, MediaType.APPLICATION_JSON})
@Produces({MediaType.APPLICATION_XML, MediaType.APPLICATION_JSON})
public Response createContainer( @PathParam("id") String id, KieContainerResource container );
@DELETE
@Path("containers/{id}")
@Produces({MediaType.APPLICATION_XML, MediaType.APPLICATION_JSON})
public Response disposeContainer( @PathParam("id") String id );
@POST
@Path("containers/{id}")
@Consumes({MediaType.APPLICATION_XML, MediaType.APPLICATION_JSON})
@Produces({MediaType.APPLICATION_XML, MediaType.APPLICATION_JSON})
public Response execute( @PathParam("id") String id, String cmdPayload );
@GET
@Path("containers/{id}/release-id")
@Produces({MediaType.APPLICATION_XML, MediaType.APPLICATION_JSON})
public Response getReleaseId( @PathParam("id") String id);
@POST
@Path("containers/{id}/release-id")
@Consumes({MediaType.APPLICATION_XML, MediaType.APPLICATION_JSON})
@Produces({MediaType.APPLICATION_XML, MediaType.APPLICATION_JSON})
public Response updateReleaseId( @PathParam("id") String id, ReleaseId releaseId );
@GET
@Path("containers/{id}/scanner")
@Produces({MediaType.APPLICATION_XML, MediaType.APPLICATION_JSON})
public Response getScannerInfo( @PathParam("id") String id );
@POST
@Path("containers/{id}/scanner")
@Consumes({MediaType.APPLICATION_XML, MediaType.APPLICATION_JSON})
@Produces({MediaType.APPLICATION_XML, MediaType.APPLICATION_JSON})
public Response updateScanner( @PathParam("id") String id, KieScannerResource resource );
}
24.41. What is New and Noteworthy in Drools 6.1.0
24.41.1. JMX support for KieScanner
Added support for JMX monitoring and management on KieScanner and KieContainer.
To enable, set the property kie.scanner.mbeans
to enabled
, for example via Java command line: -Dkie.scanner.mbeans=enabled
.
KieScannerMBean will register under the name:
It exposes the following properties:
-
Scanner Release Id: the release ID the scanner was configured with. May include maven range versions and special keywords like LATEST, SNAPSHOT, etc.
-
Current Release Id: the actual release ID the artifact resolved to.
-
Status: STARTING, SCANNING, UPDATING, RUNNING, STOPPED, SHUTDOWN
It also exposes the following operations:
-
scanNow(): forces an immediate scan of the maven repository looking for artifact updates
-
start(): starts polling the maven repository for artifact updates based on the polling interval parameter
-
stop(): stops automatically polling the maven repository
24.42. New and Noteworthy in KIE Workbench 6.1.0
24.42.1. Data Modeler - round trip and source code preservation
Full round trip between Data modeler and Java source code is now supported. No matter where the Java code was generated (e.g. Eclipse, Data modeller), data modeler will only update the necessary code blocks to maintain the model updated.
24.42.2. Data Modeler - improved annotations
New annotations @TypeSafe, @ClassReactive, @PropertyReactive, @Timestamp, @Duration and @Expires were added in order enrich current Drools annotations manged by the data modeler.
24.42.3. Standardization of the display of tabular data
We have standardized the display of tabular data with a new table widget.
The new table supports the following features:
-
Selection of visible columns
-
Resizable columns
-
Moveable columns
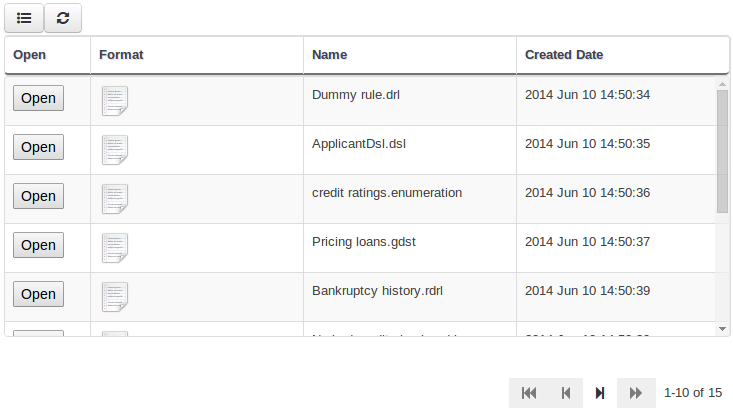
The table is used in the following scenarios:
-
Inbox (Incoming changes)
-
Inbox (Recently edited)
-
Inbox (Recently opened)
-
Project Problems summary
-
Artifact Repository browser
-
Project Editor Dependency grid
-
Project Editor KSession grid
-
Project Editor Work Item Handlers Configuration grid
-
Project Editor Listeners Configuration grid
-
Search Results grid
24.42.4. Generation of modify(x) {…}
blocks
The Guided Rule Editor, Guided Template Editor and Guided Decision Table Editor have been changed to generate modify(x){…}
Historically these editors supported the older update(x)
syntax and hence rules created within the Workbench would not respond correctly to @PropertyReactive
and associated annotations within a model.
This has now been rectified with the use of modify(x){…}
blocks.
24.43. New and Noteworthy in KIE API 6.0.0
24.43.1. New KIE name
KIE is the new umbrella name used to group together our related projects; as the family continues to grow. KIE is also used for the generic parts of unified API; such as building, deploying and loading. This replaces the kiegroup and knowledge keywords that would have been used before.
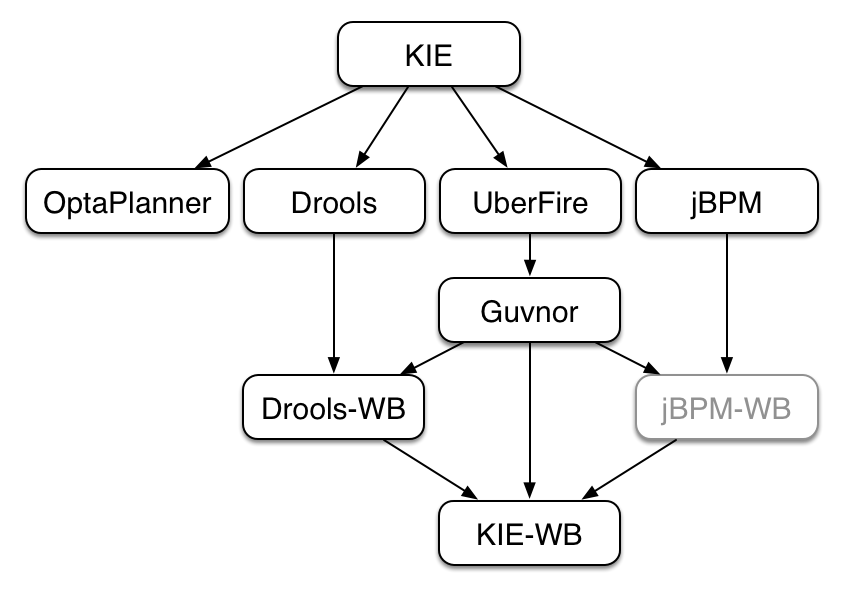
24.43.2. Maven aligned projects and modules and Maven Deployment
One of the biggest complaints during the 5.x series was the lack of defined methodology for deployment. The mechanism used by Drools and jBPM was very flexible, but it was too flexible. A big focus for 6.0 was streamlining the build, deploy and loading (utilization) aspects of the system. Building and deploying activities are now aligned with Maven and Maven repositories. The utilization for loading rules and processess is now convention and configuration oriented, instead of programmatic, with sane defaults to minimise the configuration.
Projects can be built with Maven and installed to the local M2_REPO or remote Maven repositories. Maven is then used to declare and build the classpath of dependencies, for KIE to access.
24.43.3. Configuration and convention based projects
The 'kmodule.xml' provides declarative configuration for KIE projects. Conventions and defaults are used to reduce the amount of configuration needed.
<kmodule xmlns="http://www.drools.org/xsd/kmodule">
<kbase name="kbase1" packages="org.mypackages">
<ksession name="ksession1"/>
</kbase>
</kmodule>
KieServices ks = KieServices.Factory.get();
KieContainer kContainer = ks.getKieClasspathContainer();
KieSession kSession = kContainer.newKieSession("ksession1");
kSession.insert(new Message("Dave", "Hello, HAL. Do you read me, HAL?"));
kSession.fireAllRules();
24.43.4. KieBase Inclusion
It is possible to include all the KIE artifacts belonging to a KieBase into a second KieBase. This means that the second KieBase, in addition to all the rules, function and processes directly defined into it, will also contain the ones created in the included KieBase. This inclusion can be done declaratively in the kmodule.xml file
<kmodule xmlns="http://www.drools.org/xsd/kmodule">
<kbase name="kbase2" includes="kbase1">
<ksession name="ksession2"/>
</kbase>
</kmodule>
or programmatically using the KieModuleModel
.
KieModuleModel kmodule = KieServices.Factory.get().newKieModuleModel();
KieBaseModel kieBaseModel1 = kmodule.newKieBaseModel("KBase2").addInclude("KBase1");
24.43.5. KieModules, KieContainer and KIE-CI
Any Maven produced JAR with a 'kmodule.xml' in it is considered a KieModule. This can be loaded from the classpath or dynamically at runtime from a Resource location. If the kie-ci dependency is on the classpath it embeds Maven and all resolving is done automatically using Maven and can access local or remote repositories. Settings.xml is obeyed for Maven configuration.
The KieContainer provides a runtime to utilize the KieModule, versioning is built in throughout, via Maven. Kie-ci will create a classpath dynamically from all the Maven declared dependencies for the artifact being loaded. Maven LATEST, SNAPSHOT, RELEASE and version ranges are supported.
KieServices ks = KieServices.Factory.get();
KieContainer kContainer = ks.newKieContainer(
ks.newReleaseId("org.mygroup", "myartefact", "1.0") );
KieSession kSession = kContainer.newKieSession("ksession1");
kSession.insert(new Message("Dave", "Hello, HAL. Do you read me, HAL?"));
kSession.fireAllRules();
KieContainers can be dynamically updated to a specific version, and resolved through Maven if KIE-CI is on the classpath. For stateful KieSessions the existing sessions are incrementally updated.
KieContainer kContainer.updateToVersion(
ks.newReleaseId("org.mygroup", "myartefact", "1.1") );
24.43.6. KieScanner
The KieScanner
is a Maven-oriented replacement of the KnowledgeAgent present in Drools 5.
It continuously monitors your Maven repository to check if a new release of a Kie project has been installed and if so, deploys it in the KieContainer
wrapping that project.
The use of the KieScanner
requires kie-ci.jar to be on the classpath.
A KieScanner
can be registered on a KieContainer
as in the following example.
KieServices kieServices = KieServices.Factory.get();
ReleaseId releaseId = kieServices.newReleaseId( "org.acme", "myartifact", "1.0-SNAPSHOT" );
KieContainer kContainer = kieServices.newKieContainer( releaseId );
KieScanner kScanner = kieServices.newKieScanner( kContainer );
// Start the KieScanner polling the Maven repository every 10 seconds
kScanner.start( 10000L );
In this example the KieScanner
is configured to run with a fixed time interval, but it is also possible to run it on demand by invoking the scanNow()
method on it.
If the KieScanner
finds, in the Maven repository, an updated version of the Kie project used by that KieContainer
it automatically downloads the new version and triggers an incremental build of the new project.
From this moment all the new KieBase
s and KieSession
s created from that KieContainer
will use the new project version.
24.43.7. Hierarchical ClassLoader
The CompositeClassLoader is no longer used; as it was a constant source of performance problems and bugs. Traditional hierarchical classloaders are now used. The root classloader is at the KieContext level, with one child ClassLoader per namespace. This makes it cleaner to add and remove rules, but there can now be no referencing between namespaces in DRL files; i.e. functions can only be used by the namespaces that declared them. The recommendation is to use static Java methods in your project, which is visible to all namespaces; but those cannot (like other classes on the root KieContainer ClassLoader) be dynamically updated.
24.43.8. Legacy API Adapter
The 5.x API for building and running with Drools and jBPM is still available through Maven dependency "knowledge-api-legacy5-adapter". Because the nature of deployment has significantly changed in 6.0, it was not possible to provide an adapter bridge for the KnowledgeAgent. If any other methods are missing or problematic, please open a JIRA, and we’ll fix for 6.1
24.43.9. KIE Documentation
While a lot of new documentation has been added for working with the new KIE API, the entire documentation has not yet been brought up to date. For this reason there will be continued references to old terminologies. Apologies in advance, and thank you for your patience. We hope those in the community will work with us to get the documentation updated throughout, for 6.1
24.44. What is New and Noteworthy in Drools 6.0.0
24.44.1. PHREAK - Lazy rule matching algorithm
The main work done for Drools in 6.0 involves the new PREAK algorithm. This is a lazy algorithm that should enable Drools to handle a larger number of rules and facts. AngendaGroups can now help improvement performance, as rules are not evaluated until it attempts to fire them.
Sequential mode continues to be supported for PHREAK but now 'modify' is allowed. While there is no 'inference' with sequential configuration, as rules are lazily evaluated, any rule not yet evaluated will see the more recent data as a result of 'modify'. This is more inline with how people intuitively think sequential works.
The conflict resolution order has been tweaked for PHREAK, and now is ordered by salience and then rule order; based on the rule position in the file.. Prior to Drools 6.0.0, after salience, it was considered arbitrary. When KieModules and updateToVersion are used for dynamic deployment, the rule order in the file is preserved via the diff processing.
24.44.2. Automatically firing timed rule in passive mode
When the Drools engine runs in passive mode (i.e.: using fireAllRules) by default it doesn’t fire consequences of timed rules unless fireAllRules isn’t invoked again.
Now it is possible to change this default behavior by configuring the KieSession with a TimedRuleExectionOption
as shown in the following example.
KieSessionConfiguration ksconf = KieServices.Factory.get().newKieSessionConfiguration();
ksconf.setOption( TimedRuleExectionOption.YES );
KSession ksession = kbase.newKieSession(ksconf, null);
It is also possible to have a finer grained control on the timed rules that have to be automatically executed.
To do this it is necessary to set a `FILTERED`TimedRuleExectionOption
that allows to define a callback to filter those rules, as done in the next example.
KieSessionConfiguration ksconf = KieServices.Factory.get().newKieSessionConfiguration();
conf.setOption( new TimedRuleExectionOption.FILTERED(new TimedRuleExecutionFilter() {
public boolean accept(Rule[] rules) {
return rules[0].getName().equals("MyRule");
}
}) );
24.44.3. Expression Timers
It is now possible to define both the delay and interval of an interval timer as an expression instead of a fixed value. To do that it is necessary to declare the timer as an expression one (indicated by "expr:") as in the following example:
declare Bean
delay : String = "30s"
period : long = 60000
end
rule "Expression timer"
timer( expr: $d, $p )
when
Bean( $d : delay, $p : period )
then
end
The expressions, $d and $p in this case, can use any variable defined in the pattern matching part of the rule and can be any String that can be parsed in a time duration or any numeric value that will be internally converted in a long representing a duration expressed in milliseconds.
Both interval and expression timers can have 3 optional parameters named "start", "end" and "repeat-limit". When one or more of these parameters are used the first part of the timer definition must be followed by a semicolon ';' and the parameters have to be separated by a comma ',' as in the following example:
timer (int: 30s 10s; start=3-JAN-2010, end=5-JAN-2010)
The value for start and end parameters can be a Date, a String representing a Date or a long, or more in general any Number, that will be transformed in a Java Date applying the following conversion:
new Date( ((Number) n).longValue() )
Conversely the repeat-limit can be only an integer and it defines the maximum number of repetitions allowed by the timer. If both the end and the repeat-limit parameters are set the timer will stop when the first of the two will be matched.
The using of the start parameter implies the definition of a phase for the timer, where the beginning of the phase is given by the start itself plus the eventual delay. In other words in this case the timed rule will then be scheduled at times:
start + delay + n*period
for up to repeat-limit times and no later than the end timestamp (whichever first). For instance the rule having the following interval timer
timer ( int: 30s 1m; start="3-JAN-2010" )
will be scheduled at the 30th second of every minute after the midnight of the 3-JAN-2010. This also means that if for example you turn the system on at midnight of the 3-FEB-2010 it won’t be scheduled immediately but will preserve the phase defined by the timer and so it will be scheduled for the first time 30 seconds after the midnight. If for some reason the system is paused (e.g. the session is serialized and then deserialized after a while) the rule will be scheduled only once to recover from missing activations (regardless of how many activations we missed) and subsequently it will be scheduled again in phase with the timer.
24.44.4. RuleFlowGroups and AgendaGroups are merged
These two groups have been merged and now RuleFlowGroups behave the same as AgendaGroups. The get methods have been left, for deprecation reasons, but both return the same underlying data. When jBPM activates a group it now just calls setFocus. RuleFlowGroups and AgendaGroups when used together was a continued source of errors. It also aligns the codebase, towards PHREAK and the multi-core explotation that is planned in the future.
24.45. New and Noteworthy in KIE Workbench 6.0.0
The workbench has had a big overhaul using a new base project called UberFire. UberFire is inspired by Eclipse and provides a clean, extensible and flexible framework for the workbench. The end result is not only a richer experience for our end users, but we can now develop more rapidly with a clean component based architecture. If you like he Workbench experience you can use UberFire today to build your own web based dashboard and console efforts.
As well as the move to a UberFire the other biggest change is the move from JCR to Git; there is an utility project to help with migration. Git is the most scalable and powerful source repository bar none. JGit provides a solid OSS implementation for Git. This addresses the continued performance problems with the various JCR implementations, which would slow down once the number of files and number of versions become too high. There has been a big "low tech" drive, to remove complexity. Everything is now stored as a file, including meta data. The database is only there to provide fast indexing and search. So importing and exporting is all standard Git and external sites, like GitHub, can be used to exchange repositories.
In 5.x developers would work with their own source repository and then push JCR, via the team provider. This team provider was not full featured and not available outside Eclipse. Git enables our repository to work any existing Git tool or team provider. While not yet supported in the UI, this will be added over time, it is possible to connect to the repo and tag and branch and restore things.
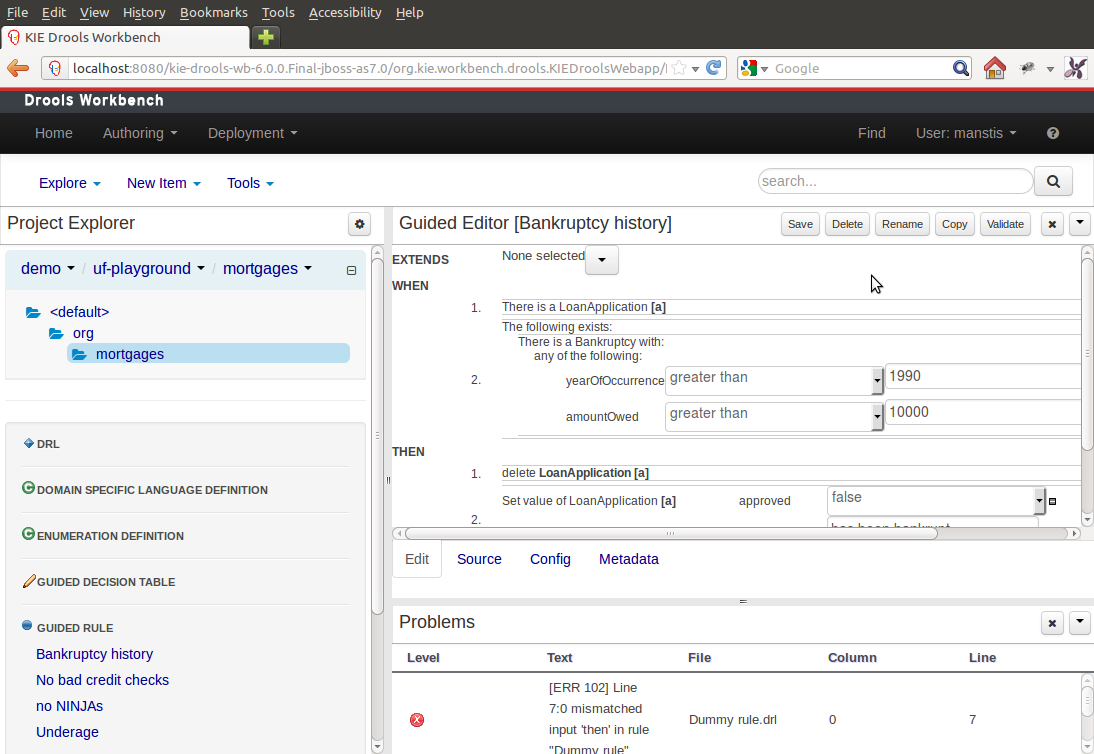
The Guvnor brand leaked too much from its intended role; such as the authoring metaphors, like Decision Tables, being considered Guvnor components instead of Drools components. This wasn’t helped by the monolithic projects structure used in 5.x for Guvnor. In 6.0 Guvnor 's focus has been narrowed to encapsulates the set of UberFire plugins that provide the basis for building a web based IDE. Such as Maven integration for building and deploying, management of Maven repositories and activity notifications via inboxes. Drools and jBPM build workbench distributions using Uberfire as the base and including a set of plugins, such as Guvnor, along with their own plugins for things like decision tables, guided editors, BPMN2 designer, human tasks.
The "Model Structure" diagram outlines the new project anatomy. The Drools workbench is called KIE-Drools-WB. KIE-WB is the uber workbench that combines all the Guvnor, Drools and jBPM plugins. The jBPM-WB is ghosted out, as it doesn’t actually exist, being made redundant by KIE-WB.
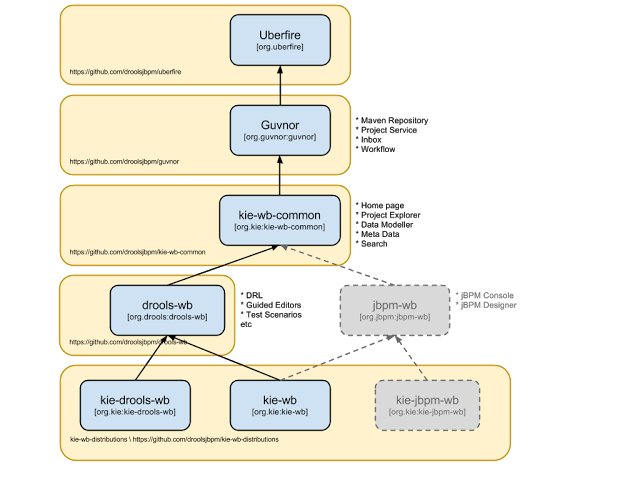
KIE Drools Workbench and KIE Workbench share a common set of components for generic workbench functionality such as Project navigation, Project definitions, Maven based Projects, Maven Artifact Repository. These common features are described in more detail throughout this documentation. |
The two primary distributions consist of:
-
KIE Drools Workbench
-
Drools Editors, for rules and supporting assets.
-
jBPM Designer, for Rule Flow and supporting assets.
-
-
KIE Workbench
-
Drools Editors, for rules and supporting assets.
-
jBPM Designer, for BPMN2 and supporting assets.
-
Business Central, runtime and Human Task support.
-
jBPM Form Builder.
-
BAM.
-
Workbench highlights:
-
New flexible Workbench environment, with perspectives and panels.
-
New packaging and build system following KIE API.
-
Maven based projects.
-
Maven Artifact Repository replaces Global Area, with full dependency support.
-
-
New Data Modeller replaces the declarative Fact Model Editor; bringing authoring of Java classes to the authoring environment. Java classes are packaged into the project and can be used within rules, processes etc and externally in your own applications.
-
Virtual File System replaces JCR with a default Git based implementation.
-
Default Git based implementation supports remote operations.
-
External modifications appear within the Workbench.
-
-
Incremental Build system showing, near real-time validation results of your project and assets.
The editors themselves are largely unchanged; however of note imports have moved from the package definition to individual editors so you need only import types used for an asset and not the package as a whole.
24.46. New and Noteworthy in Integration 6.0.0
24.46.1. CDI
@Inject
@KSession("kbase1")
@KReleaseId( groupId = "jar1", rtifactId = "art1", version = "1.0")
private KieBase kbase1v10;
@Inject
@KBase("kbase1")
@KReleaseId( groupId = "jar1", rtifactId = "art1", version = "1.1")
private KieBase kbase1v10;
@Inject
@KSession("ksession1")
@KReleaseId( groupId = "jar1", rtifactId = "art1", version = "1.0")
private KieSession ksessionv10;
@Inject
@KSession("ksession1")
@KReleaseId( groupId = "jar1", rtifactId = "art1", version = "1.1")
private KieSession ksessionv11;
CDI is now tightly integrated into the KIE API. It can be used to inject versioned KieSession and KieBases.
24.46.2. Spring
Spring has been revamped and now integrated with KIE. Spring can replace the 'kmodule.xml' with a more powerful spring version. The aim is for consistency with kmodule.xml
24.46.3. Aries Blueprints
Aries blueprints is now also supported, and follows the work done for spring. The aim is for consistency with spring and kmodule.xml
24.46.4. OSGi Ready
All modules have been refactored to avoid package splitting, which was a problem in 5.x. Testing has been moved to PAX.